Impact of systemic immune-inflammation index and its evaluation of optimal threshold in patients with limited-stage small cell lung cancer: a retrospective study based on 572 cases
Highlight box
Key findings
• Elevated systemic immune-inflammation index (SII) is an independent adverse prognostic factor for limited-stage small cell lung cancer (LS-SCLC).
What is known and what is new?
• SII has been used as an emerging biomarker. There are few studies on the prognostic value of SII in patients with LS-SCLC.
• Continuous time-dependent receiver operating characteristic curves (time-dependent ROC curves) were used to clarify the predictive ability of SII. Maximizing the log-rank test statistic was used to determine the marker threshold.
What is the implication, and what should change now?
• SII is a novel and effective clinical prognostic tool with good clinical predictive value for LS-SCLC patients. Continuous time-dependent ROC curves were used to clarify the predictive ability of SII.
Introduction
Malignant tumor is one of the most serious diseases threatening human health with significant socio-economic impacts. About 2.2 million new cases of lung cancer were diagnosed (11.4% of all new cancers worldwide) and 1.8 million died from lung cancer (18% of all cancer deaths worldwide) annually (1).
Small cell lung cancer (SCLC) accounts for about 15% of all lung cancer patients, which is featured by high aggressiveness, strong predilection for early metastasis and poor prognosis. Only approximately 30% of patients have limited-stage SCLC at diagnosis (2). Despite increasing clinical evaluations of new treatment strategies, the disappointing results still underscored the unmet need of developing more effective therapies for SCLC (3,4). Therefore, it is of great significance to identify predictive or prognostic biomarkers for individualized diagnosis and treatment.
Inflammation plays a major role in cancer progression (5,6). Several immune-inflammation-based prognostic biomarkers, such as modified Glasgow prognostic score (mGPS), neutrophil-to-lymphocyte ratio (NLR), and platelet-to-lymphocyte ratio (PLR), have shown to be associated with prognosis in a series of malignant tumors (6). For limited-stage small cell lung cancer (LS-SCLC), the pre-radiotherapy PLR was reported to be significantly associated with overall survival [OS, with a 1 unit increase in PLR corresponding with a 1.001 increase in hazard ratio (HR)] (7). Elevated systemic immune-inflammation index (SII) has been used as an emerging biomarker for the prediction of prognosis in a variety of solid tumors, including advanced gastric cancer, nasopharyngeal cancer, non-small cell lung cancer, and bladder cancer (8-10). Recent studies have also revealed the SII levels could help to predict poor prognosis in SCLC, especially in extensive-stage SCLC (11-13). However, the results remain inconsistent and the predictive value of SII in patients with LS-SCLC has not been clearly investigated.
Therefore, we conduct this retrospective analysis to investigate the prognostic value of SII in patients with LS-SCLC by calculating the optimal threshold of SII in LS-SCLC patients, and compared the predictive efficacy of SII in different survival stages of LS-SCLC patients. The clinicopathological factors affecting the SII level of patients were also screened through correlation analysis to provide evidence for patients’ outcome stratification. We present this article in accordance with the STROBE reporting checklist (available at https://tcr.amegroups.com/article/view/10.21037/tcr-24-1266/rc).
Methods
Patient selection
The study was conducted in accordance with the Declaration of Helsinki (as revised in 2013). The study was approved by the Ethics Committee of The Fourth Hospital of Hebei Medical University (Hebei Tumor Hospital) (No. 2021KY103) and the requirement for individual consent for this retrospective analysis was waived. This retrospective study included patients with SCLC diagnosed from January 2017 to February 2021 in the Radiotherapy Department of The Fourth Hospital of Hebei Medical University (Hebei Tumor Hospital). All patients had pathologically confirmed SCLC without distant metastasis. Other inclusion criteria were as follows: ≥18 years old; no treatment (including surgery, chemotherapy, radiotherapy, and other anti-cancer treatments) was performed before being incorporated into this study; no obvious evidence of infection before treatment, such as bacterial or viral infection; no recent use of hormones and other drugs affecting hematological indicators.
