Anoikis-related gene signatures predict prognosis of lung adenocarcinoma patients and reveal immune infiltration
Highlight box
Key findings
• In this study, anoikis-related genes (ARGs) were used to construct a risk model to predict the survival and prognosis of lung adenocarcinoma (LUAD) patients, and it was found that this model could sufficiently predict the survival and prognosis of LUAD patients, and reverse transcription quantitative polymerase chain reaction was used for verification.
What is known and what is new?
• Anoikis is a programmed cell death mode, and ARGs have been found to have a catalytic effect in lung adenocarcinoma, gastrointestinal and other tumors.
• Our study established a model that can sufficiently predict the prognosis of LUAD patients.
What is the implication, and what should change now?
• Our study provides potential therapeutic targets for early treatment of LUAD patients and improves early prognosis of LUAD patients.
Introduction
The second most diagnosed cancer in the world is lung cancer, which is a major contributor to death among cancer patients (1). The most common type of lung cancer is lung adenocarcinoma (LUAD), which accounts for almost 40% of all lung cancers (2). Although advances in diagnosis and treatment have been made in the past decade, the total survival rate of LUAD patients is still unsatisfactory, and the average five-year survival rate is no more than 20% (3). Consequently, it is important to find more new biomarkers to help in the prediction of prognosis in early-stage LUAD patients to enable clinicians to provide timely treatment to their patients.
Anoikis is a mode of programmed cell death (PCD) that occurs due to loss of attachment or inappropriate attachment of tumor cells onto the extracellular matrix (ECM) and neighboring cells (4). Cancer cell resistance to anoikis is essential for cancer cell metastasis, which allows cancer cells to survive in the systemic circulation, thereby leading to the formation of secondary tumors in distant organs (5). Anoikis resistance is a key process in cancer cell metastasis in LUAD (6,7).
In our study, we explored the role and effects of anoikis-related genes (ARGs) on the prognosis of LUAD and developed an ARG-based prognostic scoring model. We also validated the associated prognostic genes using reverse transcription quantitative polymerase chain reaction (RT-qPCR). We present this article in accordance with the TRIPOD reporting checklist (available at https://tcr.amegroups.com/article/view/10.21037/tcr-23-2185/rc).
Methods
Sources of clinical and transcriptome data
Transcriptome and clinical data of 226 LUAD tissues, 539 LUAD tissues and 59 normal tissues were downloaded from the Gene Expression Omnibus (GSE31210) data portal (https://www.ncbi.nlm.nih.gov/geo/) and The Cancer Genome Atlas (TCGA)-LUAD database (https://portal.gdc.cancer.gov), respectively.
Anoikis gene acquisition
We downloaded 469 ARGs from the Harmonizome portals (8) and GeneCards databases (9) (https://www.genecards.org). Furthermore, we identified 129 differentially expressed genes (DEGs) in the TCGA-LUAD cohort by the “limma” R package.
Consensus clustering
We applied the k-means method and consensus clustering to identify the different anoikis-related modes associated with the expressed anoikis regulators. Then, we verified the reliability of the clustering by unified modal approximation and projection (UMAP) using the “ggplot2” R package.
Gene functional enrichment analysis
“c2.cp.kegg.v2022.1.Hs.symbols.gmt” was downloaded from the Molecular Signatures Database (MsigDB) for use in the gene set variation analysis (GSVA). We used the “GSVA” R package to carry out GSVA analysis (10).
Prognostic model of ARGs
Genes relevant to survival were selected by univariate Cox regression analysis; thereafter, least absolute shrinkage and selection operator (LASSO) regression analysis was performed by the “glmnet” R package, with a penalization regularization parameter λ being identified via 10-fold cross-validation. Thereafter, identification of core genes and calculation of the respective coefficients were performed by multivariate Cox regression models. According to the optimal λ values and the respective coefficients, six ARGs were selected to develop risk signatures. For each patient, the following is the risk score calculation for the new ARG signature. Risk score = e^ (...... corresponding coefficients +...... + CDX2 expression), where Coe and Exp correspond to the coefficient of risk and expression level per gene. Time-dependent receiver operating characteristic (ROC) curves and Kaplan-Meier (KM) survival curve analyses were conducted to assess the predictive power of the model. Overall, LASSO analysis and univariate Cox regression identified six anoikis-related DEGs that showed a significant correlation with overall survival (OS) in the GSE31210 cohort, which we verified in the TCGA-LUAD cohort.
