Development and validation of a prognostic prediction model for cervical cancer patients treated with radical radiotherapy: a study based on TCGA database
Highlight box
Key findings
• We identify a five radioresponse-related mRNA (PTX3, LRRC66, CERS4, SLC4A11, and FMOD) signature that shows promising clinical efficacy in predicting the prognosis of cervical cancer (CC) patients treated with radical radiotherapy.
What is known and what is new?
• Previous prediction models were focused on all CC patients, neglecting the prognostic differences under different treatment modalities, leading to poor predictive accuracy.
• In this study, we have constructed a multivariate tool to predict the prognosis and sensitivity of radiation therapy for CC patients treated with radical radiotherapy.
What is the implication, and what should change now?
• When constructing a prognostic model, it is crucial to consider the patient’s treatment mode, as this will enhance the accuracy, reliability, specificity, and sensitivity of the model. Our model can help identify high-risk for CC patients who received radical radiotherapy. Early management strategies can then be implemented in these high-risk patients, aiming to improve their prognosis.
Introduction
Cervical cancers (CCs) are one of the most common malignant tumors among women. It is estimated that in the United States in 2023, 1,958,310 people were diagnosed with cancer, among them, with 13,960 diagnosed with CC (1). Among CCs, cervical squamous cell carcinoma and endocervical adenocarcinoma (CESC) comprise 10–15% of all female cancer-related mortalities and are the second most fatal malignancy in women (2). In most instances, patients have already progressed into locally advanced stages when the diseases were first definitely diagnosed. Radiotherapy or concurrent chemoradiotherapy is the standard treatment for patients with locally advanced or inoperable disease (3). Local recurrence and distant metastasis are still the main causes of treatment failure for CESC patients treated with radical radiotherapy (3,4). Thus, there is an urgent need to investigate novel biomarkers for prognosis and sensitivity of radiotherapy in these patients. However, the previous prediction models were focused on all CESC patients, neglecting the prognostic differences under different treatment modalities, leading to poor predictive accuracy (5-7). Consequently, a multivariate tool is necessary for predicting the prognosis and sensitivity of radiation to guide suitable treatment for CESC patients undergoing radical radiotherapy.
The Cancer Genome Atlas (TCGA) database is an extensive tumor database that comprises over 30 multi-omics whole-genome sequencing datasets of various tumors, such as CESC multi-omics datasets, along with accompanying clinical records. Until now, it has served as a significant global research database (8,9). The aim of this investigation was to develop an mRNA prognostic model associated with radioresponse using a comprehensive bioinformatics analysis. The model is designed to forecast the prognosis of CESC patients who undergo radical radiotherapy. Initially, mRNAs correlating with radiotherapy response were obtained from 92 TCGA-CESC patients with mRNAs expression profiles. The precision and reliability of a radioresponse-related signature comprised of five genes were then confirmed in several cohorts. In addition, we also analyzed the prognostic value of these five genes in CESC patients receiving radical radiotherapy, respectively. Finally, immune infiltration analysis and Gene Set Enrichment Analysis (GSEA) were conducted to preliminarily explore possible mechanisms. The flow chart of this research is displayed in Figure 1. We present this article in accordance with the TRIPOD reporting checklist (available at https://tcr.amegroups.com/article/view/10.21037/tcr-23-1772/rc).
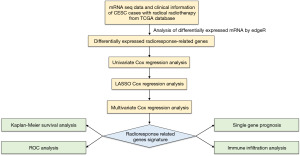
Methods
Datasets and processing
The mRNA seq expression profiles of TCGA-CESC and their associated clinical data were gathered from the TCGA project (https:/portal.gdc.cancer.gov/). The gene expression screening condition were “Project: TCGA-CESC”, “Data Category: Transcriptome profiling”, “Data Type: Gene Expression Quantification”, “Experimental Strategy: RNA-Seq”, “Workflow Type: STAR - Counts”. The clinical information screening condition were “Project: TCGA-CESC”, “Data Category: Clinical”, and “Data Type: Clinical Supplement”. The transcriptional profiles and clinical data of CESC are publicly accessible and offered on an open-access basis. Hence, authorization from a local ethics committee was deemed unnecessary. The gene annotation information for all genes was sourced from the human GENCODE project (https://www.gencodegenes.org/).
