A novel anoikis-related gene signature can predict the prognosis of hepatocarcinoma patients
Highlight box
Key findings
• Constructed a novel anoikis-related gene (ANRG) signature to predict the prognosis of hepatocellular carcinoma (HCC) patients.
What is known and what is new?
• Primary liver cancer ranked sixth among all cancers worldwide and stood as the third leading cause of cancer-related deaths. HCC, the most prevalent type, accounted for 90% of all primary liver cancers. Anoikis, a specific form of apoptosis triggered by the loss of appropriate cell-to-cell or cell-to-extracellular matrix adhesion, plays a crucial role. Research indicates that promoting resistance to anoikis is pivotal in the progression of tumor cells, as this resistance is considered an inherent characteristic of aggressive tumor cells. It facilitates the survival of cells detached from the primary tumor, thereby promoting metastasis. Distant metastasis of HCC is often associated with a poor prognosis.
• We constructed a novel ANRG signature to predict the prognosis of HCC patients. Through internal validation, our model demonstrated high predictive capability. Additionally, we not only investigated immune cell infiltration under different risk scores but also explored the expression of ANRGs in tumor microenvironment-associated cells.
What is the implication, and what should change now?
• The nomogram based on our model serves as a reliable predictive indicator for the survival of HCC patients, aiding healthcare professionals in formulating personalized treatment plans in clinical practice. Future investigations into the biological basis of this feature and prospective randomized clinical trials may have substantial clinical implications and potentially lay the foundation for precision medicine.
Introduction
According to the 2020 global cancer statistics, primary liver cancer was the sixth most common cancer worldwide in 2020 and the third leading cause of cancer deaths (1), of all primary liver cancers, hepatocellular carcinoma (HCC) is the most common tumor, accounting for 90% of cases (2), and it is estimated that more than 1 million people will be affected by HCC each year by 2025 (3). Distant metastasis often leads to a poor prognosis of HCC (4,5). Anoikis is caused by cells and extracellular matrix (ECM) and is a specific form of apoptotic process induced by absent or inappropriate attachment to adjacent cells (6). Anoikis is an important mechanism for maintaining the correct location of cells in tissues. Induction of anoikis occurs when cells lose attachment to ECM or adhere to inappropriate types of ECM (7). It has been shown that promoting anoikis resistance has an important role in tumor cell progression (8,9), resistance to anoikis is an inherent property of aggressive tumor cells. Anoikis resistance is believed to contribute to the survival of tumor cells that detach from the primary tumor, thereby facilitating metastasis (10). Without anoikis resistance, cancer cells cannot survive outside their primary site (11,12). Ye et al. discovered that nuclear MYH9 conferred anoikis resistance to gastric cancer cells by identifying the CTNNB1 promoter and, in turn, facilitated the metastasis of gastric cancer (13). Research by D’Amato indicates that TDO2 promotes anoikis resistance, migration, and invasive capabilities in triple-negative breast cancer, leading to a shorter overall survival (OS) (14). According to Wang et al., CPT1A-mediated fatty acid oxidation inhibits anoikis, which in turn encourages colorectal cancer (CRC) cells to metastasize (15). Previous studies have shown that anoikis resistance plays an important role in the progression of HCC (16,17). The main components of the tumor microenvironment (TME) are immune cells and stromal cells, responsible for tumor dissemination, recurrence, metastasis, immune therapy efficacy, and prognosis (18,19). Infiltration of CD4 and CD8 T lymphocytes in tumors exerts anti-tumor effects, and is associated with a favorable prognosis (20). Tumor-associated macrophages (TAMs) exert a tumor-promoting effect by secreting immunosuppressive factors, leading to a poorer prognosis (21). TME characteristics of HCC include abnormal angiogenesis, chronic inflammation, and dysregulated ECM remodelling, collectively leading to an immunosuppressive environment, thereby promoting the proliferation, invasion, and metastasis of HCC (22). Therefore, exploring the prognostic value of ANRGs in HCC, differences in the TME, and establishing a new prognostic model for HCC is of significant importance.
In this study, we primarily investigated the prognostic value of ANRGs in HCC. We established a prognostic scoring model based on ANRGs and further explored the differences in the TME among patients stratified by this risk-scoring system. We present this article in accordance with the TRIPOD reporting checklist (available at https://tcr.amegroups.com/article/view/10.21037/tcr-23-2096/rc).
