By integrating single-cell RNA sequencing and bulk RNA sequencing, plasma cells signature and tertiary lymphoid structures were verified to contribute to outcome in lung adenocarcinoma
Highlight box
Key findings
• Our study combined single-cell analysis and bulk RNA sequencing (RNA-seq) to uncover the dynamic changes of plasma cells during lung adenocarcinoma (LUAD) progression. Additionally, tissue section analysis revealed a strong correlation between tertiary lymphoid structures (TLS) and patient prognosis.
What is known and what is new?
• TLS are ectopic lymphoid tissue that form within non-lymphoid tissue and have been demonstrated present within all stages of human cancer. B cells eliminate tumor cells by producing antibodies, and among the various B cell subsets, plasma cells play a major role.
• Through single-cell and bulk RNA-seq analyses, we found that both TLS and plasma cells have a significant impact on the prognosis of LUAD. As a crucial component of TLS, plasma cells increase in proportion with tumor progression.
What is the implication, and what should change now?
• We found a strong correlation between plasma cells and TLS, and the functional status of plasma cells might be a potential predictive biomarker for LUAD patients.
Introduction
According to the latest global cancer statistics, although the incidence and mortality of lung cancer (LC) have declined at a steady pace since 2006, it is still the main cause of cancer-specific mortality worldwide. With nearly 2.5 times more deaths caused by LC than colorectal cancer (CRC), the second leading cause of cancer death overall (1). Non-small cell LC (NSCLC) and small cell LC (SCLC) are the two main subtypes, with NSCLC occupying about 80% to 85% of all LC cases (2), of which, lung adenocarcinoma (LUAD) is the most common histological subtype. Recently, benefiting from much progress made in treatment and diagnosis for NSCLC, covering the earlier detection, surgical procedures, chemotherapy, radiation therapy, and stereotactic ablative radiotherapy, the survival rates for patients greatly improved. The 2-year relative survival for NSCLC was 42% in 2015 to 2016 compared with 34% in 2009 to 2010 (3). The 3-year relative survival increased from 25% during 2004 through 2006 to 38% during 2016 through 2018. The 5-year relative survival for LC was 23% in 2012 to 2018 compared with 12% in 1975 to 1977, and 15% in 1995 to 1997 (1).
The tumor microenvironment (TME), which consisted of tumor cells and the associated immune and stroma cells, has been noted and widely recognized for its significant role (4,5). The quantity and quality of tumor-infiltrating lymphocytes (TILs) in the TME reflected the therapeutic efficiencies (6). The composition of TILs however varies dynamically, which regulates the tumorigenesis, local invasion, and distant metastasis (7). Until now, the anti-tumor immune responses were proved to be achieved mainly through the T cell compartment (8). However, other immune subsets may also count, although in immune checkpoint blockade (ICB) treatment this was barely mentioned (6,9,10). For example, a phase II study of neoadjuvant ICB in patients with high-risk resectable melanoma revealed that B cell markers were enriched in the responders to treatment versus the non-responders (11). The primary function of B cells is to generate antibodies that eliminate cancer cells by driving cytotoxicity and phagocytosis of antibody-dependent cells (12,13). Despite that, B cells could present antigen to T cells, activating CD4+ T helper (Th) cells to promote an immune response (14).
Tertiary lymphoid structures (TLS), which exist around chronic inflammatory sites in diseases such as tumors, infectious diseases, autoimmune diseases, and organ transplantation, are ectopic lymphoid tissue that form within non-lymphoid tissue (15). Similar to secondary lymphoid organs (SLOs), such as lymph nodes, mature TLS consist of T cell zones, B cell follicles, and germinal centers (GCs) (7). TLS are able to generate adaptive immune responses in situ, and have been demonstrated present within all stages of human cancer, in primary as well as metastatic lesions (7). The presence of TLS in autoimmune disorders is thought to be associated with a negative prognosis (16). While in the TME, TLS create a supportive niche for immune cells, strengthening the immune response against cancer and generally leading to better outcomes in most cancers, including breast, colorectal, and LCs (17). Due to its benefits for anti-tumor immunity, researchers are also beginning to focus more on TLS. We usually use the following methods to observe TLS: through immunohistochemistry or immunofluorescence staining of specific markers, using hematoxylin and eosin (H&E) staining to identify TLS by recognizing dense aggregates of lymphoid tissues, and utilizing spatial transcriptomics technology.
