Identification and validation of a glycolysis-related gene signature for predicting metastasis and survival rate in patients with thyroid cancer
Highlight box
Key findings
• A 3-gene (HSPA5, KIF20A and SDC2) signature was identified and used to predict metastasis and survival rate in patients with thyroid cancer (THCA).
What is known and what is new?
• Glycolysis is known to play an important role in the development and metastasis of THCA.
• The study reported a three-gene signature of THCA, which were found to be closely correlated with the glycolysis of THCA, and it showed a high efficacy to the prediction of metastasis and survival rate of THCA.
What is the implication, and what should change now?
• This study highlights the importance of glycolysis-related genes in clinical practice and provides a new method for the assessment of THCA patients.
Introduction
Thyroid cancer (THCA) is one of the most commonly malignant endocrine tumors worldwide. THCA currently ranks as the 13th most common cancer diagnosis overall and the 6th most common among women (1). In 1990, an estimated 95,030 cases of THCA were diagnosed and 22,070 deaths, were reported, and the number of incidents increased to 255,490 incident cases and 41,240 deaths in 2017 (2). In 2020, THCA ranked 9th in incidence of all cancer types, with more than 586,000 cases diagnosed worldwide. The incidence of THCA in China is also on the rise and has become one of top ten cancers threatening the health of the public (3).
THCA is divided into four categories, among which thyroid papillary carcinoma (PTC) accounts for more than 85% (4,5). Although an overwhelming majority of PTCs can be cured by surgical treatments and I131 treatments, 10% of the patients still die of poorly differentiated and advanced tumors (6). To a large extent, the increasing number of THCAs is attributed to the advancement of the progressively sensitive diagnostic imaging modalities (7,8). Therefore, the compelling need for early and accurate diagnosis and more effective treatment strategies are accompanied.
It is believed that the development and prognosis of THCA are related to the mutation and expression of some genes. For instance, lipid metabolism-related genes play an important role in the prognosis of THCA, and the risk of THCA has also been linked to impaired glucose metabolism (9,10). However, the pathogenesis of THCA is complicated and ambiguous, which requires further study.
In recent years, the metabolic process of tumor environment has become crucial in tumor research and treatment (11,12). Furthermore, the discovery of Warburg effect, which was introduced by German scientist Otto Warburg, is a vital breakthrough in cancer research (13,14). Cancer cells rewire their metabolism to promote growth, survival, proliferation, and long-term maintenance. The common feature of this altered metabolism is the increased glucose uptake and fermentation of glucose to lactate. This phenomenon is observed even in the presence of completely functioning mitochondria and, together, is known as the ‘Warburg Effect’. Most of the hallmarks of cancer could be the consequence of the Warburg’s effect (15,16). Therefore, the relationship between gene signatures and THCA was explored in this study by identifying glycolysis-related genes (GRGs) according to Warburg effect.
The purpose of this study was to identify the GRGs in the progression and metastasis of THCA. Gene Set Enrichment Analysis (GSEA) was used to select differentiated expressed genes. Finally, a 3-GRG risk signature was established which could effectively predict patient prognosis. Moreover, the gene-based model in the study, as an independent prediction factor, could identify patients with a high-risk score who showed a poorer prognosis than those with a low-risk score. We present this article in accordance with the TRIPOD reporting checklist (available at https://tcr.amegroups.com/article/view/10.21037/tcr-22-2548/rc).
Methods
Data acquisition
mRNA transcriptome data of THCA were obtained from TCGA. The RNA sequence data of TCHA were downloaded directly from TCGA data portal (https://portal.gdc.cancer.gov/). The disease types are all adenomas and adenocarcinomas. Data of Glycolysis-associated gene sets were obtained from GSEA official website. Data showing missing information or being inconsistent were excluded from the analysis. The study was conducted in accordance with the Declaration of Helsinki (as revised in 2013).