Methods
Data acquisition and processing
The study was conducted in accordance with the Declaration of Helsinki (as revised in 2013). The gene expression profiling and clinical information of 371 HCC tissues and 50 normal adjacent tissues were obtained from The Cancer Genome Atlas Program (TCGA) database (https://portal.gdc.cancer.gov/). The gene expression profiling and clinical information of 115 HCC tissues and 52 adjacent non-tumor tissues were obtained from The Gene Expression Omnibus (GEO) database [National Center for Biotechnology Information (https://nih.gov)]. From the GeneCard database (23) (https://www.genecards.org/) and the Harmonizome portals (24) (https://maayanlab.cloud/Harmonizome/), 516 anoikis-related genes (ANRGs) in total were obtained. In addition, the “Limma” R package was used to compare the expression of 516 ANRGs in tumor tissues and nearby normal tissues in the TCGA-Liver Hepatocellular Carcinoma (TCGA-LIHC) cohort, and 219 differentially expressed genes (DEGs) were identified. Ninety-nine ANRGs associated with prognosis in HCC patients were obtained using univariate Cox regression analysis. The TCGA-LIHC cohort was combined with the GSE76427 cohort and the batch effect was removed to obtain the new “LIHC-GSE76427” cohort.
HCC subtype analysis
The anoikis scores of each HCC patient in the LIHC-GSE76427 cohort were assessed using the R gene set variation analysis (GSVA) algorithm based on 99 anoikis genes. The cut-off values for the anoikis score were determined using the “survminer” package in R, and HCC patients were divided into the anoikis high score group and the anoikis low score group. Consistency of clustering was verified using the R package “ggplot2” for Uniform Manifold Approximation and Projection (UMAP) and t-Distributed Stochastic Neighbor Embedding (t-SNE).
Function enrichment analysis
Kyoto Encyclopedia of Genes and Genomes (KEGG) pathway analysis of ANRGs was performed using the “GSVA” R package to explore their potential function in HCC progression (25). The “c2.cp.Kegg.symbols.gmt” gene sets were derived from the Molecular Signatures Database (MSigDB) (26).
Construction of HCC prognostic model based on ANRGs
The ANRG expression associated with HCC prognosis in the LIHC-GSE76427 cohort was randomly grouped to obtain experimental and control groups, with 50% of the experimental group and 50% of the control group, the least absolute shrinkage and selection operator (LASSO) regression model was constructed using data from the training group, and the penalty regularization parameter λ was determined by cross-validation, and the Cox model was constructed using the obtained characteristic genes to obtain their corresponding coefficients. The ANRG risk model was constructed as follows: ANRG risk score = Ʃ (Coeii × Expi), Coeii is the corresponding coefficient of the ith gene, and Expi is the expression of the ith gene. The receiver operating characteristic (ROC) curves of the train and test groups were analyzed with the “TimeROC” package in R. The training and test groups were divided into high-risk and low-risk groups based on the median value of the risk score, and the Kaplan-Meier (KM) survival curves of the train and test groups were analyzed with the “SURVIVAL” package in R, to further explore the accuracy of the model for HCC prognosis.
Relationship between risk score and immune cell infiltration
Immune cell infiltration analysis was performed using the “CIBERSORT” package in R (27). Differences in immune cells between risk scores were analyzed using the “LIMMA” package. Spearman correlation analysis was used to assess the correlation between risk score and immune cell infiltration.
Relationship between risk score and clinicopathological features in patients with HCC
Univariate Cox analysis was used to investigate the correlation between OS and clinicopathological characteristics such as age, gender, pathological stage, and risk score. Clinicopathological features were associated with OS in HCC patients, and multivariate Cox regression analysis was used to screen independent prognostic factors for HCC. The nomogram was created using risk scores and clinicopathological characteristics. To ensure their accuracy, calibration charts were internally validated. The Time-dependent Concordance (Time-C) index was used to verify the nomograms’ accuracy in making predictions. Decision curve analysis (DCA) was performed to assess the clinical net benefit (28).
Tumor immune single cell hub database
A comprehensive online database of single-cell RNA sequencing (RNA-seq) focusing on the TME is called the Tumor Immune Single-Cell Hub (TISCH; http://tisch.comp-genomics.org) (29). Using this database, the TME heterogeneity in diverse data sets and cell types was methodically investigated.
Statistical analysis
Data were prepared using Perl programming language (v5.32.1). All analyses in this study were performed using R software (v4.2.1). P<0.05 was considered statistically significant (*, P<0.05; **, P<0.01; ***, P<0.001; ****, P<0.0001).