Association of risk score with immune cell infiltration
For the quantification of the relative proportions of infiltrating immune cells, we employed single sample gene set enrichment analysis (ssGSEA) and CIBERSORT R scripts (11). CIBERSORT was employed to estimate the percentages of all immune cell types between the low- and high-risk cohorts. For each sample, all assessed immune cell type scores were summed to be 1. Additionally, the relation of risk score values to immune infiltrating cells was explored using Spearman rank association analysis.
Construction and assessment of the predictive nomogram
Risk scores and clinicopathological features were applied to construct the nomogram. The calibration charts were internally validated to certify their accuracies. The time-C indices were employed to verify the predictive properties of this nomogram. Evaluation of the net clinical benefit was performed by decision curve analysis (DCA) (12).
Acquisition of tumor immune single-cell information
We obtained the immune single-cell dataset NSCLC_GSE127465 from the Tumor Immunization Single Cell Hub (TISCH; http://tisch.comp-genomics.org).
Analysis of the expression of prognostic genes
To confirm the expression of prognostic genes in LUAD, we implemented RT-qPCR. The study was conducted in accordance with the Declaration of Helsinki (as revised in 2013). Five carcinoma side normal tissue and five tumor samples were obtained from patients with individual consent waived from The First Hospital of Lanzhou University, and this study was approved by the ethics committee of The First Hospital of Lanzhou University (No.: LDYYLL2023-263). Total RNA was extracted from ten samples using TRIzol (Ambion, Austin, USA) following the manufacturer’s guidelines. Subsequently, the RNA underwent reverse transcription to cDNA using the First-strand-cDNA-synthesis-kit (Servicebio, Wuhan, China) as per the provided instructions. RT-qPCR was then conducted using the 2xUniversal Blue SYBR Green qPCR Master Mix (Servicebio) according to the manufacturer’s specifications. The sequences of the PCR primers can be found in Table S1. GAPDH served as an internal reference gene, and expression levels were determined employing the 2−ΔΔCt method (13).
Statistical analysis
We used R software v4.2.1 for statistical analysis and data visualization, and P values <0.05 were deemed statistically significant.
Results
Analysis of ARGs associated with prognosis
We downloaded a total of 640 ARGs from the GeneCards and Harmonizome portals. Then, we compared normal tissues with TCGA-LUAD and identified 129 DEGs (Figure 1A). Next, the “LUAD-GSE31210” cohort with a total of 17,662 retained genes was obtained by combining the TCGA-LUAD cohort with the GSE31210 cohort after removing the batch effect. Subsequently, we found that 64 of the 129 ARGs were related to survival by univariate Cox regression analysis and with statistical significance (P<0.05). The forest chart shows the first 32 ARGs (P<0.01) (Figure 1B). Except for DAPK2, KL, and CEACAM8, the remaining 29 genes were related to poor prognosis. Additionally, the network diagram revealed the relationships among the first 32 genes in terms of gene expression levels (Figure 1C). Moreover, copy number variants (CNVs) data were obtained from the TCGA database to determine the chromosomal alterations of these ARGs and the location of each gene on the chromosome (Figure 1D,1E). We can see that the most significant “gain” of the S100A7 change is located on chromosome 1, while the main “loss” of CDKX2 and KL is located on chromosome 13 (Figure 1E).