GSEA analysis of glycolysis-related pathways
Data of Glycolysis-associated gene sets were obtained from the official website of the GSEA. The pathways were: GO_GLYCOLYTIC_PROCESS (GGP), KEGG_GLYCOLYSIS_GLUCONEOGENESIS, REACTOME_GLYCOLYSIS, BIOCARTA_GLYCOLYSIS_PATHWAY, and HALLMARK_GLYCOLYSIS (HG). For GSEA analysis, the parameter for gene sets permutations was set 1,000 times, and a false discovery rate (FDR) <0.05 was considered significant.
Differential Expression Analysis and Glycolysis Related Genes Model
Based on the results of GSEA, glycolytic pathway with a close relationship with THCA (P<0.05) was screened. In these pathways, GRGs were summarized using the expression levels of genes. Then, using the limma software package in R ×64 v3.6.3 software, the expression level of GRGs was extracted. Between glycolysis pathway and the extracted GRGs, the dramatic differences (P<0.05, logFC ≥1 or ≤−1) in the expression profiles were analyzed. Cox risk regression analysis was used to further analyze the close correlation between GRGs and the survival rate, and the most significant genes were selected to construct the GRGs model. Receiver operating characteristic (ROC) curve was used to evaluate the accuracy and specificity of the model and the optimal cutoff point was obtained. Finally, as a basis for distinguishing the high-risk and the low-risk groups, the model’s critical value was used.
Mutation of model genes
A Human Protein Atlas (HPA) (www.proteinatlas.org) search was conducted for immunohistochemical images of the three genes in normal thyroid tissues and THCA tissues, and, by immunohistochemical staining, differences in gene expression between the two groups were confirmed. Mutation function in the cBioPortal website (www.cbioportal.org) was used to explore mutations in the 3 model genes.
Verification of GRGs model
The GRGs model was verified by univariate and multivariate COX proportional hazard analysis and survival analysis of THCA samples obtained from TCGA. Leave-one-out cross validation was performed to evaluate the accuracy of the model.
Statistical analysis
Data were presented as mean ± standard deviation (SD) except when being stated otherwise, and compared using the student’s t-test. Kaplan-Meier method was used to perform subsequent survival analysis and plot the graph survival curve. Univariate and multivariate COX regression analyses were used to filter differential expressed genes (DEGs) that significantly correlated with the prognosis of THCA (P value filter <0.05). P values less than 0.05 were considered statistically significant. Risk score was calculated with help of the ‘predict ()’ function in the survival package in the R software version 3.6.3 (www.r-project.org).
Results
GSEA analysis of glycolysis-related pathways
The obtained expression data of 12,398 genes from 567 samples including 58 normal tissues and 509 tumor tissues were used for follow-up research. At the same time, 507 patients were classified. Among these patients, 71 were over 65 years old and 436 were under 65 years old; 371 women and 136 men. The clinical demographics of patients from this cohort are presented in Table 1. GSEA analysis revealed that the genes were significantly enriched (P<0.05) in the GGP (Figure 1A) and the HG (Figure 1B) (Table 2).
Table 1
Variable | Count |
---|---|
Age (years) | |
≤65 | 436 |
>65 | 71 |
Gender | |
Female | 371 |
Male | 136 |
Stage | |
I–II | 337 |
III–IV | 168 |
Missing | 2 |
Stage T | |
T1–T2 | 311 |
T3–T4 | 194 |
Missing | 2 |
Stage N | |
N0 | 231 |
N1–3 | 226 |
Missing | 50 |
Stage M | |
M0 | 283 |
M1 | 9 |
MX | 215 |
TCGA, The Cancer Genome Atlas.
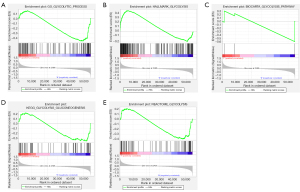
Table 2
Name | Size | ES | NES | NOM P value | FDR q value |
---|---|---|---|---|---|
GO_GLYCOLYTIC_PROCESS | 106 | −0.46 | −1.60 | 0.025 | 0.025 |
HALLMARK_GLYCOLYSIS | 200 | 0.45 | 1.57 | 0.027 | 0.027 |
BIOCARTA_GLYCOLYSIS_PATHWAY | 3 | −0.60 | −0.96 | 0.596 | 0.596 |
KEGG_GLYCOLYSIS_GLUCONEOGENESIS | 62 | −0.36 | −1.16 | 0.286 | 0.286 |
REACTOME_GLYCOLYSIS | 72 | −0.40 | −1.30 | 0.178 | 0.178 |
GSEA, Gene Set Enrichment Analysis; ES, enrichment score; NES, normalized enrichment score; NOM, nominal; FDR, false discovery rate.
