Subtyping or not subtyping—Quo vadis for precision medicine of colorectal cancer
Subtyping of colorectal cancer (CRC)—the solution is the problem
A few weeks ago, on 29th December 2022, Edson Arantes do Nascimento, in the author’s opinion, the best soccer player ever, otherwise known as Pele, passed away. He had CRC for some time. Pele is one of about one million individuals worldwide who die from this malignancy yearly. CRC ranks as one of the most lethal cancers, being the third most prevalent malignancy in men and women in large parts of the world (1). The death rate from CRC declined by about 50% over the last 50 years because of advances in surveillance, diagnosis, and treatment (2). Meanwhile, the 5-year survival rate is, on average, about 65% but drops to about 10% for metastatic CRC (3). One major challenge for further improvement is that some patients respond well to therapy while others do not. Thus, more precise, individualized diagnostics and treatment strategies are needed (4) (Figure 1A).
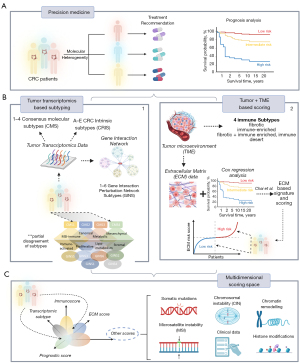
From a genomic standpoint, CRC is not a single disease but a highly heterogeneous group of malignancies arising within the colon, with clinicopathologically similar tumors developing along different molecular pathways, accumulating different patterns of somatic mutations, and differing strikingly in treatment response and patient survival. These differences are only partly explained by the two major routes of colorectal carcinogenesis. One is related to hypermutation burden and microsatellite instability (MSI) occurring through a deficiency in the mismatch repair mechanism, and the other is related to chromosomal instability (CIN) paralleled by large-scale copy number alterations along the genome (5,6). The majority of CRC (85%) develops as CIN type and is microsatellite stable (MSS), while 15% (in early stages) belongs to the MSI type. Both routes involve a series of genetic hits affecting primarily the APC, KRAS, TP53, and a few other genes, known as the Vogelstein sequence (7). These hits are paralleled by diverse patterns of somatic mutations in dozens of genes and/or copy number alterations progressively accumulating during cancerogenesis (6). However, DNA mutations alone do not fully explain the malignant transformation of CRC; co-evolution of the genome and epigenome of colorectal tumors, chromatin remodeling, and aberrant DNA and histone methylation are important mechanisms affecting the genome of CRC (8).
Gene expression plays a pivotal role in genome analytics because it directly reflects the effect of genetics and epigenetics on the gene and cell levels. During the last decade, especially whole transcriptome gene expression profiling studies improved our understanding of the molecular heterogeneity of CRC. Early microarray-based studies of various authors came up with six classification schemes of CRC, which were subsequently unified into one consensus scheme (9) (Figure 1B.1). It divided CRC tumors into four consensus molecular subtypes (CMS), namely CMS1 (MSI enriched, immune activated, 14% of cases); CMS2 (canonical, epithelial, activated WNT and MYC signaling, 37%); CMS3 (metabolic, epithelial, 13%) and CMS4 (mesenchymal, stromal invasion and angiogenesis, 23%). However, CMS classification revealed limitations, mainly related to uncertainties between genetic lesions and functional characteristics on the pathway and cellular levels. To better distinguish cancer-cell intrinsic features from the tumor microenvironmental (TME) effects, five CRC intrinsic subtypes (CRIS A-E) were proposed as an alternative classification scheme (10), showing, however, limited overlap with the CMS classes. The newest classification scheme (11), utilized a gene interaction perturbation network-based (GIN) approach, which identified six subtypes with distinguishing features such as proliferation (GINS1, immune-desert and immunotherapeutic resistance, 24–34% of cases), stroma (GINS2, immune-suppressed, unfavorable