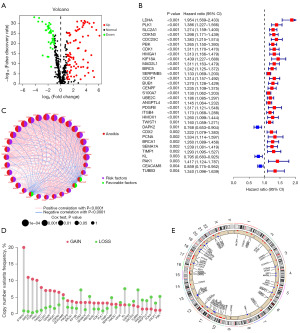
Consistent clustering of molecular subgroups of LUAD using ARGs
We conducted consensus clustering of 64 DEGs associated with prognosis (P<0.05) using the R package “ConsensusClusterPlus”. Then, we observed that the cohort could be divided into two subtypes when k=2 (Figure 2A), and prognosis was significantly different (P<0.01) between the 2 subtypes as revealed by OS analysis (Figure 2B). The result plots of UMAP and t-distributed Stochastic Neighbor Embedding (tSNE) showed that at k=2, the two clustering subtypes were clearly distinguished (Figure 2C,2D). The heat map depicted in Figure 2E illustrates a low expression of S100A7 in the majority of the two subtypes, suggesting that S100A7 may be a good prognostic factor. Finally, we used the R package “GSVA” to analyze the differences in the enrichment levels of the Kyoto Encyclopedia of Genes and Genomes (KEGG) pathways between two subtypes (Figure 2F).
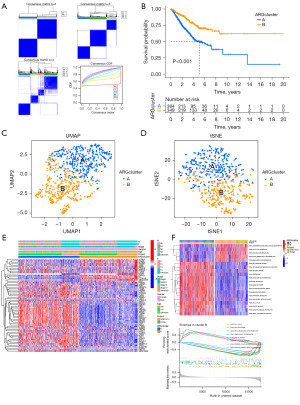
Gene-expression pattern and immune-cell infiltration in two subtypes
We can see from Figure 3A that NTRK2, DAPK2, BDNF, ZEB2, ITGA8, KL, GDF2, CEACAM8, CLDN18 and IRX1 expression were higher in Cluster B than in Cluster A; high expression patterns were also shown for other ARGs. Moreover, ssGSEA results showed a significantly lower proportion of activated CD4 and regulatory T cells in population B than in population A (Figure 3B); other immune cell infiltration levels were also significantly different.
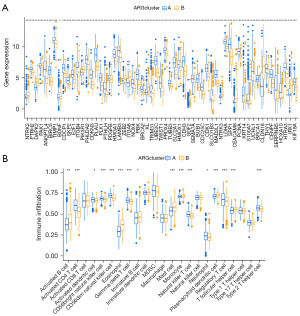
Construction and validation of anoikis-related prognostic signatures
In the univariate Cox regression analysis, we identified seven ARGs associated with the OS rate using the LASSO analysis (Figure 4A,4B). In the ensuing multivariate Cox regression analysis, six ARGs were independently selected as predictors for the prognosis of LUAD, establishing the risk model. In Table S2, the correlation coefficients based on the six ARGs characteristic are listed. The risk score is calculated as follows: Risk score = (0.300 × level of PDGFB expression) + (0.160 × level of HMOX1 expression) + (0.521 × level of GDF2 expression) + (0.582 × level of LDHA expression + (0.068 × level of S100A7 expression) + (0.232 × level of CDX2 expression). The data were divided into train and test groups and the samples from each group were divided into high and low-risk groups according to the median value of the risk scores. The K-M curves revealed a worse prognosis for patients in high-risk group in both the train and test groups (Figure 4C,4D). This model showed good predictive performance for the time-dependent ROC curves for OS at 1, 3, and 5 years in both the train and test groups (Figure 4E,4F). As seen in Figure 4G, the risk scores are clearly different in the two subtypes, and Figure 4H shows the differences in ARGs clusters, risk scores and life status. We visualized the expression of the six prognostic ARGs in high-risk and low-risk groups by heatmap and could see that GDF2, CDX2, PDGFB, HMOX1, LDHA, and S100A7 had higher expression in the high-risk group (Figure 4I).