Differential expression analysis and glycolysis related genes model
The extract of genes enriched in iconic glycolysis pathways and glycolytic process was conducted for differential expression analysis. A total of 52 differentially expressed GRGs were identified (Table S1). Three genes (HSPA5, KIF20A, SDC2) closely related to clinical prognosis were identified by COX risk regression analysis, and they are described in detail in Table 3. Then, the prognostic model was constructed. This prognostic model including three genes. It was found that HSPA5 and SDC2 were good prognostic genes in this model, whereas KIF20A was a bad prognostic gene. The area under curve (AUC) value was 0.68 (Figure 2A), based on the ROC curve of the model, indicating the moderate accuracy of the model. In the ROC curve, 1.84 was the best cutoff point. The sensitivity is 0.67, and the specificity is 0.61 in this point. After evaluating the samples and using the ‘predict ()’ function to calculate the risk score based on the GRGs model, the samples were divided into the high-risk groups and the low-risk groups using the median (50th percentile cutoff point) risk score as the boundary. The expression of the three model genes in the high-risk and the low-risk groups is provided in Figure 2B.
Table 3
Name | Coef. | Hazard ratio | Physiological role |
---|---|---|---|
HSPA5 | −0.00243 | 0.997568 | The protein encoded by HSPA5 is a member of the HSP70 family; it is localized in the lumen of the ER, and is involved in the folding and assembly of proteins in the ER |
KIF20A | 0.641416 | 1.899168 | KIF20A, a kinesin with unique structural features, has been proved to be potentially associated with prostate and liver cancer |
SDC2 | −0.00799 | 0.992038 | SDC2 methylation is a feasible biomarker for colorectal cancer detection |
Coef., coefficient; HSP70, heat shock protein 70; ER, endoplasmic reticulum.

Verification of GRGs model
The obtained genomic data were used to verify the GRGs model. The overall survival (OS) of the low-risk group was significantly higher than that of the high-risk group (Figure 2C,2D); In the lower half of Figure 2C, the y-axis represents the survival time, the red represents the dead patients in this group, and the blue dot represents the living patients in this group. In the upper part of Figure 2C, the y-axis represents the risk score, the dotted line represents the grouping, the green dot represents the patients with low-risk, and the red represents the patients with high-risk. As can be seen from the upper and lower parts of composite Figure 2C, the number of deaths in the low-risk group was lower than that in the high-risk group. Prognosis was significantly affected by age, stage, and GRGs model according to the univariate risk regression analysis (Figure 2E). P value of age was 0.035, and the Hazard ratio (HR) of age was 1.02. P value of stage was less than 0.001, and the HR of age was 1.54. P value of the risk score was less than 0.001, and the HR of the risk score was 1.22. Only GRGs model was capable of being used as an independent prognostic factor in multivariate risk regression analyses (Figure 2F). P value of the risk score was less than 0.001, and the HR of the risk score was 1.20. Leave-one-out analysis of the risk score cross validation showed the area under ROC curve (AUC) was 0.68.
Expression and mutation of model genes
An analysis of the expression levels of the genes was conducted. KIF20A (Figure 3A) was highly expressed while HSPA5 (Figure 3B) and SDC2 (Figure 3C) were decreased in THCA. HSPA5, KIF20A and SDC2’s expression is 477.80, 0.40, 177.72 respectively in normal samples, while expression of which is 307.02, 0.72, 86.77 respectively, in tumor samples. An analysis of the immunohistochemical images acquired from the HPA website of the three genes in normal thyroid gland tissues and THCA was conducted and this analysis confirmed these findings (17). The Human Protein Atlas version 21.0 provided the immunohistochemical images of the three genes shown in Figure 4. As a result of identification of mutations in model genes, SDC2 and KIF20A exhibited lower mutation rates compared to HSPA5 (Figure S1).