A total of 572 patients with LS-SCLC were recruited in the study. Electronic medical records were reviewed and demographic, clinical, and laboratory data were collected, including gender, age, smoking history, Eastern Cooperative Oncology Group (ECOG) score, regional origin, tumor location, clinical stage [determined by American Joint Committee on Cancer (AJCC) cancer staging and Veterans Administration Lung Study Group (VALG) cancer staging], SII, pre-treatment sodium level, pre-treatment albumin level, treatment approach, chemotherapy cycle, short-term efficacy, presence of prophylactic cranial irradiation (PCI), and presence of brain metastases (BMs) after treatment.
We further collected laboratory data within 1 week of treatment, including complete blood count, electrolyte, liver and kidney function test. SII was calculated using the following equation: SII = (platelet count × neutrophil counts/lymphocyte counts) based on the data within 1 week of treatment.
Response assessment and follow-up
Tumor response was assessed every 1–2 months according to the Response Evaluate Criteria for Solids Tumors (RECIST1.1) (14). Complete response (CR) was defined as disappearance of all assessable target lesions; partial response (PR) was defined as at least a 30% decrease in the sum of the longest diameters of the target lesions; progressive disease (PD) was defined as at least a 20% increase in the sum of the longest diameters of the target lesions with at least a 5-mm absolute increase, or appearance of new lesions; the remaining patients who did not meet the criteria of PD or PR were categorized as stable disease (SD).
Follow-up was performed per treating providers’ discretion as a part of routine clinical care. Follow-up data were collected by electronic medical records, out-patient visit, or contacting patients and relatives, including survival status, time for recurrence or progression, time for metastasis, metastasis site, whether receive subsequent treatment, and time of death. A total of 20 patients were lost to follow-up, with a follow-up rate of 96.5%. The data cut-off was May 1st, 2024.
Statistical analyses
Progression-free survival (PFS) was defined as the time from the start of treatment to objective disease progression. OS was calculated from the start of treatment until the time of death or the last clinical follow-up. The R Programming Language (version 4.2.2) was used to calculate the optimal threshold of SII by maximizing the log-rank test statistic based on OS and PFS (15-17). Continuous time-dependent receiver operating characteristic curves (time-dependent ROC curves) were used to evaluate the diagnostic accuracy of SII with OS. Survival curves were generated using the Kaplan-Meier method, and differences were evaluated using the log-rank test. Multivariable hazard ratios were calculated using the Cox proportional hazard model. Logistic multivariable analysis was used to determine the factors associated with high SII. All statistical tests were two-sided, and P<0.05 was considered statistically significant. Analyses were conducted by using SPSS Statistics version 25.0 [International Business Machines (IBM) Corporation, New York, USA].
Results
Patient characteristics
Study population consisted of 572 patients, including 415 (72.6%) males and 157 (27.4%) females at a median age of 60 years (range, 25–81 years). The majority of patients had central lung cancer (81.1%) with a smoking history (61.0%) and an ECOG score of 0–1 (86.0%). Patients with stage II, IIIA, IIIB, and IIIC disease accounted for 10.8% (n=62), 24.1% (n=138), 38.6% (n=221) and 26.4% (n=151) of the overall population, respectively. A total of 542 (94.8%) patients received chemoradiotherapy and 213 patients (37.2%) received PCI. The clinical characteristics are shown in Table 1.
