An innovative prognostic auxiliary for colon adenocarcinoma based on zinc finger protein genes
Highlight box
Key findings
• Our research has examined the features of zinc finger (ZNF) genes as prognostic predictive biomarkers for colon adenocarcinoma (COAD).
• There is a high probability that model genes are attached to immune-related biological processes
What is known and what is new?
• A growing number of studies revealed that ZNF protein genes are intimately related to COAD growth and progression.
• There is no such signature model taking advantage of ZNF protein genes in COAD.
What is the implication, and what should change now?
• The signature may be applied as a predictive classifier to guide treatment decisions for COAD patients.
Introduction
Deaths from colon adenocarcinoma (COAD) account for a significant proportion of all human cancer deaths (1). The five-year survival rate of colon cancer patients has remained unsatisfactory throughout the last couple of decades despite significant progress in screening, diagnosis, and management of this malignancy (2,3). Currently, there is a lack of specific and sensitive biomarker signatures in clinical practice for COAD patients to determine the risk of prognosis and distinguish which group of patients respond better to medical treatment (4). Therefore, identifying new biomarkers or assessment models to improve this situation is particularly important.
Zinc finger (ZNF) protein is a transcription factor superfamily containing at the minimum one ZNF unit member, which is crucial in the modulation of many basic mechanisms such as differentiation, proliferation, metabolism, and apoptosis of cancer cells (5,6). In particular, a growing number of studies revealed that ZNF protein genes are intimately related to COAD growth and progression (7). For instance, ZKSCAN3 was found to be more abundant in the colorectal tumor group than in adjacent nonmalignant mucosa, and it has been found to be linked to oncogenesis by transcriptionally activating integrins β4 and vascular endothelial growth factor (8). According to Hahn and Li et al., ZNF281 expression not only increases during tumor progression but also promotes the recurrence of colorectal cancer through enhancing the epithelial-mesenchymal transition (EMT), and KLF2, a carcinoma inhibitor existing in low concentrations in colorectal cancer tissues (9,10). These studies indicate that aberrant expressions of ZNF genes may represent potentially robust indicators for COAD patients’ prognosis and survival.
Owing to the development of sequencing technology and access to public databases, researchers have attempted to establish risk assessment models to identify the gene signatures of cancer. Interestingly, multiple studies have demonstrated that signatures based on ZNF protein gene expressions reveal prognostic information in multiple cancers (11-14). However, there is no such signature model taking advantage of ZNF protein genes in COAD. As a result, we searched for a model taking advantage of ZNF protein genes that might be convenient for forecasting the outcome of COAD patients and leading medication decisions. During this research, we first produced and verified a seven-gene prognosis model utilizing ZNF protein genes for COAD patients. Also, we performed relative functional analysis to investigate the bond among our prognostic model and immunity, mutation loads, and distinctions in chemotherapy and immunotherapy responses. Finally, the ZNF gene signature was proved to be beneficial in assessing clinical prognosis in COAD patients. We present this article in accordance with the TRIPOD reporting checklist (available at https://tcr.amegroups.com/article/view/10.21037/tcr-23-2158/rc).
Methods
Acquisition of metadata
The transcriptional [fragments per kilobase of exon per million mapped reads (FPKM)] and mutation of 465 COAD samples and 41 adjacent normal samples, and relational clinical data of 143 COAD patients were downloaded from The Cancer Genome Atlas (TCGA) database (https://portal.gdc.cancer.gov/). These datasets were used for the training and internal testing cohorts. The gene expression profiles and clinical data from 55 CC samples (GSE17537 cohort) were downloaded from the publicly available Gene Expression Omnibus (GEO) database (https://www.ncbi.nlm.nih.gov/geo/), with this cohort being used for external validation. We screened the data to identify patients who had attended the required number of follow-up appointments and whose survival outcome was documented. Furthermore, for the purpose to decrease the batch effect and guaranteeing that the study could be effectively replicated, we standardized the RNA-Seq data of the GEO and TCGA sets via the R software’s “limma” function. All processes in this study followed the policy and guidelines provided by the GEO and TCGA databases. A total of 1,751 ZNF protein genes came from the UniProt database (https://www.uniprot.org/).
Development and confirmation of a prognosis-linked ZNF protein genes model
A univariate Cox regression analysis was carried out applying the “survival” and “survminer” R Packages in the TCGA-COAD cohort. ZNF protein genes corresponding to prognostic were those with a P value less than 0.01. The concept of “entire cohort” referred to the TCGA data going forward. This cohort was stochastically split in half, and one dataset was used as the “training cohort”. To lessen the odds of overfitting, LASSO regression analysis was conducted on the prognosis-linked ZNF protein genes with the R Package “glmnet” in the training cohort. The regression coefficients were subsequently acquired by multivariate Cox regression analysis utilizing the R “survival” package in the training cohort. The results from the training cohort analyses were employed to make the model. The normalized expressed degree of the signature genes and the associated regression coefficients were combined to produce the patient risk score with the next equation:
We classified the patients in the training cohort into two groups with different risk statuses: high and low, adopting the median number of each patient’s risk score as a threshold. Next, the two groups’ overall survival (OS) differences were noted, and the R package’s “survival” and “survminer” functions were employed to do a Kaplan-Meier (KM) survival analysis of OS. OS was defined as the time from diagnosis to death. Next, the R package “timeROC” was employed for performing time-dependent receiver operating characteristic (ROC) curve analysis for assessing the effectiveness of the model for forecasting the future prospects of COAD patients. The risk scores of the patients in the entire cohort and the GSE17537 cohort were counted using the aforementioned formula. To confirm the risk score’s independence, we integrated the clinical characteristics (including M stage, gender, stage, age, T stage, the risk scores, as well as N stage), then univariable and multivariate Cox regression analyses were performed. P values of less than 0.05 were considered statistically significant. To illustrate the effectiveness of the model, plots of patients’ gene expression, risk score distribution, and survival status in several cohorts were created.