This study included a total of 92 CESC patients subjected to radical radiotherapy. The inclusion criteria for all cases were as follows: (I) all patients were diagnosed with CESC histologically; (II) radiotherapy was performed as the radical treatment for CESC patients; (III) expression profiles were available; and (IV) the patient’s overall survival (OS) time was more than 30 days. It should be mentioned that only 57 individuals had the curative effect evaluation. Thirty-six patients were evaluated as complete response (CR), seven patients were evaluated as partial response (PR), two patients were classified as stable disease (SD), and 12 patients were classified as radiographic progressive disease (RPD). The details of the clinical sample in the dataset were shown in Table S1.
The research procedures of this study were as follow: firstly, we obtained radioresponse-related mRNAs in CESC patients treated with radical radiotherapy. Secondly, we screened five radioresponse-related mRNAs in the training set and established a robust five-gene prognostic signature. Then, we employed both the test cohort and the entire cohort to validate the prognosis outcomes of our signature. Additionally, we formulated a nomogram model by integrating the signature with other relevant clinical factors. Finally, immune infiltration analysis and GSEA were conducted to preliminarily explore possible mechanisms. The study was conducted in accordance with the Declaration of Helsinki (as revised in 2013).
Identification and analysis of differentially expressed genes (DEGs)
To acquire radioresponse-related mRNAs in CESC, we scrutinized the differential expression of mRNAs between CR and RPD patients. We utilized the edgeR (R version 4.2.1) package to normalize and evaluate significantly differentially expressed mRNAs [log2fold change (FC) ≥1 and false discovery rate (FDR) less than 0.05] (10). Radioresponse-related genes with differential expression were depicted on volcano plots and heatmaps utilizing the ggplot2 and pheatmap packages.
Gene Ontology (GO) and Kyoto Encyclopedia of Genes and Genomes (KEGG) analysis
GO and KEGG pathway analyses were carried out by using the clusterProfiler package of R (11). The GO included molecular function (MF), biological process (BP), and cellular component (CC). Differences were deemed significant when P value less than 0.05.
Identification of radioresponse-related genes risk score model
We randomly separated the 92 cases into a training and test set using a 1:1 ratio to obtain the optimal radioresponse-related genes risk score model. Within the training set, probable prognostic mRNAs were initially evaluated using the univariate hazard Cox method that relied on radioresponse-related mRNAs. The glmnet R package was then used to conduct a least absolute shrinkage and selection operator (LASSO) Cox regression analysis to select the genes associated with the prognosis of CESC (12). Then, the genes mentioned above were further optimized by the multivariate Cox regression method. The genes risk score, associated with radioresponse, was calculated based on the chosen genes using the following formula: risk score = (exp radioresponse-related gene 1 × coef1) + (exp radioresponse-related gene 2 × coef2) + (exp radioresponse-related gene 3 × coef3) + … + (exp radioresponse-related gene n × coefn). The exp means the mRNA expression value of each radioresponse-related gene and the coef is the coefficient of each radioresponse-related gene generated by the multivariate Cox regression. Upon analyzing the median value of the risk score, all 92 CESC patients who received radical radiotherapy were segregated into low-risk and high-risk groups based on their scores. Subsequently, the differences in survival outcomes were assessed via Kaplan-Meier analysis (13). We also performed receiver operating characteristic curve (ROC curve) analysis using 1, 3 and 5 years as the predicted time to assess the predictive value of the outcome (nsROC package of R). The areas under the ROC curve, sensitivity and specificity were used to describe predictive values.
Nomogram construction
The radioresponse-related risk signature was integrated into patients’ clinical information, and the multivariate Cox regression analysis was conducted to identified the independent factors of CESC patients who underwent radical radiotherapy. In order to forecast the prognosis of patients, the nomogram was also constructed based on risk scores and other clinicopathological characteristics (14). The nomogram’s calibration curve was then obtained, and the correlation between the predicted probability of nomogram and the actual incidence rate was observed. P<0.05 was considered statistically significant.
Immune infiltration analysis
In order to investigate disparities in immune infiltration and the tumor immune microenvironment between the high- and low-risk groups, we conducted following analyses. Firstly, we used the CIBERSORT algorithm to evaluate dissimilarities in immune cell infiltration for each sample between the two groups (by CIBERSORT package) (15). To differentiate the vital functional phenotypes between the high- and low-risk groups, GSEA was executed using the clusterProfilter package (11).
Statistical analysis
All statistical evaluations were conducted utilizing R software version 4.2.1. Survival curves were plotted using the Kaplan-Meier analysis and the log-rank test was applied to analyze the difference survival groups. Univariate and multivariate Cox regression were performed to evaluate the independence of our risk model. Furthermore, the reliability of the risk model was evaluated through ROC analysis. P value <0.05 was considered statistically significant for each analysis performed.
