Comprehensive pan-cancer analysis: essential role of ABCB family genes in cancer
Highlight box
Key findings
• Certain oncogenes may elude detection through singular omics analysis methodologies. Nevertheless, through the integration of multiple databases, we have successfully identified several oncogenes within the ABC transporter subfamily B (ABCB) family gene. The upregulation of ABCB family genes serves as a notable indicator of an unfavorable prognosis in various cancers, with particular emphasis on ABCB6 in kidney renal papillary cell carcinoma (KIRP), liver hepatocellular carcinoma (LIHC), and pancreatic adenocarcinoma (PAAD).
What is known and what is new?
• The ATP-binding cassette (ABC) transporter constitutes a widespread superfamily of transporters prominently present on the cellular membrane of organisms. Functioning with the aid of ATP as an energy source, this protein orchestrates the transmembrane transport of diverse substrates. Advancements in understanding have unveiled additional roles beyond mere intracellular or extracellular transport functions for the ABC protein family, encompassing involvement in DNA repair, protein translation, and gene expression regulation. Yet its role in tumors is still unknown.
• Through our research, we found that the level of TAP1, TAP2 and ABCB6 gene expression was significantly correlated with tumor microenvironment, immune subtypes and clinical characteristics in KIRP, LIHC and PAAD.
What is the implication, and what should change now?
• ABCB family genes exhibit elevated expression levels and a notable association with the prognosis across various cancers. The results enhance our comprehension of the critical function that genes of the ABCB family serve in tumor development and provide important perspectives for pinpointing prospective therapeutic targets within cancer research.
Introduction
The global incidence and mortality rates of cancer have experienced a notable escalation (1). Presently, cancer has surpassed infectious diseases, emerging as a preeminent threat to human health, a trend accentuated by advancements in socioeconomic conditions (2). According to World Health Organization (WHO) estimates, cancer ranks among the top two leading causes of death in 112 countries worldwide (1). The underlying causative factors are multifaceted, encompassing rapid population growth, demographic aging, and shifts in lifestyle patterns (3,4). In the pathogenesis of cancer, which involves genetic and environmental determinants, investigations into environmental factors have exhibited considerable advancement, leading to the identification of various carcinogens (5). Nevertheless, a consensus prevails that individual genetic factors play a more pivotal role in the pathogenesis of cancer (6). Indeed, the accrual of genetic alterations in vivo constitutes the foundational basis for the occurrence of cancer (7).
The evolution of cancer treatment spans several centuries, witnessing advancements; however, contemporary first-line modalities still predominantly encompass surgery, radiotherapy, and chemotherapy. In recent years, the rapid strides in molecular biology and immunology have engendered transformative breakthroughs, notably in the realms of targeted therapies and immune checkpoint inhibitors. This progress signifies a paradigm shift in cancer treatment from nonselective agents to precise targeted medicine (8-10). Exemplifying this shift, inhibitors targeting epidermal growth factor receptor mutations, such as afatinib and erlotinib, have demonstrated efficacy in decelerating the progression of non-small cell lung cancer, ovarian epithelial cancer and osteosarcoma (11-14). Currently, a limited subset of targeted therapies tailored for specific tumor types has garnered success. Consequently, the crux of targeted therapy lies in the identification of pertinent target genes, enabling the precise modulation of tumor cells while preserving normal cell integrity (9,15,16).
The ATP-binding cassette (ABC) transporter constitutes a widespread superfamily of transporters prominently present on the cellular membrane of organisms. Functioning with the aid of ATP as an energy source, this protein orchestrates the transmembrane transport of diverse substrates (17). Virtually all molecules endowed with physiological functions serve as transport substrates for ABC proteins (18-20). Traditionally associated with drug resistance in extant literature, ABC transporters have been recognized for their role in mediating the efflux of chemotherapeutic drugs (21,22). Advancements in understanding have unveiled additional roles beyond mere intracellular or extracellular transport functions for the ABC protein family, encompassing involvement in DNA repair, protein translation, and gene expression regulation (23,24). The human genome houses a total of 48 ABC transporters categorized into seven subfamilies denoted as ABCDEFG (17). The structural attributes and specific functionalities of these transporters underscore their relevance to a spectrum of physiological processes, including antigen presentation, lipid transport, nutrient uptake, and stem cell formation (18,20). Consequently, the implication arises that ABC transporters are intricately linked to various human diseases (23).
Of particular interest is the ABC transporter subfamily B (ABCB) family, predominantly implicated in drug resistance, as well as processes involving polypeptide, phospholipid, iron, and cholate transport. This family exhibits heightened expression in tissues such as the liver, small intestine, ovary, and testis, with 11 discernible subtypes, ABCB1–ABCB11. Research has established correlations between certain members of the ABCB family—such as transporter associated with antigen processing 1 (TAP1, also named ABCB2), TAP2 (also named ABCB3), associated with immunodeficiency disease, ABCB4 linked to progressive familial intrahepatic cholestasis and ABCB6 associated with hepatocellular carcinoma (25-27). Notably, these diseases demonstrate close associations with the initiation and progression of various neoplastic conditions within the body. Current investigations further indicate that mutations in ABCB genes are closely tied to colorectal tumors, melanoma, hematological tumors, and similar afflictions (28-30). However, the precise and nuanced role of the ABCB family genes in diverse pathological conditions remains unclear, necessitating further exploration.
Our investigation involved a comprehensive analysis of the prognostic significance of the ABCB family gene across a spectrum of cancers, utilizing multiple databases including The Cancer Genome Atlas (TCGA), Kaplan-Meier Plotter, and Gene Expression Profiling Interactive Analysis (GEPIA). Additionally, we conducted an in-depth assessment of the associations between the expression levels of the ABCB gene family and various aspects of the tumor microenvironment (TME), stemness score, immune subtypes, clinical characteristics, and drug sensitivity. Noteworthy emphasis was placed on specific cancer types, namely, kidney renal papillary cell carcinoma (KIRP), liver hepatocellular carcinoma (LIHC), and pancreatic adenocarcinoma (PAAD). Cancer abbreviations are summarized in Table S1.
Methods
Analysis of differential ABCB family expression genes in pan-cancer
In our investigation, we accessed original data encompassing gene expressions, clinical characteristics, immune subtypes, survival data and stemness scores for 33 types of human cancer from UCSC Xena (https://xena.ucsc.edu/). ID (identification for genes) transitions were facilitated using R-package “BioMart”. Through reading and integrating with R software, the expression levels of ABCB family genes in pan-cancer are extracted (FPKM value). Wilcoxon Test is used to detect expression differences between ABCB family genes in cancers, with significance denoted by “*”, “**” and “***” indicating P values of <0.05, <0.01, and <0.001, respectively. Visualization tools such as “ggpubr”, “pheatmap”, and “corrplot” were utilized to generate box plots, heatmaps, and correlation plots, respectively. Regarding the definition of expression level: the gene expression level (FPKM value) after unified processing and normalization is a continuous variable. It is arranged from low to high, and the median value is used as the cutoff point. Below this value is defined as low expression, otherwise, it is defined as high expression. In the heatmap, we use color to distinguish low and high expression groups, with red representing the high expression group and green representing the low expression group, while the cutoff value is black. At the same time, the depth of the color represents the degree of high or low expression. The correlations within ABCB genes were characterized by Spearman analysis. When the absolute value of the Spearman correlation coefficient between genes exceeds 0.3, the pair of genes is generally considered to be related. The R package “corrplot” completes the visualization of correlation analysis. The correlation coefficient’s value is indicated by the circle’s size, while the colors signify whether the correlation is positive or negative.