Table 1
Characteristic | Total (n=572), n (%) | Low SII (n=292), n (%) | High SII (n=280), n (%) | P value |
---|---|---|---|---|
Sex | 0.59 | |||
Male | 415 (72.6) | 209 (50.4) | 206 (49.6) | |
Female | 157 (27.4) | 83 (52.9) | 74 (47.1) | |
Age, years | 0.42 | |||
<60 | 262 (45.8) | 129 (49.2) | 133 (50.8) | |
≥60 | 310 (54.2) | 163 (52.6) | 147 (47.4) | |
Smoking status | 0.75 | |||
Current or ex-smoker | 349 (61.0) | 180 (51.6) | 169 (48.4) | |
Never smoker | 223 (39.0) | 112 (50.2) | 111 (49.8) | |
ECOG score | 0.03 | |||
0–1 | 492 (86.0) | 260 (52.8) | 232 (47.2) | |
≥2 | 80 (14.0) | 32 (40.0) | 48 (60.0) | |
District | 0.01 | |||
Urban | 142 (24.8) | 86 (60.6) | 56 (39.4) | |
Rural | 430 (75.2) | 206 (47.9) | 224 (52.1) | |
Tumor location | 0.50 | |||
Central | 464 (81.1) | 240 (51.7) | 224 (48.3) | |
Peripheral | 108 (18.9) | 52 (48.1) | 56 (51.9) | |
T staging | <0.001 | |||
1–2 | 200 (35.0) | 123 (61.5) | 77 (38.5) | |
3–4 | 372 (65.0) | 169 (45.4) | 203 (54.6) | |
N staging | 0.008 | |||
0–1 | 94 (16.4) | 59 (62.8) | 35 (37.2) | |
2 | 273 (47.7) | 143 (52.4) | 130 (47.6) | |
3 | 205 (35.8) | 90 (43.9) | 115 (56.1) | |
Clinical staging | 0.001 | |||
II | 62 (10.8) | 42 (67.7) | 20 (32.3) | |
IIIA | 138 (24.1) | 81 (58.7) | 57 (41.3) | |
IIIB | 221 (38.6) | 113 (51.1) | 108 (48.9) | |
IIIC | 151 (26.4) | 56 (37.1) | 95 (62.9) | |
Serum sodium | <0.001 | |||
Lower | 178 (31.1) | 70 (39.3) | 108 (60.7) | |
Normal | 394 (68.9) | 222 (56.3) | 172 (43.7) | |
Albumin level | 0.01 | |||
Lower | 209 (36.5) | 92 (44.0) | 117 (56.0) | |
Normal | 363 (63.5) | 200 (55.1) | 163 (44.9) | |
Treatment | 0.26 | |||
Chemoradiotherapy | 542 (94.8) | 281 (51.8) | 261 (48.2) | |
Chemotherapy | 25 (4.4) | 9 (36.0) | 16 (64.0) | |
Radiotherapy | 5 (0.8) | 2 (40.0) | 3 (60.0) | |
Cycles of chemotherapy | 0.06 | |||
<4 | 102 (17.8) | 40 (39.2) | 62 (60.8) | |
4–6 | 422 (73.8) | 227 (53.8) | 195 (46.2) | |
>6 | 43 (7.5) | 23 (53.5) | 20 (46.5) | |
Response | <0.001 | |||
CR + PR | 280 (49.0) | 176 (62.9) | 104 (37.1) | |
SD | 287 (50.2) | 114 (39.7) | 173 (60.3) | |
PD | 5 (0.9) | 2 (40.0) | 3 (60.0) | |
PCI | 0.03 | |||
Yes | 213 (37.2) | 121 (56.8) | 92 (43.2) | |
No | 359 (62.8) | 171 (47.6) | 188 (52.4) | |
BM after treatment | 0.24 | |||
Yes | 221 (38.6) | 126 (57.0) | 95 (43.0) | |
No | 351 (61.4) | 166 (47.3) | 185 (52.7) |
SII, systemic immune-inflammation index; ECOG, Eastern Cooperative Oncology Group; CR, complete response; PR, partial response; SD, stable disease; PD, progressive disease; PCI, prophylactic cranial irradiation; BM, brain metastasis.