Construction of a prognostic nomogram
A nomogram is an effective instrument for forecasting the clinical survival of patients. For creating the nomogram, we merged the clinicopathologic parameters (such as gender, age, stage, T stage, and N stage) with the risk model. We subsequently applied ROC curves to determine the nomogram’s dependability in forecasting 1-, 2-, and 3-year survival.
Immunohistochemistry (IHC)
There were sixteen pairings of COAD tissues with nearby non-cancerous tissues obtained from the patients in the Harbin Medical University Cancer Hospital after tumor resection and histological confirmation. The information and dilution concentrations of all primary antibodies applied to research are shown in Table S1. This study was conducted in accordance with the Declaration of Helsinki (as revised in 2013). The Harbin Medical University Cancer Hospital’s Ethics Committee has authorized this study (Ethics Application No. KY2023-58). Each study participant participating in IHC gave their informed consent. For this paper to be published, the patients have given written informed consents. Paraffin-embedded sections (4 µm) were prepared following standard protocol. In short, we blocked the endogenous peroxidase activity first, then we recovered the antigen, incubated the primary and secondary antibodies, and lastly dyed the material. 3% hydrogen peroxide, citrate buffer, 5% bovine serum albumin, primary and secondary antibodies, and a diaminobenzidine (DAB) kit were the reagents needed for this procedure. The directions for using these reagents were followed. The IHC staining score for each sample was equal to the product of the Positive Staining Cell Score and the Staining Intensity Score, where Staining intensity ranged from 0–3 and positive Staining Cell Score: 1 portrays 0–25%; 2 portrays 26–50%; 3 portrays 51–75%; and 4 portrays 76–100%. Staining intensity ranges from 0-3.
Functional enrichment analysis
The whole patients cohort was split into two risk statuses by the genetic model. With a false discovery rate (FDR) of less than 0.05 and the criteria |log2 (fold change)| >0.5, we employed the R package “limma” to research potential changes in the functions where the differentially expressed genes (DEGs) were situated among these two subsets. These DEGs were then undergone the Kyoto Encyclopedia of Genes and Genomes (KEGG) and Gene Ontology (GO) enrichment analyses through the “clusterProfiler” and “org.Hs.eg.db” packages in R.
Gene set enrichment analysis (GSEA)
GSEA was carried out using the R packages “cluster Profiler” and “org.Hs.eg.db” to examine whether the identified gene sets were statistically different in the two groups. Reference sets for the present research were the gene sets of c2.cp.kegg.v2023.1.Hs.symbols.gmt [Hallmarks] obtained in the Molecular Signatures Database (MSigDB, http://software.broadinstitute.org/gsea/msigdb/index.jsp). The following criteria were used to filter the enriched pathways: normalized enrichment score (|NES|) >1.0 and a notional P value of 0.05.
Calculation of immune and stromal scores and immune infiltration analysis
It is widely known that stromal and immune cells are essential for forming the tumor immune microenvironment (TIME). In order to further seek the worth of our genetic model when guiding risk stratification, we used the estimation of stromal and Immune cells in malignant tumor tissues using expression data (ESTIMATE) in R to examine differences in stromal and immune scores among patients with different risk statuses. Meanwhile, single-sample gene set enrichment analysis (ssGSEA) via the R package “GSVA” was employed to identify variances in immune cells and immune function amongst various risk status groups and the results were performed multiple testing.
Mutation status analysis
Mutation data were extracted from the TCGA. We analyzed variations in mutation status among subgroups of various risk statuses in the entire cohort using the R package “map tools”. After calculating the tumor mutation burden (TMB) in different risk status groups, in order to compare whether different TMBs and risk statuses have an effect on patients’ OS, we executed KM survival analysis using the R software packages “survival” and “survminer”.
Drug sensitivity analysis and tumor immune dysfunction and exclusion (TIDE) analysis
The TIDE was employed to model distinguishable tumor immune evasion processes (15). The likelihood of immunological escape of the tumor cells exhibits a positive connection with the TIDE score. Thus, a lower TIDE score indicates a better sensitivity to immune checkpoint inhibitor (ICI) therapy. Given that this is a relatively new form of cancer therapy and that chemotherapy is still one of the most valid therapeutic methods to treat COAD, we carried out a drug sensitivity measure to predict the chemotherapeutic response for every patient. Applying the R package “oncoPredict” to data from the free pharmacogenomic database Genomics of Drug Sensitivity in Cancer (GDSC, https://www.cancerrx gene.org/), half-maximal inhibitory concentration (IC50) measurements were employed to predict drug sensitivity. If the IC50 is higher, the antitumor impact is lesser (16,17).
Statistical analysis
All the statistical analyses were performed using R software (version 4.1.2). P values of less than 0.05 were acceptable and demonstrated statistical significance for differences.