The overall functional role of tumor-infiltrating B cells was incompletely acknowledged. Recent studies have demonstrated that, when associated with TLS, tumor-infiltrating B cells could respond better to immunotherapy and improve cancer outcomes (18-21). To better identify B cells in the TME, we used single-cell analysis. Recently, single-cell analysis, as an emerging technology, has been used to study the transcriptomes of different cell types (22). It utilizes optimized next-generation sequencing to define the global gene expression profile of individual cells, facilitating a more precise identification of the cellular composition within tissues and has significantly advanced research on the TME (23). In comparison, bulk RNA-seq can often include larger sample sizes, making it suitable for large-scale analyses and differential gene screening. Therefore, based on the respective advantages of each method, many studies have attempted to integrate single-cell RNA sequencing (scRNA-seq) with traditional RNA-seq to identify novel biomarkers for malignant tumors (22,23). Herein, by using published scRNA-seq data, we systematically mapped the landscape of TME in LUAD from early to advanced stages, including several subtypes of tumor-infiltrating B cells. We found a strong correlation between B cells and TLS, and the functional status of plasma cells might be a potential predictive biomarker for LUAD patients. We present this article in accordance with the TRIPOD reporting checklist (available at https://tcr.amegroups.com/article/view/10.21037/tcr-24-1746/rc).
Methods
Data acquisition
In this study, the LUAD scRNA-seq GSE131907 dataset and anti-PD-1 treatment GSE126044 dataset were downloaded and analyzed from the Gene Expression Omnibus (GEO) database (https://www.ncbi.nlm.nih.gov/geo/). The GSE131907 dataset contains 58 samples, in which we divided the primary tumors into the early and advanced groups. The training cohort comprised LUAD RNA-seq data, corresponding clinical information and HE-stained pathological sections were obtained from The Cancer Genome Atlas (TCGA) database (https://portal.gdc.cancer.gov/). The GSE30219 dataset was extracted for validation using the GPL570 platform. All data were converted to the TPM format for further analyses to ensure data comparability.
Tissue processing
Tumor tissues were collected from stage I–III LUAD patients who underwent primary surgical resection in The First Affiliated Hospital of Nanjing Medical University. The specimens were immediately immersed in formalin in the operating room and embedded in paraffin as soon as possible following surgery. The study was conducted in accordance with the Declaration of Helsinki (as revised in 2013). The study was approved by the Ethical Committee of The First Affiliated Hospital of Nanjing Medical University (2023-SR-777) and all patients provided written informed consent.
scRNA-seq data processing and cell annotation
Based on “seurat” R tools, we checked the accuracy of the scRNA-seq data. Those genes that expressed in at least three single cells, and cells containing between 200 and 7,000 genes were reserved. Cells with more than 10% mitochondrial genes were filtered out to preserve high-quality scRNA-seq data. Then, via the “FindVariableFeatures” program and principal component analysis (PCA), top 3,000 variable genes and 20 principal components were screened for dimensionality reduction to find the meaningful clusters. The batch effects among patients were eliminated with the harmony package. Then the “FindAllMarkers” tool was taken to identify the differentially expressed genes (DEGs) in each cluster with the cutoff thresholds of adjusted (adj.) P<0.05 and log2(fold change) >0.25. Based on these marker genes, cell types were meticulously recognized after annotation in accordance with the published findings (24,25). Finally, the B cells were selected for further investigation, and the results were visualized using the “ggplot2” R package.
Single-sample gene set enrichment analysis (ssGSEA) and microenvironment cell population (MCP)-counter analysis
To quantify the immune status in different-stage tumors, ssGSEA was utilized by analyzing the expression profiles of the 30 immune signature genesets. To emphasize the role of B cells in the response to ICB treatment, we applied the MCP-counter algorithm to evaluate the distribution of immune cells between the responder and non-responder groups.
Establishment of TME gene expression signatures
The “FindAllMarkers” program of seurat was applied to list the marker genes of each cell cluster. The unique genes representing the plasma population were selected as the marker genes to construct the signature, and summarized in table available at https://cdn.amegroups.cn/static/public/tcr-24-1746-1.xlsx. The other signature genes derived from reported literature were as follows: TLS (CCL19, CCL21, CXCL13, CCR7, CXCR5, SELL, and LAMP3) (18,26).
Differential gene expression analysis of TCGA and GEO data
To identify the prognostic genes of each cell type, we employed Cox regression analysis with the “survival” package to evaluate the correlations between the DEGs selected above and survival status respectively. To minimize the risk of omission, we set 0.05 as the cut-off P value. Then multivariate Cox regression analysis was performed to narrow down the candidate genes based on the lowest Akaike information criterion (AIC). Other signature genes of TLS, class I major histocompatibility complex (MHC), and class II MHC were derived from the published literature (27). The ssGSEA algorithm was used to calculate the enrichment scores representing the relative expression of specific cell types in each sample in both the TCGA-LUAD and GSE31210 datasets. The results were sorted according to TLS score and visualized by heatmap.
Construction and validation of the risk score
We quantified TLS using H&E staining images of the TCGA LUAD cohort. Tissues containing TLS were considered as “TLS-high” cases, while tissues with no TLS observed were considered as “TLS-low” cases. Differential gene analysis was performed between two groups using the “limma” package.