Results
Identification of ANRGs associated with prognosis
Genecards and Harmonizome portals were used to get a total of 516 genes associated with anoikis. The expression of these ANRGs was compared between tumor and adjacent normal tissues in the TCGA-LIHC cohort, and 219 DEGs were identified based on logFC (fold change) >1 and false discovery rate (FDR) <0.05. The heatmap showed the first 30 DEGs (Figure 1A). Univariate Cox regression analysis showed that 99 genes in 219 ANRGs were associated with survival, and the difference was statistically significant (P<0.05). Twenty-eight genes were screened for P<0.001 and shown in a forest plot (Figure 1B), all of which were associated with poor prognosis. These DEGs may play a role in the development and progression of HCC. The network diagram shows the relationship between the expression levels of these genes (Figure 1C). It can be seen that all of these DEGs are prognosis-related high-risk genes for HCC, and all of them are positively regulated by each other. To learn more about how these ANRGs are altered on the chromosome and the location of each gene on the chromosome, copy number variation (CNV) data were retrieved from the TCGA database. As shown in Figure 1D,1E, PHLDA2, KIF18A, and CTTN have the most “gains” on chromosome 11, whereas SPP1 and MAD2L1 have the biggest “losses” on chromosome 4.
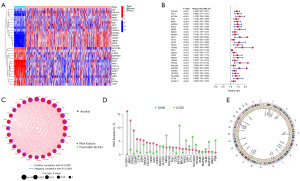
Consistent clustering of HCC molecular subgroups using ANRGs
The “Consensus clustering plus” R program was used to perform consensus clustering utilizing prognosis-associated ANRGs. The figure illustrates that the queue can be effectively separated into two subtypes when K =2 (Figure 2A). UMAP and t-SNE were used to test the accuracy of concordance clustering. The results show that when K =2, two clustering subtypes can be well identified (Figure 2B,2C). A statistically significant difference in prognosis between the two subtypes was found in the OS analysis (P<0.001) (Figure 2D). The GSVA R package was used to test the differential enrichment of KEGG pathways between cluster A and cluster B (Figure 2E). Cluster B had a poor prognosis and is mainly involved in the cell cycle and pathogenic Escherichia coli infection. Aberrancy in cell cycle progression is one of the fundamental mechanisms underlying tumorigenesis (30). HCC is frequently associated with pathogen infection-induced chronic inflammation (31).
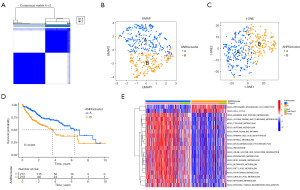
Analysis of gene expression and difference of immune cells between the two subtypes
The boxplot displays the two subgroups’ ANRG expression patterns (Figure 3A). The expression of ANRGs in cluster B was higher than that in cluster A. These genes may be important molecules that affect the prognosis of HCC patients and prospective targets for targeted therapeutics due to the poor overall prognosis of cluster B. In addition, there was also a significant difference in the level of immune cell infiltration between the two clusters (Figure 3B), and the activation ratio of myeloid-derived suppressor cells (MDSC) cells in cluster B was significantly higher than that in cluster A. It has been demonstrated that MDSC are produced as tumors grow and inhibit T and natural killer (NK) cell antitumor activity. In cancer patients, their enrichment is linked to a poor prognosis and a worse response to immunotherapy (32).
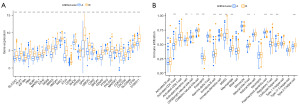
Construction of a prognostic model of ANRGs in HCC
ANRG risk characteristics were analyzed using the univariate LASSO Cox regression model. The “ANRGscore” refers to the ultimate risk score based on the seven characteristic genes (Figure 4A,4B). Prognostic index (PI) = (0.170 × ETV4 expression level) + (0.05 × SPINK1 expression level) + (0.202 × PBK expression level) + (0.167 × SLC2A1 expression level) + (0.191 × FASN expression level) + (0.259 × RAC3 expression level) + (0.220 × MMP3 expression level). Under the model, the ROC curves at 1, 3, and 5 years in both the TRAIN and TEST groups had good predictive performance (Figure 4C,4D). The KM curves in the high-risk group in the TRAIN group showed a poor prognosis, and the validation cohort in the TEST group also showed a poor prognosis (Figure 4E,4F). Risk scores were significantly different between the two subtypes (Figure 4G), showing anoikis-related clusters, ANRGscore, and alluvial layers of changes in survival status (Figure 4H).
