DNMT3A promotes glioma growth and malignancy via TNF-α/NF-κB signaling pathway
Highlight box
Key findings
• DNMT3A regulates glioma progression through the tumor necrosis factor-α (TNF-α)/nuclear factor-kappa B (NF-κB) signaling pathway and serves as a prognostic factor for predicting glioma prognosis.
What is known and what is new?
• DNMT3A affect the progression of tumor, but the specific regulatory mechanism is still unknown.
• DNMT3A can serve as a prognostic molecule for gliomas and has shown a correlation with immunity. And DNMT3A affect tumor progression through TNF-α/NF-κB signaling pathway.
What is the implication, and what should change now?
• We provide new therapeutic targets for glioma treatment and have the potential to be applied in immunotherapy.
IntroductionOther Section
Glioma is the most frequent intracranial malignant tumor in adults. The prognosis of patients with glioma is poor because of high rates of invasiveness (1). Despite decades of in-depth research into the mechanisms underlying glioma development and progression, effective treatment strategies are still not available for patients diagnosed with glioma (2). The currently available treatment strategies for patients with glioma include surgical resection, radiotherapy, and chemotherapeutics such as temozolomide (TMZ) and other drugs (3,4). In recent years, the field of cancer gene therapy has significantly advanced and improved the survival rates in several cancer types (5). However, the blood-brain barrier (BBB) hinders the efficacies of cancer therapeutics, including gene therapies in glioma patients (6,7). Therefore, in-depth research is required to discover novel avenues of gene therapy for glioma patients to identify effective personalized treatment strategies to improve the survival rates of patients with glioma.
Gliomas are categorized into grades 1–4 according to the World Health Organization (WHO) classification of tumors of the central nervous system (CNS) (8,9). The grade 2 and 3 gliomas in The Cancer Genome Atlas (TCGA) database are classified as lower-grade glioma (LGG) based on the similarity in the nature of molecular mutations and prognosis (10-12). Patients with LGG show better response to various treatment schemes. Therefore, this study mainly focuses on multiple cohorts of patients with LGG. DNMT3A is a key regulator of intracellular DNA methylation. Therefore, regulation of DNMT3A gene expression and protein stability can be used to alter gene expression levels by modulating DNA methylation levels.
DNA methylation is a common epigenetic modification that regulates gene expression and plays a key role in inflammation, innate immunity, and immunotherapy (13-15). DNMT3A is a DNA methyltransferase that participates in the de novo methylation of the whole genome (16-18). DNMT3A is required for the establishment of DNA methylation patterns in the human genome during development (19). Aberrant DNA methylation is associated with a variety of diseases, including cancer (20,21), however, by which DNA methylation regulates cancer cell development and progression is not clear and is a hotspot of basic and clinical research. Our results show that DNMT3A can regulate the progression of LGG through the tumor necrosis factor-α (TNF-α)/nuclear factor-kappa B (NF-κB) signaling pathway, which contributes to reveal the underlying mechanism of DNMT3A regulating glioma progression.
This study aims to develop novel tumor prognostic markers and treatment options (22) for LGG patients by performing bioinformatics analyses to determine the association between the expression level of DNMT3A, overall survival (OS) rates, and tumor immune characteristics of LGG patients from the TCGA, Chinese Glioma Genome Atlas (CGGA), and Gene Expression Omnibus (GEO) databases. Furthermore, we performed in vitro experiments with DNMT3A-silenced LGG cell lines to determine the mechanisms by which DNMT3A regulated glioma growth and progression. We present this article in accordance with the TRIPOD and MDAR reporting checklists (available at https://tcr.amegroups.com/article/view/10.21037/tcr-23-1943/rc).
MethodsOther Section
A detailed flow chart of the basic ideas of this study is shown in Figure 1.
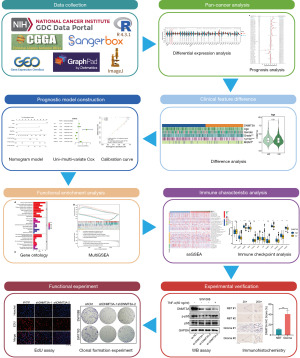
Acquisition and preprocessing of LGG data from the public databases
We downloaded the whole genome messenger RNA (mRNA) sequencing data of LGG samples from the TCGA (https://portal.gdc.cancer.gov/), CGGA (http://www.cgga.org.cn/) and GEO (https://www.ncbi.nlm.nih.gov/gds/) databases. The whole-gene mRNA sequencing data of normal tissues were obtained from the Genotype-Tissue Expression (GTEx) (https://www.gtexportal.org/) database. The mRNA sequencing data was pre-processed by transcript per million (TPM) normalization (23). We also downloaded data from three independent cohorts of LGG samples (GSE16011, GSE61374, and Rembrandt cohort) from the GEO database.
Inclusion criteria for samples in the database
The inclusion criteria were as follows: (I) only samples of patients with LGG were included in the study; (II) there were no deletion values of individual gene expression in patient samples; (III) LGG patients were followed up for ≥30 days; (IV) the clinical information was available for all the patient samples, including survival status, survival time, age, gender, grade; and (V) the patient samples did not have deletions or gene mutations, including isocitrate dehydrogenase (IDH) 1p19q co-deletion, and O6-methyguanine-DNA methytransferase (MGMT) promoter methylation. Finally, after screening, 460, 413, and 379 LGG patients from the TCGA, CGGA, and GEO databases, respectively. The RNA-sequencing data and clinical data of the included patients were downloaded from the corresponding database and match with the vlookup function. The data from two immunotherapy cohorts, namely, IMvigor210 (n=398) (24) and Gide2019 (n=41) (25) was also extracted from the GEO database to analyze whether DNMT3A was a reliable biomarker to identify patients that would respond to immunotherapy.
Analyses of the prognostic potential of DNMT3A in LGG
The optimum cutoff values for the DNMT3A expression levels in three independent LGG databases were calculated using the “survival” and “survminer” R packages. The samples were then classified into high and low DNMT3A groups based on the cutoff values. Subsequently, survival curves were plotted to compare the survival rates of LGG patients with high and low DNMT3A expression levels in the three databases. Univariate and multivariate Cox regression analyses were performed to determine whether DNMT3A expression was an independent prognostic predictor of LGG based on the clinical characteristics. Finally, receiver operating characteristic (ROC) curves were used to determine the prognostic prediction accuracy of DNMT3A expression by calculating the area under the curve (AUC) values.