H&E staining
The prepared sections were successively placed into xylene for three times for 20 min, followed by anhydrous ethanol for two times for 5 min and 75% ethanol for 5 min. Hematoxylin dye solution (C0105S-1; Beyotime, Shanghai, China) was applied according to the instructions for 5 min. The differentiation solution was differentiated, and the blue solution was returned to blue. The sections were dehydrated with 85% gradient alcohol and 95% gradient alcohol for 5 min respectively, and then stained with Beyotime (C0105S-2) for 5 min. The slices were then dehydrated and transparent and then sealed with neutral gum. Observe and capture images.
Multiple immunofluorescence assay
The prepared sections were deparaffined by xylene for 10 min and repeated three times, soaked in 100%, 95%, 85%, and 70% ethanol for 5 min successively, then subjected to citric acid repair buffer pH 6.0 at 100 ℃ antigen retrieval for 20 min, then sealed with 3% hydrogen peroxide solution at room temperature for 15 min. Add 100 µL normal goat serum and incubate at room temperature for 30 min. Add 100 µL anti-CD3 (ab16669; Abcam, Cambridge, UK; 1:2,000)/anti-CD19 (AF20215; Aifang Biological, Changsha, China; 1:2,000)/anti-CD8 (AF20211; Aifang Biological; 1:2,000)/anti-CD20 (AB64088; Abcam; 1:3,000)/anti-CD138 (67155-1-AP; Proteintech, Rockford, IL, USA; 1:2,000), incubated overnight at 4 ℃. The next day, horseradish peroxidase (HRP)-polymer anti-rabbit/mouse immunohistochemical secondary antibody was used according to the instructions, incubated at room temperature for 30 min, and TYR-520 fluorescent dye reacted for 3–10 min. The antibody incubation steps were repeated and another TYR-570 fluorescent dye was used for staining until the target marker staining was realized one by one [TYR-520, TYR-570, TYR-620, TYR-690, TYR-780, and spectral 4',6-diamidino-2-phenylindole (DAPI)]. The DAPI dye solution was incubated for 10 min at room temperature in the dark, the anti-fluorescence quenching sealing tablets were sealed and the images were collected under a multi-channel fluorescence scanner.
Statistical analysis
All statistical analyses were performed using R language (version 4.4.1). Kaplan-Meier (KM) curves with a two-sided log-rank test were employed to compare the survival rates of the two groups. All survival curves were produced using the “survminer” package. Univariate and multivariate Cox regression analysis were applied to evaluate prognostic variables. In the correlation analysis, P values were calculated using the Pearson correlation test, and data with P values <0.05 were displayed. The R software “ggplot2” was used frequently to visualize the data. All statistical tests were two-tailed, and a P value <0.05 was considered significant.
Results
The scRNA-seq profiling of LUAD
The overall flow diagram is depicted in Figure 1. The scRNA-seq data of LUAD were downloaded from the GSE131907 dataset. According to the authors’ notes, we brought some of the samples into our study and divided them into early (LUNG_T06, LUNG_T08, LUNG_T09, LUNG_T18, LUNG_T19, LUNG_T20, LUNG_T25, LUNG_T30, LUNG_T34) and advanced (EBUS_06, EBUS_28, EBUS_49, BRONCHO_58, LUNG_T28, LUNG_T31) groups. After a quality check, a total of 53,771 cells were deemed suitable for future study (Figure S1). Within the 15 samples we picked, the cell distribution was relatively consistent and there were no discernible batch differences (Figure 2A,2B). Based on the results of PCA, we adopted the t-SNE methods to reduce the dimensionality of the sample into 17 clusters (Figure 2C). Bubble plots illustrated the typical marker genes for different cell types (Figure S2), which were later used for annotation. As a result, seven cell types were identified, such as B cells, endothelial cells, epithelial cells, fibroblasts, mast cells, myeloid cells, and natural killer (NK)/T cells (Figure 2D). Then, via the “ClusterGVis” package, the functional enrichment pathways for each cluster are shown in Figure 2E.
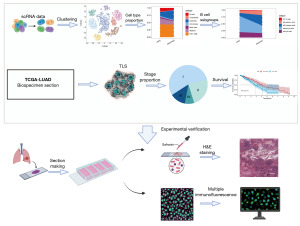
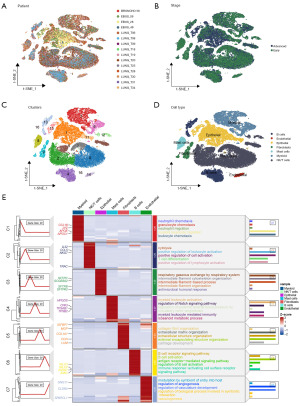
Distribution of infiltrated B cells in LUAD
Figure 3A shows the proportion of each cell type in the 15 samples with line plots. When we compared the differences between groups, it was found that the proportion of B cells was significantly reduced in the advanced group, while the NK/T cells showed no significant difference (Figure 3B). In order to compare the expression differences between the two main immune cells (T cells and B cells) in different LC stages, we classified the phase I of TCGA-LUAD as early stage, phase I of TCGA-LUAD was classified as early stage, and phases II to IV as advanced stage, so as to compare the expression levels of their respective marker genes (28). Figure 3C shows that the characteristic genes of both cell type were downregulated in the advanced LC group, and the downregulation was more significant in B cells. To generate a thorough understanding of tumor-infiltrating immune cells, we used ssGSEA to analyze the proportion of immune cell infiltration and TLS in different stages of LUAD. The results displayed that only B cells, immature dendritic cells (iDCs), and TLS had significant differences in proportion between different stages, among which B cells were the most dominant (Figure 3D). The distribution of the three types was similar with the proportion gradually decreasing with the stage. Then to corroborate the role of B cells in the response to ICB treatment, we use MCP-counter to estimate the immune composition in the ICB-treated cohorts (GSE126044). Figure 3E showed that compared to the non-response group, the response group had a higher number of B cells.