prognosis, high potential of recurrence and metastasis, immunotherapeutic resistance and sensitivity to fluorouracil-based chemotherapy, 14–22%); KRAS-inactivation (GINS3, immune-desert, CIN, immunotherapeutic resistance and sensitive to cetuximab and bevacizumab, 13%); mixed characteristics (GINS4, moderate level of stromal and immune activities, transit-amplifying-like, 10–19%); immune-activation (GINS5, neoantigen burden, MSI and CMI, BRAF mutations, favorable prognosis and sensitive to immunotherapy, 12–24%) and metabolic dysregulation (GINS6, accumulation of fatty acids, enterocyte-like characteristics, 5–8%) (11). None of these GIN subtypes maps in a one-to-one fashion to the CMS classes: a given GINS overlaps with CMS at most with 55–80% [see (11) for a detailed comparison with previous classification schemes]. Also, key genetic lesions do not strongly associate with any subtypes except moderate enrichment of mutations of PIK3CA in GINS1 (45% of cases), BRAF in GINS5 (46%), SMAD4 in GINS2 (63%), and depletion of KRAS mutations in GINS3 (12%). This lack of associations between genetics and related cancer phenotypes impedes diagnostics. In contrast to CRC, other cancer entities such as lower-grade gliomas well divide into genetic classes (mainly related to mutations of the IDH1 gene and CNA on Chr.1 and 19). These classes strongly associate with transcriptional and histological groups of different prognoses as recognized and accepted by World Health Organization (WHO) for classifying the given tumor type (12).
The different subtyping schemes of CRC have not yet led to a single proper classification. Each of them sheds light on specific molecular aspects of CRC heterogeneity and development, but, bottom line; they could not substantially improve the effective management of CRC patients. One reason can be found in methodology: the different subtyping schemes were developed under various aspects of transcriptional similarities between the tumors, leading to classes of relatively small partial mutual agreement with each other. Another presumably more substantial reason is the complex nature of this cancer type. Its genesis from a healthy colon via adenoma towards cancer of different stages follows the relatively simple Vogelstein sequence, which, however, is overlaid by myriads of genetic lesions leading to a vast spectrum of developmental options under genetic, epigenetic, and transcriptional control. Moreover, CRC arises in an extended organ of variable local physiology changing from, e.g., left and right-sided regions (13), metabolic conditions, and microbiome composition (14). The situation from the molecular diagnostics perspective still needs improvement and might lead to a situation where a better subtyping scheme is needed. On the flip side, the classification of CRC into precisely defined subtypes is not the solution but a problem. Do we need alternative approaches for better diagnostics and treatment decisions in the era of precision medicine?
Scoring of the TME provides a significant prognostic dimension
Chai and colleagues applied an alternative approach “beyond subtyping” of CRC in the manuscript of Translational Cancer Research (15). It is based on a reformed view of cancer, which is not considered a tumor-cell-centric disease. Instead, the environment of the tumor cells, the TME is considered the key determinant in cancer development and therapeutic resistance (Figure 1B.2). The TME mainly consists of tumor cells, stromal cells such as fibroblasts, immune cells, and noncellular components of the extracellular matrix (ECM). Within the TME, intimate communications among these components largely determine the fate of the tumor. According to this “seed and soil” theory, Chai et al. assumed that the stromal component might strongly impact the prognosis of CRC (15). The authors generated an ECM-based prognostic signature of marker genes, which is assumed to provide clues for survival and therapeutic response.