Functional enrichment analysis
The expression levels of DNMT3A-related genes were analyzed for the LGG samples from the TCGA database according to the DNMT3A expression levels and the differentially expressed genes (DEGs) were identified using the “limma” package. The DEGs were classified into up-regulated and down-regulated genes using the R package. Gene Ontology (GO) and Kyoto Encyclopedia of Genes and Genomes (KEGG) pathway enrichment analysis of the DEGs were performed using the “clusterProfile” R package to determine the cellular functions and pathways that are associated with the DNMT3A-related DEGs (26,27). Finally, multiple gene set enrichment analysis (multiGSEA) were performed to analyze the pathways related with the DEGs and determine the functional characteristics of LGG tumors with high and low DNMT3A expression levels.
Construction of the prognosis prediction nomogram
A nomogram model was constructed using clinical parameters for the LGG patients from the TCGA database. The nomogram included characteristics such as age, gender, WHO grade, IDH 1p19q co-deletion status, and MGMT methylation status. The graphs for the nomogram were drawn using the “rms” R package. The “Calibrate” function was used to construct calibration curves for the TCGA-LGG dataset. The same operations were also performed for the LGG datasets from the CGGA and GEO databases. The clinical utility of the nomogram was analyzed by drawing decision curves using the “stdca” algorithm.
Immune characteristics of LGG patients with high and low DNMT3A expression
Based on the mRNA-seq data of the LGG samples from TCGA database, we analyzed the stromal cell abundance (stromal score), immune cell abundance (immune score), and tumor purity (ESTIMATE score) using the “ESTIMATE” algorithm for different LGG patient groups. The expression levels of 25 immune checkpoint genes and DNMT3A.
Acquisition of clinical LGG samples
We obtained nine frozen clinical samples, including three normal brain tissues (NBTs) and six glioma tissues from the Department of Pathology of the 2nd Affiliated Hospital of Nanchang University. These tissues are stored in liquid nitrogen (−196 ℃) immediately after surgical excision. The pathological features were verified by two pathologists with more than 10 years of experience at the pathology department. This study was approved by the Ethics Committee of the 2nd Affiliated Hospital of Nanchang University. The collection and processing of clinical samples was performed in accordance with the Declaration of Helsinki (as revised in 2013). We obtained informed consent from all the patients included in this study.
Cell culture and lentivirus transfection
We purchased SVG cell line from the Culture Collection of the Chinese Academy of Sciences (Shanghai, China); BT142, SW1088 and SW1783 cell lines from American Type Culture Collection (ATCC; Manassas, VA, USA). SW1088 and SW1783 cells were grown in L-15 medium with 20% O2 as described previously (28), BT142 cells were cultured in F-12 medium and SVG cells was cultured in DMEM medium in an incubator maintained at 37 ℃ and 5% CO2. We purchased lentiviruses cloned with two DNMT3A-specific short hairpin RNAs (shRNAs) from OBIO Bioscience (Shanghai, China). The shRNA sequences were as follows: 5'-CCACCAGAAGAAGAGAAGAAT-3' and 5'-CCCAAGGTCAAGGAGATTATT-3'. The logarithmically-growing SW1088 and SW1783 cells were infected with the lentiviruses carrying the shRNA constructs. The knockdown of DNMT3A gene expression was analyzed by western blotting (WB) and real-time quantitative polymerase chain reaction (RT-qPCR).
Antibodies and agents
The antibodies used in this research are as follows: anti-DNMT3A (Proteintech, San Diego, CA, USA; Cat. No. #20954-1-AP), anti-glyceraldehyde-3-phosphate dehydrogenase (GAPDH; Proteintech; Cat. No. #10494-1-AP), anti-glial fibrillary acidic protein (GFAP; Proteintech; Cat. No. #16825-1-AP), 4',6-diamidino-2-phenylindole (DAPI; UElandy Co., Ltd., Suzhou, China; Cat. No. #D4080), cell counting kit-8 (CCK-8; Glpbio, Co., Ltd., Montclair, CA, USA; Cat. No. #GK10001), 5-ethynyl-2'-deoxyuridine (EdU; UElandy Co., Ltd.; Cat. No. #C6015S), crystal violet (Solarbio, Beijing, China; Cat. No. #G1061).
WB
The whole cell protein lysates were prepared by lysing the cells on ice for 30 min with radioimmunoprecipitation assay (RIPA) lysis buffer (Beyotime, Shanghai, China) containing 1% phenylmethylsulfonyl fluoride (PMSF). The lysates were centrifuged at 12,000 rpm for 15 min at 4 ℃ and the supernatant was collected. The protein concentration of samples was determined using the bicinchoninic acid (BCA) assay (GLPBio). Equal amounts of protein samples were separated by sodium dodecyl sulfate-polyacrylamide gel electrophoresis (SDS-PAGE) and then transferred to the polyvinylidene difuoride (PVDF) membrane with 0.22 μm pore size. The membranes were then blocked with 10% skimmed milk at room temperature for 30 min. The membranes were then incubated overnight at 4 ℃ with the primary antibody. Then, the membranes were washed thrice for 30 min each with TBST. Subsequently, the membranes were incubated with the secondary antibodies at room temperature for 3 h. The protein bands were developed with the enhanced chemiluminescence (ECL) substrate using the GV6000M automatic chemiluminescence imaging system (GelView 6000pro, Guangzhou, China). The gray values of the protein bands were measured using ImageJ and DNMT3A protein levels were normalized to the GAPDH protein levels.
RT-qPCR
Total RNA was extracted from the control and DNMT3A knockdown cells using the RNA extraction kit (BioFlux Co., Ltd., Beijing, China). The complementary DNA (cDNA) was prepared from the total RNA using the reverse transcription kit (UElandy Co., Ltd.). The RT-qPCR analysis was performed using the RT-qPCR kit (UElandy, Co., Ltd.) according to the manufacturer’s instructions. The relative mRNA levels were analyzed using the 2−ΔΔCT method with GAPDH as the internal control. The qPCR primers used in this experiment were as follows: GAPDH-forward (F), TATGAGAGCTGGGGAATGGGA; GAPDH-reverse (R), ATGGCATGGACTGTGGTCTG; DNMT3A-F, TACCTGGTCCTTGGGCTTCT; DNMT3A-R, GTGGGGTGGGAGGTAGAGAT.