Optimal threshold of SII & prediction performance
The maximum log-rank test analysis of SII based on OS and PFS defined cut-off values of 760.6 each, respectively (Figure 1). Continuous time-dependent ROC showed that the area under the curves (AUC) of SII in 12-, 24- and 36-months were 0.727, 0.708, 0.680, respectively (Figure 2). The disparities of the area under the time-dependent ROC curves of SII in 12-, 24-, and 36-months were statistically significant (P=0.048). It is generally considered that the AUC of 0.5 suggests a non-effective predictive ability and the range of 0.7 or above indicates acceptable diagnostic accuracy (an AUC of 0.8 or above is deemed to be good discriminatory ability).
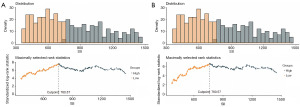
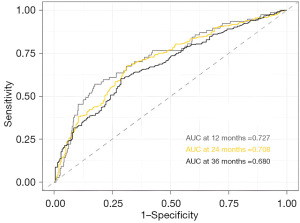
Survival outcomes
Overall, the median OS and PFS was 26.0 months [95% confidence interval (CI): 23.8–28.2] and 13.0 months (95% CI: 11.3–14.7), respectively, and the 1-, 3-, and 5-year OS rates and PFS rates were 81.1%, 35.5%, and 27.1%, and 52.8%, 24.8%, and 19.9%, respectively (Figure 3).
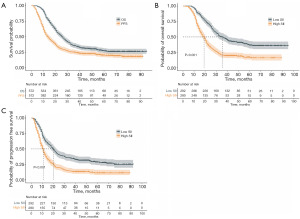
We calculated the association between OS and PFS with SII by the Kaplan-Meier method and the median OS and PFS of the low SII group (35.0 months, 95% CI: 30.0–40.0, P<0.001; 20.0 months, 95% CI: 17.3–22.7, P<0.001) were better than those in the high SII group (19.0 months, 95% CI: 17.1–20.9, P<0.001; 11.0 months, 95% CI: 9.9–12.1, P<0.001) (Figure 3).
In multivariable survival analysis, SII remained an independent prognostic factor for OS (HR: 1.699; 95% CI: 1.374–2.100; P=0.001) and PFS (HR: 1.482; 95% CI: 1.214–1.809; P<0.001). In addition, gender, N staging, serum sodium, treatment modality, response, PCI and BM after treatment were significantly associated with OS. N staging, treatment modality, cycles of chemotherapy, response and PCI were significantly associated with PFS (Table 2). Sensitivity analysis was conducted by selecting patients who was treated in 2 weeks between diagnosis and treatment. This sensitivity analysis reviewed clinical data of 366 patients with LS-SCLC and the results showed that this analysis was similar to the results of the full group analysis (Table S1).
Table 2
Characteristic | OS | PFS | |||
---|---|---|---|---|---|
HR (95% CI) | P value | HR (95% CI) | P value | ||
SII | |||||
Low | 1 (reference) | NA | 1 (reference) | NA | |
High | 1.699 (1.374–2.100) | 0.001 | 1.482 (1.214–1.809) | <0.001 | |
Sex | |||||
Male | 1 (reference) | NA | 1 (reference) | NA | |
Female | 0.614 (0.435–0.867) | 0.01 | 0.811 (0.589–1.116) | 0.19 | |
Age, years | |||||
<60 | 1 (reference) | NA | 1 (reference) | NA | |
≥60 | 1.144 (0.924–1.416) | 0.21 | 0.914 (0.749–1.114) | 0.37 | |
Smoking status | |||||
Current or ex-smoker | 1 (reference) | NA | 1 (reference) | NA | |