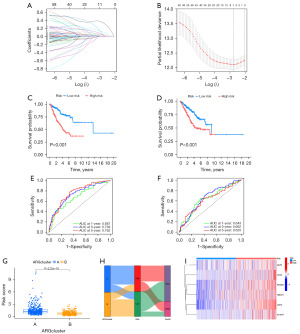
Analysis of immune activity in LUAD
Tumor cells may have inherent resistance to cancer therapy, often conferred by nonmalignant cells that constitute the tumor microenvironment (TME) (14). Therefore, we further investigated the TME in both high and low-risk groups, and the “CIBERSORT” R script was used to measure the relative proportion of immune cell infiltrations. Figure 5A shows the relative percentage of each immune cell that corresponds with their risk score. As seen in Figure 5B, the macrophage M0 ratio became gradually larger with higher score (R=0.24). In addition, macrophages represent a large proportion of immune cells in LUAD patients (Figure 5C). This finding suggests that targeted therapy against macrophages could be a therapeutic approach for LUAD (14). We can use the correlation plot among immune cells from LUAD patients to better understand the TME composition (Figure 5D). In addition, six genes for model construction were associated with several immune cell infiltrations (Figure 5E). Moreover, in examining the stromal cell component of the TME, assessing immune cell infiltration levels, and estimating tumor purity, we computed immune scores, stromal scores, and estimation scores for the high-risk and low-risk groups based on the estimation of expression profiling scores (Figure 5F). Finally, the potential sensitivity to clinical agents was increased for both the high and low-risk groups (Figure S1).
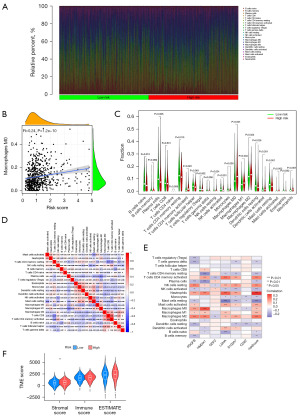
Prognostic nomogram for patients with LUAD
Based on the clinicopathological characteristics and risk scores of the ARGs, we developed a nomogram model that could complete the prediction of the survival probability at 1, 3 and 5 years for LUAD patients (Figure 6A). The nomogram construction relies on regression coefficients’ magnitudes for all predictors, establishing a scale that assigns scores based on each predictor’s value. In instances with multiple predictors, a cumulative score is calculated, facilitating the subsequent computation of the probability of correlation with the occurrence of a clinical outcome for each patient, determined by their total score. We used calibration plot to verify the accuracy of this nomogram (Figure 6B) and decision curve analysis (DCA) to evaluate whether this strategy would benefit patients. DCA showed that this nomogram was a good predictor for predicting both short-term and long-term survival of LUAD patients (Figure 6C-6E). We can see in the nomogram that the cumulative risk curves show a gradual increase in OS risk among the LUAD patients in the high-risk and low-risk groups (Figure 6F). Figure 6G shows that the main influencing factors in the nomogram. In conclusion, the nomogram based on risk score can be a valid method for clinical prediction of prognosis in LUAD patients.
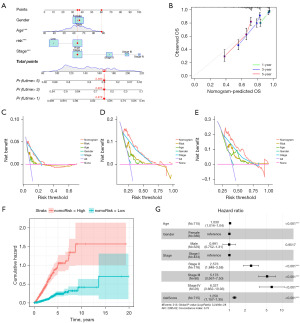
Analysis of tumor immune microenvironment
As shown in Figure 7A, 25 cell populations with 12 cell types are included in the NSCLC_GSE127465 dataset. HMOX1 is found mainly in malignant cells and immune cells, but is rarely expressed in fibroblasts. PDGFB is mainly expressed in endothelial cells, but is barely expressed in immune cells. GDF2 and CDX2 are barely detected in TME, LDHA is mainly expressed in immune cells and malignant cells. S100A7 is barely detected in TME (Figure 7B,7C).
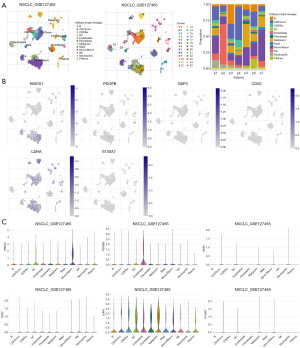
Expression validation of the prognostic genes
The expression of prognostic genes was analyzed in the training set (TCGA-LUAD). We observed higher expression of PDGFB, HMOX1 and GDF2 in the normal group than in the tumor group (Figure 8A). We further verified the expression trend by RT-qPCR experiments (Figure 8B).
