Identification of risk factors of EGFR-TKIs primary resistance in lung adenocarcinoma patients and construction of a risk predictive model: a case-control study
Highlight box
Key findings
• A predictive model based on serum neuron-specific enolase concentration, pro-gastrin-releasing peptide concentration, and Ki67 expression was established. This model can be used to predict epidermal growth factor receptor-tyrosine kinase inhibitors (EGFR-TKIs) primary resistance in lung adenocarcinoma patients.
What is known and what is new?
• Some patients with EGFR-sensitive mutations develop primary resistance to EGFR-TKIs.
• We analyzed the clinical characteristics of lung adenocarcinoma patients with primary resistance to EGFR-TKIs, identified independent risk factors for primary resistance, and established a risk prediction model to provide reference for clinical decision-making.
What is the implication, and what should change now?
• This predictive model holds promise for clinical application, aiding in the development of personalized treatment strategies and providing a scientific basis for early identification of primary resistance patients.
• Future diagnosis and treatment will further focus on mechanism research.
Introduction
Background
Lung cancer is one of the most prevalent and deadliest malignancies, with approximately 2.2 million new cases diagnosed annually and nearly 1.8 million deaths related to lung cancer (1). Non-small-cell lung carcer (NSCLC) accounts for about 85% of all lung cancer cases, with lung adenocarcinoma (LUAD) being the most common histological type, representing approximately 50% of all NSCLC cases (2). Epidermal growth factor receptor (EGFR), anaplastic lymphoma kinase (ALK), and c-ros oncogene 1 receptor tyrosine kinase (ROS1) are common driver genes in LUAD. EGFR mutations are the most frequent, accounting for approximately 50.2% with exon 19 deletion (19del) and exon 21 L858R mutation (21L858R) being sensitive mutations associated with good response to EGFR-tyrosine kinase inhibitors (EGFR-TKIs). Both National Comprehensive Cancer Network (NCCN) and Chinese Society of Clinical Oncology (CSCO) guidelines recommend EGFR-TKIs as the first-line treatment for advanced-stage LUAD patients with EGFR mutations.
Rationale and knowledge gap
EGFR-TKIs can significantly improve the progression-free survival (PFS) of patients with 19del and L858R mutations in LUAD, but these patients usually develop resistance to first-generation EGFR-TKIs after 9–12 months (3). Common resistance mechanisms include (4): (I) EGFR re-mutations, with T790M mutation being the most common in patients receiving first-generation EGFR-TKIs, accounting for approximately 55% (5); (II) EGFR amplification; (III) downstream or bypass pathway activation; (IV) phenotypical changes. In addition, 5–20% (6-8) of LUAD patients have EGFR-sensitive mutations but are not responsive to EGFR-TKIs, or they initially respond but experience rapid progression within 3 months. These patients represent a subtype of LUAD commonly referred to as primary resistance by the international academic community (9-11). The resistance mechanisms of primary resistance to EGFR-TKIs are currently unclear, and it is generally believed to be related to biological individuality marker (BIM) polymorphism inhibiting apoptosis, Kirsten rat sarcoma viral oncogene (KRAS) gene mutations, complex mutations of EGFR, and multiple co-mutations (12). Whole-genome sequencing (13) can effectively identify patients with primary resistance to EGFR-TKIs, but the difficulty in obtaining sequencing samples limits its clinical application. It is crucial to seek an alternative approach for screening patients with primary resistance.
Objective
This study aims to provide clinical evidence for predicting primary resistance to EGFR-TKIs in patients with EGFR-sensitive mutation LUAD by comparing the clinical characteristics of patients with primary resistance and sensitivity. By identifying statistically significant risk factors, a risk predictive model can be constructed to assist clinicians in predicting whether patients with EGFR-sensitive mutations will develop primary resistance to EGFR-TKIs. We present this article in accordance with the TRIPOD reporting checklist (available at https://tcr.amegroups.com/article/view/10.21037/tcr-23-2172/rc).