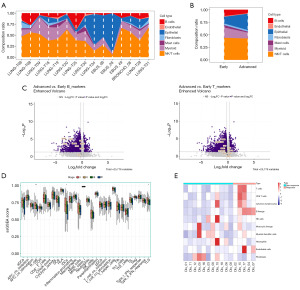
Identification of B cell subsets by scRNA-seq
To generate an integrated transcriptional atlas of B cells in LUAD, we extracting all B cells populations for further subtype analysis. Two main B cell populations were detected: CD20+ B cells and CD138+ plasma cells, which were further refined to characterize five subtypes [naive B cells, memory B cells, GC B cells, immunoglobulin A (IgA)+ plasma cells, and immunoglobulin G (IgG)+ plasma cells] (Figure 4A). When we compared the differences of subtypes between groups, we were surprised to find that while the overall proportion of B cells decreased in the advanced group, the proportion of plasma cells increased significantly (Figure 4B), indicating that plasma cells may be in a state of functional exhaustion in the advanced tumors. Next, we examined whether the level of plasma can predict LUAD prognosis. With the “FindAllMarkers” tool, we identified the marker genes of plasma cells cluster, which were then selected for the signature construction. Here we discovered that patients in the high-level of plasma cells group had a relatively shorter overall survival (OS) (P<0.001) (Figure 4C).
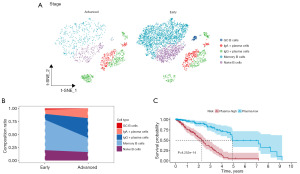
Identification of TLS in TCGA database
We obtained the pathological section information of H&E staining from the TCGA database and classified the samples according to the number of TLS. The samples with TLS in the visual field were distributed to the TLS-high group, and vice versa to the TLS-low group. For each case, slides were reviewed by two independent LC pathologists who were blinded to the patient’s clinical data. The grouping results are shown in table available at https://cdn.amegroups.cn/static/public/tcr-24-1746-1.xlsx. To verify the accuracy of assessment, we compared the distribution of TLS in samples from stage I to stage IV. The results displayed that the number of TLS was inversely proportional to the LUAD stage, similar with those we calculated earlier with ssGSEA algorithm (Figure 5A). Then, to investigate the correlation between TLS and clinical characteristics, we included age, gender, pathological stage, T stage, N stage, M stage, and tumor location. The TLS score was derived using the ssGSEA algorithm. In Figure 5B, we displayed only the correlation coefficients for characteristics with significant associations. The results showed that TLS was significantly correlated with stage, T stage, age, and gender. Next, we elucidated the clinical significance of TLS in LUAD. We brought eight immune and two stromal cell signature gene sets, together with the expression levels of antigen-presentation-related genes (CD40, CD80, CD86, MHC class I, and MHC class II), immune-checkpoint-related genes (PD-1, PD-L1, and CTLA4) and other immunosuppressive genes (TGF-β1, IL-10, TIGHT, HAVCR2, and LAG3) into analysis. As shown in Figure 5C,5D, both results based on TCGA-LUAD and GSE30219 showed a positive correlation between TLS and immune cells, and the correlation with plasma cells was more significant. And higher the TLS score is, better the immune response may be. Furthermore, we examined whether TLS could predict patients’ prognosis. First, we calculated the TLS score of each sample in TCGA-LUAD by ssGSEA algorithm. According to the score, the optimal cutoff value was taken to divided samples into two groups. We found that there were significant differences in prognosis between the two groups with high and low TLS scores (P=0.01) (Figure 6A). Then, we compared survival differences directly between groups based on pathological section, and gained similar results (P=0.008) (Figure 6B). Finally, the KM analysis of the signature constructed by the DEGs between the groups above was conducted, and the result suggested that the signature performed well in predicting survival (P=0.02) (Figure 6C). Since both plasma cells and TLS have strong predictive abilities for the prognosis of LUAD patients, it was hypothesized that the combination of the two signatures might achieve even better predictive performance, as confirmed in Figure 6D, especially in 5-year survival.