Results
Constructing a new tool: a prognostic model for COAD consisting of seven ZNF genes
The flowchart of the procedure for this research is displayed in Figure 1. To figure out the ZNF protein genes that affect prognosis, we employed univariate Cox regression analysis on the TCGA-COAD set. We identified 29 distinctly prognosis-associated genes (all P<0.01; Table S2). Genes (17/29) with a hazard ratio (HR) value >1 were considered risk factors for colorectal cancer, whereas those (12/29 genes) with HR value <1 were considered to be protective factors against COAD. Furthermore, we used a heatmap to contrast the expression of these 29 genes in tumor versus normal colon tissue (Figure 2A). Then, to eliminate overfitting, we ran a LASSO regression analysis in the training cohort (Figure 2B; Figure S1). The sample scores can be determined through the regression coefficients obtained from the multivariate Cox regression analysis in the training cohort. Ultimately, seven genes, consisting of INSM1, PHF21B, RNF138, SYTL4, WRNIP1, ZNF514, and ZNF585B, comprised the model (Figure 2C), and Table S3 presents the values of coefficients for these genes. The formula mentioned in the methodology was applied by us to determine the risk score for each patient in the training cohort. The counted risk scores of the patients were ranked from low to high and then the middle value was chosen as the threshold to label the patients as high or low risk, respectively.
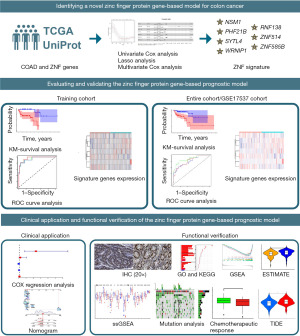
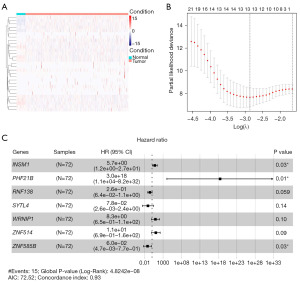
Individual patient risk scores ranged from low to high is illustrated in Figure 3A. Furthermore, the survival status plot revealed that, the percentage of survival was significantly lower in high-risk patients than that in low-risk patients (Figure 3B). The KM survival analysis of OS revealed that patients in the low-risk subset had a significantly greater survival probability than patients in the high-risk subset (Figure 3C, P<0.001). The time-dependent ROC curves also provide further evidence of the reliability of the gene model through the area under the curve (AUC) (0.943 for 1 year, 0.939 for 2 years, and 0.960 for 3 years) (Figure 3D). The heatmap (Figure 3E) displays the expression values of model genes.
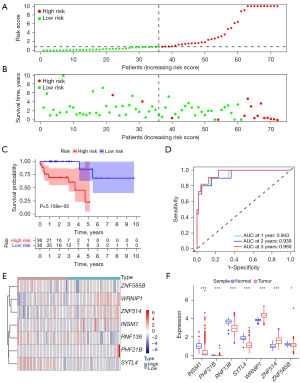
Combining theory with practice: analyzing and validating ZNF model genes expression
The results of our differential expression analysis of structural genes in the TCGA cohort revealed that WRNIP1, PHF21B, and ZNF514 were caught to be more abundant in carcinoma tissues than cancerous adjacent normal tissues, while INSM1, RNF138, SYTL4, and ZNF585B were opposite (Figure 3F). Since the bioinformatics analysis was based on transcriptomic data, we used IHC to confirm the protein expression of ZNF genes in 16 couples of COAD and near-healthy tissues. IHC staining showed that compared to paired adjacent normal tissues, INSM1 (Figure 4A), RNF138 (Figure 4B), and SYTL4 (Figure 4C) staining was stronger in adjacent cancer tissues, while WRNIP1 (Figure 4D) and ZNF514 (Figure 4E) staining was stronger in COAD tissues. The distinction in PHF21B staining between the two groups was not statistically meaningful (Figure 4F). These results indicated that WRNIP1 and ZNF514 proteins were significantly overexpressed in COAD, while INSM1, RNF138, and SYTL4 were significantly downregulated. Figure S2 shows that the IHC scores of INSM1, RNF138, and SYTL4 were higher in normal tissues, while WRNIP1 and ZNF514 were the opposite. The TCGA cohort survival analysis testified the clinical survival possibility between the high- and low-expression group of these genes (Figure S3). The results indicated that the survival possibility was worse in the RNF138 (Figure S3C) and ZNF585B (Figure S3G) decreased expression subset compared to the high expression subset, in contrast, the chances of survival were widely more in the decreased expression subset of WRNIP1 (Figure S3E) and ZNF514 (Figure S3F) compared to the rich expression subset. Unfortunately, there were no differences of survival possibility between high- and low-expression groups of INSM1 (Figure S3A), PHF2B (Figure S3B), and SYTL4 (Figure S3D).
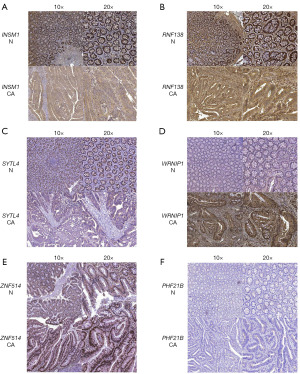
Using new tools: validating seven ZNF genes model in the entire cohort and GSE17537
Samples from the entire cohort and an external cohort (GSE17537) were utilized as testing data to check the efficacy of the predictive gene model. OS for both cohorts was used to gauge the success of the capability of our model. Applying the same formula as the training subset, we computed risk scores for 143 COAD patients in the entire set. Again, adopting the middle value as the threshold, patients were sorted into either the subsets with high-risk (n=72) or low-risk (n=71). This process was repeated with 55 patients from the external set: high-risk (n=27) and low-risk (n=28) subsets. Results were shown in Figure 5. The ranking of risk scores of patients in low-risk status to those in high-risk status for the entire cohort and for the GSE17537 cohort is presented in Figure 5A,5D. Figure 5B and Figure 5E show that for both the entire and GSE17537 (GEO) cohorts, the number of deaths in the low-risk subsets was trivial compared to the high-risk subsets. The heat maps of the gene expression value of our signature are exhibited in Figure 5C and Figure 5F. Furthermore, patients in the high-risk subsets were less likely to live than patients in the low-risk subsets in the entire cohort (P=9.221e−05, Figure 5G), as evidenced in the GSE17537 cohort (P=0.03, Figure 5H). With respect to the entire cohort, the AUCs of the genetic model for OS prediction demonstrated considerable reliability: 0.877 (1 year), 0.802 (2 years), and 0.813 (3 years), individually, with the GEO cohort following closely behind: 0.625 (1 year), 0.656 (2 years), and 0.656 (3 years) (Figure 5I,5J).