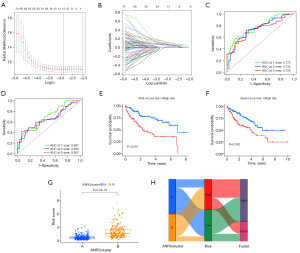
Immune cell infiltration with different risk scores
The relative proportions of infiltrating immune cells were quantified using the CIBERSORT R script. First, using a risk score to rank HCC from low to high, the number of various immune cells was displayed (Figure 5A). As the risk score rose, the percentage of activated T-cell CD4 steadily increased (R=0.44) (Figure 5B). In addition, a higher proportion of activated memory T-cell CD4 cells among immune cells in the high-risk group (Figure 5C), shows that memory T-cell CD4 activation may have a significant role in the patient’s poor prognosis for HCC. Immune cell interactions in HCC patients may offer hints to better comprehend the makeup of the immune microenvironment during particular periods (Figure 5D).
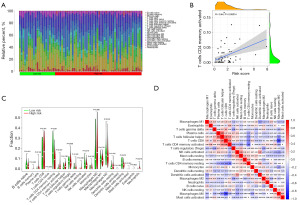
The risk score is an independent prognostic factor in HCC patients
Nomogram was created using the clinical data and the ANRGscore model (Figure 6A). The calibrated plot demonstrates the reliability of the nomogram (Figure 6B). The DCA curve shows that the model has guiding significance for clinical application and may be beneficial to patients with HCC (Figure 6C-6E). Patients with a higher HCC score on the nomogram had a gradually increased OS risk, as indicated by the cumulative risk curve (Figure 6F). The forest plot shows that risk score and stage are the primary influencing elements in the nomogram (Figure 6G). These findings indicate the reliability of the nomogram based on the ANRGs risk score in predicting the clinical prognosis of patients with HCC.
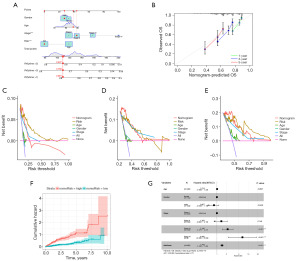
Analysis of the correlation between the tumor immune microenvironment and ANRGs
The expression of seven ANRGs in TME was examined using the single-cell dataset LIHC-GSE166635 from the TISCH database. There are 10 different cell types and 20 different cell populations in the GSE166635 dataset (Figure 7A-7C). BAK1 was mainly expressed in malignant cells, with lower expression in endothelial cells, epithelial cells, fibroblasts, and immune cells (Figure 7D). SPINK1 was highly expressed in all cells, especially in malignant and epithelial cells (Figure 7E), PBK was less expressed in all cells (Figure 7F), SLC2A1 was more expressed in dendritic cells (Figure 7G), and FASN was more expressed in malignant cells (Figure 7H). RAC3 expression was generally low in all cells and relatively high in malignant cells (Figure 7I), and MMP3 was mainly expressed in endothelial cells, epithelial cells, and fibroblasts (Figure 7J).
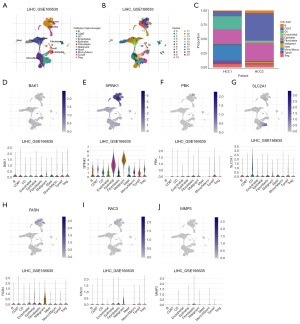
Discussion
HCC is an aggressive disease with a poor clinical prognosis (33). Therefore, the establishment of predictive models may provide an important tool for early intervention. For example, Long et al. developed a four-gene prognostic model that performed well in predicting the prognosis of HCC (34). Tang et al. established a novel tumor immunological phenotype-related gene index (TIPRGPI), consisting of 11 genes, to predict HCC prognosis and response to immunotherapy (35). A recently published work builds anoikis-based signatures by machine learning for HCC prognosis prediction. The article was studied by examining data from TCGA-LIHC, and validated using data from Hepatocellular Carcinoma Expression Database (HCCDB) to obtain risk score signatures for nine genes, with a focus on the role of DAP3 in HCC progression (36). In addition, in recent years, there have been articles studying the apoptotic-related genes ANRGs and their association with HCC prognosis (37-40). In comparison to previous research, our study is based on the merged LIHC-GSE76427 cohort, derived by combining the TCGA and GEO databases after removing batch effects. This approach provides a more comprehensive coverage of information and enhances credibility compared to individual databases. Through internal validation, our model has demonstrated high predictive capability. Moreover, we not only investigated immune cell infiltration under different risk scores but also explored the expression of ANRGs in TME-associated cells.