The method uses about one thousand genes of stromal functional context, selects differentially expressed genes between CRC and a healthy colon, and utilizes them together with the survival status of, in total, more than 700 patients as input for Cox regression analysis. It finally extracts prognosis-related ECM genes and constructs a mathematical risk score model as the weighted sum of the expression of four signature genes. Two of the four risk genes correlate positively (THBS4 and SFRP5), and two correlate negatively (CXCL13 and CXCL14) with the hazard ratio of CRC. The mean survival rates of CRC patients’ low and high-risk groups differ markedly. This difference is virtually retained for covariates (age, gender, etc.) as well as the stage of the tumors and metastasis (M-stage). Most interestingly, a remarkable difference is also retained for the assignment of the tumors to the different CMS subtypes differing in stromal functional context (CMS4 vs. non-CMS4), showing that ECM is a significant prognostic factor. Immune cell deconvolution revealed increased infiltration of B-cells, M0-macrophages, regulatory T-cells, and CD4+ T-cells into the TME at high risk paralleled by depletion of M1 and M2 macrophages of plasma and CD8+ cells. Interestingly, the authors concluded from these results that this novel ECM-based signature might play a critical role in tumor progression through the immune system, e.g., via immune escape, as found for MSI-type CRC (16). Note that under a conceptual perspective, this scoring approach, in contrast to the subtyping schemes, assumes a continuous dimension of risk-related features without clear-cut borderlines between the classes, which more adequately displays continuous developmental processes in a living cell.
The approach of Chai et al. is supported by previous reports, which suggest that the stromal content of CRC is a strong indicator of tumor aggressiveness and poor prognosis (10). The inferior prognosis of the stromal-derived GINS2 subtype, characterized by abundant fibrous content, resistance against immune therapy, and a high potential for recurrence and metastasis (11), also supports this approach. The immuno-therapeutic resistance is mainly due to infiltrating immunosuppressive cells, such as fibroblasts, T-regulatory cells, and M2 macrophages. It is therefore dubbed as an immune-suppressed phenotype, which accumulates at high values of the ECM-score related to inferior prognosis. In contrast, the better prognosis range of the ECM score accumulates immuno-activated tumors partly responsive to immunotherapy. Interestingly, a recent Pan-cancer study underlined the impact of the TME and identified four immune/fibrotic TME-related tumor types (fibrotic, immune-enriched, fibrotic and immune enriched, immune desert) (17). They are conserved across diverse cancers and correlate with immunotherapy response, and can aid in clinical decision-making. Notably, the fibrotic and immune-enriched types associated with the ECM-score ranked CRC cases in Chai et al. These results underline the pivotal roles of the stroma for tumorigenesis, tumor progression, therapeutic response, and tumor immunity and thus, the potential impact of the ECM-scoring system presented by Chai and colleagues (15).
Quo vadis: towards a multidimensional scoring space?
The latest 5th edition WHO classification of digestive system tumors from 2019 for the first time defines certain tumor types by their molecular phenotype; however, in most instances, the histopathological classification remains the gold standard for diagnosis (18). As for other tumor types, such as that of the Central Nervous System (12), the trend goes towards molecular classification and/or scoring schemes, which are expected to outperform previous schemes, at least in perspective. Molecular subtyping, in combination with molecular classifiers, will partly replace histopathological schemes in the future. For CRC, there is still a long way to go, despite the progress in understanding this malignancy on the molecular level.
Several possible perspectives seem reasonable. Firstly, the somewhat successful but prognostically and biologically limited subtyping schemes can be combined with the scoring approach presented by Chai and colleagues (15). The scoring along the ECM-axis could be complemented by scorings along other molecular and prognostic axes known as relevant from the CRC subtypes, such as proliferation, metabolic activity, or epigenetic plasticity leading to a multidimensional coordinate system of different scores (Figure 1C). A cancer stemness and drug resistance score was previously developed for glioma prognosis (19) using a similar method to that used by Chai et al. (15). A specific tumor is then characterized by its position in the multidimensional coordinate system spanned by the scores, combining continuous scales of different associations with molecular features and prognosis. Secondly, this transcriptional scoring system could be combined with genetic (e.g., somatic mutations, CNA) or other omics (methylation, chromatin accessibility, proteomic) features and also with clinical characteristics related to treatment (e.g., sensitivity, adverse effects, combinations of drugs). The position of an individual tumor in this scoring space would predict prognosis, support treatment decisions, and characterize its molecular background. Clear-cut demarcated subtypes would appear as regions characterized by probabilistic metrics related to treatment decisions and prognosis. They would enable the combination of aspects of personalized medicine (e.g., decision-making regarding individual patients) with a holistic view of the cancer entity of interest or even in the Pan-cancer context.