Methods
The general information
The medical records of LUAD patients who were admitted and treated at Tongji Hospital, Tongji Medical College, Huazhong University of Science and Technology from 2020 to 2022 were retrospectively reviewed. Inclusion criteria were as follows: (I) EGFR mutation-positive with sensitive mutations including 19del or 21L858R; (II) first-line treatment with EGFR-TKIs; (III) regular assessment of treatment efficacy with a follow-up period of ≥6 months. Exclusion criteria were: (I) presence of other malignant tumors; (II) missing follow-up data. A total of 154 patients were finally included, among whom 27 patients developed primary resistance to EGFR-TKIs with a PFS ≤3 months, classified into the resistant group (R-group). Additionally, 127 patients showed good response to EGFR-TKIs with a PFS >3 months, classified into the sensitive group (S-group). The study was conducted in accordance with the Declaration of Helsinki (as revised in 2013). The study was approved by Medical Ethics Committee of Tongji Hospital, Tongji Medical College, Huazhong University of Science and Technology (No. TJ-IRB20231217). Informed consent was taken from all the patients.
Data collection
Patients’ general clinical information (including gender, presence of clinical symptoms, EGFR-TKI sensitive mutation type) was collected, pre-treatment serum examinations [including white blood cell (WBC), hemoglobin (HB), platelets (PLT), carcinoembryonic antigen (CEA), neuron-specific enolase (NSE), cytokeratin-19 fragment 21-1 (CYFRA21-1), pro-gastrin-releasing peptide (ProGRP)] were performed, tumor-related characteristics (including lesion location, size, presence of lobulation sign, spiculation sign, pleural retraction sign, lymph node and distant metastasis status, Ki67 expression) were collected.
Clinical staging was based on the 8th edition of the International Association for the Study of Lung Cancer (IASLC) Tumor Node Metastasis (TNM) staging system for lung cancer (14), and treatment response assessment was conducted according to the Response Evaluation Criteria in Solid Tumors (RECIST) criteria (15).
Statistical analysis
Selection of predictive factors
Data analysis was performed using SPSS 25.0 software. Categorical data were presented as frequencies, while continuous quantitative data were expressed as mean ± standard deviation () if normally distributed, or as median (interquartile range) [M (P25, P75)] if not normally distributed. Initially, differences between the sensitive group and resistant group were analyzed. Chi-squared test was used to compare the differences in clinical characteristics and tumor-related features between the two groups. If the theoretical frequency was less than five, Fisher’s exact test was employed. For comparing differences in serum examinations between the two groups, t-test was used for normally distributed data, whereas Mann-Whitney U test was used for non-normally distributed data. Risk factors with statistically significant differences identified through inter-group comparative analysis were included in a multivariable logistic regression analysis. Odds ratios (ORs) and confidence intervals (CIs) were calculated to estimate the associations and correlations between various factors and the occurrence of primary resistance to EGFR-TKIs. Eventually independent risk factors were subsequently identified.
Analysis of the predictive value of risk factors
The selected independent risk factors were subjected to predictive value analysis using R3.6.1 software. The quantitative analysis of the predictive value for both single-factor and multi-factor combinations of primary resistance to EGFR-TKIs was assessed through the receiver operating characteristic (ROC) curve and the area under the curve (AUC). The risk factors included in constructing the nomogram predictive model were those that maximized the AUC.
Construction and evaluation of the predictive model
Using R3.6.1 software on Windows, a nomogram predictive model was constructed to predict the occurrence of primary resistance to EGFR-TKIs based on the selected risk factors with the maximum AUC. The clinical applicability of the predictive model was determined through calibration curve analysis and decision curve analysis.
Results
General clinical data
There were no statistically significant differences in gender (P=0.10) and EGFR sensitive mutation types (P=0.49) between patients with primary resistance to EGFR-TKIs and sensitive patients. Among the patients, those with hemoptysis had the highest incidence of primary resistance (61.11%), which was significantly higher than patients with only cough and sputum (17.95%) and those with no clinical symptoms (9.27%), with a statistically significant difference (P<0.001) (Table 1).
Table 1
General clinical data | S-group | R-group | Chi-squared test (P) |
---|---|---|---|
Gender | 0.10 | ||
Male | 58 (45.67) | 17 (62.96) | |
Female | 69 (54.33) | 10 (37.04) | |
Clinical symptoms | <0.001 | ||
No | 88 (69.29) | 9 (33.33) | |
Cough | 32 (25.20) | 7 (25.93) | |
Bloody sputum | 7 (5.51) | 11 (40.74) | |
EGFR mutation type | 0.49 | ||
19del | 75 (59.06) | 14 (51.85) | |
21L858R | 52 (40.94) | 13 (48.15) |
S-group, the sensitive group; R-group, the resistant group. Data were presented as frequency (percentage). EGFR, epidermal growth factor receptor.