Immunohistochemistry and immunohistofluorescence
The paraffin embedded tissues were cut into 3 µm thick slices, mounted on slides, air dried, and processed by immunohistochemistry and immunohistofluorescence. The slices were dewaxed, dehydrated with increasing concentrations of ethanol, incubated with hydrogen peroxide, and boiled in sodium citrate buffer for antigen repair in a microwave oven. Then, the tissue slices were incubated overnight with the diluted primary antibody against DNMT3A (1:100) at 4 ℃. Subsequently, the slices were incubated with the secondary antibody at room temperature for 2 h. Then, the slides were washed thrice with phosphate-buffered saline (PBS) at room temperature for 2 h. The slides were developed with the diaminobenzidine (DAB) staining solution for 5 minutes. Development of a brownish yellow color indicated a positive signal. The slices were counterstained with the hematoxylin staining solution for nuclear staining. The developed sections were imaged under a confocal microscope (Leica Co., Ltd., Wetzlar, Germany). and the signals were quantitatively analyzed using the “IHC Profiler” package of the ImageJ software. The preliminary steps for immunofluorescence staining were similar to immunohistochemistry. The sections were incubated overnight with antibodies against GFAP and DNMT3A. Subsequently, the sections were incubated with the Cy3-tagged (1:300) and AF488-tagged (1:400) secondary antibodies in a dark box for 1 h. Finally, the sections were incubated with DAPI for 20 s for nuclear staining. The stained sections were observed and photographed by confocal microscopy.
Enzyme-linked immunosorbent assay (ELISA)
TNF-α levels in the culture supernatants of SW1088 cells were analyzed using the ELISA assay kit (Cat. No. #BSKH1014; Bioss Company, Beijing, China). Briefly, SW1088 cells were cultured in six-well plates. Then, the cells were transfected with plasmids. The culture supernatants were collected after 24 h and assayed with the ELISA kit according to the manufacturer’s instructions. Finally, the optical density (OD) values were estimated using a microplate reader. TNF-α levels were estimated using the standard curve.
CCK-8
The control and sh-DNMT3A-transfected SW1088 cells (1,000 cells per well) were grown in 96-well plates with 200 µL medium for different time points (0, 24, 48, 72, and 96 h). Then, the cells in each well were incubated with 10 µL CCK-8 test solution at 37 ℃ for 1 h. Subsequently, the OD values were estimated at 450 nm using the enzyme-labeling instrument.
Colony formation experiment
We seeded control and sh-DNMT3A-transfected SW1088 cells in six-well plates (1,000 cells per well). The cells were grown until visible colonies were observed. The colonies were stained with the crystal violet solution (Solarbio) at 4 ℃ overnight and photographed under a microscope. The number of clones were estimated using the ImageJ software.
EdU assay
The control and sh-DNMT3A-transfected SW1088 cells were seeded in 24-well plates. After growing to 70% density, the medium was removed and fresh medium with the EdU dye was added. The cells were then cultured at 37 ℃ for 2 h, washed with PBS, fixed with 4% paraformaldehyde solution, and permeabilized with 0.2% Triton X-100 solution. Finally, the cells were incubated with the reaction solution and Hoechst33342 solution in the dark for 5 minutes. The stained cells were photographed using a fluorescence microscope and the proportion of EdU-positive cells were estimated using the ImageJ software.
Transwell migration and invasion assay
The migration and invasion ability of the control and sh-DNMT3A-transfected cells were analyzed using BD Transwell chamber. For the invasion experiments, the upper chambers were precoated with the matrix glue. Then, control and sh-DNMT3A-transfected SW1088 and SW1783 cells were harvested and diluted with serum-free medium to obtain a concentration of 4×105/mL. Subsequently, we added 500 µL of cell suspension in the upper chamber and 500 µL of DMEM containing 20% FBS in the bottom chamber. The transwell chambers were incubated at 37 ℃ for 48 h. Then the cells on the upper surface of the chamber were carefully removed with a cotton swab. The migrated or invading cells were fixed with 4% paraformaldehyde, stained with 0.1% crystal violet, and photographed using a confocal microscope in three random frames. Subsequently, the cells were counted using the ImageJ software. The experiment was repeated three times.
Cell cycle analysis
The control and sh-DNMT3A-transfected SW1088 cells were harvested and counted. Then, equal number of cells from all samples were fixed overnight in 70% ethanol at −20 ℃. Subsequently, the cells were pelleted by centrifugation to remove the 70% ethanol. Then, the cells were incubated with freshly prepared propidium iodide solution on ice for 1 h. Subsequently, flow cytometry was performed to determine the proportion of cells in the G1 and S-G2/M phases of the cell cycle.
Statistical analysis
Kaplan-Meier survival curve analysis was performed using the “survminer” package. The survival rates of the high- and low-DNMT3A expression groups of LGG patients were compared using the log-rank test. The decision curve analysis (DCA) curve analysis was performed using the “stdca” package to determine the clinical predictive potential of DNMT3A expression. The Students’ t-test was used to compare the differences in data between two groups including differences in DNMT3A expression, immune scores, stromal scores, tumor mutation load, and other parameters. Univariate and multivariate regression analyses were performed using the “rms” packages to determine the prognostic efficacy of DNMT3A and nomogram models were constructed based on the results. The performance of the nomogram was verified using C-index and calibration curves. All the experiments were replicated at least three times under the same conditions. The statistical analyses were performed using the R, Perl, SPSS, ImageJ software. Graphpad and Adobe Illustrate (AI) software were used for data presentation.
ResultsOther Section
High expression of DNMT3A is associated with poor prognosis in LGG
We first analyzed the DNMT3A expression levels in tumor tissues from 33 cancer types, including LGG, based on data from the TCGA and GTEx databases. DNMT3A expression was significantly higher in most tumor tissues compared with the corresponding normal tissues (Figure 2A). Furthermore, higher DNMT3A expression was associated with poor prognosis in tumors such as LGG, adrenocortical carcinoma (ACC), liver hepatocellular carcinoma (LIHC), acute myeloid leukemia (LAML), prostate adenocarcinoma (PRAD), sarcoma (SARC). Furthermore, higher DNMT3A expression was associated with good prognosis in thymoma (THYM) (Figure 2B). We then analyzed the prognosis of LGG patients in three different cohorts based on DNMT3A expression levels and observed that high expression of DNMT3A in the LGG patients was associated with poor prognosis (Figure 2C-2E). These results suggested that DNMT3A promoted LGG development and progression.