Both approaches mutually support each other in a dualistic way: personalized decision-making in precision medicine requires broad information about the environment in the multidimensional scoring space, which, in turn, collects holistic information from a vast number of individual cases. The prognostic ECM-scoring of CRC, as published in this issue of Translational Cancer Research, represents a proof-of-principle building block on the way to reaching this goal. Combining multidimensional axes can be beneficial but tricky because using mathematical models that are unaware of the biological background of the data can give misleading results. Attention needs to be directed toward biologically meaningful models. Here Cox regressions constitute only one option. When considering sparse data such as somatic mutations, biological enrichment of the data becomes necessary for better interpretability of the results. In this context also, a trade-off balancing accuracy and stability becomes essential (20): small numbers of marker genes, e.g., the four ECM-markers extracted by Chai et al. (15), can lead to noisy scorings in practical applications and could be substituted by larger sets of marker genes (also called metagenes) making the scores more robust without significant loss of accuracy. Large stability gains can be reached at the small cost of classification accuracy utilizing metagene approaches. Grouping genes into gene sets of a defined functional context and assigning a summarizing score to them also reduces the feature set, transforms the scale of data, and lowers their dimensionality.
The score and subtype space can be validated by correlating metagenes with clinical covariates, such as survival duration and status and treatment data. Much research is directed to analyze the effect of multi-omics subtyping on the prognostic value with different methods considering mixed effects (21). The treatment-dependent survival bias is a significant challenge in the clinical analysis and validation of molecular scoring. A chosen metric heavily depends on the treatment each patient assigned to a score/cluster has received. Here, treatment-based averaging of the survival of a given cohort may mislead the clinical relevance of the used score. A possible solution would be to use treatment-specific data. However, it has minimal availability and relatively poor quality at the moment. A possible alternative is an experimental validation by modeling human genetic or phenotypic signatures taken from patients in model systems such as cancer cell lines (22), mice, or even non-vertebrate organisms such as flies (23): this approach provides a fruitful ground for existing and novel drug and drug-combination screenings. Scoring variables could be taken under control for covariates to ensure unbiased analysis. Overall, biologically meaningful scoring, subtyping, and association with treatment-relevant data will pave the way toward precision medicine of CRC and other cancer entities.
Acknowledgments
Funding: None.
Footnote
Provenance and Peer Review: This article was commissioned by the editorial office, Translational Cancer Research. The article did not undergo external peer review.
Conflicts of Interest: Both authors have completed the ICMJE uniform disclosure form (available at https://tcr.amegroups.com/article/view/10.21037/tcr-23-133/coif).
Ethical Statement: The authors are accountable for all aspects of the work in ensuring that questions related to the accuracy or integrity of any part of the work are appropriately investigated and resolved.
Open Access Statement: This is an Open Access article distributed in accordance with the Creative Commons Attribution-NonCommercial-NoDerivs 4.0 International License (CC BY-NC-ND 4.0), which permits the non-commercial replication and distribution of the article with the strict proviso that no changes or edits are made and the original work is properly cited (including links to both the formal publication through the relevant DOI and the license). See: https://creativecommons.org/licenses/by-nc-nd/4.0/.