Serum analysis
Patients with primary resistance showed significantly higher serum NSE concentrations [28.31 (22.02, 34.12) ng/mL] compared to the sensitive group [15.31 (13.02, 19.80) ng/mL], with a significant difference (P<0.001). ProGRP levels in the resistance group [58.70 (40.60, 70.50) pg/mL] were much higher than in the sensitive group [46.80 (33.35, 62.80) pg/mL], and this difference was statistically significant (P<0.001). There were no statistically significant differences in serum CEA and CYFRA21-1 concentrations between the two groups (P=0.64, 0.10). Other laboratory parameters such as WBC, HB, and PLT showed no statistically significant differences between the two groups (P=0.83, 0.47, 0.53) (Table 2).
Table 2
Serological examinations | S-group | R-group | T-test (P) |
---|---|---|---|
WBC (109/L) | 6.53±2.18 | 6.64±1.86 | 0.83 |
HB (g/L) | 131.32±19.22 | 128.19±20.24 | 0.47 |
PLT (109/L) | 240.14±75.99 | 249.89±58.72 | 0.53 |
CEA (ng/mL) | 20.92 [4.53, 61.18] | 10.59 [4.16, 68.90] | 0.64 |
NSE (ng/mL) | 15.31 [13.02, 19.80] | 28.31 [22.02, 34.12] | <0.001 |
CYFRA21-1 (ng/mL) | 4.01 [2.55, 6.36] | 5.26 [2.91, 11.66] | 0.10 |
ProGRP (pg/mL) | 46.80 [33.35, 62.80] | 58.70 [40.60, 70.50] | <0.001 |
S-group, the sensitive group; R-group, the resistant group. Data were expressed as mean ± standard deviation () or as median [interquartile range] [M (P25, P75)]. WBC, white blood cell; HB, hemoglobin; PLT, platelets; CEA, carcinoembryonic antigen; NSE, neuron-specific enolase; CYFRA21-1, cytokeratin-19 fragment 21-1; ProGRP, pro-gastrin-releasing peptide.
Tumor-related features
There were no statistically significant differences between the two groups in terms of lesion lung lobe distribution, lobulation signs, spiculation signs, pleural retraction signs, lymph node metastasis, and distant metastasis (P=0.51, 0.06, 0.60, 0.16, 0.13, 0.30). The tumor diameter in patients with primary resistance [47 mm (32, 58 mm)] was larger than that in the sensitive group [28 mm (22, 31 mm)], and the difference was statistically significant (P<0.001). Ki67 expression, as detected through pathological examination, showed a marked difference between the two groups. The Ki67 expression in the primary resistance group [60% (50%, 70%)] was significantly higher than in the sensitive group [15% (10%, 30%)], with a statistically significant difference (P<0.001) (Table 3).
Table 3
Tumor-related features | S-group | R-group | Chi-squared test, Mann-Whitney U test (P)† |
---|---|---|---|
Lobe location | 0.51 | ||
The left upper lobe | 34 (26.77) | 8 (29.63) | |
The left lower lobe | 24 (18.90) | 4 (14.81) | |
The right upper lobe | 38 (29.92) | 7 (25.93) | |
The right middle lobe | 7 (5.51) | 4 (14.81) | |
The right lower lobe | 24 (18.90) | 4 (14.81) | |
Tumor size (mm) | 28 [22, 31] | 47 [32, 58] | <0.001 |
Lobulation sign | 0.06 | ||
No | 89 (70.08) | 14 (51.85) | |
Yes | 38 (29.92) | 13 (48.15) | |
Spiculation sign | 0.60 | ||
No | 82 (64.57) | 16 (59.26) | |
Yes | 45 (35.43) | 11 (40.74) | |
Pleural retraction sign | 0.16 | ||
No | 88 (69.29) | 15 (55.56) | |
Yes | 39 (30.71) | 12 (44.44) | |
Lymph node metastasis | 0.13 | ||
No | 67 (52.76) | 10 (37.04) | |
Yes | 60 (47.24) | 17 (62.96) | |
Distant metastasis | 0.30 | ||
No | 76 (59.84) | 19 (70.37) | |
Yes | 51 (40.16) | 8 (29.63) | |
Ki67 expression (%) | 15 [10, 30] | 60 [50, 70] | <0.001 |
S-group, the sensitive group; R-group, the resistant group. Data were presented as frequency (percentage), or as median [interquartile range] [M (P25, P75)]. †, tumor size (mm) and Ki67 expression (%) are analyzed using the Mann-Whitney U test, while the remaining variables are analyzed using the Chi-squared test.