Anoikis is a form of apoptosis in which when cells are separated from the right ECM, they disrupt the binding of integrins. This is a key mechanism to prevent dysplastic cell growth or attachment to inappropriate substrates (41). Anoikis prevents shed epithelial cells from colonizing elsewhere and is therefore critical for tissue homeostasis and development (42). Normal epithelial cells undergo an apoptotic process known as anoikis as they detach, losing important survival elements (43). To survive before metastasis, tumor cells must develop anoikis resistance, and metastatic tumor cells of HCC acquire resistance to anoikis, enabling them to initiate metastasis (44). The role of promoting anoikis resistance in liver cancer progression has been reported (45,46).
In our study, 219 DEGs were obtained by comparing the expression of ANRGs in the TCGA-LIHC cohort between tumors and adjacent normal tissues, of which 99 ANRGs were associated with the prognosis of HCC, TCGA-LIHC and GSE76427 were combined and randomly divided into training and test groups, and data from the training group were used to construct the LASSO regression model and determine the penalty regularization parameter λ by cross-validation, and the Cox model was constructed using the obtained signature genes to obtain their corresponding coefficients, and risk scores based on the seven signature genes obtained and validated using the test group data, and finally, ETV4, SPINK1, PBK, SLC2A1, FASN, RAC3, and MMP3 were used to construct a prognostic prediction model for HCC. Among the seven prognosis genes signatures, a specific link between these genes and cancer progression has been identified in earlier studies. For example, ETV4 promotes pancreatic ductal adenocarcinoma metastasis by activating the CXCL13/CXCR5 signaling axis (47). SPINK1 is upregulated in HCC and its upregulation is associated with poor prognosis and is an oncogenic factor for HCC progression (48). PBK/TOPK is associated with the development, progression, and metastasis of malignancies (49). SLC2A1 plays a critical role in cancer progression and is primarily associated with functions such as epithelial-mesenchymal transition (EMT), glycolysis, hypoxia, cell cycle regulation, and DNA repair (50). Stabilization of FASN by ACAT1-mediated GNPAT acetylation plays a critical role in hepatocarcinogenesis (51). Rac3 regulates breast cancer invasion and metastasis by controlling adhesion and matrix degradation (52). Matrix metalloproteinases (MMP) contribute to the local and distant progression of HCC by participating in the EMT of HCC (53). By examining immune cell infiltration at different risk scores, we found a higher proportion of activated memory T-cell CD4 among immune cells in the high-risk group, suggesting that activation of memory T-cell CD4 may play an important role in patients’ poor HCC prognosis. We created nomograms using clinical data and the ANRGscore model, and the DCA curves indicated that risk score was an independent prognostic factor for HCC patients, and the ANRGs risk score-based nomograms were reliable in predicting the clinical prognosis of HCC patients, in addition, we further explored the relevance of these seven ANRGs to the tumor immune microenvironment.
The HCC patients were divided into groups based on the high or low expression of genes related to anoikis. Different subgroups significantly differed in the expression and prognosis of ANRGs, and the risk score was an independent factor impacting the prognosis of HCC. It is suggested that our seven signature genes can be used to effectively judge the prognosis of the patients. This aids clinicians in designing various treatment plans. The DCA curves also showed that nomograms based on the seven genetic characteristics could benefit HCC patients within 1, 3 and 5 years.
Although our risk score and the established nomograms based on the risk score it are good predictors of clinical outcomes in patients with HCC, our study has some inadequacies. Firstly, the construction and validation of our prognostic model are based on a retrospective database; therefore, our research findings should be further confirmed through prospective clinical studies. Secondly, the potential mechanisms of these seven ANRGs associated with HCC prognosis require further experimental investigation.
Conclusions
Our study established a novel risk feature based on seven ANRGs, demonstrating significant efficacy in predicting the survival outcomes of HCC patients. Furthermore, our research revealed a close association between the risk score and the immune microenvironment, exploring the expression of ANRGs in TME-associated cells. In summary, the nomogram based on our model serves as a reliable predictive indicator for the survival of HCC patients, aiding healthcare professionals in formulating personalized treatment plans in clinical practice. Future investigations into the biological basis of this feature and prospective randomized clinical trials may have substantial clinical implications and potentially lay the foundation for precision medicine.