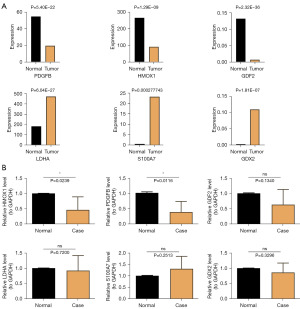
Discussion
Since patients with metastatic LUAD have a poor prognosis and a low five-year survival rate, early diagnosis is essential for improving survival time for patients with LUAD. However, although the novel prognostic model we developed with six ARGs had good predictive performance, the number of markers used is insufficient. Consequently, more screening biomarkers are urgently needed to help diagnose early LUAD and improve the survival rate of LUAD patients.
PDGFB is involved in the process of recruiting PDGF receptor β-positive pericytes to the vasculature (15-17). PDGFB has been reported to stimulate cell proliferation, survival and migration in a variety of tumor cells, including LUAD (18). The oncoprotein PDGFB has been reported to be essential for cancer proliferation and migration (19). These results suggest that PDGFB contributes to lung adenocarcinogenesis, which was consistent with our data indicating that PDGFB is highly expressed in high-risk groups. Given the significant role of the oncoprotein encoded by this gene in cancer cell proliferation and metastasis, further studies on PDGFB have the potential for clinical application.
HMOX1 could help promote tumor progression and metastasis in several cancers, including LUAD, and is expressed in malignant tumor cells and tumor associated macrophages (TAM) (20). HMOX1 is an anti-apoptotic, antioxidant and anti-inflammatory protein that promotes not only metabolic reprogramming but also antioxidant defense (21). Moreover, it is possible that HMOX1 can promote LUAD metastasis by affecting macrophages and mitochondrial complexes (22). In our data, HMOX1 was overexpressed in the high-risk group and the proportion of macrophages M0 increased progressively with increasing risk score, and we searched for new therapeutic approaches by investigating the effect of HMOX1 on TAM.
GDF2, also known as BMP9, belongs to the transforming growth factor (TGF)-β superfamily, mainly generated by the liver, and induces osteo/odontogenic differentiation of mesenchymal stem cells (MSCs) (23,24). Alk1 is a BMP receptor expressed on endothelial cells (25). It has been reported that GDF2 overexpression in LUAD tumors delays tumor growth and promotes vascular normalization in LUAD tumors, resulting in significant alterations in the TME characterized by reduced hypoxia and increased immune infiltration (26). In addition, GDF2 has been reported to increase anoikis sensitivity in epithelial cells (27). However, studies on the role of GDF2 in LUAD are scarce, and large-scale studies with more LUAD patients are still needed to confirm the relationship between GDF2 and LUAD.
LDHA is an essential member involved in glycolysis, catalyzing the conversion of pyruvate and NADH to lactate and NAD+ (28). LDHA tyrosine phosphorylation promotes cancer cell metabolism and tumor cell proliferation through the modulation of dysregulated NADH/NAD+ redox homeostasis of fibroblast growth factor receptor 1 (FGFR1) in LUAD cells (29). The phosphorylation of LDHA at Y10 has been proven to enhance the invasiveness and anti-inflammatory properties of cancer cells (30). LDHA is an emerging anticancer target that has an important role in regulating cancer metabolism and anoikis resistance. In our data, LDHA was overexpressed in the high-risk group, and inhibitors targeting LDHA have potential for clinical application in the treatment of LUAD.
Increased S100A7 protein expression has been described in lung cancer and correlates with poor prognosis (31), and its overexpression is significantly associated with the early stages of many cancers (32,33). However, the function of S100A7 expression in lung cancer tissues is not clear, S100A7 protein may act as a chemokine and serum marker for lung cancer (34). S100A7 has also been shown to promote the transdifferentiation of adenocarcinoma to squamous carcinoma in lung cancer cells (35). In addition, S100A7 enhances macrophage infiltration (36).
CDX2 is a transcriptional regulator of several genes responsible for cell proliferation, differentiation and migration (37). CDX2 expression reduction can lead to tumor metastasis (38). It has been reported that the synergistic effect of simultaneous deletion of CDX2 and NKX2-1 activates the metastatic program of LUAD (39). Moreover, CDX2 expression was found to be a reliable marker for determining the colorectal origin of pulmonary metastases (40). Recent reports suggest that CDX2 expression can be detected by RT-PCR and immunohistochemistry in primary LUAD (41).