Independent risk factor analysis for primary resistance
Factors that showed statistically significant differences between the resistance and sensitive groups were included in a multiple logistic regression analysis. The results indicated that serum NSE concentration, ProGRP concentration, and Ki67 expression were independent risk factors for EGFR-TKIs resistance (P=0.03, 0.01, P<0.001) (Table 4).
Table 4
Influence factor | OR | 95% CI | P |
---|---|---|---|
Clinical symptoms | 2.016 | 0.562–7.230 | 0.28 |
NSE (ng/mL) | 1.137 | 1.012–1.278 | 0.03 |
ProGRP (pg/mL) | 1.046 | 1.010–1.084 | 0.01 |
Tumor size (mm) | 1.032 | 0.987–1.080 | 0.17 |
Ki67 | 1.170 | 1.080–1.267 | <0.001 |
OR, odds ratio; CI, confidence interval; NSE, neuron-specific enolase; ProGRP, pro-gastrin-releasing peptide.
Analysis of the predictive value of single and combined factors for primary resistance to EGFR-TKIs
Single factors such as serum NSE concentration, ProGRP concentration, and Ki67 expression had some predictive value for identifying primary resistance to EGFR-TKIs, with respective AUC values of 0.880, 0.635, and 0.956 (Figure 1). When combining NSE and ProGRP, the AUC was 0.877 with a 95% CI of 0.819–0.936. (Figure 2A). Combining NSE and Ki67 resulted in an AUC of 0.968 with a 95% CI of 0.928–1.000 (Figure 2B). Combining ProGRP and Ki67 resulted in an AUC of 0.969 with a 95% CI of 0.924–1.000 (Figure 2C). Combining NSE, ProGRP, and Ki67 yielded an AUC of 0.975 with a 95% CI of 0.937–1.000 (Figure 2D), which was superior to using single or paired factors (Table 5).
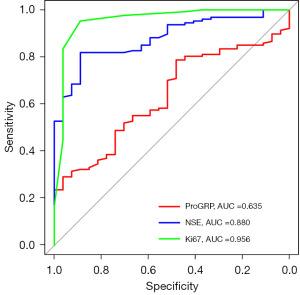
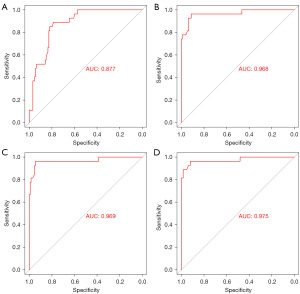
Table 5
Influence factor | AUC | 95% CI | P |
---|---|---|---|
NSE (ng/mL) | 0.880 | 0.822–0.938 | <0.001 |
ProGRP (pg/mL) | 0.635 | 0.534–0.727 | 0.02 |
Ki67 | 0.956 | 0.904–1.00 | <0.001 |
NSE + ProGRP | 0.877 | 0.819–0.936 | <0.001 |
NSE + Ki67 | 0.968 | 0.928–1.000 | <0.001 |
ProGRP + Ki67 | 0.969 | 0.924–1.000 | <0.001 |
NSE + ProGRP + Ki67 | 0.975 | 0.937–1.000 | <0.001 |
EGFR-TKIs, epidermal growth factor receptor-tyrosine kinase inhibitors; AUC, area under the curve; CI, confidence interval; NSE, neuron-specific enolase; ProGRP, pro-gastrin-releasing peptide.