Results
Identification of radioresponse-related genes in CESC patients treated with radical radiotherapy
The study’s infographic flowchart is displayed in Figure 1. A total of 92 cases of CESC patients underwent radical radiotherapy were selected for the analysis. It should be noted that only 57 people received an evaluation of the curative impact. Of these, 36 patients were evaluated as CR, seven patients were evaluated as PR, two patients were classified as SD, and 12 patients were classified as RPD. We used edgeR (R software version 4.2.1) package to compare the differential expression of mRNAs between the CR group and RPD group (|log2FC| >1.0 and FDR <0.05) in order to identify radioresponse-related mRNAs in CESC patients treated with radical radiotherapy. As a result, 49 mRNAs with high expression and 359 mRNAs with low expression were identified (available online: https://cdn.amegroups.cn/static/public/10.21037tcr-23-1772-1.pdf). The radioresponse-related mRNAs between the CR and RPD groups differed significantly, as shown in Figure 2A,2B.
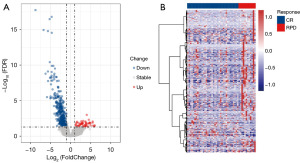
Functional analysis of DEGs
ClusterProfiler was performed to analyze the 408 DEGs. The result showed that DEGs were mostly enriched in the BP of muscle system process, muscle contraction and muscle tissue development (Figure 3A). Furthermore, the main MFs of these DEGs were related to channel activity, passive transmembrane transporter activity, and receptor ligand activity (Figure 3B). With regard to CCs, the DEGs were primarily enriched in the basal part of the cell, transporter complex, and basal plasma membrane (Figure 3C). The KEGG pathway analysis showed that DEGs were mainly enriched in Wnt signaling pathway (Figure 3D).
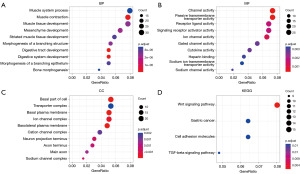
Construction of the radioresponse-related genes risk score model
Given the close association between radiotherapy response and prognosis for CESC patients, we constructed a prognostic model based on the radioresponse-related genes. Initially, the all cases were divided randomly into a training cohort (n=46) and a test cohort (n=46). Subsequently, using the univariate Cox method on the training cohort, we identified 194 representative prognostic radioresponse-related genes that were significantly correlated with survival (Table S2). These genes were chosen for LASSO Cox regression analysis, and the top-performing model comprised of ten genes, namely protein sprouty homolog 4 (SPRY4), pentraxin-3 (PTX3), leucine-rich repeat-containing protein 66 (LRRC66), prokineticin-2 (PROK2), prolyl 4-hydroxylase subunit alpha-3 (P4HA3), ceramide synthase 4 (CERS4), protein naked cuticle homolog 2 (NKD2), phorbolin-2/3 (APOBEC3B), solute carrier family 4 member 11 (SLC4A11), and fibromodulin (FMOD). Then, these ten genes were further optimized by the multivariate Cox regression method, and five genes (PTX3, LRRC66, CERS4, SLC4A11, and FMOD) were finally obtained (Figure 4A-4C). The risk score model was established as follows: risk score = 0.7590697 × PTX3 + 3.4636239 × LRRC66 + (−1.6841630) × CERS4 + (−1.1667508) × SLC4A11 + 1.0525174 × FMOD. Then, all patients were classified into high- and low-risk score groups using the median risk value. Figure 4D showcases the arrangement of the risk score and OS of CESC patients treated with radical radiotherapy in the two risk groups. As showed in Figure 4E, our survival analysis revealed a significant difference between the two risk groups in CESC patients who received radical radiotherapy, with the low-risk group exhibiting more favorable outcomes compared to the high-risk group. The ROC plots were utilized to assess the dependability of the model, and it was indicated that the area under the ROC curve (AUC) values for 1-, 3-, and 5-year OS rates were 0.983, 0.987, and 0.966 respectively, indicating the strong predictive potential of the signature, as demonstrated in Figure 4F.