For these six bases, we conducted RT-qPCR validation, and the PCR results were consistent with those analyzed in our experiment, which further confirmed the therapeutic potential of these six genes in LUAD.
TME research is meaningful in that it can help physicians develop new targeted therapeutic agents and observe tumor metastasis progression. Our analysis of the immune cell composition shows a significantly higher proportion of macrophages among the high-risk group, suggesting that macrophages have a critical effect in LUAD development. In addition, both S100A7 and HMOX1 were associated with macrophages, and in particular, HMOX1 showed the highest related coefficient to macrophage M0. Thus, the HMOX1/macrophage M0 axis may be an interesting pathway.
However, there are many limitations in this study. Due to the limited amount of data and cell-to-cell heterogeneity, we had problems calibrating predictive models and reflecting the accuracy of ARGs on the prognostic impact of LUAD patients. Moreover, the results of TME were obtained from data analysis. The role of anoikis in targeted therapy and immune microenvironment needs to be confirmed with further study.
Conclusions
Our novel 6-ARG nomogram model can sufficiently predict the survival of patients with early-stage LUAD, while the nomogram may help to develop personalized treatment plans for patients. We are confident that this nomogram model will serves as a good predictor of the survival of patients with LUAD, and the validated gene will provide an effective therapeutic target for the treatment of LUAD.
Acknowledgments
The authors thank AJE for their language assistance during the writing of this article.
Funding: This work was supported by
Footnote
Reporting Checklist: The authors have completed the TRIPOD reporting checklist. Available at https://tcr.amegroups.com/article/view/10.21037/tcr-23-2185/rc
Data Sharing Statement: Available at https://tcr.amegroups.com/article/view/10.21037/tcr-23-2185/dss
Peer Review File: Available at https://tcr.amegroups.com/article/view/10.21037/tcr-23-2185/prf
Conflicts of Interest: All authors have completed the ICMJE uniform disclosure form (available at https://tcr.amegroups.com/article/view/10.21037/tcr-23-2185/coif). The authors have no conflicts of interest to declare.
Ethical Statement:
Open Access Statement: This is an Open Access article distributed in accordance with the Creative Commons Attribution-NonCommercial-NoDerivs 4.0 International License (CC BY-NC-ND 4.0), which permits the non-commercial replication and distribution of the article with the strict proviso that no changes or edits are made and the original work is properly cited (including links to both the formal publication through the relevant DOI and the license). See: https://creativecommons.org/licenses/by-nc-nd/4.0/.
References
- Sung H, Ferlay J, Siegel RL, et al. Global Cancer Statistics 2020: GLOBOCAN Estimates of Incidence and Mortality Worldwide for 36 Cancers in 185 Countries. CA Cancer J Clin 2021;71:209-49. [Crossref] [PubMed]
- Denisenko TV, Budkevich IN, Zhivotovsky B. Cell death-based treatment of lung adenocarcinoma. Cell Death Dis 2018;9:117. [Crossref] [PubMed]
- Lin JJ, Cardarella S, Lydon CA, et al. Five-Year Survival in EGFR-Mutant Metastatic Lung Adenocarcinoma Treated with EGFR-TKIs. J Thorac Oncol 2016;11:556-65. [Crossref] [PubMed]
- Zhou X, Li L, Guo X, et al. HBXIP induces anoikis resistance by forming a reciprocal feedback loop with Nrf2 to maintain redox homeostasis and stabilize Prdx1 in breast cancer. NPJ Breast Cancer 2022;8:7. [Crossref] [PubMed]