Subsequently, based on the extraction of ABCB family gene expression in the previous step, the tissues were divided into tumor groups and normal tissue groups according to their source, and then the gene expression differences and statistical significance were calculated through statistical analysis. Specifically, R-package “limma” with built-in relevant statistical algorithms and functions was employed to execute the differential analysis between tumor and normal tissue.
Correlation between pan-cancer survival and ABCB family gene expression
For the examination of the correlation between pan-cancer survival data and ABCB family gene expression, overall survival (OS) data from TCGA databases were extracted for comprehensive analysis. The Kaplan-Meier method and log-rank test were applied to assess OS. Patients were categorized into low and high expression groups based on the median expression level. The R-packages “survminer” and “survival” to conduct Kaplan-Meier analysis were employed to delineate survival curve which link the different expression groups with clinical outcome. R packages “forest plot” were employed to visualize survival analysis outcome and construct Cox model and forest plots. Additionally, online databases (Kaplan-Meier Plotter, GEPIA) were utilized to further verify the relationship between gene expression and OS across pan-cancers. The analysis covered diverse original data sources, including Gene Expression Omnibus (GEO, https://www.ncbi.nlm.nih.gov/geo/), European Genome-phenome Archive (EGA, https://www.ebi.ac.uk/ega/), and TCGA. The Human Protein Atlas (https://www.proteinatlas.org/) was consulted to validate protein expression status in tumor and paired normal tissues.
Dependence analysis between stemness score, TME and ABCB family gene expression
Furthermore, in the investigation of the dependence between stemness score, TME, and ABCB family gene expression, RNA stemness score (RNAss) is an index calculated based on expression data, and DNA stemness score (DNAss) is an index calculated based on methylation data. The index ranges between 0 and 1. A value close to 1 indicates that the lower the degree of cell differentiation, the stronger the stem cell characteristics. The Spearman’s method was utilized to analyze the correlation of ABCB genes with DNAss/RNAss via the “cor.test” command. R-packages “estimate” and “limma” were employed to calculate stromal and immune scores which could predict the content of stromal and immune cells and represent tumor purity and immune infiltrating characteristics across pan-cancer tissues (based on ABCB family gene expression). Spearman’s method was also employed to assess the connection between stromal/immune scores and ABCB expression level. The assessment of TME and stemness scores involved the use of R-packages “reshape2”, “ggpubr”, “ggplot2”, and “limma”, with “corrplot” utilized for the visualization of the results.
Correlation between drug sensitivity, immune subtype, clinical character and ABCB family gene expression
For our investigation into drug sensitivity, data were acquired from the CellMiner database (31) (https://discover.nci.nih.gov/cellminer/home.do). The CellMiner database, primarily derived from the 60 types of cancer cell lines (also called NCI-60 cell line) cataloged by the National Cancer Institute’s Center for Cancer Research, is the predominant cancer cell sample set for testing anticancer drugs. It facilitates queries for data on 22,379 confirmed genes and 20,503 compounds analyzed within the NCI-60 cell line. These anticancer drugs include multiple drugs approved by the US Food and Drug Administration (FDA), as well as drug molecules in clinical trials. We extracted the expression of ABCB gene in NCI-60 cell line from the database and performed correlation analysis with the sensitivity of anti-cancer drugs. To perform drug sensitivity correlation analysis, we utilized R-packages “impute”, “limma”, and “ggpubr”. These tools facilitated the examination of the relationships between drug sensitivity and ABCB family gene expression. Furthermore, for the correlation analysis between ABCB family gene expression and immune subtypes, as well as clinical characteristics, we employed R-packages “limma” and “reshape2”. Thorsson and colleagues integrated the immune genomic data from over 10,000 TCGA tumor patients to classify six immune subtypes. These are: wound healing (C1), IFN-γ dominant (C2), inflammatory (C3), lymphocyte depleted (C4), immunologically quiet (C5), and TGF-β dominant (C6). Each subtype is distinct, marked by variations in macrophage or lymphocyte signatures, Th1 to Th2 cell ratios, levels of intratumor heterogeneity, aneuploidy, neoantigen load, cellular proliferation rates, immunomodulatory gene expression, and prognostic outcomes (32). These analyses allowed us to explore potential associations between the expression patterns of ABCB family genes and immune subtype classification, as well as various clinical features.
Statistical analysis
Gene expression data from the TCGA, GTEx, and EGA databases were analyzed using Student’s t-test. Wilcoxon test was employed for comparing gene expression differences between normal and tumor tissues and the Kruskal-Wallis test was for comparing expression in pan-cancer. OS was calculated using the Kaplan-Meier method, and survival curves were compared using log-rank tests. Pearson analysis was performed to evaluate the correlation between ABCB expression levels with other factors (DNAss/RNAss, immune/stromal scores). All statistical analysis was conducted using R software (version 3.6.1). A P value <0.05 was considered statistically significant.
Ethical statement
The study was conducted in accordance with the Declaration of Helsinki (as revised in 2013).
Results
The expression levels of ABCB family gene in pan-cancers
The findings of our investigation revealed that the expression levels of TAP1, TAP2, and ABCB7 were notably elevated across pan-cancers, closely followed by ABCB6, ABCB8, and ABCB10. Conversely, the gene expression levels of ABCB5 and ABCB11 were observed to be comparatively diminished (Figure 1A). Consequently, these two genes, namely ABCB5 and ABCB11, were excluded from subsequent analyses. Further examination delineated that the expression levels of TAP1 and TAP2 reached their zenith in cholangiocarcinoma (CHOL), while ABCB7 exhibited its highest expression in glioblastoma multiforme (GBM). In contrast to other family members, the expression level of ABCB1 was observed to be lower, particularly evident in kidney chromophobe (KICH) and kidney renal clear cell carcinoma (KIRC). Overall, the ABCB gene family manifested heightened expression in pan-cancers except ABCB1 (Figure 1B). Furthermore, TAP1 and TAP2 emerged as the genes displaying the highest positive correlation, with a correlation coefficient of 0.85. Upon excluding the genes with low expression abundance, ABCB5 and ABCB11, the most negative correlation was observed between ABCB4 and ABCB9, with a correlation coefficient of −0.18 (Figure 1C).