Acknowledgments
Funding: None.
Footnote
Reporting Checklist: The authors have completed the TRIPOD reporting checklist. Available at https://tcr.amegroups.com/article/view/10.21037/tcr-23-2096/rc
Peer Review File: Available at https://tcr.amegroups.com/article/view/10.21037/tcr-23-2096/prf
Conflicts of Interest: All authors have completed the ICMJE uniform disclosure form (available at https://tcr.amegroups.com/article/view/10.21037/tcr-23-2096/coif). The authors have no conflicts of interest to declare.
Ethical Statement: The authors are accountable for all aspects of the work in ensuring that questions related to the accuracy or integrity of any part of the work are appropriately investigated and resolved. The study was conducted in accordance with the Declaration of Helsinki (as revised in 2013).
Open Access Statement: This is an Open Access article distributed in accordance with the Creative Commons Attribution-NonCommercial-NoDerivs 4.0 International License (CC BY-NC-ND 4.0), which permits the non-commercial replication and distribution of the article with the strict proviso that no changes or edits are made and the original work is properly cited (including links to both the formal publication through the relevant DOI and the license). See: https://creativecommons.org/licenses/by-nc-nd/4.0/.
References
- Sung H, Ferlay J, Siegel RL, et al. Global Cancer Statistics 2020: GLOBOCAN Estimates of Incidence and Mortality Worldwide for 36 Cancers in 185 Countries. CA Cancer J Clin 2021;71:209-49. [Crossref] [PubMed]
- Forner A, Reig M, Bruix J. Hepatocellular carcinoma. Lancet 2018;391:1301-14. [Crossref] [PubMed]
- Llovet JM, Kelley RK, Villanueva A, et al. Hepatocellular carcinoma. Nat Rev Dis Primers 2021;7:6. [Crossref] [PubMed]
- Liu D, Song T. Changes in and challenges regarding the surgical treatment of hepatocellular carcinoma in China. Biosci Trends 2021;15:142-7. [Crossref] [PubMed]
- Massagué J, Obenauf AC. Metastatic colonization by circulating tumour cells. Nature 2016;529:298-306. [Crossref] [PubMed]
- Taddei ML, Giannoni E, Fiaschi T, et al. Anoikis: an emerging hallmark in health and diseases. J Pathol 2012;226:380-93. [Crossref] [PubMed]
- Gilmore AP. Anoikis. Cell Death Differ 2005;12:1473-7. [Crossref] [PubMed]
- Ayla S, Karahüseyinogluc S. Cancer Stem Cells, Their Microenvironment and Anoikis. Crit Rev Oncog 2019;24:27-34. [Crossref] [PubMed]
- Khan SU, Fatima K, Malik F. Understanding the cell survival mechanism of anoikis-resistant cancer cells during different steps of metastasis. Clin Exp Metastasis 2022;39:715-26. [Crossref] [PubMed]
- Simpson CD, Anyiwe K, Schimmer AD. Anoikis resistance and tumor metastasis. Cancer Lett 2008;272:177-85. [Crossref] [PubMed]
- Paoli P, Giannoni E, Chiarugi P. Anoikis molecular pathways and its role in cancer progression. Biochim Biophys Acta 2013;1833:3481-98. [Crossref] [PubMed]
- Guadamillas MC, Cerezo A, Del Pozo MA. Overcoming anoikis–pathways to anchorage-independent growth in cancer. J Cell Sci 2011;124:3189-97. [Crossref] [PubMed]
- Ye G, Yang Q, Lei X, et al. Nuclear MYH9-induced CTNNB1 transcription, targeted by staurosporin, promotes gastric cancer cell anoikis resistance and metastasis. Theranostics 2020;10:7545-60. [Crossref] [PubMed]
- D’Amato NC, Rogers TJ, Gordon MA, et al. A TDO2-AhR signaling axis facilitates anoikis resistance and metastasis in triple-negative breast cancer. Cancer Res 2015;75:4651-64. [Crossref] [PubMed]
- Wang YN, Zeng ZL, Lu J, et al. CPT1A-mediated fatty acid oxidation promotes colorectal cancer cell metastasis by inhibiting anoikis. Oncogene 2018;37:6025-40. [Crossref] [PubMed]