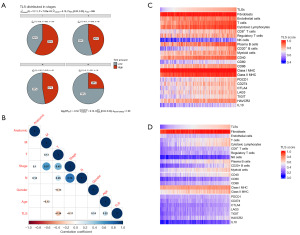
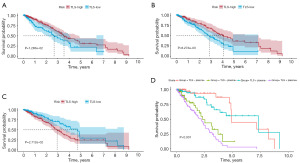
H&E staining and multiplex immunofluorescence analysis
In previous literature, intratumoral B cells have been proved existed in organized TLS. Therefore, to verify our previous speculation, we selected two tumor tissues of different stages for H&E staining and multiple immunofluorescences (Figure 7A: IA tumor; Figure 7B: IIIA tumor). We found that several TLS defined by H&E in Figure 7A,7B, where the typical ones were selected and magnified. Then, to further understand the relationship between different types of immune cells in TLS, we conducted multiplex immunofluorescence. Here, we found that a mix of CD20 B cells (CD19+/CD20+) and CD8 T cells (CD3+/CD8+) distributed surrounding the TLS. In stage IA tumor, CD20 B cells were widely existed, also containing CD8 T cells, while plasma cells (CD138+) were rarely distributed. However, in stage IIIA tumors, the results were quite different. It was clear that there was a decline in the distribution of CD20 B cells (CD19+/CD20+), but a significant increase in the number of plasma cells (CD138+). Taken together, these data suggested that CD20 B cells and plasma cells infiltration may be linked to the tumor stage, verifying our previous speculation.
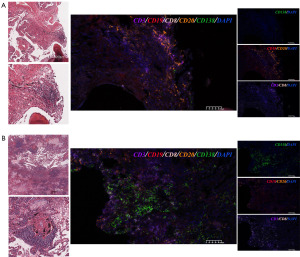
Discussion
LC is the leading cause of cancer morbidity and mortality globally. Thus, how to accurately diagnose and predict prognosis has always been the key research nowadays. Several studies have demonstrated that the presence of B cells may be associated with the prognosis of various types of cancer (27,29-31). Previous exploration of B cells and plasma cells in NSCLC or TLS found that they were associated with better outcomes (21,32-34). As an aggregate composed of various immune cells, TLS enhance immune function through the following mechanisms: promoting antigen presentation and T cell activation, supporting B cell activation and antibody production, producing chemokines and cytokines, and inducing the generation of memory T cells (35-38). While most of these studies were almost exclusively carried on with early-stage samples.
To date, most studies on the effectiveness of immunotherapy focus on T cells, while B cells receive less attention. In our study, we identified several cell types via scRNA analysis based on GSE131907. Most immune cells decreased with the increase of stage, and the trend of B cells was significant. Compared to T cells, marker genes of B cells were more significantly expressed in early-stage LUAD. As an important component of the TME, B cells also play a key role in immunotherapy (39,40). To emphasize the role of B cells in the response to immunotherapy, the MCP-counter algorithm was taken to confirm that the level of B cells was significantly upregulated in the cohort that responded to immunotherapy. It’s well known that B cells are a heterogenous population with phenotypically and functionally distinct subsets. Therefore, characterization of B cells phenotypes was a critical step for further analysis. Functional assessment of tumor-infiltrated B cells subpopulations was also needed to better inform potential targeting strategies. Plasma cells, as a subtype of B cells, play a major role in humoral immunity. While we were surprised to find that the proportion of plasma cells was higher in tumors of advanced stage, in contrast to the trend of remaining B cells. Besides, we proved that a high frequency of plasma cells trended toward shorter OS. These results implied that in the TME of advanced tumors, plasma cells, though abundant, may be in a state of functional exhaustion.
Previous studies have clarified that the function for tumor-infiltrated B cells that is definitively related with augmented survival and immunotherapeutic response in patients is their role in TLS (7,18,33,41). It was a hot topic of research about TLS formation and maintenance in tumors. Several literatures have demonstrated that the presence of inflammatory cytokines and interactions of lymphocyte with stromal cells such as fibroblasts and mesenchymal stem cells were important for the initiation of TLS (42-44). Recent studies have revealed that the presence of B cells and TLS in melanoma, renal cell carcinoma, sarcoma, and head and neck squamous cell carcinoma are associated with better responses to ICB and better prognosis (19-21). While TLS are quite heterogeneous structures (45), where the composition of tumor-infiltrated B cells has not been fully elucidated. In our study, based on the review of pathological sections, the TCGA-LUAD samples were distributed into two groups according to the number of TLS in the field of vision. The results showed that the number of TLS was correlated with the tumor stage. The higher the stage, the less the number of TLS. Multiple immunofluorescences showed that in the IA stage tumor, CD20 B cells were mainly localized in TLS, where also contain CD8 T cells. While, in the IIIA stage tumor, plasma cells were widely existed in TLS. TLS are sites where B cells differentiate and mature. In previous single-cell studies about CRC, ovarian cancer, and hepatocellular carcinoma, CD20 B cells within TLS were found have the tendency to differentiate to IgG plasma cells to produce antibodies to boost the anti-tumor immune response (46-48). This might explain the phenomenon observed in our immunofluorescence experiments. Via KM survival analysis, we found that when both plasma cells and TLS signatures were included, the prediction for the prognosis of LUAD was more effective, especially for 5-year survival (P<0.001).