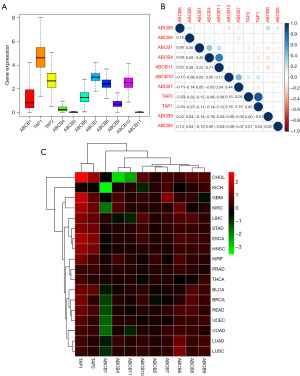
In our effort to assess the variance in the transcriptional levels of family genes, we employed R-packages within the R software framework to analyze RNA sequencing data from the TCGA database. Our investigation unveiled that ABCB1 exhibited lower expression in tumor tissues compared to adjacent tissues across multiple cancer types, including bladder urothelial carcinoma (BLCA), breast invasive carcinoma (BRCA), colon adenocarcinoma (COAD), head and neck squamous cell carcinoma (HNSC), KICH, KIRC, lung adenocarcinoma (LUAD), lung squamous cell carcinoma (LUSC), uterine corpus endometrial carcinoma (UCEC) and rectum adenocarcinoma (READ) (Figure 2A). Simultaneously, our analysis indicated that TAP1 exhibited a state of elevated expression in several cancer types, including esophageal carcinoma (ESCA), prostate adenocarcinoma (PRAD), stomach adenocarcinoma (STAD), thyroid carcinoma (THCA), KIRP and UCEC, BLCA, BRCA, CHOL, COAD, GBM, HNSC, KIRC, LIHC, LUAD and READ (Figure 2B). The examination further revealed that TAP2 demonstrated higher expression levels in KICH, BLCA, CHOL, COAD, ESCA, GBM, HNSC, KIRC, KIRP, LIHC, PRAD, READ, and STAD. However, it exhibited lower expression specifically in KICH (Figure 2C). Our analysis indicated that ABCB4 exhibited elevated expression levels in BRCA, GBM, KIRC, KIRP, LUAD, and LUSC. Conversely, a lower expression of ABCB4 was observed in BLCA, CHOL, COAD, HNSC, KICH, READ, UCEC and LIHC (Figure 2D). The examination revealed that ABCB6 demonstrated higher expression levels in COAD, ESCA, HNSC, KIRC, KIRP, LIHC, LUAD, LUSC, PRAD, READ, STAD, and UCEC. Conversely, it exhibited lower expression in KICH and THCA (Figure 2E). The analysis unveiled that ABCB7 exhibited higher expression levels in CHOL, COAD, GBM, KICH, KIRC, LIHC, LUAD, LUSC, and STAD. Conversely, it demonstrated lower expression in BRCA, KIRP, and UCEC (Figure 2F). Our investigation found that ABCB8 displayed higher expression levels in BLCA, BRCA, CHOL, COAD, ESCA, HNSC, KIRP, LIHC, LUAD, LUSC, PRAD, READ, STAD, and UCEC. Conversely, it demonstrated lower expression in KIRC and THCA (Figure 2G). Our examination revealed that ABCB9 exhibited higher expression levels in BLCA, BRCA, CHOL, ESCA, HNSC, KICH, LIHC, LUAD, LUSC, READ, STAD, THCA, and UCEC. Conversely, it displayed lower expression in GBM, KIRC, and PRAD (Figure 2H). Our analysis demonstrated that ABCB10 exhibited higher expression levels in BRCA, CHOL, ESCA, GBM, KIRC, LIHC, LUAD, LUSC, and STAD. Conversely, it displayed lower expression in KICH, KIRP, THCA, and UCEC (Figure 2I). The details of overexpression and low expression status of ABCB genes in cancers were summarized in Table S2.
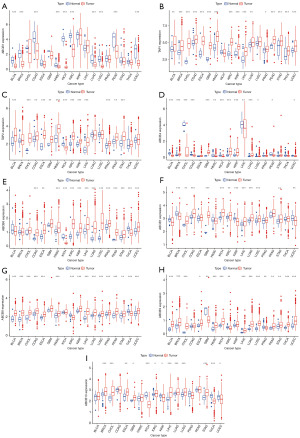
Correlation analysis of the expression levels of ABCB family gene and prognosis in pan-cancers
In the pursuit of verifying the prognostic value of the ABCB gene family, various databases were employed. Kaplan-Meier survival curves, derived from TCGA datasets, illuminated the association between ABCB family genes and the prognosis of diverse cancers. Generally, higher expression levels of ABCB family genes were linked to a poorer prognosis in patients, with the exception of ABCB1, which played a protective role in skin cutaneous melanoma (SKCM), sarcoma (SARC), KIRC, HNSC, COAD, LUAD, PAAD (Figure 3A-3G). Specifically, TAP1 demonstrated a detrimental role in three cancers—uveal melanoma (UVM), lower grade glioma (LGG) and PAAD—while playing a protective role in ovarian serous cystadenocarcinoma (OV) and SKCM (Figure 3H-3L). TAP2 exhibited a detrimental role in four cancers—acute myeloid leukemia (LAML), brain LGG, KIRP, and PAAD—while exerting a protective role in SKCM (Figure 3M-3Q). ABCB4 displayed a detrimental role in adrenocortical carcinoma (ACC), UVM, LGG, READ, and STAD, while concurrently having a protective role in KIRC, PAAD, and SKCM (Figure S1). ABCB6 was detrimental in SKCM, UVM, ACC, KIRP, LIHC, and PAAD, and had a protective role in LGG (Figure 3R-3X). ABCB7 has a protective role in GBM and KIRC (Figure S1). ABCB8 exhibited a detrimental role in GBM, KIRC, LGG, SKCM, THCA, and UVM, with a protective role in lymphoid neoplasm diffuse large B-cell lymphoma (DLBC) (Figure S1). ABCB9 had a detrimental role in thymoma (THYM), KIRC, LGG, THCA and UVM, and a protective role in cervical squamous cell carcinoma and endocervical adenocarcinoma (CESC) (Figure S1). ABCB10 played a protective role in mesothelioma (MESO), ACC, and ESCA, with additional protective roles in HNSC and KIRC (Figure S1).
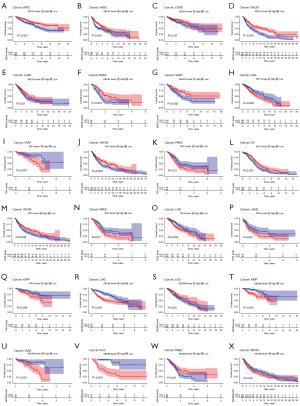
Further insights were gained through Cox analysis (Figure S2, Table S3), GEPIA (Figure S3) and Kaplan-Meier plotter database (Figure S4), culminating in a summarized table (Table 1). In Figure S2, correlation analysis was done between the expression levels of ABCB family gene and prognosis by the Cox method in pan-cancers. Results manifested that ABCB1 takes a protective role in PAAD, HNSC, KIRC, LUAD, SKCM and SARC. TAP1 had a detrimental role in LGG, UVM, PAAD, but took a protective role in SKCM and OV. TAP2 took a detrimental role in LGG, LAML, KIRP, PAAD but had a protective in SKCM. ABCB6 displayed a detrimental role in SKCM, UVM, ACC, KIRP, LIHC and PAAD but owned a protective role in LGG. With regard to GEPIA analysis, ABCB1 manifested a protective role in CESC, KIRC, LUAD and PAAD. Meanwhile, TAP1 exhibited a detrimental role in LGG, OV, SKCM and UVM. TAP2 also played a detrimental role in LAML, LGG, KIRP and PAAD. ABCB6 had a detrimental role in ACC, KIRP, LIHC, SKCM, and UVM (Figure S3). In Kaplan-Meier plotter database, ABCB1 played a detrimental role in LIHC and TAP2 manifested a detrimental role in KIRP and PAAD. Meanwhile, ABCB6 displayed a detrimental role in KIRC, KIRP and LIHC (Figure S4). Notably, three consistent prognostic results were observed across all methods—KIRP, LIHC, and PAAD.