Never smoker | 1.166 (0.857–1.587) | 0.32 | 1.065 (0.795–1.428) | 0.67 | |
ECOG score | |||||
0–1 | 1 (reference) | NA | 1 (reference) | NA | |
≥2 | 1.046 (0.787–1.390) | 0.75 | 0.853 (0.647–1.124) | 0.25 | |
District | |||||
Urban | 1 (reference) | NA | 1 (reference) | NA | |
Rural | 0.988 (0.774–1.261) | 0.92 | 0.863 (0.685–1.086) | 0.20 | |
Tumor location | |||||
Central | 1 (reference) | NA | 1 (reference) | NA | |
Peripheral | 0.924 (0.708–1.204) | 0.55 | 0.85 (0.660–1.093) | 0.20 | |
T staging | |||||
1–2 | 1 (reference) | NA | 1 (reference) | NA | |
3–4 | 1.12 (0.893–1.404) | 0.32 | 1.033 (0.836–1.276) | 0.76 | |
N staging | |||||
0–1 | 1 (reference) | NA | 1 (reference) | NA | |
2 | 1.784 (1.245–2.557) | 0.002 | 1.571 (1.145–2.157) | 0.005 | |
3 | 2.761 (1.958–4.155) | 0.001 | 2.007 (1.437–2.803) | <0.001 | |
Serum sodium | |||||
Lower | 1 (reference) | NA | 1 (reference) | NA | |
Normal | 0.854 (0.685–0.945) | 0.01 | 0.887 (0.720–1.094) | 0.26 | |
Albumin level | |||||
Lower | 1 (reference) | NA | 1 (reference) | NA | |
Normal | 0.924 (0.751–1.137) | 0.45 | 0.928 (0.760–1.134) | 0.46 | |
Treatment modality | |||||
Chemoradiotherapy | 1 (reference) | NA | 1 (reference) | NA | |
Chemotherapy | 2.382 (1.287–4.408) | 0.006 | 3.257 (1.832–5.791) | <0.001 | |
Radiotherapy | 1.908 (0.572–6.363) | 0.29 | 1.808 (0.649–5.038) | 0.25 | |
Cycles of chemotherapy | |||||
<4 | 1 (reference) | NA | 1 (reference) | NA | |
4–6 | 0.81 (0.625–1.050) | 0.11 | 0.748 (0.585–0.957) | 0.02 | |
>6 | 0.823 (0.538–1.258) | 0.36 | 0.6 (0.401–0.899) | 0.01 | |
Response | |||||
CR + PR | 1 (reference) | NA | 1 (reference) | NA | |
SD | 3.282 (2.594–4.154) | 0.001 | 2.398 (1.926–2.986) | <0.001 | |
PD | 8.306 (2.940–23.464) | <0.001 | – | – | |
PCI | |||||
Yes | 1 (reference) | NA | 1 (reference) | NA | |
No | 1.621 (1.267–2.075) | 0.001 | 1.709 (1.360–2.149) | <0.001 | |
BM after treatment | |||||
Yes | 1 (reference) | NA | – | – | |
No | 2.481 (1.267–2.075) | <0.001 | – | – |
OS, overall survival; PFS, progression free survival; 1 (reference): control; HR, hazard ratio; NA, not applicable; –, not counted; SII, systemic immune-inflammation index; ECOG, Eastern Cooperative Oncology Group; CR, complete response; PR, partial response; SD, stable disease; PD, progressive disease; PCI, prophylactic cranial irradiation; BM, brain metastasis.
Correlations between SII and clinicopathological parameters
We found that patients with higher T [T3–4: odds ratio (OR), 1.707; 95% CI: 1.181–2.468, P=0.004] and N stages (N2: OR, 1.400; 95% CI: 0.847–2.311; P=0.18; N3: OR, 1.907; 95% CI: 1.119–3.249; P=0.01) were more likely to have higher SII levels; while the normal serum sodium before treatment was negatively associated with SII level (normal: OR, 0.541; 95% CI: 0.370–0.791; P=0.001) (Table 3).