Based on previous studies, we believe that TLS is an important site for immune responses in the TME. This study analyzed the distribution of various cell populations in the TME at different stages, with a particular focus on TILs, especially B cells. In the immunofluorescence experiment results, the positioning of B cells, plasma cells, and T cells within TLS can be clearly identified. Our data demonstrated the evolution of TLS and plasma cells in tumor development and suggested that plasma cells might gradually become functionally exhausted during tumor progression. Therefore, if the mechanism of functional failure in plasma cells within TLS can be thoroughly investigated and understood, it might offer new therapeutic hope for patients who are resistant to immunotherapy.
Our study has several limitations. First, our study is that it primarily relies on bioinformatics analysis, with a limited sample size and only basic experimental validation, so we were only able to observe preliminary phenomena. Second, it was yet unclear how plasma cells tend to exhaust their function. Moreover, further biochemical experiments such as immunohistochemistry, cell function experiments, etc. are required to confirm the findings.
Conclusions
This study demonstrates the significance of an integrated analysis approach combining scRNA-seq and bulk RNA-seq technologies in identifying novel biomarkers and predicting responses to immunotherapy. Through our analysis, we identified that plasma cells and TLS may play critical roles in the occurrence and progression of LUAD. They not only serve as potential novel biomarkers for LUAD patients but also help predict patient responses to immunotherapy. Subsequently, we constructed prognostic signatures based on their respective characteristic genes and discovered that combining the two signatures resulted in improved predictive performance. These findings have the potential to provide more accurate prognostic evaluations and predictions for immunotherapy in LUAD, offering new directions and insights for future LC treatment research.
Overall, this study provides novel ideas and directions for LC treatment research. Future studies can further explore the role of plasma cells and TLS in LUAD, refine and validate the prognostic signatures, and conduct more clinical trials to confirm their predictive capabilities. This will pave the way for more accurate and personalized prediction and treatment strategies for LUAD.
Acknowledgments
We are very grateful for data provided by databases such as TCGA and GEO. Thanks to reviewers and editors for their sincere comments.
Footnote
Reporting Checklist: The authors have completed the TRIPOD reporting checklist. Available at https://tcr.amegroups.com/article/view/10.21037/tcr-24-1746/rc
Data Sharing Statement: Available at https://tcr.amegroups.com/article/view/10.21037/tcr-24-1746/dss
Peer Review File: Available at https://tcr.amegroups.com/article/view/10.21037/tcr-24-1746/prf
Funding: None.
Conflicts of Interest: All authors have completed the ICMJE uniform disclosure form (available at https://tcr.amegroups.com/article/view/10.21037/tcr-24-1746/coif). The authors have no conflicts of interest to declare.
Ethical Statement: The authors are accountable for all aspects of the work in ensuring that questions related to the accuracy or integrity of any part of the work are appropriately investigated and resolved. The study was conducted in accordance with the Declaration of Helsinki (as revised in 2013). The study was approved by the Ethical Committee of The First Affiliated Hospital of Nanjing Medical University (2023-SR-777) and all patients provided written informed consent.
Open Access Statement: This is an Open Access article distributed in accordance with the Creative Commons Attribution-NonCommercial-NoDerivs 4.0 International License (CC BY-NC-ND 4.0), which permits the non-commercial replication and distribution of the article with the strict proviso that no changes or edits are made and the original work is properly cited (including links to both the formal publication through the relevant DOI and the license). See: https://creativecommons.org/licenses/by-nc-nd/4.0/.