Table 1
Gene | Role | OS | ||||||
---|---|---|---|---|---|---|---|---|
TCGA (Kaplan-Meier) | TCGA (Cox) | Kaplan-Meier plotter | GEPIA | |||||
ABCB1 | Detrimental | – | – | LIHC | – | |||
Protective | PAAD, COAD, HNSC, KIRC, LUAD, SKCM, SARC | SKCM, KIRC, HNSC, PAAD, MESO, LUAD | – | CESC, KIRC, LUAD, PAAD | ||||
TAP1 | Detrimental | LGG, UVM, PAAD | LUAD, UVM, KIRP, PAAD, LGG, THYM | – | LGG, OV, SKCM, UVM | |||
Protective | OV, SKCM | REA, SKCM, STAD, OV | – | – | ||||
TAP2 | Detrimental | LGG, LAML, KIRP, PAAD | LUAD, LAML, UVM, LGG, ACC, KIRP, PAAD | KIRP, PAAD | LAML, LGG, KIRP, PAAD | |||
Protective | SKCM | SKCM | – | – | ||||
ABCB4 | Detrimental | UVM, ACC, LGG, READ, STAD | LGG, UVM, ACC, KICH, THCA, DLBC | KIRP, STAD, THCA | ACC, LGG | |||
Protective | KIRC, PAAD, SKCM | KIRC, PAAD, SKCM, HNSC, SARC | – | HNSC, PAAD | ||||
ABCB6 | Detrimental | SKCM, UVM, ACC, KIRP, LIHC, PAAD | ACC, KIRP, LIHC, UVM, SKCM, KIRC, COAD, KICH | KIRC, KIRP, LIHC | ACC, KIRP, LIHC, SKCM, UVM | |||
Protective | LGG | PAAD, LGG | – | – | ||||
ABCB7 | Detrimental | UCEC | LGG, ESCA, UCEC, SARC, BRCA | BRCA, HNSC, PAAD, SARC, UCEC | – | |||
Protective | GBM, KIRC | KIRC, MESO, GBM, SKCM | – | GBM, KIRC, MESO | ||||
ABCB8 | Detrimental | GBM, KIRC, LGG, SKCM, THCA, UVM | UVM, KIRC, LGG, KICH, GBM, SKCM | KIRC, LIHC, THCA | ACC, KICH, LGG, SKCM, UVM | |||
Protective | DLBC | DLBC, HNSC, KIRP, PAAD | – | DLBC | ||||
ABCB9 | Detrimental | KIRC, LGG, THCA, THYM, UVM | KIRC, LGG, THYM, KIRP, UVM, DLBC, BLCA, KICH | BLCA, BRCA, KIRC, LIHC, SARC, THCA | BLCA, BRCA, DLBC, LGG, THCA, UVM | |||
Protective | CESC | – | – | – | ||||
ABCB10 | Detrimental | MESO, ACC, ESCA | PCPG, ACC, MESO | BLCA, CESC, PCPG | ACC, CESC, LUAD, MESO, PCPG | |||
Protective | HNSC, KIRC | KIRC | – | – |
The abbreviations of the cancer name can be found in Table S1. ABCB, ABC transporter subfamily B; OS, overall survival; TCGA, The Cancer Genome Atlas; GEPIA, Gene Expression Profiling Interactive Analysis.
Correlation analysis of the expression levels of ABCB family gene and TME, stemness scores in pan-cancers
The TME plays a pivotal role in the initiation and progression of tumors, influencing cellular heterogeneity, drug resistance, and subsequent tumor development and metastasis (33). In our quest to understand the correlation between gene expression levels and the TME, we employed the ESTIMATE algorithm to quantify the stromal and immune scores across various cancers. This allowed us to explore tumor purity (represented by stromal score) and the presence of infiltrating immune cells (represented by immune score) in pan-cancer tissues. Our research indicated a notable correlation, either positive or negative, between the expression of ABCB genes and RNAss (Figure 4A) as well as DNAss (Figure 4B) in pan-cancers. Similarly, the expression levels of ABCB family genes exhibited significant positive or negative correlations with stromal scores (Figure 4C) and immune scores (Figure 4D). These results illuminated the intricate relationship between ABCB gene expression and the composition of the TME across a spectrum of cancers.
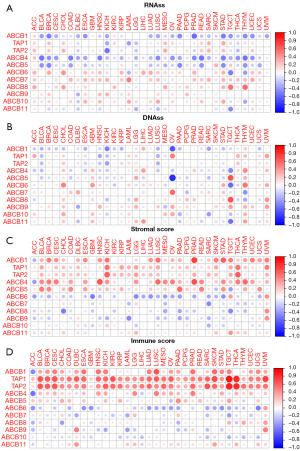
Correlation analysis of the expression levels of ABCB family gene and TME, stemness scores in selected cancers
Our exploration delved deeper into the correlation between gene expression levels within the ABCB gene family and the TME as well as stemness scores in specific cancers—KIRP, LIHC, and PAAD. In the context of KIRP, the results indicated a positive correlation between the expression levels of ABCB6 and ABCB7 with RNAss. Conversely, ABCB1, TAP2, ABCB4, and ABCB9 exhibited a negative correlation with RNAss. Examining the association with DNAss in KIRP, TAP2 and ABCB9 demonstrated a positive correlation, while ABCB1 exhibited a negative correlation. Regarding stromal scores, the expression levels of TAP1 and TAP2 were found to be positively associated. Moreover, TAP1 and TAP2 exhibited a positive correlation with immune scores, while ABCB1, ABCB7, and ABCB10 displayed a negative correlation with immune scores in KIRP. The exploration extended to ESTIMATE scores, revealing a positive correlation between the expression levels of TAP1 and TAP2, and a negative correlation with ABCB1 and ABCB10 in KIRP (Figure 5A).
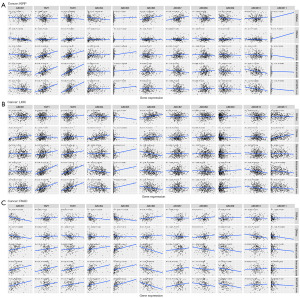
In the context of LIHC, our analysis revealed that the expression level of ABCB6 exhibited a positive correlation with RNAss, while ABCB4 displayed a negative correlation with RNAss. Examining DNAss, the expression levels of ABCB1, ABCB4, ABCB6, and ABCB7 were found to be positively correlated. Moving on to stromal scores, the expression levels of TAP1 and TAP2 were positively related, and ABCB6 showed a negative correlation with stromal scores in LIHC. In terms of immune scores, TAP1 and TAP2 exhibited a positive correlation, while ABCB4, ABCB6, and ABCB10 displayed a negative correlation with immune scores in LIHC. The exploration extended to ESTIMATE scores, revealing a positive correlation between the expression levels of TAP1 and TAP2, and a negative correlation with ABCB4, ABCB6, and ABCB10 in LIHC (Figure 5B).
In the context of PAAD, the analysis indicated that the expression level of ABCB6 exhibited a positive correlation with RNAss, while ABCB1 and ABCB4 displayed a negative correlation with RNAss. Examining DNAss, ABCB1 and ABCB10 were found to have a negative correlation. Moving on to stromal scores, the expression levels of ABCB1 and ABCB4 were positively related, while ABCB6, ABCB8, and ABCB9 showed a negative correlation with stromal scores in PAAD. In terms of immune scores, ABCB1, TAP1, TAP2, ABCB4, and ABCB7 exhibited a positive correlation, while ABCB6, ABCB8, and ABCB9 displayed a negative correlation with immune scores in PAAD. The exploration extended to ESTIMATE scores, revealing a positive correlation between the expression levels of ABCB1, TAP1, TAP2, and ABCB4, and a negative correlation with ABCB6, ABCB8, and ABCB9 in PAAD (Figure 5C). Detailed correlation analyses of ABCB gene expression levels and stromal/immune scores, stemness scores in KIRP, LIHC and PAAD are summarized in Table 2.