Table 3
Characteristic | OR (95% CI) | P value |
---|---|---|
Sex | ||
Male | 1 (reference) | NA |
Female | 0.684 (0.385–1.215) | 0.68 |
Age, years | ||
<60 | 1 (reference) | NA |
≥60 | 0.943 (0.662–1.343) | 0.74 |
Smoking status | ||
Current or ex-smoker | 1 (reference) | NA |
Never smoker | 1.292 (0.759–2.198) | 0.34 |
ECOG | ||
0–1 | 1 (reference) | NA |
≥2 | 1.277 (0.766–2.128) | 0.34 |
District | ||
Urban | 1 (reference) | NA |
Rural | 1.193 (0.825–2.550) | 0.11 |
Tumor location | ||
Central | 1 (reference) | NA |
Peripheral | 1.318 (0.843–2.061) | 0.22 |
T staging | ||
1–2 | 1 (reference) | NA |
3–4 | 1.707 (1.181–2.468) | 0.004 |
N staging | ||
0–1 | 1 (reference) | NA |
2 | 1.4 (0.847–2.311) | 0.18 |
3 | 1.907 (1.119–3.249) | 0.01 |
Serum sodium | ||
Lower | 1 (reference) | NA |
Normal | 0.541 (0.370–0.791) | 0.001 |
Albumin | ||
Lower | 1 (reference) | NA |
Normal | 0.739 (0.516–1.060) | 0.10 |
1 (reference), control; OR, odds ratio; NA, not applicable; ECOG, Eastern Cooperative Oncology Group; CI, confidence interval.
Discussion
To our knowledge, the study may be the largest study reported thus far for assessing the prognostic value of SII for survival in patients with LS-SCLC. We found that elevated SII was independent adverse prognostic factor of OS (P=0.001) and PFS (P<0.001) with certain predictive value for survival outcomes. Thus, as an easy-to-obtain and noninvasive prognostic biomarker, SII has the potential for survival and tumor progression surveillance in patients with LS-SCLC, which might provide a powerful test for assessing LS-SCLC prognosis in future clinical practice.
Changes in SII value indicated fluctuations in platelet, neutrophil or lymphocyte counts, which partly reflected the balance between the host’s immune and inflammatory (18). Several recent studies investigated the underlying mechanisms that explained the relationship between SII and tumor prognosis (19,20). Tumor cells induced inflammation by stimulating the pro-inflammatory mediators, which promoted angiogenesis and inhibited apoptosis to boost tumor cell proliferation and invasion (18). The SII incorporated neutrophil, lymphocyte and platelet counts in the peripheral blood. As an indicator for acute and chronic inflammation, neutrophil in peripheral blood can enhance the adhesion of circulating tumor cells (CTCs) and promote CTCs metastases to target organs (21). It can also inhibit the cytolytic activity of immune cells by secreting cytokines and chemokines, such as matrix metalloproteinase-9, leading to tumorigenesis and metastasis (22,23). Platelets were involved in the tumor migration through the secretion of vascular endothelial growth factor (VEGF), transforming growth factor β (TGF-β), and platelet-derived growth factor (PDGF) (24,25). PDGF can promote epithelial mesenchymal transformation (EMT) by activating SMAD (signal transduction molecules downstream of TGF-β family of receptors) and nuclear factor kappa-light-chain-enhancer of activated B cells (NF-κB) pathways, which plays an important role in early tumor metastasis (26). Immune monitoring effects of lymphocytes are attributed to the inhibition of tumor cell proliferation, invasion and migration (27). The results of these related studies may reveal that high SII level contribute to cancer progression and lead to poorer survival. Consistent with previous studies, we also found that SII level was correlated with T staging, N staging and pre-treatment serum sodium by multivariable analysis (28). In general, higher SII was positively associated with advanced disease. SII cannot replace the role of T and N stage in patient prognosis. The findings of this large sample sized study can serve as an extended validation of the conventional blood testing during our daily practice.