References
- Siegel RL, Miller KD, Wagle NS, et al. Cancer statistics, 2023. CA Cancer J Clin 2023;73:17-48. [Crossref] [PubMed]
- Duma N, Santana-Davila R, Molina JR. Non-Small Cell Lung Cancer: Epidemiology, Screening, Diagnosis, and Treatment. Mayo Clin Proc 2019;94:1623-40. [Crossref] [PubMed]
- Siegel RL, Miller KD, Fuchs HE, et al. Cancer Statistics, 2021. CA Cancer J Clin 2021;71:7-33. [Crossref] [PubMed]
- Quail DF, Joyce JA. Microenvironmental regulation of tumor progression and metastasis. Nat Med 2013;19:1423-37. [Crossref] [PubMed]
- Zhu G, Falahat R, Wang K, et al. Tumor-Associated Tertiary Lymphoid Structures: Gene-Expression Profiling and Their Bioengineering. Front Immunol 2017;8:767. [Crossref] [PubMed]
- Fridman WH, Zitvogel L, Sautès-Fridman C, et al. The immune contexture in cancer prognosis and treatment. Nat Rev Clin Oncol 2017;14:717-34. [Crossref] [PubMed]
- Sautès-Fridman C, Petitprez F, Calderaro J, et al. Tertiary lymphoid structures in the era of cancer immunotherapy. Nat Rev Cancer 2019;19:307-25. [Crossref] [PubMed]
- Vesely MD, Kershaw MH, Schreiber RD, et al. Natural innate and adaptive immunity to cancer. Annu Rev Immunol 2011;29:235-71. [Crossref] [PubMed]
- Sarvaria A, Madrigal JA, Saudemont A. B cell regulation in cancer and anti-tumor immunity. Cell Mol Immunol 2017;14:662-74. [Crossref] [PubMed]
- Tsou P, Katayama H, Ostrin EJ, et al. The Emerging Role of B Cells in Tumor Immunity. Cancer Res 2016;76:5597-601. [Crossref] [PubMed]
- Amaria RN, Reddy SM, Tawbi HA, et al. Neoadjuvant immune checkpoint blockade in high-risk resectable melanoma. Nat Med 2018;24:1649-54. [Crossref] [PubMed]
- Kurai J, Chikumi H, Hashimoto K, et al. Antibody-dependent cellular cytotoxicity mediated by cetuximab against lung cancer cell lines. Clin Cancer Res 2007;13:1552-61. [Crossref] [PubMed]
- Gilbert AE, Karagiannis P, Dodev T, et al. Monitoring the systemic human memory B cell compartment of melanoma patients for anti-tumor IgG antibodies. PLoS One 2011;6:e19330. [Crossref] [PubMed]
- Rivera A, Chen CC, Ron N, et al. Role of B cells as antigen-presenting cells in vivo revisited: antigen-specific B cells are essential for T cell expansion in lymph nodes and for systemic T cell responses to low antigen concentrations. Int Immunol 2001;13:1583-93. [Crossref] [PubMed]
- Munoz-Erazo L, Rhodes JL, Marion VC, et al. Tertiary lymphoid structures in cancer - considerations for patient prognosis. Cell Mol Immunol 2020;17:570-5. [Crossref] [PubMed]
- Carragher DM, Rangel-Moreno J, Randall TD. Ectopic lymphoid tissues and local immunity. Semin Immunol 2008;20:26-42. [Crossref] [PubMed]
- Dieu-Nosjean MC, Goc J, Giraldo NA, et al. Tertiary lymphoid structures in cancer and beyond. Trends Immunol 2014;35:571-80. [Crossref] [PubMed]
- Cabrita R, Lauss M, Sanna A, et al. Tertiary lymphoid structures improve immunotherapy and survival in melanoma. Nature 2020;577:561-5. [Crossref] [PubMed]
- Kim SS, Shen S, Miyauchi S, et al. B Cells Improve Overall Survival in HPV-Associated Squamous Cell Carcinomas and Are Activated by Radiation and PD-1 Blockade. Clin Cancer Res 2020;26:3345-59. [Crossref] [PubMed]
- Helmink BA, Reddy SM, Gao J, et al. B cells and tertiary lymphoid structures promote immunotherapy response. Nature 2020;577:549-55. [Crossref] [PubMed]
- Petitprez F, de Reyniès A, Keung EZ, et al. B cells are associated with survival and immunotherapy response in sarcoma. Nature 2020;577:556-60. [Crossref] [PubMed]
- Chen Z, Zhao M, Li M, et al. Identification of differentially expressed genes in lung adenocarcinoma cells using single-cell RNA sequencing not detected using traditional RNA sequencing and microarray. Lab Invest 2020;100:1318-29. [Crossref] [PubMed]
- Liang L, Yu J, Li J, et al. Integration of scRNA-Seq and Bulk RNA-Seq to Analyse the Heterogeneity of Ovarian Cancer Immune Cells and Establish a Molecular Risk Model. Front Oncol 2021;11:711020. [Crossref] [PubMed]
- Lambrechts D, Wauters E, Boeckx B, et al. Phenotype molding of stromal cells in the lung tumor microenvironment. Nat Med 2018;24:1277-89. [Crossref] [PubMed]
- Travaglini KJ, Nabhan AN, Penland L, et al. A molecular cell atlas of the human lung from single-cell RNA sequencing. Nature 2020;587:619-25. [Crossref] [PubMed]