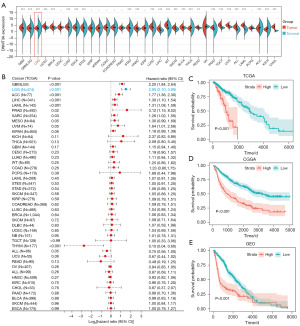
DNMT3A expression levels correlate with the clinical features in LGG
We then analyzed the relationship between various clinicopathological features and DNMT3A expression in LGG patients. The patients were divided into high and low DNMT3A expression groups. The characteristics of different clinicopathological parameters in LGG patients with high and low DNMT3A expression levels are shown in the heatmaps in Figure 3A. We analyzed the relationship between various clinicopathological features and DNMT3A expression separately. Patients were grouped according to clinicopathological features and the differences in the expression levels of DNMT3A were analyzed for different subgroups and the results were shown as violin plots in Figure 3B-3D. Our data showed that DNMT3A expression correlated with glioma grades, 1p19q co-deletion, and MGMT methylation levels in the LGG patients. In addition, we examined the differential expression of DNMT3A in different types of tumors and found that astrocytomas have a higher expression of DNMT3A compared to oligodendrogliomas. We also performed the same analysis in the validation dataset and obtained results that were consistent with the test dataset.
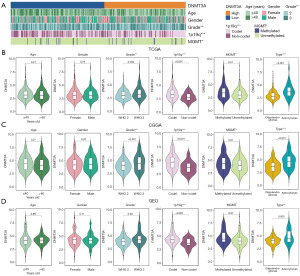
Construction and validation of the prognosis prediction nomogram model
We then performed univariate and multivariate regression analyses of various clinicopathological parameters, including DNMT3A expression to determine whether DNMT3A expression was an independent prognostic indicator in LGG. We calculated the hazard ratios and P values for each of the clinicopathological feature in the test dataset of LGG patients. The forest plots show the independent prognostic prediction performances of all the clinicopathological indicators in the patients with LGG. The results showed that age, WHO grade, 1p19q co-deletion, and DNMT3A expression were independent prognostic predictors in LGG (Figure 4A,4B). However, the results of the regression analyses in the validation dataset were unsatisfactory (Figure 4C-4F). We then constructed a nomogram prognosis model with the following three risk factors: age, LGG grade, and DNMT3A expression level (Figure 5A). From the above figure, we can find the corresponding scores based on the patient’s basic information. After adding them up, we can obtain the total score to determine the 1-, 3-, and 5-year survival rates of the patients and determine their prognosis. We applied meta-CGGA and GEO databases for nomogram validation, and calculated the corrected C-index for each cohort from the calibration curves. The results show that nomogram model showed significant accuracy in predicting the 1-, 3-, and 5-year prognosis of the LGG patients (Figure 5B-5D). The DCA curve results showed that the nomogram model was more accurate than the independent risk factors alone in predicting the 3- and 5-year OS rate of LGG patients (Figure 5E-5J).
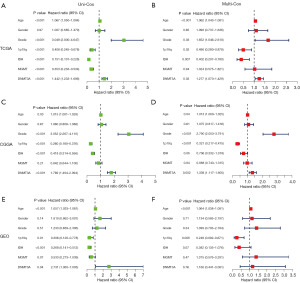
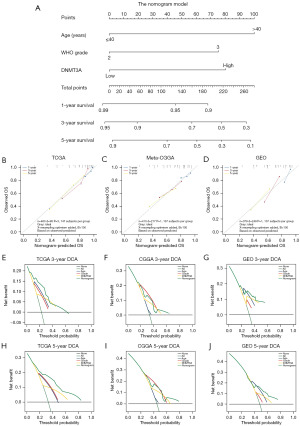
Function enrichment analysis of DNMT3A-related DEGs
We then identified 948 DEGs (P<0.05) between LGG patients with high and low DNMT3A expression using the “limma” package (29). Subsequently, we performed functional enrichment analysis and multiGSEA of these 948 DEGs (30) to identify the underlying mechanisms by which DNMT3A regulates LGG development and progression. The GO analyses of the TCGA-LGG dataset showed enrichment of GO terms such as process of ontogeny, organ development, and DNA transcription (Figure 6A). However, only one relevant pathway was found in the KEGG pathway analyses of 948 DEGs. Furthermore, we performed multiGSEA analysis and found that DNMT3A expression was closely associated with several developmental diseases, including Huntington’s disease and Parkinson’s disease (Figure 6B). Finally, we performed gene set variation analysis (GSVA) to analyze the regulatory functions of DNMT3A in LGG patients. As shown in the heat maps, DNMT3A expression levels regulated various inflammatory signaling pathways like IL6-JAK-STAT3, IL2-STAT5, and TNF-α/NF-κB signal pathways (Figure 6C).
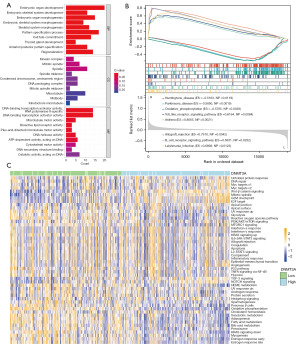
DNMT3A expression is associated with tumor immune infiltration in LGG
The GO analysis results showed that the expression of DNMT3A was significantly correlated with the development and progression of immune cells. This suggested that the expression of DNMT3A was closely related with the infiltration of immune cells into the LGG tissues. The expression profiles of LGG patients in the TCGA database were analyzed and the immune infiltration scores were calculated for each LGG patient using single-sample GSEA (ssGSEA). We observed significant differences between LGG patients with high and low DNMT3A expression levels based on different types of scores. Furthermore, the infiltration levels of immune cells, including antigen presenting cells (APCs), dendritic cells (DCs), mast cells, and natural killer (NKs) cells were significantly different between LGG patients with high and low DNMT3A expression levels (Figure 7A). The infiltration levels of distinct types of immune cells in all the LGG patients were analyzed using the TIMER, CIBERSORT, CIBERSORT-ABS, QUANTISEQ, XCELL, and EPIC algorithms. Our data showed that the infiltration levels of multiple immune cell types were slightly higher in the high DNMT3A expression group of LGG patients Then, different algorithms (TIMER, CIBERSORT, CIBERSORT-ABS, QUANTISEQ, XCELL, EPIC, etc.) (Figure 7B). The ESTIMATE algorithm was used to calculate the ESTIMATE score, immune score, and stromal score of each LGG patient in the training dataset (31). Subsequently, we analyzed the data using scatter plots and calculated the best regression curve. Our analysis showed significant positive correlation between various immune scores and DNMT3A expression (P<0.05) in the LGG patients (Figure 7C-7E). Subsequently, we analyzed the expression levels of multiple immune checkpoint genes in the LGG patients with high and low DNMT3A expression groups. Our data showed significant differences in the expression levels of multiple immune checkpoint genes, including CD274, CD276 (Figure 7F).