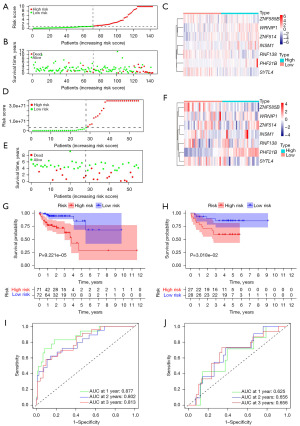
Focus on model and clinics: independence of the model and nomogram in COAD
The question of whether genetic models can be used as independent predictors of prognosis was tested by univariate and multivariate Cox regression analyses. Figure 6A depicts the result: risk score and staging were elements that were considerably linked to the prognosis. The product of the multivariate analyses suggested that the model could play as a self-contained prognostic implement (Figure 6B). As a result, our genetic model possesses some reliability for guiding clinical patient stratification. To improve clinical utility, we used available data to create a nomogram to forecast survival of 1-, 2-, and 3- year for COAD patients. We also used ROC curves to judge the rigor of this nomogram’s robustness. The total score obtained from the patient information was used to predict the patient’s probable OS (Figure 6C), and the 1-, 2-, and 3-year AUCs of the ROC curves based on this predicted nomogram were 0.937, 0.902, and 0.952, in turn (Figure 6D).
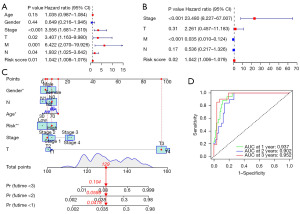
Going deeper: functional and GSEA
In our prior research, the genetic model was employed to split TCGA-COAD patients into two risk subsets: high and low. Following that, we investigated what gene sets and functional differences exist between the different subsets identified by GSEA, GO enrichment, and KEGG enrichment analysis. Firstly, DEGs were identified among the high-risk and low-risk subsets in the TCGA cohort for further investigation (table available at https://cdn.amegroups.cn/static/public/tcr-23-2158-1.xlsx), which included 686 genes (|logFC| >0.5, FDR <0.05). These results are displayed in a volcano plot (Figure 7A). whereas against the high-risk subset, 428 genes had reduced expression and 258 were elevated in the low-risk subset. Figure 7B draws a heatmap of the expression profile values of these 686 genes. According to the GO enrichment results, the DEGs identified were primarily involved with immune response: immune response−activating signal transduction; regulating cell surface receptor signaling pathway; positive regulation of cell activation in biological processes, activation of the immune response; immune response−activating cell surface receptor signaling pathway; and immune response activation. Regarding for molecular functions, DEGs were particularly strong in immune receptor activity, antigen binding, cytokine receptor binding, and immunoglobulin receptor binding. About cellular components, the enriched DEGs were most closely linked with the immunoglobulin complex, as well as the immunoglobulin complex, circulating and external side of the plasma membrane (Figure 7C, Figure S4). The DEGs were particularly rich in the chemokine signaling pathway, cell adhesion molecules, cytokine-cytokine receptor interaction, and neutrophil extracellular trap formation according to the KEGG enrichment analysis (Figure 7D; Figure S5). Finally, the JAK-STAT signaling pathway, the apoptosis, cytokine-cytokine receptor interaction, and chemokine signaling pathway had a crucial enrichment in the GSEA gene expression patterns (Figure S6).
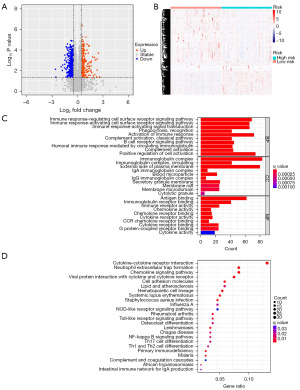
Combined immunity: differences in TIME and immune score analysis
The above analyses supported that the model was strongly influenced by immune function, prompting us to be interested in investigating into the discrepancy in immune cells, function, and the TIME among the opposite subsets. An ESTIMATE algorithm was constructed to assess whether our 7-gene signature can discriminate differences in TIME for two risk groups of COAD patients. The methodology could be carried out to count the score of tumor purity, stromal cells, and immune cells in the immune microenvironment, from which we can compare the gaps in the cellular components of the immune microenvironment among the two subsets. The stromal score ranged from −2,068.98 to 2,029.63 (Figure 8A). The immune score ranged from −819.85 to 3,003.12, the ESTIMATE score from −2,679.15 to 4,554.83, and the tumor purity from 0.292 to 0.978 (Figure 8B-8D, respectively). The immunity score, which represents the index of immune cell infiltration, was inferior in the immune microenvironment of the high-risk subset to that of the low-risk subset (Figure 8B, P<0.001, Figure 8C, P<0.01). This situation indicated a richer index of immune cell infiltration in patients in the low-risk subset. Given the differences in immune scores among the two subsets, we used ssGSEA to determine what strains of immune cells and functional discrepancies existed among the two subsets, and the findings revealed that the two groups differed in B-cells, T helper cells, natural killer (NK) tumor-infiltrating lymphocyte (TIL), CD8+ T cells, interdigitating dendritic cells (iDCs), neutrophils, Th2, Treg cells, follicle-helper T cells (Tfh), dendritic cells (DCs), and Th1 cells (Figure 8E, all P<0.05 in mentioned cells).