- André T, Shiu KK, Kim TW, et al. Pembrolizumab in Microsatellite-Instability-High Advanced Colorectal Cancer. N Engl J Med 2020;383:2207-18. [Crossref] [PubMed]
- Xia J, Xie Z, Niu G, et al. Single-cell landscape and clinical outcomes of infiltrating B cells in colorectal cancer. Immunology 2023;168:135-51. [Crossref] [PubMed]
- Becht E, Giraldo NA, Lacroix L, et al. Estimating the population abundance of tissue-infiltrating immune and stromal cell populations using gene expression. Genome Biol 2016;17:218. [Crossref] [PubMed]
- Blenman KRM, He TF, Frankel PH, et al. Sentinel lymph node B cells can predict disease-free survival in breast cancer patients. NPJ Breast Cancer 2018;4:28. [Crossref] [PubMed]
- Greiff V, Bhat P, Cook SC, et al. A bioinformatic framework for immune repertoire diversity profiling enables detection of immunological status. Genome Med 2015;7:49. [Crossref] [PubMed]
- Knief J, Reddemann K, Petrova E, et al. High Density of Tumor-infiltrating B-Lymphocytes and Plasma Cells Signifies Prolonged Overall Survival in Adenocarcinoma of the Esophagogastric Junction. Anticancer Res 2016;36:5339-45. [Crossref] [PubMed]
- Patil NS, Nabet BY, Müller S, et al. Intratumoral plasma cells predict outcomes to PD-L1 blockade in non-small cell lung cancer. Cancer Cell 2022;40:289-300.e4. [Crossref] [PubMed]
- Germain C, Gnjatic S, Tamzalit F, et al. Presence of B cells in tertiary lymphoid structures is associated with a protective immunity in patients with lung cancer. Am J Respir Crit Care Med 2014;189:832-44. [Crossref] [PubMed]
- Gentles AJ, Newman AM, Liu CL, et al. The prognostic landscape of genes and infiltrating immune cells across human cancers. Nat Med 2015;21:938-45. [Crossref] [PubMed]
- Mellman I, Chen DS, Powles T, et al. The cancer-immunity cycle: Indication, genotype, and immunotype. Immunity 2023;56:2188-205. [Crossref] [PubMed]
- Raskov H, Orhan A, Christensen JP, et al. Cytotoxic CD8(+) T cells in cancer and cancer immunotherapy. Br J Cancer 2021;124:359-67. [Crossref] [PubMed]
- Schumacher TN, Thommen DS. Tertiary lymphoid structures in cancer. Science 2022;375:eabf9419. [Crossref] [PubMed]
- Silina K. B cell-rich niches support stem-like CD8(+) T cells in cancer microenvironment. Cancer Cell 2023;41:824-5. [Crossref] [PubMed]
- Lauss M, Donia M, Svane IM, et al. B Cells and Tertiary Lymphoid Structures: Friends or Foes in Cancer Immunotherapy? Clin Cancer Res 2022;28:1751-8. [Crossref] [PubMed]
- Wang SS, Liu W, Ly D, et al. Tumor-infiltrating B cells: their role and application in anti-tumor immunity in lung cancer. Cell Mol Immunol 2019;16:6-18. [Crossref] [PubMed]
- Sautès-Fridman C, Lawand M, Giraldo NA, et al. Tertiary Lymphoid Structures in Cancers: Prognostic Value, Regulation, and Manipulation for Therapeutic Intervention. Front Immunol 2016;7:407. [Crossref] [PubMed]
- Willard-Mack CL. Normal structure, function, and histology of lymph nodes. Toxicol Pathol 2006;34:409-24. [Crossref] [PubMed]
- Gu-Trantien C, Willard-Gallo K. Tumor-infiltrating follicular helper T cells: The new kids on the block. Oncoimmunology 2013;2:e26066. [Crossref] [PubMed]
- Jing F, Choi EY. Potential of Cells and Cytokines/Chemokines to Regulate Tertiary Lymphoid Structures in Human Diseases. Immune Netw 2016;16:271-80. [Crossref] [PubMed]
- Kroeger DR, Milne K, Nelson BH. Tumor-Infiltrating Plasma Cells Are Associated with Tertiary Lymphoid Structures, Cytolytic T-Cell Responses, and Superior Prognosis in Ovarian Cancer. Clin Cancer Res 2016;22:3005-15. [Crossref] [PubMed]
- Lee HO, Hong Y, Etlioglu HE, et al. Lineage-dependent gene expression programs influence the immune landscape of colorectal cancer. Nat Genet 2020;52:594-603. [Crossref] [PubMed]
- Montfort A, Pearce O, Maniati E, et al. A Strong B-cell Response Is Part of the Immune Landscape in Human High-Grade Serous Ovarian Metastases. Clin Cancer Res 2017;23:250-62. [Crossref] [PubMed]
- Wei Y, Lao XM, Xiao X, et al. Plasma Cell Polarization to the Immunoglobulin G Phenotype in Hepatocellular Carcinomas Involves Epigenetic Alterations and Promotes Hepatoma Progression in Mice. Gastroenterology 2019;156:1890-1904.e16. [Crossref] [PubMed]