Table 2
Cancer | TME | Positive | Negative |
---|---|---|---|
KIRP | RNAss | ABCB6, ABCB7 | ABCB1, TAP2, ABCB4, ABCB9 |
DNAss | TAP2, ABCB9 | ABCB1 | |
Stromal scores | TAP1, TAP2 | – | |
Immune scores | TAP1, TAP2 | ABCB1, ABCB7, ABCB10 | |
ESTIMATE scores | TAP1, TAP2 | ABCB1, ABCB10 | |
LIHC | RNAss | ABCB6 | ABCB4 |
DNAss | ABCB1, ABCB4, ABCB6, ABCB7 | – | |
Stromal scores | TAP1, TAP2 | ABCB6 | |
Immune scores | TAP1, TAP2 | ABCB4, ABCB6, ABCB10 | |
ESTIMATE scores | TAP1, TAP2 | ABCB4, ABCB6, ABCB10 | |
PAAD | RNAss | ABCB6 | ABCB1, ABCB4 |
DNAss | – | ABCB1, ABCB10 | |
Stromal scores | ABCB1, ABCB4 | ABCB6, ABCB8 | |
Immune scores | ABCB1, TAP1, TAP2, ABCB4, ABCB7 | ABCB6, ABCB8, ABCB9 | |
ESTIMATE scores | ABCB1, TAP1, TAP2, ABCB4 | ABCB6, ABCB8, ABCB9 |
The abbreviations of the cancer name can be found in Table S1. ABCB, ABC transporter subfamily B; TME, tumor microenvironment; RNAss, RNA stemness score; DNAss, DNA stemness score.
Correlation analysis of the expression levels of ABCB family gene and immune subtypes
In the analysis of immune subtypes in KIRP, LIHC, and PAAD, six immune subtypes, including C1 (wound healing), C2 (IFN-γ dominant), C3 (inflammatory), C4 (lymphocyte depleted), C5 (immunologically quiet), and C6 (TGF-β dominant), were considered (32). C1 (wound healing) exhibited elevated angiogenic gene expression, high cellular proliferation, and a Th2 cell-biased adaptive immune infiltrate. C2 (IFN-γ Dominant) demonstrated the most pronounced M1/M2 macrophage polarization and CD8 signal, sharing the highest T cell receptor (TCR) diversity with C6. This subtype also presented a high proliferation rate, potentially overriding an emerging Type I immune response. C3 (inflammatory) was characterized by increased Th17 and Th1 gene levels, low to moderate tumor proliferation, and, in conjunction with C5, the least aneuploidy and somatic copy number alterations among the subtypes. C4 (lymphocyte depleted) showed a significant macrophage presence, subdued Th1 activity, and an elevated M2 response. C5 (immunologically quiet) had the minimal lymphocyte and maximal macrophage responses, predominantly M2 macrophages. Lastly, C6 (TGF-β dominant), a diverse group of mixed tumors not specifically categorized in any TCGA subtype, had the strongest TGF-β signature and a balanced distribution of Type I and II T cells (32). This classification serves as the foundation for immunoassays. Our analysis aimed to explore potential crosstalk between ABCB family genes and immune subtypes in these specific cancers. In KIRP, the results revealed that the expressions of TAP1, TAP2, ABCB6, and ABCB7 were associated with immune subtypes (Figure 6A). Specifically, TAP1 and TAP2 were found to be more expressed in C2, while ABCB6 was more expressed in C1 and C2 in KIRP (Figure 6A). Moving on to LIHC, the analysis indicated that the expression of TAP1, TAP2, ABCB6, ABCB4, ABCB1, and ABCB10 was associated with immune subtypes (Figure 6B). TAP1 and TAP2 were more expressed in C2, while ABCB6 was more expressed in C2 and C4 in LIHC (Figure 6B). In PAAD, the results showed that the expression levels of TAP1, TAP2, and ABCB1 were associated with immune subtypes (Figure 6C). Consistent with KIRP and LIHC, TAP1 and TAP2 were found to be more expressed in C2 in PAAD (Figure 6C). Combining the expression levels of ABCB family genes with the results of immune subtypes across pan-cancers, TAP1 and TAP2 were consistently found to be more expressed in C2 (Figure S5). This research illuminates the possible interactions between ABCB family genes and immune subtypes within certain cancer classifications.
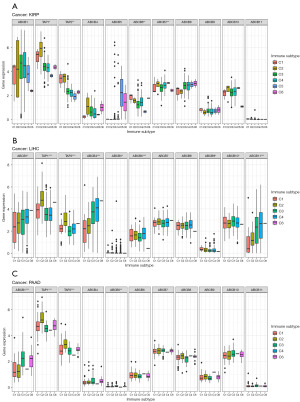
Correlation analysis of the expression levels of ABCB family gene and drug sensitivity in pan-cancers
In our investigation of the relationship between ABCB gene expression levels and the sensitivity of clinical drugs, we utilized the CellMiner database for correlation analysis (Figure 7). The findings revealed that the expression level of ABCB1 gene was negatively correlated with the drug sensitivity of depsipeptide, actinomycin D, mithramycin, carfilzomib, homoharringtonine, doxorubicin, vinorelbine, paclitaxel, vinblastine, and daunorubicin. Similarly, the expression of ABCB4 gene showed a negative correlation with depsipeptide, mithramycin, actinomycin D, and homoharringtonine. The ABCB5 was positively correlated with vemurafenib and dabrafenib.
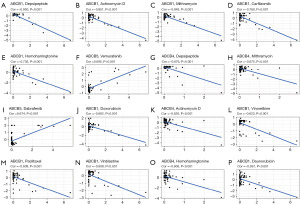
Correlation analysis of the expression levels of ABCB family gene with clinical characteristics in selected cancers and protein expression status
The relationships between ABCB family gene and clinical characteristics are shown in Figure 8. The results showed that the expression levels of ABCB1 and TAP2 genes was positively correlated with patients’ age in LIHC and PAAD, respectively, as well as ABCB10 in KIRP. Moreover, the expression level of ABCB6 gene was positively correlated with clinical stage in KIRP and LIHC. TAP1 gene was found positively correlated with tumor grade in LIHC. TAP1, TAP2 genes were positively correlated with tumor grade in PAAD, however, the expression level of TAP1 gene was negatively correlated with clinical stage in PAAD. Besides, the expression levels of ABCB1, ABCB4, ABCB6 and ABCB7, ABCB8, ABCB9, ABCB10 were found to correlate with gender differences in LIHC and KIRP, respectively. In KIRP, TAP1, TAP2 and ABCB6 manifested lower expression status in normal tissue, at the same time, they showed higher expression status in KIRP tumor tissue (Figure 9A-9F).