Construction of a risk predictive model for primary resistance to EGFR-TKIs
Based on the results of the multiple logistic regression analysis and the predictive value analysis of risk factors, the three independent risk factors (serum NSE concentration, serum ProGRP concentration, and Ki67 expression) with the highest AUC values were included in the construction of a risk predictive model for primary resistance to EGFR-TKIs using R software. This model was presented in the form of a nomogram (Figure 3). By assigning values to the variables on the nomogram, the total score can be calculated, allowing for the estimation of the risk of developing primary resistance to EGFR-TKIs in patients with EGFR-sensitive mutations in LUAD.
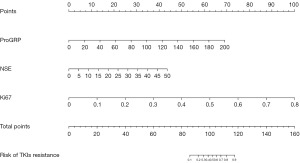
Risk predictive model evaluation
The calibration curve is a scatter plot of actual occurrence probability versus predicted probability. The diagonal line represents the ideal condition of actual occurrence rates, while the solid line formed by the scatter points represents the predicted occurrence rates. The closer the solid line is to the diagonal line, the closer the predicted and actual occurrences are, indicating higher model accuracy. We conducted internal validation of the column line graph model using Bootstrap resampling method. After repeated Bootstrap resampling 100 times, the calibration curve showed an absolute error of 0.023 between the predicted curve and the actual curve, indicating that the two curves had a consistent trend and strong consistency (Figure 4).
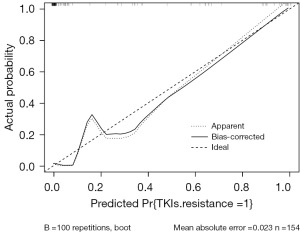
Finally, we performed decision curve analysis to evaluate the clinical utility of the nomogram (Figure 5). The decision curve shows two extreme scenarios: when the EGFR-TKIs primary resistance risk predictive model predicts that no LUAD patients taking EGFR-TKIs will experience primary resistance (represented by the black horizontal line), the net benefit is 0; when the risk predictive model predicts that all LUAD patients will experience primary resistance (represented by the gray diagonal line), the situation is also extreme. The results of our decision curve analysis indicate that the red curve of the nomogram predictive n model, which integrates NSE, ProGRP, and Ki67, is consistently higher than the black and gray extreme lines, suggesting that the model has good clinical utility. Using this nomogram to predict the occurrence of primary resistance to EGFR-TKIs provides a significant net benefit, making dynamic treatment strategy selection more effective than “treat all” or “no treatment” strategies.
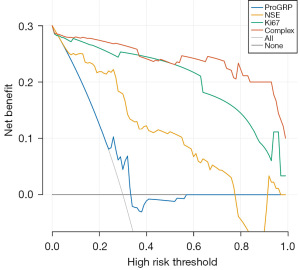
Discussion
LUAD is one of the malignant tumors with persistently high incidence and mortality rates. With advancements in medicine, the emergence of EGFR-TKIs, targeted therapies, has brought hope for improved survival in patients with EGFR driver gene mutations in LUAD, significantly enhancing treatment efficacy. However, some patients with EGFR-sensitive mutations do not respond to EGFR-TKIs and develop resistance rapidly, a condition known as primary resistance. Therefore, it is crucial in clinical practice to explore the risk factors for primary resistance to EGFR-TKIs and develop risk predictive models for tailoring individual treatment strategies.