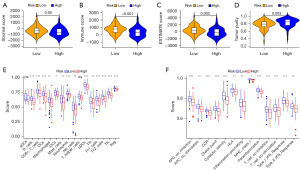
This result demonstrated that the infiltration of the mentioned cells was much greater in the low-risk subset than in the high-risk subset. Surprisingly, the differences in immune function among the two subsets were consistent, with greater scores in the low-risk subset for chemokine receptor (CCR), cytolytic activity, type I interferon (IFN) response, check-point, type II IFN response, T-cell co-stimulation, inflammation-promotion, human leukocyte antigen (HLA), and T cell co-inhibition (all P<0.01 in mentioned functions, Figure 8F). Furthermore, antigen-presenting cell (APC) co-inhibition and APC synergistic stimulation, two molecular processes closely linked to antigen presentation, were decreased in the high-risk subset.
Treatment-oriented: mutation landscape, analysis of drug sensitivity, and TIDE analysis
It is well established that somatic mutation is one of the essential characteristics of carcinogenesis (18). We used mutation data from TCGA to compare gene mutation landscapes between the two risk patients. As shown in Figure 9A,9B, the overall mutation rate for high-risk patients was greater than that of low-risk patients (98.51% vs. 95.24%). With respect to gene mutation frequency of specific genes, 75%, 43%, and 36% of the high-risk subset had APC, TTN, and KRAS mutations, respectively, compared with 83%, 52%, and 44% of low-risk score patients. Furthermore, the tumor suppressor TP53 mutated with greater frequency in the high-risk subset (64% vs. 52%). The oncogene MUC16, on the other hand, had a lower mutation frequency in the group with a higher risk of cancer (19% vs. 32%). The mutational frequency of BRAF and PIK3CA was more in the high-risk group than that in the low-risk group (9% vs. 8%, 27% vs. 24%). These results indicated that the possibility of progress in the high-risk patients is more than that in the low-risk patients, so that the high-risk patients have a worse survival time and prognosis. Using the results of our TMB calculations for each individual in the entire cohort, patients were classified into high-TMB and low-TMB subsets, with the median value of all TMB serving as a threshold. The subsequent KM survival analyses proved that patients with high (H)-TMB had more odds of survival than patients with low (L)-TMB (Figure 9C). This prompted us to wonder if there are discrepancies in survival probability when patients stay in different risk statuses and TMB. To that end, we divided the entire cohort into four groups: high-risk/H-TMB, low-risk/H-TMB, high-risk/L-TMB, and low-risk/L-TMB, and then used KM survival analysis to compare the discrepancies among these subsets, and the findings revealed that patients in the low-risk subset with a high TMB load depicted much greater chance of survival than those in the other groups (Figure 9D). However, the efficacy of colorectal cancer chemotherapy and immunotherapy is still an important concern. We employed TIDE analyses to anticipate the discrepancies in immunotherapy among the two subsets. The TIDE score, which represents the possibility of immune dysfunction and rejection, was greater in the high-risk subset than in the low-risk subset. This suggests that high-risk patients are more inclined to experience tumor immune dysfunction and rejection, resulting in poor immunotherapy outcomes (Figure 9E).
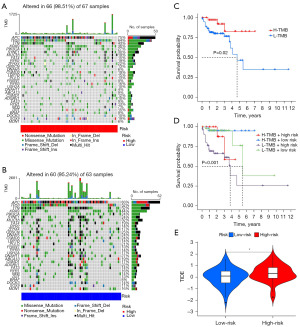
Finally, we examined the drug sensitivity (as determined by “oncoPredict”) of all patients in each of the two risk groups to the 25 various medications administered (table available at https://cdn.amegroups.cn/static/public/tcr-23-2158-2.xlsx). With regard to the IC50 values of the 25 drugs, the outcomes testified notable discrepancies between the two subsets (P<0.05 for all) (Figure 10). Patients with high-risk status had better sensitivity to Linsitinib (P=0.001, Figure 10A), Navitoclax (P=0.003, Figure 10B), OSI-027 (P=0.01, Figure 10C), Vorinostat (P=0.04, Figure 10D) and WEHI-539 (P=0.01, Figure 10E). In contrast, patients with low-risk status had better sensitivity to the other 20 drugs. This testified that low-risk patients may be more responsive to the requirements of chemotherapeutics.
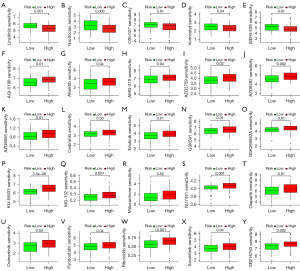
Discussion
The most frequent kind of colon cancer is COAD, and its morbidity and mortality remain high. The lack of effective biomarkers restricts clinical prognosis evaluation and individualized treatment. As sequencing technology advances and public databases such as TCGA and GEO become more accessible, it has enabled researchers to evaluate the role of biomarkers in clinical practice from a more comprehensive perspective. Compared to single-gene biomarkers, gene signatures have taken a step forward in patient risk stratification and individualized treatment guidance (19,20).