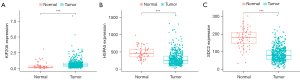
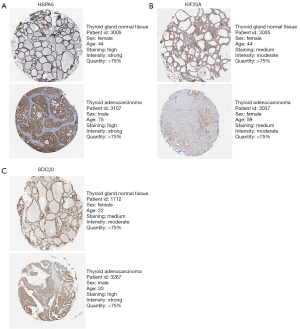
GRGs model and clinical characteristics
An analysis of clinical demographics and survival rates of THCA patients was conducted to discover their relationship. In the analysis, age (P=0.10) and gender (P=0.14) were not statistically significant (Figure 5A,5B). At the same time, only grades (Figure 5C) and T stages (Figure 5D) significantly influenced survival rate of patients (P<0.001).
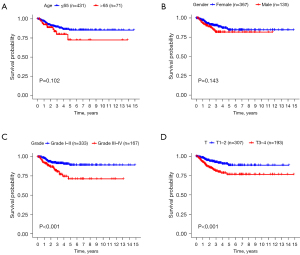
Based on the GRGs model, the clinical traits were grouped and the survival rates were analyzed later. Six patients with varying clinical characteristics could be explicitly distinguished by GRGs (P<0.05, Figure 6). This indicated that this GRGs model can distinguish high-risk and low-risk groups in the face of these six clinical characteristics. Nonetheless, result from distinguishing patients using age >65, male, M0–1, N0, Stage III–IV (Figure S2) was not significant.
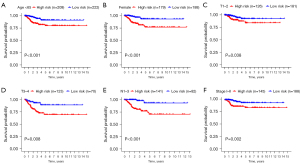
Discussion
THCA is the most common endocrine malignancy worldwide. In recent years, the incidence of THCA has been increasing (18,19). Although the incidence is high, the pathogenesis of thyroid has not been elucidated, so we need to further study. The purpose of this study is to identify GRGs in the process of THCA progression and metastasis. In this study, we constructed a glycolytic gene model associated with the prognosis of THCA patients, including three genes (HSPA5, KIF20A and SDC2). Our model can serve as a significant independent prognostic factor for THCA. Through this model, we can effectively predict the tumor metastasis and prognosis of THCA patients, and better select personalized programs for further treatment of patients.
In clinical practice, thyroid nodules are very common (20). Ultrasound guided fine needle aspiration (US-FNA) is the most commonly available way to evaluate thyroid nodules. However, rapid On-Site Evaluation (ROSE) is considered to be the most helpful with small sized nodules or nodules that are more difficult to sample fo9r less experienced radiologists (21). PTC patients with appropriate treatment and radiotherapy have a high 10-year survival rate (22). In radiotherapy, we must consider the harmful effects of radiation for normal tissues surrounding the tumor tissues. Accurate calculation of out-of-field dose to be critical for informing risk estimates, such as estimation of out-of-field dose variation using Monte Carlo simulations (23). Therefore, exploring novel therapeutic targets of THCA is still a major challenging issue.
Recently, studies on cellular energy metabolism have attracted people’s attention, especially the Warburg effect. Altered energy metabolism is a biochemical fingerprint of cancer cells that represents one of the “hallmarks of cancer”. Even when oxygen is abundant, cancer cells count primarily on glycolysis to produce ATP energy unlike normal cells (24). There has also been a great deal of research done on THCA’s glucose metabolism (25). In order to find probable biological targets for the treatment and diagnosis of THCA, the study of THCA glycolytic related genes and pathways is a potential approach. In addition, the current research on THCA glycolytic pathway mainly focuses on the role of single lncRNA in tumor growth and progression (26,27). It has also been proved that the expression level of glycolysis related proteins is different in different THCA subtypes, and is related to poor prognosis (28). This research is based on the protein molecular level. From the perspective of cell metabolism, this paper finds out the glycolytic genes related to THCA through the gene level. At the same time, as in this paper, we analyze the relationship between GRGs and survival rate in THCA patients by constructing a prediction model and sequencing clinical patient samples.