ProGRP is the precursor structure of gastrin-releasing peptide (GRP), a 27-amino acid neuropeptide hormone that stimulates gastrin release. GRP has a short half-life of approximately 2.2 minutes in serum, making it challenging to be detected clinically. ProGRP, on the other hand, is characterized by its structural stability and longer half-life, and its levels are positively correlated with GRP levels. It has emerged as a serum tumor marker in recent years. The study has shown that ProGRP (16) is currently one of the best auxiliary diagnostic serum markers for small cell lung cancer (SCLC). NSE (17) is an enolase enzyme involved in the glycolytic pathway, primarily found in neural and neuroendocrine tissues. It is overexpressed in tumors related to neuroendocrine tissue origin, particularly in SCLC, leading to a significant increase in NSE levels in serum. The combined use of ProGRP and NSE (18) has high diagnostic value for detecting SCLC. One mechanism of EGFR-TKI resistance is the transformation of LUAD into SCLC. Research suggests that the incidence of transformation from EGFR-positive LUAD to SCLC is 15% (19), higher than in EGFR wild-type patients. The mechanisms behind this histological transformation from LUAD to SCLC are not fully understood, but two possibilities exist (20): first, the original tumor contains both components (adenocarcinoma and small cell carcinoma), with the initial diagnosis dominated by one component. Second, molecular targeted therapy may induce the transformation of adenocarcinoma into SCLC. The case report study by Fang et al. (21) demonstrated that the transformation of LUAD to SCLC after EGFR-TKI treatment contributes to resistance to EGFR-TKIs, and the serum levels of NSE can also be used to detect early SCLC transformation. Similarly, a study by Jin et al. (22) with nine case reports also yielded the same research conclusion. In our study, patients in the primary resistance group had higher serum concentrations of ProGRP and NSE compared to the sensitive group, suggesting that serum ProGRP and NSE have some value in predicting whether LUAD is primary resistant to EGFR-TKIs. From this, we hypothesize that although the pathological specimens from lung biopsies are relatively small and may be able to detect only one adenocarcinoma subtype, tumor tissues are heterogeneous and may contain both LUAD and SCLC components. Patients with high ProGRP and NSE levels may have a higher proportion of SCLC, making them more susceptible to early resistance. Since patients’ resistance after biopsy retesting is extremely low, and repeated biopsies cannot guarantee that samples come from the same tissue site, this hypothesis remains unproven, and further research is needed to elucidate the exact mechanisms.
Ki67 is a non-histone protein expressed throughout the cell cycle, representing the degree of cell proliferation activity, with expression in cells beyond the G0 phase. In many solid tumors, Ki67 has been utilized as a predictive biomarker for assessing treatment efficacy and prognosis, such as in breast cancer (23) and prostate cancer (24). Currently, the value of Ki67 expression in lung cancer remains a subject of debate, with the study indicating a negative correlation between Ki67 expression and survival prognosis in lung cancer patients (25). Research (26) has shown that low Ki67 expression is significantly associated with the effectiveness of EGFR-TKI treatment (P=0.02). Additionally, Gu et al.’s study (27) suggests that in late-stage EGFR mutant LUAD patients, whether in the EGFR-19del group or EGFR-L858R group, high Ki67 expression can reduce objective response rate (ORR) (P<0.001) and shorten PFS (P<0.001). In this study, Ki67 expression was significantly higher in the primary resistance group compared to the sensitive group, potentially linked to primary resistance to EGFR-TKIs due to excessive cancer cell proliferation caused by elevated Ki67 and resulting cancer cell heterogeneity.
A nomogram (28) is a visual graphic based on a multivariate regression model that integrates multiple parameters, represented by varying lengths of line segments assigned to different variables. It provides a comprehensive and intuitive representation of predictive outcomes. In this study, we conducted a single-center retrospective study to identify three independent risk factors for building a personalized risk predictive model, assessing the risk of primary resistance in LUAD patients following EGFR-TKI treatment. Further validation showed that this model has excellent predictive accuracy and clinical application potential. Presented in the form of a nomogram, it allows for the prediction of the risk of primary resistance in patients before EGFR-TKI targeted therapy based on cumulative calculations of various feature values. It is concise and intuitive, and when combined with decision curve analysis, it assists clinical physicians in making personalized treatment choices, maximizing clinical benefits. For high-risk patients with primary resistance, clinicians should select appropriate diagnostic and treatment strategies based on individual patient circumstances, such as considering a combination of chemotherapy or radiotherapy to delay resistance development.
Our study has some limitations. Firstly, being a retrospective, single-center study, it inevitably carries inherent biases. Secondly, due to the limited number of cases in the primary resistance group, this study only conducted internal validation. Future research will require external validation and broader application across other centers to further confirm the clinical utility of the model. Given these limitations, we will continue to expand the sample size of primary resistance patients, delve deeper into clinically relevant predictive factors, and improve the model based on the existing study results to optimize the prediction of primary resistance to EGFR-TKIs.
Conclusions
In summary, based on a multifactorial logistic regression analysis, we have constructed a predictive model for the occurrence of primary resistance to EGFR-TKIs, incorporating serum levels of NSE, ProGRP, and Ki67. Further evaluation has demonstrated that this model exhibits good predictive capability and holds significant clinical utility. This model shows promise in identifying individuals at high risk for primary resistance, enabling clinicians to take proactive measures to delay the onset of resistance, thereby enhancing patients’ quality of life and extending prognosis.