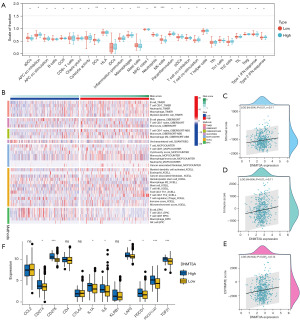
We then investigated the relationship between DNMT3A and the immune checkpoint genes in various tumors, we performed correlation analyses of the expression levels of 47 immunomodulatory genes and DNMT3A in various tumors. As shown in the heat map, DNMT3A expression correlated with the expression levels of multiple immunomodulatory genes in several tumors (Figure S1A). In lymphoid neoplasm diffuse large B-cell lymphoma (DLBC), kidney chromophobe (KICH), and uveal melanoma (UVM) tumors, we observed significant positive correlation between the expression levels of DNMT3A and most of the immunomodulatory genes, whereas, in testicular germ cell tumor (TGCT), we observed significant negative correlation between the expression levels of DNMT3A and most of the immunomodulatory genes. This again highlighted the immunomodulatory effects of DNMT3A.This again highlighted the immunomodulatory effects of DNMT3A.
Next, we analyzed the effects of DNMT3A expression on the immunotherapy response by calculating the tumor mutational burden (TMB) and microsatellite instability (MSI) scores for various types of tumors. Our data showed that the DNMT3A expression levels were closely associated with the TMB scores in bladder urothelial carcinoma (BLCA), breast invasive carcinoma (BRCA), colon adenocarcinoma (COAD), DLBC, esophageal carcinoma (ESCA), kidney renal clear cell carcinoma (KIRC), kidney renal papillary cell carcinoma (KIRP), LAML, LGG, lung adenocarcinoma (LUAD), PRAD, rectum adenocarcinoma (READ), SARC, stomach adenocarcinoma (STAD), thyroid carcinoma (THCA), THYM, uterine corpus endometrial carcinoma (UCEC), and uterine carcinosarcoma (USC). Furthermore, we observed significant positive correlation between DNMT3A and expression levels and the MSI index in BLCA, BRCA, LAML, LGG, LUAD, and PRAD (Figure S1B). DNMT3A expression levels showed significant negative correlation with the MSI index in COAD, DLBC, ESCA (Figure S1C). These results suggested that DNMT3A expression was a potential predictor of the efficacy of immune checkpoint inhibitor therapy in several tumors. Finally, we analyzed the prognostic effects of DNMT3A expression in two immunotherapy cohorts, GSE91061 and VanAllen2015 cohorts. We performed survival analysis of patients with high or low DNMT3A expression to determine the efficacy of immunotherapy. In both GSE91061 and VanAllen2015 cohorts, high DNMT3A expression was associated with worse prognosis after immunotherapy, whereas, the immunotherapeutic response was significantly stronger in the low DNMT3A expression group than in the high DNMT3A expression group (Figure S1D,S1E).
DNMT3A is highly expressed in clinical LGG samples and LGG cell lines
We compared DNMT3A mRNA and protein expression levels in three LGG cell lines and one normal neuronal cell line by RT-qPCR and WB, respectively. Our data showed that DNMT3A mRNA and protein levels were significantly higher in the LGG cell lines compared with the normal neuronal cell line (Figure 8A,8B). Since SW-1088 cell line showed the highest DNMT3A expression, we designed DNMT3A knock-down lentiviruses to significantly decrease DNMT3A levels in the SW-1088 cell line. WB and RT-qPCR analyses showed that the knock-down effect was significantly higher with sh-DNMT3A-1 (Figure 8C,8D). Therefore, we selected sh-DNMT3A-2 for subsequent functional assays. Immunohistochemical analysis of clinical LGG tissue samples showed that the expression levels of DNMT3A were significantly up-regulated in the glioma tissues compared with the normal neuronal tissues (Figure 8E). This further confirmed that DNMT3A was highly expressed in the LGG cells. We then performed the immunofluorescence assay with anti-GFAP and anti-DNMT3A antibodies in the glioma sections (32) to analyze the association between the DNMT3A expression levels and the glioma cells. Our data showed co-expression of DNMT3A and GFAP; moreover, higher DNMT3A expression in the glioma tissues correlated with GFAP overexpression (Figure 8F).
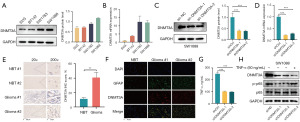
DNMT3A regulates malignancy of LGG cells through the TNF-α/NF-κB signaling pathway
We then analyzed if DNMT3A promoted the progression of glioma. Pan-cancer GSVA was performed to identify the DNMT3A-related cellular signaling pathways in different cancers. GSVA results showed that DNMT3A regulated the malignant progression of glioma through the TNF-α/NF-κB signaling pathway (Figure S2). Therefore, we analyzed TNF-α secretion levels in the control and DNMT3A-silenced LGG cells using an ELISA kit. Our results showed that DNMT3A knockdown decreased the secretion of TNF-α by the LGG cells (Figure 8G). Furthermore, knock down of DNMT3A inhibited NF-κB phosphorylation, but this effect was reversed by exogenous addition of TNF-α (Figure 8H). These experimental results clearly demonstrated that DNMT3A modulated NF-κB phosphorylation in the LGG cells by regulating the secretion of TNF-α, and thereby affected the malignant progression of glioma cells.
DNMT3A silencing suppresses in vitro proliferation and progression of LGG cells
Subsequently, to clarify the role of DNMT3A expression in the malignant phenotype of the LGG cell lines, we performed in vitro functional assays to determine the effects of DNMT3A knockdown on the proliferation, migration, and invasion properties of the LGG cell lines. CCK-8 assay results demonstrated that DNMT3A knockdown significantly reduced the proliferation of glioma cells (Figure 9A). The colony formation assay results demonstrated that DNMT3A knockdown significantly inhibited the colony formation ability of the glioma cells (Figure 9B). Transwell assay results showed that DNMT3A knockdown significantly reduced in vitro migration and invasiveness of the glioma cells (Figure 9C-9E). EdU assay results showed that DNMT3A knockdown significantly reduced proliferation of the glioma cells (Figure 9F). Finally, cell cycle analyses showed that the knockdown of DNMT3A significantly reduced the number of cells in the division phase (S + G2/M), thereby suggesting cell cycle inhibition in the G1 phase (Figure 9G). These results showed that knockdown of DNMT3A inhibited cell cycling, proliferation, migration, and invasion of the LGG cells. This suggested that DNMT3A played a significant role in the malignancy of LGG.