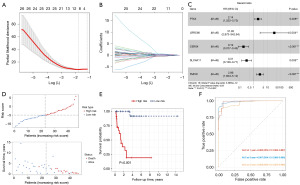
To evaluate the validity and reliability of the radioresponse-related genes risk score model, Kaplan-Meier analysis and ROC analysis were also performed on both the test cohort and the entire cohort. The risk score distribution and OS outcomes between the high- and low-risk groups in both the test cohort and the entire cohort are displayed in Figure 5A,5B, respectively. The results were consistent with those from the training cohort, with the low-risk group having a better survival outcome compared to the high-risk group, as illustrated in the test cohort (Figure 5C) and the entire cohort (Figure 5D). Additionally, the AUC values for both the test cohort and the entire cohort are shown in Figure 5E,5F, respectively, further emphasizing the robustness of the predictive signature.
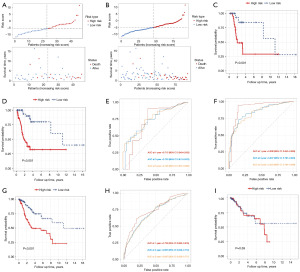
Furthermore, we evaluated the performance of this radioresponse-related gene risk score model with additional patient cohorts. The results revealed that the low-risk group had significantly better survival outcomes than the high-risk group for all CC patients (Figure 5G), and the AUC of 1-, 3-, and 5-year OS rates were 0.733, 0.697, and 0.697, respectively (Figure 5H). However, no statistically significant difference was observed between the two groups in patients who did not receive radical radiotherapy (Figure 5I). These results also further confirmed the specificity of our risk score in patients receiving radical radiotherapy.
The correlation between the signature and clinical characteristics in CESC patients treated with radical radiotherapy
To assess the clinical potential of our signature, clinical association analysis was conducted. The heatmap exhibited that the risk score signature was significantly associated with patient status (P=1.7e−07) and radiotherapy response (P=4.3e−06), as shown in Figure 6A-6E. Our results indicated that cases of death and radiographic progression exhibited significantly higher risk scores compared to cases of survival and CR, respectively (Figure 6D,6E).
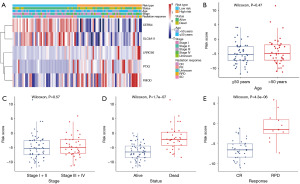
Univariate and multivariate Cox regression analyses
Univariate and multivariate Cox regression analyses were performed to examine whether our signature was an independent prognostic signature for CESC patients treated with radical radiotherapy. The factors considered in the analysis included age, International Federation of Gynecology and Obstetrics (FIGO) stage, and the risk score. The results showed that the risk score and FIGO stage were the independent risk factors, indicating that the risk score could predict the prognosis of CESC patients treated with radical radiotherapy independently (Figure 7A,7B).
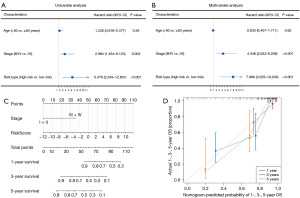
Nomogram construction
Then, a nomogram model was formulated on the basis of significant independent prognostic signatures comprising the FIGO stage and risk score, as illustrated in Figure 7C. The outcomes revealed that our nomogram exhibited impressive capability in anticipating the 1-, 3-, and 5-year OS of CESC patients subjected to radical radiotherapy, depicted in Figure 7D.
Expression profiles and prognostic capability of the five radioresponse-related mRNAs
We preliminary evaluated the expression patterns and prognostic potential of these five radioresponse-related mRNAs. As exhibited by Figure 8, PTX3, LRRC66 and FMOD were significantly upregulated in the RPD group (Figure 8A-8C), whereas CERS4 and SLC4A11 were significantly downregulated in the RPD group (Figure 8D,8E). Based on the dataset of CESC patients who underwent radical radiotherapy, Kaplan-Meier analysis indicated that PTX3, LRRC66, and FMOD were unfavorable prognostic factors (Figure 8F-8H), while CERS4 and SLC4A11 were favorable prognostic factors (Figure 8I,8J).