Acknowledgments
Funding: None.
Footnote
Reporting Checklist: The authors have completed the TRIPOD reporting checklist. Available at https://tcr.amegroups.com/article/view/10.21037/tcr-23-2172/rc
Data Sharing Statement: Available at https://tcr.amegroups.com/article/view/10.21037/tcr-23-2172/dss
Peer Review File: Available at https://tcr.amegroups.com/article/view/10.21037/tcr-23-2172/prf
Conflicts of Interest: All authors have completed the ICMJE uniform disclosure form (available at https://tcr.amegroups.com/article/view/10.21037/tcr-23-2172/coif). The authors have no conflicts of interest to declare.
Ethical Statement: The authors are accountable for all aspects of the work in ensuring that questions related to the accuracy or integrity of any part of the work are appropriately investigated and resolved. The study was conducted in accordance with the Declaration of Helsinki (as revised in 2013). The study was approved by the Medical Ethics Committee of Tongji Hospital, Tongji Medical College, Huazhong University of Science and Technology (No. TJ-IRB20231217). Informed consent was taken from all the patients.
Open Access Statement: This is an Open Access article distributed in accordance with the Creative Commons Attribution-NonCommercial-NoDerivs 4.0 International License (CC BY-NC-ND 4.0), which permits the non-commercial replication and distribution of the article with the strict proviso that no changes or edits are made and the original work is properly cited (including links to both the formal publication through the relevant DOI and the license). See: https://creativecommons.org/licenses/by-nc-nd/4.0/.
References
- Sung H, Ferlay J, Siegel RL, et al. Global Cancer Statistics 2020: GLOBOCAN Estimates of Incidence and Mortality Worldwide for 36 Cancers in 185 Countries. CA Cancer J Clin 2021;71:209-49. [Crossref] [PubMed]
- Thai AA, Solomon BJ, Sequist LV, et al. Lung cancer. Lancet 2021;398:535-54. [Crossref] [PubMed]
- Tan CS, Kumarakulasinghe NB, Huang YQ, et al. Third generation EGFR TKIs: current data and future directions. Mol Cancer 2018;17:29. [Crossref] [PubMed]
- Rotow J, Bivona TG. Understanding and targeting resistance mechanisms in NSCLC. Nat Rev Cancer 2017;17:637-58. [Crossref] [PubMed]
- Stewart EL, Tan SZ, Liu G, et al. Known and putative mechanisms of resistance to EGFR targeted therapies in NSCLC patients with EGFR mutations-a review. Transl Lung Cancer Res 2015;4:67-81. [PubMed]
- Jiang H, Zhu M, Li Y, et al. Association between EGFR exon 19 or exon 21 mutations and survival rates after first-line EGFR-TKI treatment in patients with non-small cell lung cancer. Mol Clin Oncol 2019;11:301-8. [Crossref] [PubMed]
- Lee JK, Shin JY, Kim S, et al. Primary resistance to epidermal growth factor receptor (EGFR) tyrosine kinase inhibitors (TKIs) in patients with non-small-cell lung cancer harboring TKI-sensitive EGFR mutations: an exploratory study. Ann Oncol 2013;24:2080-7. [Crossref] [PubMed]
- Giuliani J, Martelli S, Remo A, et al. Primary TKI resistance in advanced non-small cell lung cancer with EGFR mutation: an open question. Tumori 2015;101:e115-e117. [Crossref] [PubMed]
- Mok TS, Wu YL, Thongprasert S, et al. Gefitinib or carboplatin-paclitaxel in pulmonary adenocarcinoma. N Engl J Med 2009;361:947-57. [Crossref] [PubMed]
- Zhou C, Wu YL, Chen G, et al. Erlotinib versus chemotherapy as first-line treatment for patients with advanced EGFR mutation-positive non-small-cell lung cancer (OPTIMAL, CTONG-0802): a multicentre, open-label, randomised, phase 3 study. Lancet Oncol 2011;12:735-42. [Crossref] [PubMed]