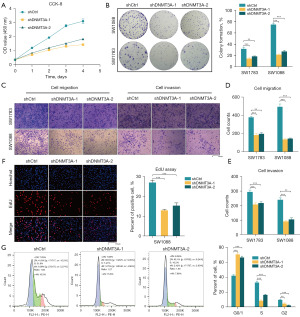
DiscussionOther Section
Currently, the curative effects of conventional treatments for gliomas are not satisfactory. In recent years, immunotherapy has shown significant promise in cancer therapy and has become the mainstream treatment for a variety of tumors (33-35). Immune checkpoint inhibitor therapy and chimeric antigen receptor T cell (CAR-T) therapy have significantly improved the treatment outcomes and prognosis of cancer patients (36-38). However, treatment outcomes vary significantly among human subjects because of individual differences. In this study, we comprehensively analyzed whether DNMT3A expression was a prognostic indicator for patients with LGG. The goal was to improve clinical decisions regarding the choice of personalized treatment plans with chemotherapeutic drugs and identify patients that are amenable for immunotherapy to improve survival outcomes. Our data suggested that DNMT3A expression was a promising biomarker for determining the efficacy of immunotherapy in patients with LGG and other cancers.
Our study shows that DNMT3A was highly expressed in several tumors. Furthermore, higher DNMT3A expression was significantly associated with poor survival outcomes in cancer patients. We performed bioinformatics analyses of the RNA-sequencing and clinical data of 1265 LGG patients from three public databases to determine whether DNMT3A was a tumor prognostic marker in LGG. We performed survival analysis, univariate and multivariate regression analysis, prognostic model construction and calibration. The results of this comprehensive bioinformatics analyses demonstrated that DNMT3A was a tumor prognostic marker in LGG. Next, we analyzed the relationship between DNMT3A expression and the expression levels of immunomodulatory genes to determine whether DNMT3A modulated infiltration of immune cell types into the tumor microenvironment. Our data showed that higher DNMT3A expression was associated with increased infiltration of immune cells, including DC cells and NK cells (39,40). We then performed prognostic analysis and Cox regression analyses in two independent cohorts of cancer patients receiving immune checkpoint therapy. The results demonstrated that DNMT3A expression levels predicted treatment outcomes in patients receiving immunotherapy. Cancer patients with high DNMT3A expression levels were sensitive to immunotherapy. Furthermore, in vitro experiments demonstrated that downregulation of DNMT3A by shRNA knockdown significantly reduced proliferation and malignant progression of the LGG cells.
Aberrant activation of the TNF-α/NF-κB signaling pathways is closely related with cancer development and progression (41,42). TNF-α is an important regulator of immunity and inflammatory responses. It also plays a significant role in several physiological and pathological processes. NF-κB is a key transcription factor that regulates expression levels of genes related with inflammation, apoptosis, and metabolism (43). Previous studies have shown that multiple types of tumor cells and pro-inflammatory immune cells secrete large amounts of TNF-α. Excessive stimulation of TNF-α via its cell surface receptor triggers activation and phosphorylation of NF-κB. The activated NF-κB translocates to the nucleus and induces gene transcription response that promotes tumor cell proliferation and survival as well as the inflammatory response, thereby providing favorable conditions for the growth and metastasis of tumor cells (44). Therefore, targeted inhibition of the TNF-α/NF-κB signaling pathway is one of the mechanisms by which the growth and malignant progression of the glioma cells can be effectively inhibited.
Tumor therapy outcomes are closely related with the status of the tumor microenvironment (45). The tumor microenvironment is complex and involves several different cell types, including tumor cells, stromal cells, immune cell types, and other cells. These cell types co-operatively regulate the survival of tumor cells (46). Therefore, the outcomes of cancer treatment other than the surgical treatment is dependent on its effects on the tumor microenvironment (47). Therefore, in this study, we specifically analyzed the effects of the tumor microenvironment on the efficacy of tumor immunotherapy, especially in relation to the DNMT3A expression levels in the tumor tissues. The soil-seed theory has now been accepted by most cancer researchers. The tumor cells are regarded as seeds that need to be in a conducive immune microenvironment for growth and progression. Therefore, altered tumor immune environment can be used as an effective strategy to inhibit the growth of tumor cells (48). For example, immune checkpoint inhibitor therapy is successfully used to enhance the specific recognition of tumor cells by the immune cells (49). This reduces the tumor immune escape but has no adverse effects on other normal cells in the tumor microenvironment. Therefore, immunotherapy has shown significant tumor therapy effects and improved targeting of tumor cells.
DNA methylation is an essential epigenetic mechanism that regulates gene expression in cells by recruiting of gene expression inhibitory proteins or inhibitory transcription factors to the DNA methylation sites (50,51). DNMT3A is one of the executors of intracellular DNA methylation. Aberrant expression of DNAMT3A is associated with abnormal DNA methylation, which is commonly found in cancer cells and is also related with increased cancer risk and malignant tumor behavior (52,53). Therefore, strict monitoring of intracellular DNMT3A expression is of great significance for determining the status of DNA methylation. In recent times, several studies have shown that DNMT3A expression is associated with tumor development and patient prognosis.
Our study has a few limitations., Most of our clinical data was based on the public databases. Therefore, further validation in large cohort prospective studies is required to confirm our results. However, based on our experimental results and available literature, our data suggested strongly that DNMT3A expression regulated the growth and progression of LGG patients.
In summary, we constructed a nomogram with DNMT3A expression as one of the biomarkers and verified that it accurately predicted the prognosis of LGG patients. We demonstrated that high DNMT3A expression promoted cell cycling, proliferation, and malignancy of the glioma cells. We identified DNMT3A as a potential prognostic biomarker for patients with LGG. Therefore, DNMT3A is a promising target for gene therapy in LGG patients.
ConclusionsOther Section
DNMT3A, as a tumor prognostic marker, can predict the prognosis of glioma patients robustly. High expression in tumor tissue may be one of the risk factors for poor prognosis in glioma patients. By constructing a clinical prediction model, we have identified the role of DNMT3A expression in predicting survival in glioma patients. Through enrichment analysis results and experiments, it has been demonstrated that DNMT3A regulates the progression of gliomas through the TNF-α/NF-κB signaling pathway.