References
- Sung H, Ferlay J, Siegel RL, et al. Global Cancer Statistics 2020: GLOBOCAN Estimates of Incidence and Mortality Worldwide for 36 Cancers in 185 Countries. CA Cancer J Clin 2021;71:209-49. [Crossref] [PubMed]
- Dekker E, Tanis PJ, Vleugels JLA, et al. Colorectal cancer. Lancet 2019;394:1467-80. [Crossref] [PubMed]
- Xie YH, Chen YX, Fang JY. Comprehensive review of targeted therapy for colorectal cancer. Signal Transduct Target Ther 2020;5:22. [Crossref] [PubMed]
- Sadanandam A, Lyssiotis CA, Homicsko K, et al. A colorectal cancer classification system that associates cellular phenotype and responses to therapy. Nat Med 2013;19:619-25. [Crossref] [PubMed]
- Kim JC, Bodmer WF. Genomic landscape of colorectal carcinogenesis. J Cancer Res Clin Oncol 2022;148:533-45. [Crossref] [PubMed]
- Comprehensive molecular characterization of human colon and rectal cancer. Nature 2012;487:330-7. [Crossref] [PubMed]
- Fearon ER, Vogelstein B. A genetic model for colorectal tumorigenesis. Cell 1990;61:759-67. [Crossref] [PubMed]
- Heide T, Househam J, Cresswell GD, et al. The co-evolution of the genome and epigenome in colorectal cancer. Nature 2022;611:733-43. [Crossref] [PubMed]
- Guinney J, Dienstmann R, Wang X, et al. The consensus molecular subtypes of colorectal cancer. Nat Med 2015;21:1350-6. [Crossref] [PubMed]
- Isella C, Brundu F, Bellomo SE, et al. Selective analysis of cancer-cell intrinsic transcriptional traits defines novel clinically relevant subtypes of colorectal cancer. Nat Commun 2017;8:15107. [Crossref] [PubMed]
- Liu Z, Weng S, Dang Q, et al. Gene interaction perturbation network deciphers a high-resolution taxonomy in colorectal cancer. Elife 2022;11:e81114. [Crossref] [PubMed]
- Louis DN, Perry A, Wesseling P, et al. The 2021 WHO Classification of Tumors of the Central Nervous System: a summary. Neuro Oncol 2021;23:1231-51. [Crossref] [PubMed]
- Mukund K, Syulyukina N, Ramamoorthy S, et al. Right and left-sided colon cancers - specificity of molecular mechanisms in tumorigenesis and progression. BMC Cancer 2020;20:317. [Crossref] [PubMed]
- Wong SH, Yu J. Gut microbiota in colorectal cancer: mechanisms of action and clinical applications. Nat Rev Gastroenterol Hepatol 2019;16:690-704. [Crossref] [PubMed]
- Chai R, Su Z, Zhao Y, et al. Extracellular matrix-based gene signature for predicting prognosis in colon cancer and immune microenvironment. Transl Cancer Res 2023;12:321-39. [Crossref] [PubMed]
- Binder H, Hopp L, Schweiger MR, et al. Genomic and transcriptomic heterogeneity of colorectal tumours arising in Lynch syndrome. J Pathol 2017;243:242-54. [Crossref] [PubMed]
- Bagaev A, Kotlov N, Nomie K, et al. Conserved pan-cancer microenvironment subtypes predict response to immunotherapy. Cancer Cell 2021;39:845-865.e7. [Crossref] [PubMed]
- Nagtegaal ID, Odze RD, Klimstra D, et al. The 2019 WHO classification of tumours of the digestive system. Histopathology 2020;76:182-8. [Crossref] [PubMed]
- Zeng F, Wang K, Liu X, et al. Comprehensive profiling identifies a novel signature with robust predictive value and reveals the potential drug resistance mechanism in glioma. Cell Commun Signal 2020;18:2. [Crossref] [PubMed]
- Loeffler-Wirth H, Kreuz M, Schmidt M, et al. Classifying Germinal Center Derived Lymphomas-Navigate a Complex Transcriptional Landscape. Cancers (Basel) 2022;14:3434. [Crossref] [PubMed]
- Ayton SG, Pavlicova M, Robles-Espinoza CD, et al. Multiomics subtyping for clinically prognostic cancer subtypes and personalized therapy: A systematic review and meta-analysis. Genet Med 2022;24:15-25. [Crossref] [PubMed]
- Jaaks P, Coker EA, Vis DJ, et al. Effective drug combinations in breast, colon and pancreatic cancer cells. Nature 2022;603:166-73. [Crossref] [PubMed]
- Cagan RL, Zon LI, White RM. Modeling Cancer with Flies and Fish. Dev Cell 2019;49:317-24. [Crossref] [PubMed]