ZNF proteins are intimately involved in the transcription of the human genome. Accumulating evidence has indicated the important role of ZNF protein genes in various aspects of cancer biology (21). It is not surprising that the alternations of ZNF protein genes can affect cancer development and vice versa. Indeed, recent studies have reported the model consisting of ZNF protein genes in certain tumors. The expression signature of ZNF factors linked to cancer that was discovered in TCGA pan-cancer transcriptome data was initially reported by Machnik et al. (22) Then, prognostic assessment models based on their expression signatures were established in bladder cancer, breast cancer, osteosarcoma, and esophageal carcinoma (11-14). These studies provided a large amount of integrated clinical information, suggesting their potential prognostic application values.
Inspired by the above studies, we investigated the prognostic and therapeutic value of ZNF protein genes in COAD and built a novel model containing seven ZNF protein genes for COAD. The 7-gene model assigns COAD patients to groups with different risk statuses. The KM analysis confirmed that patients in the low-risk subset had a greater probability of survival than patients in the high-risk subset. The reliability of the analysis was evaluated by a time-dependent ROC curve analysis, which resulted in a high degree of confidence in the ZNF protein gene model to forecast the survival of patients. Moreover, except for the absence of commercial antibodies for ZNF585B, the IHC results depicted that at the protein level, the expression patterns of six genes (INSM1, RNF138, SYTL4, WRNIP1, PHF21B, and ZNF514) were consistent with the transcriptional data. IHC had trouble detecting PHF21B because of its slight expression in the tumor and surrounding tissues, which may be caused by low transcriptional expression (Figure 3F).
TIME profoundly affects the initiation, progression, and drug treatment response of tumors. We discovered that DEGs were primarily enriched in biological processes connected to the immune system in accordance with the findings of GO and KEGG analysis. Patients in the low-risk subset showed greater immune cell infiltration via ESTIMATE and ssGSEA than the high-risk patients and the two subsets exhibited substantial distinctions in related immune functions. Indeed, the result of TIDE analysis suggested that patients in the high-risk subset had an elevated risk of developing tumor immunological dysfunction than those with low-risk status. We confirmed that the immune status of patients in the high-risk status group may have been suppressed, and can postulate that they may have mild reactions to ICIs. Finally, the low-risk/H-TMB subgroup of patients had significantly better survival probability than the other 3 sub-groups. This agrees with the findings of previous research and is believed to be because the more novel epitopes generated (increased in H-TMB), the more likely one will be recognized as an immunogenic antigen and trigger a T-cell attack (23). Collectively, these findings implied that there may be a relationship between our ZNF gene signature and the TIME of COAD.
The model in our research consists of INSM1, PHF21B, RNF138, SYTL4, WRNIP1, ZNF585B, and ZNF514. INSM1 is mainly expressed during the development of mammalian neuroendocrine tissues and nervous systems (24). It can directly suppress the cell cycle’s advancement and promote cell differentiation (25,26). In addition, the prospective biomarker and treatable position for neuroendocrine carcinomas may be INSM1 (27). PHF21B, an individual belonging to the PHD ZNF superfamily, participates in tumorigenesis and the development of multiple types of tumors (28,29). Li et al. found that as an oncogene, PHF21B represents an excellent candidate prognostic biomarker in prostate cancer (30). In addition, another study showed that overexpression of PHF21B, as a gene that suppresses tumors, can reduce cell migration and colony formation (31). RNF138 is an E3 ligase (32-34), which can confer cisplatin resistance on gastric cancer cells by weakening the cell cycle arrest and Chk1-mediated apoptosis induced by cisplatin (35). It is interesting that the incidence of colorectal cancer tumors is directly associated with the decreased levels of RNF138, and RNF138 can inhibit the transformation of chronic colitis into colon tumors (36). SYTL4, as a Rab27 effector (37,38), has been testified to be a suitable prognostic indicator in breast cancer patients whose cancer kind is triple-negative breast cancer receiving taxane treatment (39). According to reports, WRNIP1 can significantly affect the restoration of DNA damage and the preservation of genomic stability (40). According to Jiang et al., miR-22 may decrease WRNIP1 expression, improving the reactivity to radiation in small-cell lung cancer, which suggests that WRNIP1 exists as a risk element for lung cancer patients with non-small cell (41). In contrast, there is little research on ZNF585B and ZNF514 so further research is needed regarding their roles in tumors.
Our research had examined the features of ZNF genes as prognostic predictive biomarkers for COAD. Nevertheless, we also have certain limitations with our research. First, the present study is a study utilizing public databases. Although we conducted IHC experiments, the sample size is small. Secondly, this study lacks basic experiments to verify the function of the seven ZNF genes in COAD, especially in the signaling pathways closely linked to the present study. Thirdly, the treatment of colon cancer is largely associated with the mutational status of KRAS, BRAF, and PIK3CA and microsatellite/mismatch repair status. As a result, the potential mechanism or combination for ZNF protein genes signature with these mutational genes and status needs to be further explored.
Conclusions
We employed seven ZNF protein genes to construct a reliable prognostic model of COAD. This model enables doctors to classify patients into either high- or low-risk subset with different survival probabilities. We believe our model may help elucidate the role of ZNF protein genes and have the potential to be clinically useful prognostic biomarkers for COAD patients.