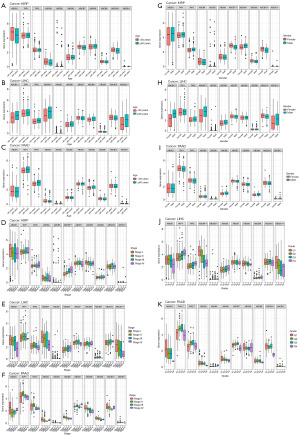
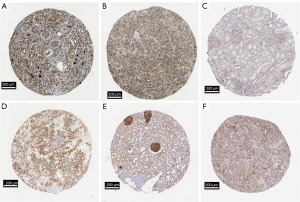
Discussion
Our investigation delved into the expression status of ABCB genes across 10,327 pairs of tumors and their corresponding adjacent samples from 33 types of human primary cancers, utilizing independent datasets from TCGA. Previous research has highlighted the close association of ABCB genes with various human diseases, particularly in the context of cancer occurrence (34-36). In general, tumors with differential expression of ABCB genes exhibit a higher expression level in the majority of tumor types, although there is a smaller percentage in which they show lower expression. This complexity in expression patterns underscores the intricate role of ABCB genes in the landscape of diverse human cancers. To the best of our knowledge, this study represents the inaugural endeavor to substantiate the elevated expression status of the ABCB gene across a spectrum of pan-cancers. Consequently, the research furnishes substantial evidence for prognostic predictions in the realm of pan-cancers by elucidating the expression status of ABCB genes. Moreover, this investigation holds the promise of contributing significantly to the prospective advancement of targeting therapeutic modalities aimed at the ABCB family gene.
Our investigation established a correlation between the expression levels of ABCB genes and the prognosis of KIRP, LIHC, and PAAD. The consistency of prognostic outcomes across diverse databases and statistical algorithms reinforces the robustness of our findings in relation to these three cancers. A positive correlation was observed between increased expression levels of TAP2 and ABCB6 genes and a concomitant decrease in overall survival rates in KIRP, LIHC, and PAAD. This aligns with findings from Zhao et al.’s report, which indicated that the gene expression of ABCB6 is associated with diminished treatment response and unfavorable outcomes in glioma (37). ABCB6 exhibits elevated expression levels in hepatocellular carcinoma, and its expression serves as a predictive indicator for hepatitis C-related hepatocellular carcinoma. Moreover, it has been elucidated that ABCB6 exerts regulatory control over the growth and proliferation of tumor cells through its targeting of the cell cycle (27).
In the course of our investigation, we observed an association between TAP1 and the prognosis of OV, LGG, and other entities. Contrary findings from other studies have indicated that heightened TAP1 expression is correlated with adverse clinical outcomes, encompassing diminished OS, increased likelihood of local recurrence, drug resistance, and treatment failure in conditions such as hepatocellular carcinoma and breast cancer (38,39). Nevertheless, it is noteworthy to acknowledge the existence of inconsistent data regarding the expression of ABCB genes and the survival duration of TCGA cancers across various databases. These disparities may stem from variations in sample composition and algorithmic methodologies employed in distinct databases. Notably, within the entire family of genes studied, the expression levels of TAP1 and TAP2 exhibited the most pronounced correlation. High expression of this TAP complex enhances MHC I assembly, increases drug efflux, and decreases drug sensitivity, ultimately contributing to drug resistance. Simultaneously, it also elevates tumor stemness, impacting patient survival. As per our analytical outcomes, TAP2 manifested a negative correlation with the prognosis of cancers across multiple databases. Research has demonstrated that the overexpression of the TAP2 gene may serve as an indicator of aggressive breast tumors (39). Consequently, the association between the TAP2 gene and the aforementioned three diseases merits further experimental exploration. In summary, our investigations underscore the utility of ABCB family genes as significant biomarkers for prognostic predictions in tumors, with particular emphasis on TAP2 and ABCB6.
Sokolov employed the logistic regression algorithm to conduct an analysis encompassing transcriptome data, methyl groups, and transcription factor binding sites (40). The terms RNAss denote gene expression, while DNAss reflect epigenetic characteristics. Additionally, a heightened stemness index signifies an active state of cancer stem cells and dedifferentiation. Consequently, the stemness score is intricately associated with tumor pathology, clinical prognosis, and the TME. Notably, the stemness score holds promise as a potential target for anticancer therapy. Our research indicates a notable correlation, either positive or negative, between ABCB gene expression and RNAss, DNAss across various cancers, especially noted in KIRP, PAAD, and LIHC. Additionally, ABCB6 gene exhibited a notably positive correlation with DNAss stemness scores in the aforementioned three types of tumors. This observation provides comprehensive evidence supporting the activation of tumor stem cells through the heightened expression of the ABCB6 gene. Furthermore, this aligns with the findings from our prior study, indicating a negative correlation between ABCB6 expression and cancer prognosis. Taken together, these results underscore the characterization of ABCB6 as an oncogene, implicating its role in promoting unfavorable outcomes in cancer.
The study further examined the link between ABCB family genes and the TME. The ESTIMATE algorithm, leveraging single-sample gene set enrichment analysis, was employed to quantify infiltrating stromal and immune cells and estimate tumor purity (41). Elevated scores on these parameters signify increased presence of stromal and immune cells, coupled with reduced tumor purity. Understanding potential therapeutic targets holds the promise of steering the TME towards a tumor-suppressive state (42).
The influence of the immune microenvironment on treatment outcomes and patient survival is readily apparent (43). This microenvironment encompasses infiltrating immune cells, diverse immune regulatory factors, and stromal cells surrounding tumor sites. Theoretically, tumor cells will create an immunosuppressive microenvironment and inhibit immune cells from playing the role of immune surveillance and immune clearance, thereby conducive to tumor proliferation and metastasis. But at the same time, the activation of immune microenvironment can also enhance the function of immune cells to kill tumor cells, including stimulating and upregulating immune presentation functions by immune regulatory factors, increasing the differentiation of cytotoxic T cells, etc. (44). Under such an immune background, drug treatments that modulate immune function are poised to amplify the tumor-killing effect. An immune-promoting microenvironment correlates with improved response to drug therapy and a more favorable prognosis. In conjunction with the findings in our article, the ABCB gene—encoding a transport protein on the cell membrane—merits attention. As a highly conserved housekeeping gene, ABCB governs material transport functions critical to the immune microenvironment. Our analysis reveals a close association between ABCB gene expression, DNA/RNA stemness, tumor purity, and immune profiling. Thus, ABCB gene expression significantly influences the immune context, treatment response, and overall prognosis.
Notably, our findings indicated a significant correlation between TME and gene expression in the aforementioned three tumors. Specifically, the results of this study revealed a negative correlation between TME-related scores and the expression of ABCB6 in LIHC and PAAD. This implies that heightened expression of the ABCB6 gene is associated with increased tumor purity in cancers, providing further substantiation for our assertion that ABCB6 functions as an oncogene.
Delving further into the analysis, we investigated the association between ABCB family genes and immune subtypes in KIRP, PAAD, and LIHC. The findings revealed that, whether across pan-cancers or within the specified three tumors, the genes TAP1 and TAP2 exhibited higher expression in the C2 immune subtype. The C2 immune subtype is characterized by IFN-aggregation, marked by increased infiltration of CD8+ lymphocytes and a higher polarization ratio of M1 macrophages, indicating heightened immune activity (32). These results imply the potential development of targeted drugs based on TAP1 and TAP2, which could effectively operate through the C2 immune subtype.