AcknowledgmentsOther Section
We sincerely acknowledge the contributions from the TCGA, CGGA, GEO database and Sanger box (http://vip.sangerbox.com/login.html) to this study.
Funding: This research was funded by
FootnoteOther Section
Reporting Checklist: The authors have completed the TRIPOD and MDAR reporting checklists. Available at https://tcr.amegroups.com/article/view/10.21037/tcr-23-1943/rc
Peer Review File: Available at https://tcr.amegroups.com/article/view/10.21037/tcr-23-1943/prf
Conflicts of Interest: All authors have completed the ICMJE uniform disclosure form (available at https://tcr.amegroups.com/article/view/10.21037/tcr-23-1943/coif). The authors have no conflicts of interest to declare.
Ethics Statement: The authors are accountable for all aspects of the work in ensuring that questions related to the accuracy or integrity of any part of the work are appropriately investigated and resolved. This study was conducted in accordance with the Declaration of Helsinki (as revised in 2013). All LGG and NBT samples from human body in this study have been certified by the Ethics Committee of the 2nd Affiliated Hospital of Nanchang University. All the patients have signed the informed consent.
Open Access Statement: This is an Open Access article distributed in accordance with the Creative Commons Attribution-NonCommercial-NoDerivs 4.0 International License (CC BY-NC-ND 4.0), which permits the non-commercial replication and distribution of the article with the strict proviso that no changes or edits are made and the original work is properly cited (including links to both the formal publication through the relevant DOI and the license). See: https://creativecommons.org/licenses/by-nc-nd/4.0/.
ReferencesOther Section
- Ostrom QT, Gittleman H, Truitt G, et al. CBTRUS Statistical Report: Primary Brain and Other Central Nervous System Tumors Diagnosed in the United States in 2011-2015. Neuro Oncol 2018;20:iv1-iv86. [Crossref] [PubMed]
- Alifieris C, Trafalis DT. Glioblastoma multiforme: Pathogenesis and treatment. Pharmacol Ther 2015;152:63-82. [Crossref] [PubMed]
- Karachi A, Dastmalchi F, Mitchell DA, et al. Temozolomide for immunomodulation in the treatment of glioblastoma. Neuro Oncol 2018;20:1566-72. [Crossref] [PubMed]
- van den Bent MJ, Tesileanu CMS, Wick W, et al. Adjuvant and concurrent temozolomide for 1p/19q non-co-deleted anaplastic glioma (CATNON; EORTC study 26053-22054): second interim analysis of a randomised, open-label, phase 3 study. Lancet Oncol 2021;22:813-23. [Crossref] [PubMed]
- Wan PK, Ryan AJ, Seymour LW. Beyond cancer cells: Targeting the tumor microenvironment with gene therapy and armed oncolytic virus. Mol Ther 2021;29:1668-82. [Crossref] [PubMed]
- van Tellingen O, Yetkin-Arik B, de Gooijer MC, et al. Overcoming the blood-brain tumor barrier for effective glioblastoma treatment. Drug Resist Updat 2015;19:1-12. [Crossref] [PubMed]
- Lim M, Xia Y, Bettegowda C, et al. Current state of immunotherapy for glioblastoma. Nat Rev Clin Oncol 2018;15:422-42. [Crossref] [PubMed]
- Wesseling P, Capper D. WHO 2016 Classification of gliomas. Neuropathol Appl Neurobiol 2018;44:139-50. [Crossref] [PubMed]
- Weller M, Wick W, Aldape K, et al. Glioma. Nat Rev Dis Primers 2015;1:15017. [Crossref] [PubMed]
- Mair MJ, Geurts M, van den Bent MJ, et al. A basic review on systemic treatment options in WHO grade II-III gliomas. Cancer Treat Rev 2021;92:102124. [Crossref] [PubMed]
- Louis DN, Perry A, Reifenberger G, et al. The 2016 World Health Organization Classification of Tumors of the Central Nervous System: a summary. Acta Neuropathol 2016;131:803-20. [Crossref] [PubMed]
- Suzuki H, Aoki K, Chiba K, et al. Mutational landscape and clonal architecture in grade II and III gliomas. Nat Genet 2015;47:458-68. [Crossref] [PubMed]
- Luo H, Ye M, Hu Y, et al. DNA methylation regulator-mediated modification patterns and tumor microenvironment characterization in glioma. Aging (Albany NY) 2022;14:7824-50. [Crossref] [PubMed]
- Klutstein M, Nejman D, Greenfield R, et al. DNA Methylation in Cancer and Aging. Cancer Res 2016;76:3446-50. [Crossref] [PubMed]
- Li E, Zhang Y. DNA methylation in mammals. Cold Spring Harb Perspect Biol 2014;6:a019133. [Crossref] [PubMed]
- Chen T, Ueda Y, Xie S, et al. A novel Dnmt3a isoform produced from an alternative promoter localizes to euchromatin and its expression correlates with active de novo methylation. J Biol Chem 2002;277:38746-54. [Crossref] [PubMed]
- Heyn P, Logan CV, Fluteau A, et al. Gain-of-function DNMT3A mutations cause microcephalic dwarfism and hypermethylation of Polycomb-regulated regions. Nat Genet 2019;51:96-105. [Crossref] [PubMed]
- Lyko F. The DNA methyltransferase family: a versatile toolkit for epigenetic regulation. Nat Rev Genet 2018;19:81-92. [Crossref] [PubMed]
- Viré E, Brenner C, Deplus R, et al. The Polycomb group protein EZH2 directly controls DNA methylation. Nature 2006;439:871-4. [Crossref] [PubMed]
- Zhang ZM, Lu R, Wang P, et al. Structural basis for DNMT3A-mediated de novo DNA methylation. Nature 2018;554:387-91. [Crossref] [PubMed]
- Cao J, Wu Q, Huang Y, et al. The role of DNA methylation in syndromic and non-syndromic congenital heart disease. Clin Epigenetics 2021;13:93. [Crossref] [PubMed]
- Meng H, Cao Y, Qin J, et al. DNA methylation, its mediators and genome integrity. Int J Biol Sci 2015;11:604-17. [Crossref] [PubMed]