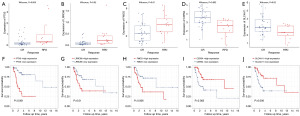
Immune infiltration analysis
A comprehensive analysis was conducted to examine immune infiltration and the tumor immune microenvironment in 92 CESC patients who had undergone radical radiotherapy. The CIBERSORT package was employed to analyze the immune infiltration of 22 immune cells. Results revealed that T cells CD4 memory resting was comparatively up-regulated, whereas macrophages M2, T cells CD4 memory activated, and T cells gamma delta were down-regulated in the high-risk group (Figure 9A). Correlation analysis revealed that the risk score was significantly associated with T cells CD4 memory resting, T cells CD4 memory activated, and T cells gamma delta (Figure 9B-9E). Macrophages M2 (Figure 9F) and T cells gamma delta (Figure 9G) demonstrated no prognostic value in CESC patients who had undergone radical radiotherapy. However, high infiltration of T cells CD4 memory activated (Figure 9H) or low infiltration of T cells CD4 memory resting (Figure 9I) was associated with favorable survival outcomes. These findings indicated that the inhibition of T cell immunity may impact the prognosis of the high-risk group in these patients. Finally, we used GSEA to explore the immune-related BP between the high- and low-risk groups. As shown in Figure 10A-10E, negative regulation of immune response, innate immune response, T cell proliferation, CD4 positive T cell activation and CD4 positive T cell proliferation were significantly enriched in high-risk group.
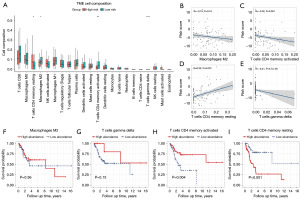
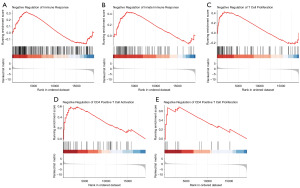
Discussion
CC is a prevalent malignancy among women, with cervical squamous cell carcinoma (CESC) comprising 10–15% of all female cancer-related mortalities and being the second most fatal malignancy in women (1,2). The primary treatment options for CC include surgery, radiation therapy, and chemotherapy. For patients with locally advanced or inoperable disease, radiotherapy or concurrent chemoradiotherapy is the standard radical treatment (3,16). Despite this, the prognosis for CESC patients treated with radical radiotherapy remains poor, with local recurrence and distant metastasis being the primary causes of treatment failure (3,4,17). Hence, there is a pressing need for the identification of novel biomarkers for the prediction of prognosis and sensitivity to radiotherapy in these patients. mRNAs, which encode proteins involved in numerous cellular processes, hold a pivotal position in the progression, relapse, and spreading of cancerous cells. Given their central role in cellular metabolic processes, the selection of appropriate mRNA biomarkers is of significant importance (18-20). Single biomarker may not fully capture the heterogeneity and complexity of the tumor, and thus a multi-parameter approach is necessary to achieve more accurate predictions. However, the previous prediction models were focused on all CESC patients, neglecting the prognostic differences under different treatment modalities, leading to poor predictive accuracy. In addition, considering other studies that take into account additional functional backgrounds such as immune-related factors, tumor microenvironment-related factors, DNA damage repair-related factors, and necroptosis-related factors when screening candidate genes, these selected genes may not possess the most optimal prognostic predictive effect (5-7). In this study, we have constructed a multivariate tool to predict the prognosis and sensitivity of radiation therapy and to guide appropriate treatment for CESC patients treated with radical radiotherapy.
We carried out a thorough analysis of the TCGA datasets to develop a novel radioresponse-related mRNAs signature that is able to precisely identify patients at high risk among CESC patients who undergo radical radiotherapy. Firstly, we obtained 408 radioresponse-related mRNAs with differential expression from 92 CESC patients who received radical radiotherapy. Using univariate Cox regression analysis, LASSO Cox regression analysis, and multivariate Cox regression analysis, we screened five radioresponse-related mRNAs in the training set and established a robust five-gene prognostic signature (PTX3, LRRC66, CERS4, SLC4A11, and FMOD). Kaplan-Meier analysis revealed a significant difference between the two risk groups, with the high-risk group exhibiting more unfavorable outcomes compared to the low-risk group. We employed both the test cohort and the entire cohort to validate the aforementioned outcomes. Additionally, we formulated a nomogram model by integrating the signature with other relevant clinical factors. Our risk score possessed an advantageous conformity and superior predictive ability in comparison to other clinical features validated by the calibration plots. We also found that the low-risk group had significantly better survival outcomes than the high-risk group for all CC patients, but no statistically significant difference was observed between the two groups in patients who did not receive radical radiotherapy. In addition, we preliminary evaluated the prognostic potential of these five radioresponse-related mRNAs, Kaplan-Meier analysis indicated that PTX3, LRRC66, and FMOD were unfavorable prognostic factors, while CERS4 and SLC4A11 were favorable prognostic factors.