Additionally, we delved into the potential associations between the gene expressions of the ABCB family and drug sensitivity across various tumor cell lines utilizing data from the CellMiner database. The underlying methodology of the CellMiner database involves subjecting 60 cancer cell lines (NCI-60 cell line set) to treatment with 20,503 analyzed compounds, followed by the assessment of genetic changes in more than 20,000 genes within these cell lines. Given the diverse array of compounds within this database, it is noteworthy that the identified drugs associated with sensitivity to the ABCB gene may not necessarily align with those commonly employed in clinical settings. For a better clinical practice, we screened the drugs in CellMiner, leaving only those drugs that have been approved by the FDA or have entered the clinical trial stage. Our investigation revealed that the expressions of ABCB1 and ABCB4 hold particular significance in guiding clinical drug usage. The results demonstrated that ABCB1 contributed to increased drug resistance against paclitaxel, vinblastine, and daunorubicin. This observation aligns with the well-established role of ABCB1 as a drug resistance gene mediating drug efflux. However, our results presented an intriguing divergence, indicating a positive correlation between the expression level of ABCB1 gene and the prognosis of numerous cancers. In other words, higher expression levels of ABCB1 gene were associated with better patient prognosis. This incongruity might be attributed to the fact that the drug sensitivity database utilized in our study was derived from cell lines, while the survival data was sourced from clinical patients. Further exploration is warranted to elucidate the specific function of the ABCB1 gene in this context.
Examining clinical characteristics, our analysis revealed a notable positive correlation between ABCB6 gene expression and clinical stage in KIRP and LIHC, this finding aligns with our earlier prognostic and expression analysis, as a more aggressive clinical stage typically leads to a poorer prognosis. Indeed, corroborating studies have demonstrated that the upregulation of ABCB6 gene expression is linked to the progression of prostate cancer and melanoma (45,46). This observed phenomenon may be attributed to the high expression of ABCB6, which potentially contributes to heme biosynthesis in tumors (47,48). In both LIHC and PAAD, a positive correlation was identified between the expression levels of TAP1 and TAP2 genes and tumor grade. This observation might be linked to the drug resistance function of the TAP1 gene, particularly evident in hepatocellular carcinoma (35). In KIRP, the expression statuses of TAP1, TAP2, and ABCB6 proteins were concordant, with higher protein expression observed in tumor tissue. These findings align with our gene expression results in Figure 2B,2C,2E and our overall survival analysis results in Figure 3Q,3T.
This research supplied comprehensive insights into the expression status of ABCB family genes in pan-cancers and elucidated the correlation between gene expression and prognosis, along with subsequent TME and immune analyses, making it possess certain clinical significance. There are inherent limitations that cannot be overlooked. Firstly, our data were obtained from public databases, lacking further in vivo or in vitro experiments, which we intend to conduct in the future. Additionally, the molecular mechanisms and downstream pathways of the identified oncogenes—TAP1, TAP2, and ABCB6—remain unclear and warrant exploration through subsequent experiments.
Conclusions
ABCB family genes exhibit elevated expression levels and exhibit a notable association with the prognosis across various cancers. Specifically, the expression levels of TAP1, TAP2, and ABCB6 genes demonstrated significant correlations with the TME, immune subtypes, and clinical characteristics in KIRP, LIHC, and PAAD, with a heightened emphasis on the intricate dynamics observed in KIRP. The results enhance our comprehension of the critical function that ABCB family genes serve in tumor development and provide important perspectives for pinpointing prospective therapeutic targets in oncology studies.
Acknowledgments
We appreciate the databases of UCSC Xena, TCGA, Kaplan-Meier Plotter, GEPIA, and CellMiner for the availability of the data.
Funding: This article was funded by
Footnote
Peer Review File: Available at https://tcr.amegroups.com/article/view/10.21037/tcr-23-2050/prf
Conflicts of Interest: All authors have completed the ICMJE uniform disclosure form (available at https://tcr.amegroups.com/article/view/10.21037/tcr-23-2050/coif). The authors have no conflicts of interest to declare.
Ethical Statement: The authors are accountable for all aspects of the work in ensuring that questions related to the accuracy or integrity of any part of the work are appropriately investigated and resolved. The study was conducted in accordance with the Declaration of Helsinki (as revised in 2013).
Open Access Statement: This is an Open Access article distributed in accordance with the Creative Commons Attribution-NonCommercial-NoDerivs 4.0 International License (CC BY-NC-ND 4.0), which permits the non-commercial replication and distribution of the article with the strict proviso that no changes or edits are made and the original work is properly cited (including links to both the formal publication through the relevant DOI and the license). See: https://creativecommons.org/licenses/by-nc-nd/4.0/.
References
- Sung H, Ferlay J, Siegel RL, et al. Global Cancer Statistics 2020: GLOBOCAN Estimates of Incidence and Mortality Worldwide for 36 Cancers in 185 Countries. CA Cancer J Clin 2021;71:209-49. [Crossref] [PubMed]
- Lortet-Tieulent J, Georges D, Bray F, et al. Profiling global cancer incidence and mortality by socioeconomic development. Int J Cancer 2020;147:3029-36. [Crossref] [PubMed]
- Grosso G, Bella F, Godos J, et al. Possible role of diet in cancer: systematic review and multiple meta-analyses of dietary patterns, lifestyle factors, and cancer risk. Nutr Rev 2017;75:405-19. [Crossref] [PubMed]
- Bray F, Ferlay J, Soerjomataram I, et al. Global cancer statistics 2018: GLOBOCAN estimates of incidence and mortality worldwide for 36 cancers in 185 countries. CA Cancer J Clin 2018;68:394-424. [Crossref] [PubMed]
- Danaei G, Vander Hoorn S, Lopez AD, et al. Causes of cancer in the world: comparative risk assessment of nine behavioural and environmental risk factors. Lancet 2005;366:1784-93. [Crossref] [PubMed]
- Stratton MR, Campbell PJ, Futreal PA. The cancer genome. Nature 2009;458:719-24. [Crossref] [PubMed]
- Golemis EA, Scheet P, Beck TN, et al. Molecular mechanisms of the preventable causes of cancer in the United States. Genes Dev 2018;32:868-902. [Crossref] [PubMed]
- Topalian SL, Taube JM, Anders RA, et al. Mechanism-driven biomarkers to guide immune checkpoint blockade in cancer therapy. Nat Rev Cancer 2016;16:275-87. [Crossref] [PubMed]