- Hu X, Zhao Y, Wei L, et al. CCDC178 promotes hepatocellular carcinoma metastasis through modulation of anoikis. Oncogene 2017;36:4047-59. [Crossref] [PubMed]
- Peng YF, Shi YH, Ding ZB, et al. Autophagy inhibition suppresses pulmonary metastasis of HCC in mice via impairing anoikis resistance and colonization of HCC cells. Autophagy 2013;9:2056-68. [Crossref] [PubMed]
- Ge Z, Ding S. The Crosstalk Between Tumor-Associated Macrophages (TAMs) and Tumor Cells and the Corresponding Targeted Therapy. Front Oncol 2020;10:590941. [Crossref] [PubMed]
- Bagaev A, Kotlov N, Nomie K, et al. Conserved pan-cancer microenvironment subtypes predict response to immunotherapy. Cancer Cell 2021;39:845-865.e7. [Crossref] [PubMed]
- Ostroumov D, Fekete-Drimusz N, Saborowski M, et al. CD4 and CD8 T lymphocyte interplay in controlling tumor growth. Cell Mol Life Sci 2018;75:689-713. [Crossref] [PubMed]
- Xiang X, Wang J, Lu D, et al. Targeting tumor-associated macrophages to synergize tumor immunotherapy. Signal Transduct Target Ther 2021;6:75. [Crossref] [PubMed]
- Chen C, Wang Z, Ding Y, et al. Tumor microenvironment-mediated immune evasion in hepatocellular carcinoma. Front Immunol 2023;14:1133308. [Crossref] [PubMed]
- Stelzer G, Rosen N, Plaschkes I, et al. The GeneCards Suite: From Gene Data Mining to Disease Genome Sequence Analyses. Curr Protoc Bioinformatics 2016;54:1.30.1-1.30.33.
- Rouillard AD, Gundersen GW, Fernandez NF, et al. The harmonizome: a collection of processed datasets gathered to serve and mine knowledge about genes and proteins. Database (Oxford) 2016;2016:baw100. [Crossref] [PubMed]
- Hänzelmann S, Castelo R, Guinney J. GSVA: gene set variation analysis for microarray and RNA-seq data. BMC Bioinformatics 2013;14:7. [Crossref] [PubMed]
- Liberzon A, Birger C, Thorvaldsdóttir H, et al. The Molecular Signatures Database (MsigDB) hallmark gene set collection. Cell Syst 2015;1:417-25. [Crossref] [PubMed]
- Newman AM, Liu CL, Green MR, et al. Robust enumeration of cell subsets from tissue expression profiles. Nat Methods 2015;12:453-7. [Crossref] [PubMed]
- Kerr KF, Brown MD, Zhu K, et al. Assessing the Clinical Impact of Risk Prediction Models With Decision Curves: Guidance for Correct Interpretation and Appropriate Use. J Clin Oncol 2016;34:2534-40. [Crossref] [PubMed]
- Sun D, Wang J, Han Y, et al. TISCH: a comprehensive web resource enabling interactive single-cell transcriptome visualization of tumor microenvironment. Nucleic Acids Res 2021;49:D1420-30. [Crossref] [PubMed]
- Liu J, Peng Y, Wei W. Cell cycle on the crossroad of tumorigenesis and cancer therapy. Trends Cell Biol 2022;32:30-44. [Crossref] [PubMed]
- Li W, Xiao J, Zhou X, et al. STK4 regulates TLR pathways and protects against chronic inflammation-related hepatocellular carcinoma. J Clin Invest 2015;125:4239-54. [Crossref] [PubMed]
- Weber R, Groth C, Lasser S, et al. IL-6 as a major regulator of MDSC activity and possible target for cancer immunotherapy. Cell Immunol 2021;359:104254. [Crossref] [PubMed]
- Lee TK, Guan XY, Ma S. Cancer stem cells in hepatocellular carcinoma – from origin to clinical implications. Nat Rev Gastroenterol Hepatol 2022;19:26-44. [Crossref] [PubMed]
- Long J, Wang A, Bai Y, et al. Development and validation of a TP53-associated immune prognostic model for hepatocellular carcinoma. EbioMedicine 2019;42:363-74. [Crossref] [PubMed]