By analyzing the THCA data in TCGA, our study analyzed and found three glycolytic genes. A prognostic model, which is correlated with overall survival rate of THCA, was constructed using three GRGs (HSPA5, KIF20A and SDC2). Leave-one-out cross validation and ROC analysis indicated that the gene feature had a moderate effect on predicting survival rate. Previous studies suggested that the three GRGs we found were all related to the occurrence and development of some cancers (29-31). For example, decreased expression of HSPA5 is associated with the deterioration of clinical pathological characteristics, shortened PFI, and increased immune infiltration of various immune cells, indicating that HSPA5 is a biomarker for the prognosis of THCA (32). It has also been pointed out that HSPA5 contributes to the survival of head and neck cancer by maintaining lysosomal activity (33). These studies indicate that our results are consistent with those of our predecessors to a certain extent. Furthermore, multiple roles of KIF20A in central spindle assembly and cleavage furrow formation in mitosis and meiosis (34). KIF20A is highly expressed in many cancers, such as lung adenocarcinoma and hepatocellular carcinoma, and is a potential target for tumor gene therapy (35,36). SDC2 methylation is a feasible biomarker for colorectal cancer detection (37). These results suggest that these glycolytic related genes may play an important role in determining the prognosis of patients with THCA.
We know that the current TMN clinical staging of tumor is the most widely used malignant potential and the methods of disease progression. However, this method also has some disadvantages. For instance, in the case of patients suffering from the same disease at the same stage are treated with the same treatment, the prognosis of patients can make a big different. Despite being in the same stage, clinical outcomes differ for patients, indicating that the existing staging system is not sufficient for effective prognosis. Our study showed that only T stage was significantly associated with OS of THCA patients in the analyzed sample. In addition, there is no significant relationship between the survival rate of patients and the age and sex of cancer. Then, using the established model, we can identify high and low risk groups through six clinical characteristics. These six characteristics are age <65, female, T1–2, T3–4, N1–3, Stage I–III (Figure 6). However, we could not judge the risk by other characteristics in Figure S2. A novel method for assessing THCA patients is provided by our model, which can predict THCA patients’ prognosis more accurately.
In addition, since our research is a retrospective analysis, there are some limitations. A prospective study using GRGs model for prospective research would be more persuasive. Moreover, in the analysis, the majority of the data was obtained from developed countries’ public databases, but there was a lack of data from developing countries. It also needs to be reiterated that this is a secondary analysis of previous studies, and a prospective clinical study is needed to verify the accuracy of our results.
Conclusions
In conclusion, this study has identified a GRG model (including HSPA5, KIF20A and SDC2) which is related to the prognosis of THCA patients. Furthermore, these three GRGs may be potential targets for the treatment of THCA, while further prospective large-scale clinical trial should be conducted to confirm our conclusion.
Acknowledgments
Funding: This study was funded by
Footnote
Reporting Checklist: The authors have completed the TRIPOD reporting checklist. Available at https://tcr.amegroups.com/article/view/10.21037/tcr-22-2548/rc
Conflicts of Interest: All authors have completed the ICMJE uniform disclosure form (available at https://tcr.amegroups.com/article/view/10.21037/tcr-22-2548/coif). The authors have no conflicts of interest to declare.
Ethical Statement: The authors are accountable for all aspects of the work in ensuring that questions related to the accuracy or integrity of any part of the work are appropriately investigated and resolved. The study was conducted in accordance with the Declaration of Helsinki (as revised in 2013).
Open Access Statement: This is an Open Access article distributed in accordance with the Creative Commons Attribution-NonCommercial-NoDerivs 4.0 International License (CC BY-NC-ND 4.0), which permits the non-commercial replication and distribution of the article with the strict proviso that no changes or edits are made and the original work is properly cited (including links to both the formal publication through the relevant DOI and the license). See: https://creativecommons.org/licenses/by-nc-nd/4.0/.