- Paoli P, Giannoni E, Chiarugi P. Anoikis molecular pathways and its role in cancer progression. Biochim Biophys Acta 2013;1833:3481-98. [Crossref] [PubMed]
- Chunhacha P, Chanvorachote P. Roles of caveolin-1 on anoikis resistance in non small cell lung cancer. Int J Physiol Pathophysiol Pharmacol 2012;4:149-55. [PubMed]
- Prateep A, Sumkhemthong S, Karnsomwan W, et al. Avicequinone B sensitizes anoikis in human lung cancer cells. J Biomed Sci 2018;25:32. [Crossref] [PubMed]
- Rouillard AD, Gundersen GW, Fernandez NF, et al. The harmonizome: a collection of processed datasets gathered to serve and mine knowledge about genes and proteins. Database (Oxford) 2016;2016:baw100. [Crossref] [PubMed]
- Rebhan M, Chalifa-Caspi V, Prilusky J, et al. GeneCards: integrating information about genes, proteins and diseases. Trends Genet 1997;13:163. [Crossref] [PubMed]
- Hänzelmann S, Castelo R, Guinney J. GSVA: gene set variation analysis for microarray and RNA-seq data. BMC Bioinformatics 2013;14:7. [Crossref] [PubMed]
- Newman AM, Liu CL, Green MR, et al. Robust enumeration of cell subsets from tissue expression profiles. Nat Methods 2015;12:453-7. [Crossref] [PubMed]
- Vickers AJ, Cronin AM, Elkin EB, et al. Extensions to decision curve analysis, a novel method for evaluating diagnostic tests, prediction models and molecular markers. BMC Med Inform Decis Mak 2008;8:53. [Crossref] [PubMed]
- Livak KJ, Schmittgen TD. Analysis of relative gene expression data using real-time quantitative PCR and the 2(-Delta Delta C(T)) Method. Methods 2001;25:402-8. [Crossref] [PubMed]
- Cassetta L, Pollard JW. Targeting macrophages: therapeutic approaches in cancer. Nat Rev Drug Discov 2018;17:887-904. [Crossref] [PubMed]
- Lindahl P, Johansson BR, Levéen P, et al. Pericyte loss and microaneurysm formation in PDGF-B-deficient mice. Science 1997;277:242-5. [Crossref] [PubMed]
- Hellström M, Kalén M, Lindahl P, et al. Role of PDGF-B and PDGFR-beta in recruitment of vascular smooth muscle cells and pericytes during embryonic blood vessel formation in the mouse. Development 1999;126:3047-55. [Crossref] [PubMed]
- Soriano P. Abnormal kidney development and hematological disorders in PDGF beta-receptor mutant mice. Genes Dev 1994;8:1888-96. [Crossref] [PubMed]
- Bartoschek M, Pietras K. PDGF family function and prognostic value in tumor biology. Biochem Biophys Res Commun 2018;503:984-90. [Crossref] [PubMed]
- Roskoski R Jr. Sunitinib: a VEGF and PDGF receptor protein kinase and angiogenesis inhibitor. Biochem Biophys Res Commun 2007;356:323-8. [Crossref] [PubMed]
- Luu Hoang KN, Anstee JE, Arnold JN. The Diverse Roles of Heme Oxygenase-1 in Tumor Progression. Front Immunol 2021;12:658315. [Crossref] [PubMed]
- Biswas C, Shah N, Muthu M, et al. Nuclear heme oxygenase-1 (HO-1) modulates subcellular distribution and activation of Nrf2, impacting metabolic and anti-oxidant defenses. J Biol Chem 2014;289:26882-94. [Crossref] [PubMed]
- Chen B, Zhang L, Zhou H, et al. HMOX1 promotes lung adenocarcinoma metastasis by affecting macrophages and mitochondrion complexes. Front Oncol 2022;12:978006. [Crossref] [PubMed]
- Chen Q, Zheng L, Zhang Y, et al. Special AT-rich sequence-binding protein 2 (Satb2) synergizes with Bmp9 and is essential for osteo/odontogenic differentiation of mouse incisor mesenchymal stem cells. Cell Prolif 2021;54:e13016. [Crossref] [PubMed]