Acknowledgments
We sincerely acknowledge the publicly available databases, including The Cancer Genome Atlas (TCGA) database (https://portal.gdc.cancer.gov/), Gene Expression Omnibus (GEO) database (https://www.ncbi.nlm.nih.gov/geo/) and UniProt database (https://www.uniprot.org/).
Funding: This project was supported by
Footnote
Reporting Checklist: The authors have completed the TRIPOD reporting checklist. Available at https://tcr.amegroups.com/article/view/10.21037/tcr-23-2158/rc
Data Sharing Statement: Available at https://tcr.amegroups.com/article/view/10.21037/tcr-23-2158/dss
Peer Review File: Available at https://tcr.amegroups.com/article/view/10.21037/tcr-23-2158/prf
Conflicts of Interest: All authors have completed the ICMJE uniform disclosure form (available at https://tcr.amegroups.com/article/view/10.21037/tcr-23-2158/coif). The authors have no conflicts of interest to declare.
Ethical Statement: The authors are accountable for all aspects of the work in ensuring that questions related to the accuracy or integrity of any part of the work are appropriately investigated and resolved. The research was carried out in compliance with the Declaration of Helsinki (as revised in 2013). The Harbin Medical University Cancer Hospital’s Ethics Committee has authorized this study (Ethics Application No. KY2023-58). Each study participant participating in IHC gave their informed consent. For this paper to be published, the patients have given written informed consent.
Open Access Statement: This is an Open Access article distributed in accordance with the Creative Commons Attribution-NonCommercial-NoDerivs 4.0 International License (CC BY-NC-ND 4.0), which permits the non-commercial replication and distribution of the article with the strict proviso that no changes or edits are made and the original work is properly cited (including links to both the formal publication through the relevant DOI and the license). See: https://creativecommons.org/licenses/by-nc-nd/4.0/.
References
- Siegel RL, Miller KD, Wagle NS, et al. Cancer statistics, 2023. CA Cancer J Clin 2023;73:17-48. [Crossref] [PubMed]
- Siegel RL, Wagle NS, Cercek A, et al. Colorectal cancer statistics, 2023. CA Cancer J Clin 2023;73:233-54. [Crossref] [PubMed]
- Spanos CP. Colon cancer. In: Digestive System Malignancies. Academic Press; 2022:67-71.
- Ogunwobi OO, Mahmood F, Akingboye A. Biomarkers in Colorectal Cancer: Current Research and Future Prospects. Int J Mol Sci 2020;21:5311. [Crossref] [PubMed]
- Jen J, Wang YC. Zinc finger proteins in cancer progression. J Biomed Sci 2016;23:53. [Crossref] [PubMed]
- Beerli RR, Barbas CF 3rd. Engineering polydactyl zinc-finger transcription factors. Nat Biotechnol 2002;20:135-41. [Crossref] [PubMed]
- Liu S, Sima X, Liu X, et al. Zinc Finger Proteins: Functions and Mechanisms in Colon Cancer. Cancers (Basel) 2022;14:5242. [Crossref] [PubMed]
- Yang L, Zhang L, Wu Q, et al. Unbiased screening for transcriptional targets of ZKSCAN3 identifies integrin beta 4 and vascular endothelial growth factor as downstream targets. J Biol Chem 2008;283:35295-304. [Crossref] [PubMed]
- Li J, Jiang JL, Chen YM, et al. KLF2 inhibits colorectal cancer progression and metastasis by inducing ferroptosis via the PI3K/AKT signaling pathway. J Pathol Clin Res 2023;9:423-35. [Crossref] [PubMed]
- Hahn S, Jackstadt R, Siemens H, et al. SNAIL and miR-34a feed-forward regulation of ZNF281/ZBP99 promotes epithelial-mesenchymal transition. EMBO J 2013;32:3079-95. [Crossref] [PubMed]
- Hong K, Yang Q, Yin H, et al. Comprehensive analysis of ZNF family genes in prognosis, immunity, and treatment of esophageal cancer. BMC Cancer 2023;23:301. [Crossref] [PubMed]
- Sun X, Zheng D, Guo W. Comprehensive Analysis of a Zinc Finger Protein Gene-Based Signature with Regard to Prognosis and Tumor Immune Microenvironment in Osteosarcoma. Front Genet 2022;13:835014. [Crossref] [PubMed]
- Ye M, Li L, Liu D, et al. Identification and validation of a novel zinc finger protein-related gene-based prognostic model for breast cancer. PeerJ 2021;9:e12276. [Crossref] [PubMed]
- Zhang J, Zhang C, Cao P, et al. A zinc finger protein gene signature enables bladder cancer treatment stratification. Aging (Albany NY) 2021;13:13023-38. [Crossref] [PubMed]
- Jiang P, Gu S, Pan D, et al. Signatures of T cell dysfunction and exclusion predict cancer immunotherapy response. Nat Med 2018;24:1550-8. [Crossref] [PubMed]
- Maeser D, Gruener RF, Huang RS. oncoPredict: an R package for predicting in vivo or cancer patient drug response and biomarkers from cell line screening data. Brief Bioinform 2021;22:bbab260. [Crossref] [PubMed]