References
- Kitahara CM, Schneider AB. Epidemiology of Thyroid Cancer. Cancer Epidemiol Biomarkers Prev 2022;31:1284-97. [Crossref] [PubMed]
- Deng Y, Li H, Wang M, et al. Global Burden of Thyroid Cancer From 1990 to 2017. JAMA Netw Open 2020;3:e208759. [Crossref] [PubMed]
- Du L, Zhao Z, Zheng R, et al. Epidemiology of Thyroid Cancer: Incidence and Mortality in China, 2015. Front Oncol 2020;10:1702. [Crossref] [PubMed]
- Wu M, Yuan H, Li X, et al. Identification of a Five-Gene Signature and Establishment of a Prognostic Nomogram to Predict Progression-Free Interval of Papillary Thyroid Carcinoma. Front Endocrinol (Lausanne) 2019;10:790. [Crossref] [PubMed]
- Carling T, Udelsman R. Thyroid cancer. Annu Rev Med 2014;65:125-37. [Crossref] [PubMed]
- Albero A, Lopéz JE, Torres A, et al. Effectiveness of chemotherapy in advanced differentiated thyroid cancer: a systematic review. Endocr Relat Cancer 2016;23:R71-84. [Crossref] [PubMed]
- Udelsman R, Zhang Y. The epidemic of thyroid cancer in the United States: the role of endocrinologists and ultrasounds. Thyroid 2014;24:472-9. [Crossref] [PubMed]
- Brito JP, Morris JC, Montori VM. Thyroid cancer: zealous imaging has increased detection and treatment of low risk tumours. BMJ 2013;347:f4706. [Crossref] [PubMed]
- Kushchayeva Y, Kushchayev S, Jensen K, et al. Impaired Glucose Metabolism, Anti-Diabetes Medications, and Risk of Thyroid Cancer. Cancers (Basel) 2022;14:555. [Crossref] [PubMed]
- Wen S, Luo Y, Wu W, et al. Identification of lipid metabolism-related genes as prognostic indicators in papillary thyroid cancer. Acta Biochim Biophys Sin (Shanghai) 2021;53:1579-89. [Crossref] [PubMed]
- Jones NP, Schulze A. Targeting cancer metabolism--aiming at a tumour’s sweet-spot. Drug Discov Today 2012;17:232-41. [Crossref] [PubMed]
- Vander Heiden MG, Cantley LC, Thompson CB. Understanding the Warburg effect: the metabolic requirements of cell proliferation. Science 2009;324:1029-33. [Crossref] [PubMed]
- Kozal K, Jóźwiak P, Krześlak A. Contemporary Perspectives on the Warburg Effect Inhibition in Cancer Therapy. Cancer Control 2021;28:10732748211041243. [Crossref] [PubMed]
- Iorio J, Petroni G, Duranti C, et al. Potassium and Sodium Channels and the Warburg Effect: Biophysical Regulation of Cancer Metabolism. Bioelectricity 2019;1:188-200. [Crossref] [PubMed]
- Liberti MV, Locasale JW. The Warburg Effect: How Does it Benefit Cancer Cells? Trends Biochem Sci 2016;41:211-8. [Crossref] [PubMed]
- Schwartz L, Supuran CT, Alfarouk KO. The Warburg Effect and the Hallmarks of Cancer. Anticancer Agents Med Chem 2017;17:164-70. [Crossref] [PubMed]
- Uhlén M, Fagerberg L, Hallström BM, et al. Proteomics. Tissue-based map of the human proteome. Science 2015;347:1260419. [Crossref] [PubMed]
- La Vecchia C, Malvezzi M, Bosetti C, et al. Thyroid cancer mortality and incidence: a global overview. Int J Cancer 2015;136:2187-95. [Crossref] [PubMed]
- Zha T, Wu H. Expression of serum AMPD1 in thyroid carcinoma and its clinical significance. Exp Ther Med 2018;15:3357-61. [Crossref] [PubMed]
- Haugen BR, Alexander EK, Bible KC, et al. 2015 American Thyroid Association Management Guidelines for Adult Patients with Thyroid Nodules and Differentiated Thyroid Cancer: The American Thyroid Association Guidelines Task Force on Thyroid Nodules and Differentiated Thyroid Cancer. Thyroid 2016;26:1-133. [Crossref] [PubMed]