- Zhao Y, Li MC, Konaté MM, et al. TPM, FPKM, or Normalized Counts? A Comparative Study of Quantification Measures for the Analysis of RNA-seq Data from the NCI Patient-Derived Models Repository. J Transl Med 2021;19:269. [Crossref] [PubMed]
- Rosenberg JE, Hoffman-Censits J, Powles T, et al. Atezolizumab in patients with locally advanced and metastatic urothelial carcinoma who have progressed following treatment with platinum-based chemotherapy: a single-arm, multicentre, phase 2 trial. Lancet 2016;387:1909-20. [Crossref] [PubMed]
- Gide TN, Quek C, Menzies AM, et al. Distinct Immune Cell Populations Define Response to Anti-PD-1 Monotherapy and Anti-PD-1/Anti-CTLA-4 Combined Therapy. Cancer Cell 2019;35:238-255.e6. [Crossref] [PubMed]
- The Gene Ontology Resource. 20 years and still GOing strong. Nucleic Acids Res 2019;47:D330-8. [Crossref] [PubMed]
- Yu G, Wang LG, Han Y, et al. clusterProfiler: an R package for comparing biological themes among gene clusters. OMICS 2012;16:284-7. [Crossref] [PubMed]
- Camby I, Salmon I, Danguy A, et al. Influence of gastrin on human astrocytic tumor cell proliferation. J Natl Cancer Inst 1996;88:594-600. [Crossref] [PubMed]
- Ritchie ME, Phipson B, Wu D, et al. limma powers differential expression analyses for RNA-sequencing and microarray studies. Nucleic Acids Res 2015;43:e47. [Crossref] [PubMed]
- Subramanian A, Tamayo P, Mootha VK, et al. Gene set enrichment analysis: a knowledge-based approach for interpreting genome-wide expression profiles. Proc Natl Acad Sci U S A 2005;102:15545-50. [Crossref] [PubMed]
- Yoshihara K, Shahmoradgoli M, Martínez E, et al. Inferring tumour purity and stromal and immune cell admixture from expression data. Nat Commun 2013;4:2612. [Crossref] [PubMed]
- Wefers AK, Stichel D, Schrimpf D, et al. Isomorphic diffuse glioma is a morphologically and molecularly distinct tumour entity with recurrent gene fusions of MYBL1 or MYB and a benign disease course. Acta Neuropathol 2020;139:193-209. [Crossref] [PubMed]
- Huang B, Li X, Li Y, et al. Current Immunotherapies for Glioblastoma Multiforme. Front Immunol 2021;11:603911. [Crossref] [PubMed]
- Xun Y, Yang H, Kaminska B, et al. Toll-like receptors and toll-like receptor-targeted immunotherapy against glioma. J Hematol Oncol 2021;14:176. [Crossref] [PubMed]
- Zhang Y, Zhang Z. The history and advances in cancer immunotherapy: understanding the characteristics of tumor-infiltrating immune cells and their therapeutic implications. Cell Mol Immunol 2020;17:807-21. [Crossref] [PubMed]
- Brown CE, Mackall CL. CAR T cell therapy: inroads to response and resistance. Nat Rev Immunol 2019;19:73-4. [Crossref] [PubMed]
- Tang L, Wang J, Lin N, et al. Immune Checkpoint Inhibitor-Associated Colitis: From Mechanism to Management. Front Immunol 2021;12:800879. [Crossref] [PubMed]
- Sterner RC, Sterner RM. CAR-T cell therapy: current limitations and potential strategies. Blood Cancer J 2021;11:69. [Crossref] [PubMed]
- Shimasaki N, Jain A, Campana D. NK cells for cancer immunotherapy. Nat Rev Drug Discov 2020;19:200-18. [Crossref] [PubMed]
- Wculek SK, Cueto FJ, Mujal AM, et al. Dendritic cells in cancer immunology and immunotherapy. Nat Rev Immunol 2020;20:7-24. [Crossref] [PubMed]
- Coelho-Santos V, Leitão RA, Cardoso FL, et al. The TNF-α/NF-κB signaling pathway has a key role in methamphetamine-induced blood-brain barrier dysfunction. J Cereb Blood Flow Metab 2015;35:1260-71. [Crossref] [PubMed]
- Ren X, Zhong W, Li W, et al. Human Umbilical Cord-Derived Mesenchymal Stem Cells Alleviate Psoriasis Through TNF-α/NF-κB/MMP13 Pathway. Inflammation 2023;46:987-1001. [Crossref] [PubMed]
- Ma DJ, Li SJ, Wang LS, et al. Temporal and spatial profiling of nuclei-associated proteins upon TNF-alpha/NF-kappaB signaling. Cell Res 2009;19:651-64. [Crossref] [PubMed]
- Yan T, Tan Y, Deng G, et al. TGF-β induces GBM mesenchymal transition through upregulation of CLDN4 and nuclear translocation to activate TNF-α/NF-κB signal pathway. Cell Death Dis 2022;13:339. [Crossref] [PubMed]
- Hinshaw DC, Shevde LA. The Tumor Microenvironment Innately Modulates Cancer Progression. Cancer Res 2019;79:4557-66. [Crossref] [PubMed]
- Xiao Y, Yu D. Tumor microenvironment as a therapeutic target in cancer. Pharmacol Ther 2021;221:107753. [Crossref] [PubMed]
- Pitt JM, Marabelle A, Eggermont A, et al. Targeting the tumor microenvironment: removing obstruction to anticancer immune responses and immunotherapy. Ann Oncol 2016;27:1482-92. [Crossref] [PubMed]
- Langley RR, Fidler IJ. The seed and soil hypothesis revisited--the role of tumor-stroma interactions in metastasis to different organs. Int J Cancer 2011;128:2527-35. [Crossref] [PubMed]
- Moslehi J, Lichtman AH, Sharpe AH, et al. Immune checkpoint inhibitor-associated myocarditis: manifestations and mechanisms. J Clin Invest 2021;131:e145186. [Crossref] [PubMed]
- Moore LD, Le T, Fan G. DNA methylation and its basic function. Neuropsychopharmacology 2013;38:23-38. [Crossref] [PubMed]
- Héberlé É, Bardet AF. Sensitivity of transcription factors to DNA methylation. Essays Biochem 2019;63:727-41. [Crossref] [PubMed]
- Mahmoud AM, Ali MM. Methyl Donor Micronutrients that Modify DNA Methylation and Cancer Outcome. Nutrients 2019;11:608. [Crossref] [PubMed]
- Kondo Y, Issa JP. DNA methylation profiling in cancer. Expert Rev Mol Med 2010;12:e23. [Crossref] [PubMed]