- Iorio F, Knijnenburg TA, Vis DJ, et al. A Landscape of Pharmacogenomic Interactions in Cancer. Cell 2016;166:740-54. [Crossref] [PubMed]
- Comprehensive molecular characterization of human colon and rectal cancer. Nature 2012;487:330-7. [Crossref] [PubMed]
- Nguyen LC, Naulaerts S, Bruna A, et al. Predicting Cancer Drug Response In Vivo by Learning an Optimal Feature Selection of Tumour Molecular Profiles. Biomedicines 2021;9:1319. [Crossref] [PubMed]
- Ma J, Mi C, Wang KS, et al. Zinc finger protein 91 (ZFP91) activates HIF-1α via NF-κB/p65 to promote proliferation and tumorigenesis of colon cancer. Oncotarget 2016;7:36551-62. [Crossref] [PubMed]
- Zhao J, Wen D, Zhang S, et al. The role of zinc finger proteins in malignant tumors. FASEB J 2023;37:e23157. [Crossref] [PubMed]
- Machnik M, Cylwa R, Kiełczewski K, et al. The expression signature of cancer-associated KRAB-ZNF factors identified in TCGA pan-cancer transcriptomic data. Mol Oncol 2019;13:701-24. [Crossref] [PubMed]
- Addeo A, Friedlaender A, Banna GL, et al. TMB or not TMB as a biomarker: That is the question. Crit Rev Oncol Hematol 2021;163:103374. [Crossref] [PubMed]
- Mahalakshmi B, Baskaran R, Shanmugavadivu M, et al. Insulinoma-associated protein 1 (INSM1): a potential biomarker and therapeutic target for neuroendocrine tumors. Cell Oncol (Dordr) 2020;43:367-76. [Crossref] [PubMed]
- Osipovich AB, Long Q, Manduchi E, et al. Insm1 promotes endocrine cell differentiation by modulating the expression of a network of genes that includes Neurog3 and Ripply3. Development 2014;141:2939-49. [Crossref] [PubMed]
- Zhang T, Liu WD, Saunee NA, et al. Zinc finger transcription factor INSM1 interrupts cyclin D1 and CDK4 binding and induces cell cycle arrest. J Biol Chem 2009;284:5574-81. [Crossref] [PubMed]
- Roy M, Buehler DG, Zhang R, et al. Expression of Insulinoma-Associated Protein 1 (INSM1) and Orthopedia Homeobox (OTP) in Tumors with Neuroendocrine Differentiation at Rare Sites. Endocr Pathol 2019;30:35-42. [Crossref] [PubMed]
- Björkman M, Östling P, Härmä V, et al. Systematic knockdown of epigenetic enzymes identifies a novel histone demethylase PHF8 overexpressed in prostate cancer with an impact on cell proliferation, migration and invasion. Oncogene 2012;31:3444-56. [Crossref] [PubMed]
- Wei M, Liu B, Su L, et al. A novel plant homeodomain finger 10-mediated antiapoptotic mechanism involving repression of caspase-3 in gastric cancer cells. Mol Cancer Ther 2010;9:1764-74. [Crossref] [PubMed]
- Li Q, Ye L, Guo W, et al. PHF21B overexpression promotes cancer stem cell-like traits in prostate cancer cells by activating the Wnt/β-catenin signaling pathway. J Exp Clin Cancer Res 2017;36:85. [Crossref] [PubMed]
- Bertonha FB, Barros Filho Mde C, Kuasne H, et al. PHF21B as a candidate tumor suppressor gene in head and neck squamous cell carcinomas. Mol Oncol 2015;9:450-62. [Crossref] [PubMed]
- Zhou YX, Chen SS, Wu TF, et al. A novel gene RNF138 expressed in human gliomas and its function in the glioma cell line U251. Anal Cell Pathol (Amst) 2012;35:167-78. [Crossref] [PubMed]
- Matsuoka S, Ballif BA, Smogorzewska A, et al. ATM and ATR substrate analysis reveals extensive protein networks responsive to DNA damage. Science 2007;316:1160-6. [Crossref] [PubMed]
- Yamada M, Ohnishi J, Ohkawara B, et al. NARF, an nemo-like kinase (NLK)-associated ring finger protein regulates the ubiquitylation and degradation of T cell factor/lymphoid enhancer factor (TCF/LEF). J Biol Chem 2006;281:20749-60. [Crossref] [PubMed]
- Lu Y, Han D, Liu W, et al. RNF138 confers cisplatin resistance in gastric cancer cells via activating Chk1 signaling pathway. Cancer Biol Ther 2018;19:1128-38. [Crossref] [PubMed]
- Lu Y, Huang R, Ying J, et al. RING finger 138 deregulation distorts NF-кB signaling and facilities colitis switch to aggressive malignancy. Signal Transduct Target Ther 2022;7:185. [Crossref] [PubMed]
- Fukuda M. Rab27 effectors, pleiotropic regulators in secretory pathways. Traffic 2013;14:949-63. [Crossref] [PubMed]
- Fukuda M. Slp4-a/granuphilin-a inhibits dense-core vesicle exocytosis through interaction with the GDP-bound form of Rab27A in PC12 cells. J Biol Chem 2003;278:15390-6. [Crossref] [PubMed]
- Liu XY, Jiang W, Ma D, et al. SYTL4 downregulates microtubule stability and confers paclitaxel resistance in triple-negative breast cancer. Theranostics 2020;10:10940-56. [Crossref] [PubMed]
- Leuzzi G, Marabitti V, Pichierri P, et al. WRNIP1 protects stalled forks from degradation and promotes fork restart after replication stress. EMBO J 2016;35:1437-51. [Crossref] [PubMed]
- Jiang W, Han X, Wang J, et al. miR-22 enhances the radiosensitivity of small-cell lung cancer by targeting the WRNIP1. J Cell Biochem 2019;120:17650-61. [Crossref] [PubMed]