- Zou J, Wang E. Cancer Biomarker Discovery for Precision Medicine: New Progress. Curr Med Chem 2019;26:7655-71. [Crossref] [PubMed]
- Jackson SE, Chester JD. Personalised cancer medicine. Int J Cancer 2015;137:262-6. [Crossref] [PubMed]
- Xiong L, Li R, Sun J, et al. Erlotinib as Neoadjuvant Therapy in Stage IIIA (N2) EGFR Mutation-Positive Non-Small Cell Lung Cancer: A Prospective, Single-Arm, Phase II Study. Oncologist 2019;24:157-e64. [Crossref] [PubMed]
- Tagliamento M, Genova C, Rijavec E, et al. Afatinib and Erlotinib in the treatment of squamous-cell lung cancer. Expert Opin Pharmacother 2018;19:2055-62. [Crossref] [PubMed]
- Poursheikhani A, Yousefi H, Tavakoli-Bazzaz J, et al. EGFR Blockade Reverses Cisplatin Resistance in Human Epithelial Ovarian Cancer Cells. Iran Biomed J 2020;24:370-8. [Crossref] [PubMed]
- Cruz-Ramos M, Zamudio-Cuevas Y, Medina-Luna D, et al. Afatinib is active in osteosarcoma in osteosarcoma cell lines. J Cancer Res Clin Oncol 2020;146:1693-700. [Crossref] [PubMed]
- Mukherjee S. Genomics-Guided Immunotherapy for Precision Medicine in Cancer. Cancer Biother Radiopharm 2019;34:487-97. [Crossref] [PubMed]
- Tsimberidou AM, Fountzilas E, Nikanjam M, et al. Review of precision cancer medicine: Evolution of the treatment paradigm. Cancer Treat Rev 2020;86:102019. [Crossref] [PubMed]
- Dean M, Rzhetsky A, Allikmets R. The human ATP-binding cassette (ABC) transporter superfamily. Genome Res 2001;11:1156-66. [Crossref] [PubMed]
- Liu X. ABC Family Transporters. Adv Exp Med Biol 2019;1141:13-100. [Crossref] [PubMed]
- Kos V, Ford RC. The ATP-binding cassette family: a structural perspective. Cell Mol Life Sci 2009;66:3111-26. [Crossref] [PubMed]
- Locher KP. Mechanistic diversity in ATP-binding cassette (ABC) transporters. Nat Struct Mol Biol 2016;23:487-93. [Crossref] [PubMed]
- Zhou HH, Chen X, Cai LY, et al. Erastin Reverses ABCB1-Mediated Docetaxel Resistance in Ovarian Cancer. Front Oncol 2019;9:1398. [Crossref] [PubMed]
- Luo X, Teng QX, Dong JY, et al. Antimicrobial Peptide Reverses ABCB1-Mediated Chemotherapeutic Drug Resistance. Front Pharmacol 2020;11:1208. [Crossref] [PubMed]
- Nobili S, Lapucci A, Landini I, et al. Role of ATP-binding cassette transporters in cancer initiation and progression. Semin Cancer Biol 2020;60:72-95. [Crossref] [PubMed]
- Srikant S. Evolutionary history of ATP-binding cassette proteins. FEBS Lett 2020;594:3882-97. [Crossref] [PubMed]
- Praest P, Luteijn RD, Brak-Boer IGJ, et al. The influence of TAP1 and TAP2 gene polymorphisms on TAP function and its inhibition by viral immune evasion proteins. Mol Immunol 2018;101:55-64. [Crossref] [PubMed]
- Bull LN, Thompson RJ. Progressive Familial Intrahepatic Cholestasis. Clin Liver Dis 2018;22:657-69. [Crossref] [PubMed]
- Tsunedomi R, Iizuka N, Yoshimura K, et al. ABCB6 mRNA and DNA methylation levels serve as useful biomarkers for prediction of early intrahepatic recurrence of hepatitis C virus-related hepatocellular carcinoma. Int J Oncol 2013;42:1551-9. [Crossref] [PubMed]
- Varma A, Mathaiyan J, Shewade D, et al. Influence of ABCB-1, ERCC-1 and ERCC-2 gene polymorphisms on response to capecitabine and oxaliplatin (CAPOX) treatment in colorectal cancer (CRC) patients of South India. J Clin Pharm Ther 2020;45:617-27. [Crossref] [PubMed]
- Damiani D, Tiribelli M, Calistri E, et al. The prognostic value of P-glycoprotein (ABCB) and breast cancer resistance protein (ABCG2) in adults with de novo acute myeloid leukemia with normal karyotype. Haematologica 2006;91:825-8. [PubMed]
- Chen KG, Szakács G, Annereau JP, et al. Principal expression of two mRNA isoforms (ABCB 5alpha and ABCB 5beta) of the ATP-binding cassette transporter gene ABCB 5 in melanoma cells and melanocytes. Pigment Cell Res 2005;18:102-12. [Crossref] [PubMed]
- Reinhold WC, Sunshine M, Liu H, et al. CellMiner: a web-based suite of genomic and pharmacologic tools to explore transcript and drug patterns in the NCI-60 cell line set. Cancer Res 2012;72:3499-511. [Crossref] [PubMed]
- Thorsson V, Gibbs DL, Brown SD, et al. The Immune Landscape of Cancer. Immunity 2018;48:812-830.e14. [Crossref] [PubMed]
- Hinshaw DC, Shevde LA. The Tumor Microenvironment Innately Modulates Cancer Progression. Cancer Res 2019;79:4557-66. [Crossref] [PubMed]
- Feng W, Zhang M, Wu ZX, et al. Erdafitinib Antagonizes ABCB1-Mediated Multidrug Resistance in Cancer Cells. Front Oncol 2020;10:955. [Crossref] [PubMed]
- Zhou XT, Ding J, Li HY, et al. Hedgehog signalling mediates drug resistance through targeting TAP1 in hepatocellular carcinoma. J Cell Mol Med 2020;24:4298-311. [Crossref] [PubMed]
- Nayagam JS, Williamson C, Joshi D, et al. Review article: liver disease in adults with variants in the cholestasis-related genes ABCB11, ABCB4 and ATP8B1. Aliment Pharmacol Ther 2020;52:1628-39. [Crossref] [PubMed]
- Zhao SG, Chen XF, Wang LG, et al. Increased expression of ABCB6 enhances protoporphyrin IX accumulation and photodynamic effect in human glioma. Ann Surg Oncol 2013;20:4379-88. [Crossref] [PubMed]
- Zhang J, Zhang X, Li J, et al. Systematic analysis of the ABC transporter family in hepatocellular carcinoma reveals the importance of ABCB6 in regulating ferroptosis. Life Sci 2020;257:118131. [Crossref] [PubMed]
- Henle AM, Nassar A, Puglisi-Knutson D, et al. Downregulation of TAP1 and TAP2 in early stage breast cancer. PLoS One 2017;12:e0187323. [Crossref] [PubMed]
- Sokolov A, Paull EO, Stuart JM. ONE-CLASS DETECTION OF CELL STATES IN TUMOR SUBTYPES. Pac Symp Biocomput 2016;21:405-16. [Crossref] [PubMed]
- Yoshihara K, Shahmoradgoli M, Martínez E, et al. Inferring tumour purity and stromal and immune cell admixture from expression data. Nat Commun 2013;4:2612. [Crossref] [PubMed]
- Bader JE, Voss K, Rathmell JC. Targeting Metabolism to Improve the Tumor Microenvironment for Cancer Immunotherapy. Mol Cell 2020;78:1019-33. [Crossref] [PubMed]
- Tian H, Cao J, Li B, et al. Managing the immune microenvironment of osteosarcoma: the outlook for osteosarcoma treatment. Bone Res 2023;11:11. [Crossref] [PubMed]
- Wu B, Shi X, Jiang M, et al. Cross-talk between cancer stem cells and immune cells: potential therapeutic targets in the tumor immune microenvironment. Mol Cancer 2023;22:38. [Crossref] [PubMed]
- Karatas OF, Guzel E, Duz MB, et al. The role of ATP-binding cassette transporter genes in the progression of prostate cancer. Prostate 2016;76:434-44. [Crossref] [PubMed]
- Heimerl S, Bosserhoff AK, Langmann T, et al. Mapping ATP-binding cassette transporter gene expression profiles in melanocytes and melanoma cells. Melanoma Res 2007;17:265-73. [Crossref] [PubMed]
- Krishnamurthy PC, Du G, Fukuda Y, et al. Identification of a mammalian mitochondrial porphyrin transporter. Nature 2006;443:586-9. [Crossref] [PubMed]
- Lynch J, Fukuda Y, Krishnamurthy P, et al. Cell survival under stress is enhanced by a mitochondrial ATP-binding cassette transporter that regulates hemoproteins. Cancer Res 2009;69:5560-7. [Crossref] [PubMed]