- Santoni-Rugiu E, Melchior LC, Urbanska EM, et al. Intrinsic resistance to EGFR-Tyrosine Kinase Inhibitors in EGFR-Mutant Non-Small Cell Lung Cancer: Differences and Similarities with Acquired Resistance. Cancers (Basel) 2019;11:923. [Crossref] [PubMed]
- He J, Huang Z, Han L, et al. Mechanisms and management of 3rd generation EGFR TKI resistance in advanced non small cell lung cancer Int J Oncol 2021;59:90. (Review). [Crossref] [PubMed]
- Lim SM, Kim HR, Cho EK, et al. Targeted sequencing identifies genetic alterations that confer primary resistance to EGFR tyrosine kinase inhibitor (Korean Lung Cancer Consortium). Oncotarget 2016;7:36311-20. [Crossref] [PubMed]
- Goldstraw P, Chansky K, Crowley J, et al. The IASLC Lung Cancer Staging Project: Proposals for Revision of the TNM Stage Groupings in the Forthcoming (Eighth) Edition of the TNM Classification for Lung Cancer. J Thorac Oncol 2016;11:39-51. [Crossref] [PubMed]
- Schwartz LH, Litière S, de Vries E, et al. RECIST 1.1-Update and clarification: From the RECIST committee. Eur J Cancer 2016;62:132-7. [Crossref] [PubMed]
- Molina R, Filella X, Augé JM. ProGRP: a new biomarker for small cell lung cancer. Clin Biochem 2004;37:505-11. [Crossref] [PubMed]
- Xu CM, Luo YL, Li S, et al. Multifunctional neuron-specific enolase: its role in lung diseases. Biosci Rep 2019;39:BSR20192732. [Crossref] [PubMed]
- Peng Y, Wang Y, Li J, et al. Utility of NSE, ProGRP and LDH in Diagnosis and Treatment in Patients with Small Cell Lung Cancer. Zhongguo Fei Ai Za Zhi 2016;19:590-4. [PubMed]
- Mambetsariev I, Arvanitis L, Fricke J, et al. Small Cell Lung Cancer Transformation following Treatment in EGFR-Mutated Non-Small Cell Lung Cancer. J Clin Med 2022;11:1429. [Crossref] [PubMed]
- Oser MG, Niederst MJ, Sequist LV, et al. Transformation from non-small-cell lung cancer to small-cell lung cancer: molecular drivers and cells of origin. Lancet Oncol 2015;16:e165-72. [Crossref] [PubMed]
- Fang L, He J, Xia J, et al. Resistance to epithelial growth factor receptor tyrosine kinase inhibitors in a patient with transformation from lung adenocarcinoma to small cell lung cancer: A case report. Oncol Lett 2017;14:593-8. [Crossref] [PubMed]
- Jin CB, Yang L. Histological transformation of non-small cell lung cancer: Clinical analysis of nine cases. World J Clin Cases 2021;9:4617-26. [Crossref] [PubMed]
- Zhu X, Chen L, Huang B, et al. The prognostic and predictive potential of Ki-67 in triple-negative breast cancer. Sci Rep 2020;10:225. [Crossref] [PubMed]
- Lobo J, Rodrigues Â, Antunes L, et al. High immunoexpression of Ki67, EZH2, and SMYD3 in diagnostic prostate biopsies independently predicts outcome in patients with prostate cancer. Urol Oncol 2018;36:161.e7-161.e17. [Crossref] [PubMed]
- Martin B, Paesmans M, Mascaux C, et al. Ki-67 expression and patients survival in lung cancer: systematic review of the literature with meta-analysis. Br J Cancer 2004;91:2018-25. [Crossref] [PubMed]
- Lara-Guerra H, Chung CT, Schwock J, et al. Histopathological and immunohistochemical features associated with clinical response to neoadjuvant gefitinib therapy in early stage non-small cell lung cancer. Lung Cancer 2012;76:235-41. [Crossref] [PubMed]
- Gu W, Hu M, Xu L, et al. The Ki-67 Proliferation Index-Related Nomogram to Predict the Response of First-Line Tyrosine Kinase Inhibitors or Chemotherapy in Non-small Cell Lung Cancer Patients With Epidermal Growth Factor Receptor-Mutant Status. Front Med (Lausanne) 2021;8:728575. [Crossref] [PubMed]
- Park SY. Nomogram: An analogue tool to deliver digital knowledge. J Thorac Cardiovasc Surg 2018;155:1793. [Crossref] [PubMed]