- Song D, Zhang F, Reid RR, et al. BMP9 induces osteogenesis and adipogenesis in the immortalized human cranial suture progenitors from the patent sutures of craniosynostosis patients. J Cell Mol Med 2017;21:2782-95. [Crossref] [PubMed]
- David L, Mallet C, Mazerbourg S, et al. Identification of BMP9 and BMP10 as functional activators of the orphan activin receptor-like kinase 1 (ALK1) in endothelial cells. Blood 2007;109:1953-61. [Crossref] [PubMed]
- Viallard C, Audiger C, Popovic N, et al. BMP9 signaling promotes the normalization of tumor blood vessels. Oncogene 2020;39:2996-3014. [Crossref] [PubMed]
- Varadaraj A, Patel P, Serrao A, et al. Epigenetic Regulation of GDF2 Suppresses Anoikis in Ovarian and Breast Epithelia. Neoplasia 2015;17:826-38. [Crossref] [PubMed]
- Bui T, Thompson CB. Cancer's sweet tooth. Cancer Cell 2006;9:419-20. [Crossref] [PubMed]
- Fan J, Hitosugi T, Chung TW, et al. Tyrosine phosphorylation of lactate dehydrogenase A is important for NADH/NAD(+) redox homeostasis in cancer cells. Mol Cell Biol 2011;31:4938-50. [Crossref] [PubMed]
- Jin L, Chun J, Pan C, et al. Phosphorylation-mediated activation of LDHA promotes cancer cell invasion and tumour metastasis. Oncogene 2017;36:3797-806. [Crossref] [PubMed]
- Zhang H, Wang Y, Chen Y, et al. Identification and validation of S100A7 associated with lung squamous cell carcinoma metastasis to brain. Lung Cancer 2007;57:37-45. [Crossref] [PubMed]
- Semprini S, Capon F, Bovolenta S, et al. Genomic structure, promoter characterisation and mutational analysis of the S100A7 gene: exclusion of a candidate for familial psoriasis susceptibility. Hum Genet 1999;104:130-4. [Crossref] [PubMed]
- Alowami S, Qing G, Emberley E, et al. Psoriasin (S100A7) expression is altered during skin tumorigenesis. BMC Dermatol 2003;3:1. [Crossref] [PubMed]
- Zhang H, Zhao Q, Chen Y, et al. Selective expression of S100A7 in lung squamous cell carcinomas and large cell carcinomas but not in adenocarcinomas and small cell carcinomas. Thorax 2008;63:352-9. [Crossref] [PubMed]
- Wang R, Li Y, Hu E, et al. S100A7 promotes lung adenocarcinoma to squamous carcinoma transdifferentiation, and its expression is differentially regulated by the Hippo-YAP pathway in lung cancer cells. Oncotarget 2017;8:24804-14. [Crossref] [PubMed]
- Lu Z, Zheng S, Liu C, et al. S100A7 as a potential diagnostic and prognostic biomarker of esophageal squamous cell carcinoma promotes M2 macrophage infiltration and angiogenesis. Clin Transl Med 2021;11:e459. [Crossref] [PubMed]
- Olsen AK, Coskun M, Bzorek M, et al. Regulation of APC and AXIN2 expression by intestinal tumor suppressor CDX2 in colon cancer cells. Carcinogenesis 2013;34:1361-9. [Crossref] [PubMed]
- Asgari-Karchekani S, Karimian M, Mazoochi T, et al. CDX2 Protein Expression in Colorectal Cancer and ItsCorrelation with Clinical and Pathological Characteristics, Prognosis, and Survival Rate of Patients. J Gastrointest Cancer 2020;51:844-9. [Crossref] [PubMed]
- Li CM, Gocheva V, Oudin MJ, et al. Foxa2 and Cdx2 cooperate with Nkx2-1 to inhibit lung adenocarcinoma metastasis. Genes Dev 2015;29:1850-62. [Crossref] [PubMed]
- Saad RS, Cho P, Silverman JF, et al. Usefulness of Cdx2 in separating mucinous bronchioloalveolar adenocarcinoma of the lung from metastatic mucinous colorectal adenocarcinoma. Am J Clin Pathol 2004;122:421-7. [Crossref] [PubMed]
- Yatabe Y, Koga T, Mitsudomi T, et al. CK20 expression, CDX2 expression, K-ras mutation, and goblet cell morphology in a subset of lung adenocarcinomas. J Pathol 2004;203:645-52. [Crossref] [PubMed]