- Tang Y, Guo C, Yang Z, et al. Identification of a Tumor Immunological Phenotype-Related Gene Signature for Predicting Prognosis, Immunotherapy Efficacy, and Drug Candidates in Hepatocellular Carcinoma. Front Immunol 2022;13:862527. [Crossref] [PubMed]
- Guizhen Z, Weiwei Z, Yun W, et al. An anoikis-based signature for predicting prognosis in hepatocellular carcinoma with machine learning. Front Pharmacol 2023;13:1096472. [Crossref] [PubMed]
- Zhong Z, Xie F, Yin J, et al. Development of a prognostic model for anoikis and identifies hub genes in hepatocellular carcinoma. Sci Rep 2023;13:14723. [Crossref] [PubMed]
- Zhang D, Liu S, Wu Q, et al. Prognostic model for hepatocellular carcinoma based on anoikis-related genes: immune landscape analysis and prediction of drug sensitivity. Front Med (Lausanne) 2023;10:1232814. [Crossref] [PubMed]
- Chen Y, Huang W, Ouyang J, et al. Identification of Anoikis-Related Subgroups and Prognosis Model in Liver Hepatocellular Carcinoma. Int J Mol Sci 2023;24:2862. [Crossref] [PubMed]
- Chen Y, Lin QX, Xu YT, et al. An anoikis-related gene signature predicts prognosis and reveals immune infiltration in hepatocellular carcinoma. Front Oncol 2023;13:1158605. [Crossref] [PubMed]
- Li S, Zhang J, Qian S, et al. S100A8 promotes epithelial-mesenchymal transition and metastasis under TGF-β/USF2 axis in colorectal cancer. Cancer Commun (Lond) 2021;41:154-70. [Crossref] [PubMed]
- Bakir B, Chiarella AM, Pitarresi JR, et al. EMT, MET, Plasticity, and Tumor Metastasis. Trends Cell Biol 2020;30:764-76. [Crossref] [PubMed]
- Nirmala JG, Lopus M. Cell death mechanisms in eukaryotes. Cell Biol Toxicol 2020;36:145-64. [Crossref] [PubMed]
- Mo CF, Li J, Yang SX, et al. IQGAP1 promotes anoikis resistance and metastasis through Rac1-dependent ROS accumulation and activation of Src/FAK signalling in hepatocellular carcinoma. Br J Cancer 2020;123:1154-63. [Crossref] [PubMed]
- Song J, Liu Y, Liu F, et al. The 14-3-3σ protein promotes HCC anoikis resistance by inhibiting EGFR degradation and thereby activating the EGFR-dependent ERK1/2 signaling pathway. Theranostics 2021;11:996-1015. [Crossref] [PubMed]
- Guo P, Qiu Y, Ma X, et al. Tripartite motif 31 promotes resistance to anoikis of hepatocarcinoma cells through regulation of p53-AMPK axis. Exp Cell Res 2018;368:59-66. [Crossref] [PubMed]
- Gao X, Jiang M, Chu Y, et al. ETV4 promotes pancreatic ductal adenocarcinoma metastasis through activation of the CXCL13/CXCR5 signaling axis. Cancer Lett 2022;524:42-56. [Crossref] [PubMed]
- Lin S, Lin Y, Wu Z, et al. circRPS16 Promotes Proliferation and Invasion of Hepatocellular Carcinoma by Sponging miR-876-5p to Upregulate SPINK1. Front Oncol 2021;11:724415. [Crossref] [PubMed]
- Han Z, Li L, Huang Y, et al. PBK/TOPK: A Therapeutic Target Worthy of Attention. Cells 2021;10:371. [Crossref] [PubMed]
- Zheng H, Long G, Zheng Y, et al. Glycolysis-Related SLC2A1 Is a Potential Pan-Cancer Biomarker for Prognosis and Immunotherapy. Cancers (Basel) 2022;14:5344. [Crossref] [PubMed]
- Gu L, Zhu Y, Lin X, et al. Stabilization of FASN by ACAT1-mediated GNPAT acetylation promotes lipid metabolism and hepatocarcinogenesis. Oncogene 2020;39:2437-49. [Crossref] [PubMed]
- Donnelly SK, Cabrera R, Mao SPH, et al. Rac3 regulates breast cancer invasion and metastasis by controlling adhesion and matrix degradation. J Cell Biol 2017;216:4331-49. [Crossref] [PubMed]
- Scheau C, Badarau IA, Costache R, et al. The Role of Matrix Metalloproteinases in the Epithelial-Mesenchymal Transition of Hepatocellular Carcinoma. Anal Cell Pathol (Amst) 2019;2019:9423907. [Crossref] [PubMed]