Among these five genes mentioned above, PTX3 plays an important role in various biological mechanisms including regulation of inflammation, immunity response, angiogenesis, and tumor progression (21-23). It had been reported that PTX3 contributes to tumorigenesis and metastasis of human CC cells (24). Despite the lack of studies examining the effects of the remaining four genes on CC, they have been shown to be involved in the development of other types of cancer. FMOD is known to exert significant influence on the modulation of various BPs such as angiogenesis, transforming growth factor-β (TGF-β) activity, human fibroblast differentiation into pluripotent cells, inflammatory mechanisms, apoptosis, and metastatic-related phenotypes (25). FMOD drives oral squamous cell carcinoma progression by the activation of the EGFR signaling axis (26). CESR4 mRNA levels have been found to be decreased in advanced, metastatic tumors of head and neck squamous cell carcinoma, melanoma, and renal cell carcinoma patients (27). However, CESR4 overexpression promotes the progression and invasiveness of breast cancer by activating multiple signaling pathways associated with cancer, including Akt/mTOR, NF-κB, and β-catenin, as well as inducing epithelial-mesenchymal transition (28). While there is no direct research on the role of LRRC66, another member of this family, leucine-rich repeat-containing protein 59 (LRRC59), has been found to be associated with the metastatic potential of breast cancer (29). And LRRC59 has also been reported to be a poor prognosis factor for breast cancer (30). The expression of SLC4A11 has been recognized as a potential risk factor in ovarian cancer and recent study found evidence of an association between high expression of SLC4A11 and poor outcomes in colon cancer (31). The association of these genes with CESC still needs further study. However, further investigation is required to explore the correlation between these genes and CESC.
For patients with inoperable or locally advanced CC, radical radiotherapy or concurrent chemoradiotherapy represents the standard radical treatment. Despite this approach having remained unchanged for several decades, the prognosis for these patients remains poor. Immunotherapy, which represents an effective cancer treatment, may offer an alternative to traditional therapy (32). The integration of radiotherapy and immunotherapy has exhibited enhanced therapeutic results, attributed to the pivotal contribution of the immune microenvironment in cancer pathogenesis along with the response of cancer patients to radiotherapy (33-35). Our immune infiltration analysis showed increased activated memory CD4 T cells and decreased resting memory CD4 T cells in the low-risk group, which had a superior prognosis compared to the high-risk group based on the risk score using five radioresponse-related genes in CESC patients treated with radical radiotherapy. Previous research has revealed that the distribution level of CD4 T cells is predictive of radiotherapy response (36-38). Therefore, CD4 T cells are potentially involved in the regulation of radiotherapy response in CESC, leading to a better survival outcome in the low-risk group. However, an in-depth investigation is warranted to elucidate the underlying mechanism between memory CD4 T cells and the prognosis of CESC patients who undergo radiotherapy.
Our study has certain limitations that deserve attention. Firstly, the research cohorts employed in this study were exclusively sourced from the TCGA database. Consequently, it is imperative to authenticate our signature using comprehensive clinical cohort data or other external datasets. Moreover, the screening of the radioresponse-related module was based on a limited sample size of 57 individuals with clear records of radiotherapy response. Therefore, further investigations involving larger sample sizes and more comprehensive information regarding radiotherapy response are warranted.
Conclusions
In conclusion, the present study has identified five genes that exhibit a potential correlation with the radiotherapy response of CESC patients. The risk score model, based on the expression levels of five radioresponse-related genes (PTX3, LRRC66, CERS4, SLC4A11, and FMOD), has demonstrated its reliability in predicting the prognosis of CESC patients who have undergone radical radiotherapy. This prognostic model provides a valuable tool for predicting the prognosis of CESC patients with radical radiotherapy.
Acknowledgments
We acknowledge TCGA database for providing their platforms and contributors for uploading their meaningful datasets.
Funding: This work was supported by
Footnote
Reporting Checklist: The authors have completed the TRIPOD reporting checklist. Available at https://tcr.amegroups.com/article/view/10.21037/tcr-23-1772/rc
Peer Review File: Available at https://tcr.amegroups.com/article/view/10.21037/tcr-23-1772/prf
Conflicts of Interest: All authors have completed the ICMJE uniform disclosure form (available at https://tcr.amegroups.com/article/view/10.21037/tcr-23-1772/coif). The authors have no conflicts of interest to declare.
Ethical Statement: The authors are accountable for all aspects of the work in ensuring that questions related to the accuracy or integrity of any part of the work are appropriately investigated and resolved. The study was conducted in accordance with the Declaration of Helsinki (as revised in 2013).