- Medina Chamorro FM, Calle JA, Stein JE, et al. Experience of the Implementation of Rapid On-Site Evaluation in Ultrasound-Guided Fine-Needle Aspiration Biopsy of Thyroid Nodules. Curr Probl Diagn Radiol 2018;47:220-4. [Crossref] [PubMed]
- Leboulleux S, Rubino C, Baudin E, et al. Prognostic factors for persistent or recurrent disease of papillary thyroid carcinoma with neck lymph node metastases and/or tumor extension beyond the thyroid capsule at initial diagnosis. J Clin Endocrinol Metab 2005;90:5723-9. [Crossref] [PubMed]
- Sánchez-Nieto B, Medina-Ascanio KN, Rodríguez-Mongua JL, et al. Study of out-of-field dose in photon radiotherapy: A commercial treatment planning system versus measurements and Monte Carlo simulations. Med Phys 2020;47:4616-25. [Crossref] [PubMed]
- Akram M. Mini-review on glycolysis and cancer. J Cancer Educ 2013;28:454-7. [Crossref] [PubMed]
- Wu M, Ou-Yang DJ, Wei B, et al. A Prognostic Model of Differentiated Thyroid Cancer Based on Up-Regulated Glycolysis-Related Genes. Front Endocrinol (Lausanne) 2022;13:775278. [Crossref] [PubMed]
- Jiang B, Chen Y, Xia F, et al. PTCSC3-mediated glycolysis suppresses thyroid cancer progression via interfering with PGK1 degradation. J Cell Mol Med 2021;25:8454-63. [Crossref] [PubMed]
- Huo N, Cong R, Sun ZJ, et al. STAT3/LINC00671 axis regulates papillary thyroid tumor growth and metastasis via LDHA-mediated glycolysis. Cell Death Dis 2021;12:799. [Crossref] [PubMed]
- Nahm JH, Kim HM, Koo JS. Glycolysis-related protein expression in thyroid cancer. Tumour Biol 2017;39:1010428317695922. [Crossref] [PubMed]
- Zhang C, Liu Q, Zhou Y, et al. HSPA5 Could Be a Prognostic Biomarker Correlated with Immune Infiltration in Breast Cancer. Dis Markers 2022;2022:7177192. [Crossref] [PubMed]
- Saito K, Ohta S, Kawakami Y, et al. Functional analysis of KIF20A, a potential immunotherapeutic target for glioma. J Neurooncol 2017;132:63-74. [Crossref] [PubMed]
- Lei R, Zhao Y, Huang K, et al. The methylation of SDC2 and TFPI2 defined three methylator phenotypes of colorectal cancer. BMC Gastroenterol 2022;22:88. [Crossref] [PubMed]
- Dong W, Du D, Huang H. HSPA5 is a prognostic biomarker correlated with immune infiltrates in thyroid carcinoma. Endokrynol Pol 2022;73:680-9. [Crossref] [PubMed]
- Kim SY, Kim HJ, Kim HJ, et al. HSPA5 negatively regulates lysosomal activity through ubiquitination of MUL1 in head and neck cancer. Autophagy 2018;14:385-403. [Crossref] [PubMed]
- Wu WD, Yu KW, Zhong N, et al. Roles and mechanisms of Kinesin-6 KIF20A in spindle organization during cell division. Eur J Cell Biol 2019;98:74-80. [Crossref] [PubMed]
- Wu C, Qi X, Qiu Z, et al. Low expression of KIF20A suppresses cell proliferation, promotes chemosensitivity and is associated with better prognosis in HCC. Aging (Albany NY) 2021;13:22148-63. [Crossref] [PubMed]
- Zhao X, Zhou LL, Li X, et al. Overexpression of KIF20A confers malignant phenotype of lung adenocarcinoma by promoting cell proliferation and inhibiting apoptosis. Cancer Med 2018;7:4678-89. [Crossref] [PubMed]
- Zhang L, Dong L, Lu C, et al. Methylation of SDC2/TFPI2 and Its Diagnostic Value in Colorectal Tumorous Lesions. Front Mol Biosci 2021;8:706754. [Crossref] [PubMed]