Open Access Statement: This is an Open Access article distributed in accordance with the Creative Commons Attribution-NonCommercial-NoDerivs 4.0 International License (CC BY-NC-ND 4.0), which permits the non-commercial replication and distribution of the article with the strict proviso that no changes or edits are made and the original work is properly cited (including links to both the formal publication through the relevant DOI and the license). See: https://creativecommons.org/licenses/by-nc-nd/4.0/.
References
- Siegel RL, Miller KD, Wagle NS, et al. Cancer statistics, 2023. CA Cancer J Clin 2023;73:17-48. [Crossref] [PubMed]
- Ojesina AI, Lichtenstein L, Freeman SS, et al. Landscape of genomic alterations in cervical carcinomas. Nature 2014;506:371-5. [Crossref] [PubMed]
- Cohen PA, Jhingran A, Oaknin A, et al. Cervical cancer. Lancet 2019;393:169-82. [Crossref] [PubMed]
- Larionova I, Rakina M, Ivanyuk E, et al. Radiotherapy resistance: identifying universal biomarkers for various human cancers. J Cancer Res Clin Oncol 2022;148:1015-31. [Crossref] [PubMed]
- Zuo Z, Xiong J, Zeng C, et al. Exploration of a Robust and Prognostic Immune Related Gene Signature for Cervical Squamous Cell Carcinoma. Front Mol Biosci 2021;8:625470. [Crossref] [PubMed]
- Xiang X, Kang J, Jiang J, et al. A novel DNA damage repair-related gene signature predicting survival, immune infiltration and drug sensitivity in cervical cancer based on single cell sequencing. Front Immunol 2023;14:1198391. [Crossref] [PubMed]
- Xing X, Tian Y, Jin X. Immune infiltration and a necroptosis-related gene signature for predicting the prognosis of patients with cervical cancer. Front Genet 2023;13:1061107. [Crossref] [PubMed]
- Cancer Genome Atlas Research Network. The Cancer Genome Atlas Pan-Cancer analysis project. Nat Genet 2013;45:1113-20. [Crossref] [PubMed]
- Hutter C, Zenklusen JC. The Cancer Genome Atlas: Creating Lasting Value beyond Its Data. Cell 2018;173:283-5. [Crossref] [PubMed]
- Robinson MD, McCarthy DJ, Smyth GK. edgeR: a Bioconductor package for differential expression analysis of digital gene expression data. Bioinformatics 2010;26:139-40. [Crossref] [PubMed]
- Yu G, Wang LG, Han Y, et al. clusterProfiler: an R package for comparing biological themes among gene clusters. OMICS 2012;16:284-7. [Crossref] [PubMed]
- Friedman J, Hastie T, Tibshirani R. Regularization Paths for Generalized Linear Models via Coordinate Descent. J Stat Softw 2010;33:1-22. [Crossref] [PubMed]
- Li M, Spakowicz D, Burkart J, et al. Change in neutrophil to lymphocyte ratio during immunotherapy treatment is a non-linear predictor of patient outcomes in advanced cancers. J Cancer Res Clin Oncol 2019;145:2541-6. [Crossref] [PubMed]
- Li W, Liu J, Zhao H. Identification of a nomogram based on long non-coding RNA to improve prognosis prediction of esophageal squamous cell carcinoma. Aging (Albany NY) 2020;12:1512-26. [Crossref] [PubMed]
- Chen B, Khodadoust MS, Liu CL, et al. Profiling Tumor Infiltrating Immune Cells with CIBERSORT. Methods Mol Biol 2018;1711:243-59. [Crossref] [PubMed]
- Jiang Z, Zhang G, Sun T, et al. Advantages of IMRT optimization with MCO compared to IMRT optimization without MCO in reducing small bowel high dose index for cervical cancer patients-individualized treatment options. Transl Cancer Res 2023;12:3255-65. [Crossref] [PubMed]
- Guaraldi L, Pastina P, Tini P, et al. Locally advanced cervical cancer treated with chemo-radiotherapy: a case report of a particular recurrence. Gynecol Pelvic Med 2021;4:30. [Crossref]
- Wieczorek E, Reszka E. mRNA, microRNA and lncRNA as novel bladder tumor markers. Clin Chim Acta 2018;477:141-53. [Crossref] [PubMed]
- Huang BL, Wei LF, Lin YW, et al. Serum IGFBP-1 as a promising diagnostic and prognostic biomarker for colorectal cancer. Sci Rep 2024;14:1839. [Crossref] [PubMed]
- Liu YY, Zhu WH, Li W, et al. Identifying the risk factors and developing a predictive model for postoperative pelvic floor dysfunction in cervical cancer patients. Transl Cancer Res 2023;12:1307-14. [Crossref] [PubMed]
- Chen FW, Wu YL, Cheng CC, et al. Inactivation of pentraxin 3 suppresses M2-like macrophage activity and immunosuppression in colon cancer. J Biomed Sci 2024;31:10. [Crossref] [PubMed]
- Zajkowska M, Mroczko B. The Role of Pentraxin 3 in Gastrointestinal Cancers. Cancers (Basel) 2023;15:5832. [Crossref] [PubMed]
- Zhang H, Wang Y, Zhao Y, et al. PTX3 mediates the infiltration, migration, and inflammation-resolving-polarization of macrophages in glioblastoma. CNS Neurosci Ther 2022;28:1748-66. [Crossref] [PubMed]
- Ying TH, Lee CH, Chiou HL, et al. Knockdown of Pentraxin 3 suppresses tumorigenicity and metastasis of human cervical cancer cells. Sci Rep 2016;6:29385. [Crossref] [PubMed]
- Pourhanifeh MH, Mohammadi R, Noruzi S, et al. The role of fibromodulin in cancer pathogenesis: implications for diagnosis and therapy. Cancer Cell Int 2019;19:157. [Crossref] [PubMed]
- Xia L, Zhang T, Yao J, et al. Fibromodulin overexpression drives oral squamous cell carcinoma via activating downstream EGFR signaling. iScience 2023;26:108201. [Crossref] [PubMed]
- Brachtendorf S, El-Hindi K, Grösch S. Ceramide synthases in cancer therapy and chemoresistance. Prog Lipid Res 2019;74:160-85. [Crossref] [PubMed]
- Kim SJ, Seo I, Kim MH, et al. Ceramide synthase 4 overexpression exerts oncogenic properties in breast cancer. Lipids Health Dis 2023;22:183. [Crossref] [PubMed]
- Kim J, Kang J, Kang YL, et al. Ketohexokinase-A acts as a nuclear protein kinase that mediates fructose-induced metastasis in breast cancer. Nat Commun 2020;11:5436. [Crossref] [PubMed]
- Zhang P, Xie X, Li C, et al. LRRC59 serves as a novel biomarker for predicting the progression and prognosis of bladder cancer. Cancer Med 2023;12:19758-76. [Crossref] [PubMed]
- Qin L, Li T, Liu Y. High SLC4A11 expression is an independent predictor for poor overall survival in grade 3/4 serous ovarian cancer. PLoS One 2017;12:e0187385. [Crossref] [PubMed]
- Zhang Y, Zhang Z. The history and advances in cancer immunotherapy: understanding the characteristics of tumor-infiltrating immune cells and their therapeutic implications. Cell Mol Immunol 2020;17:807-21. [Crossref] [PubMed]
- Arina A, Gutiontov SI, Weichselbaum RR. Radiotherapy and Immunotherapy for Cancer: From "Systemic" to "Multisite". Clin Cancer Res 2020;26:2777-82. [Crossref] [PubMed]
- Feng CH, Mell LK, Sharabi AB, et al. Immunotherapy With Radiotherapy and Chemoradiotherapy for Cervical Cancer. Semin Radiat Oncol 2020;30:273-80. [Crossref] [PubMed]
- Cao Y, Ding S, Hu Y, et al. An Immunocompetent Hafnium Oxide-Based STING Nanoagonist for Cancer Radio-immunotherapy. ACS Nano 2024;18:4189-204. [Crossref] [PubMed]
- Liu C, Hu Q, Xu B, et al. Peripheral memory and naïve T cells in non-small cell lung cancer patients with lung metastases undergoing stereotactic body radiotherapy: predictors of early tumor response. Cancer Cell Int 2019;19:121. [Crossref] [PubMed]
- Ben Khelil M, Godet Y, Abdeljaoued S, et al. Harnessing Antitumor CD4(+) T Cells for Cancer Immunotherapy. Cancers (Basel) 2022;14:260. [Crossref] [PubMed]
- Yasuda K, Nirei T, Sunami E, et al. Density of CD4(+) and CD8(+) T lymphocytes in biopsy samples can be a predictor of pathological response to chemoradiotherapy (CRT) for rectal cancer. Radiat Oncol 2011;6:49. [Crossref] [PubMed]