Inconsistent optimal threshold for inflammatory indicators were reported in different studies (29,30), indicating various cut-off values for inflammatory markers and indexes, such as SII, neutrophil counts/lymphocyte counts, and platelet counts/lymphocyte counts. Among them, Geng’s study used the median value of corresponding inflammatory indicators as the cut-off point, on the basis of which prognostic analysis could be performed in high- and low-level groups (31). This method of transforming continuous variables into dichotomy variables is simple with certain limitations, which may affect statistical efficiency or introduce new biases (32). In addition, most studies use the traditional ROC curve to convert continuous variables to dichotomy variables (8,33,34), which is a common analysis method for diagnostic tests. However, it should be noted that disease status and marker value is assumed to be fixed (do not change over time) in the traditional ROC curve analysis, while in practice, disease status and marker changed over time. The traditional ROC curve ignores the time dependence of disease status or marker value, resulting in bias in the sensitivity and specificity. Censoring is a common issue in time-dependent ROC curve data and cannot be ignored. In this study, we followed every patient carefully and almost every imaging examination was done in our hospital. In our study, the threshold for SII was determined using an outcome-based method by maximizing the log-rank test statistic, as previously shown in other malignant tumors (35). It makes up for the deficiencies that the traditional ROC curve does not consider the association between longitudinal marker and the corresponding event-time processes. Furthermore, we calculated the AUC of SII at 12-, 24-, and 36-months using time-dependent ROC, revealing favorable predictive values. The discriminatory ability of the SII becomes less effective over time. There are several possible reasons for this phenomenon: cancer-specific survival (CSS) was changed and prognostic accuracy is reduced due to the occurrence of non-cancer-related deaths among long-term survival patients; in addition to the SII, the prognostic factors also include treatment style, treatment effect and other clinicopathological factors. The dissimilar timepoints detecting SII during cancer treatment or measurement bias influenced the final results.
Our study analyzed clinicopathological factors, such as clinical baseline characteristics, treatment methods, and hematological indicators of patients before treatment. Together with previous studies, the current study further confirmed that SII levels have a significant impact on survival outcomes in patients with SCLC (8,36-38). Different from Yu et al.’s study, which calculated SII using blood routine testing results before radiotherapy (7), blood routine examination testing results 1 week before treatment were chosen for SII calculation. We also eliminated the factors that might cause changes in SII value, such as infection.
Our study was limited by the retrospective design, which is prone to introduce information bias or selection bias. Another limitation was that information on potential biomarker was not available. Moreover, the present study is a single-center study, thus the results cannot be 100% replicated or generalized due to the lack of external validation. Future prospective multicenter studies in larger sample size are warranted.
Conclusions
In conclusion, our study demonstrated that the SII, an easy-to-assess inflammation-based biomarker, is an independent prognostic factor for OS and PFS in patients with LS-SCLC with favorable predictive value. Further researches would be warranted to optimize treatment for newly diagnosed patients based on SII status.
Acknowledgments
We appreciated Hebei Tumor Hospital’s support and assistance for this research.
Footnote
Reporting Checklist: The authors have completed the STROBE reporting checklist. Available at https://tcr.amegroups.com/article/view/10.21037/tcr-24-1266/rc
Data Sharing Statement: Available at https://tcr.amegroups.com/article/view/10.21037/tcr-24-1266/dss
Peer Review File: Available at https://tcr.amegroups.com/article/view/10.21037/tcr-24-1266/prf
Funding: None.
Conflicts of Interest: All authors have completed the ICMJE uniform disclosure form (available at https://tcr.amegroups.com/article/view/10.21037/tcr-24-1266/coif). The authors have no conflicts of interest to declare.
Ethical Statement: The authors are accountable for all aspects of the work in ensuring that questions related to the accuracy or integrity of any part of the work are appropriately investigated and resolved. The study was conducted in accordance with the Declaration of Helsinki (as revised in 2013). The study was approved by the Ethics Committee of The Fourth Hospital of Hebei Medical University (Hebei Tumor Hospital) (No. 2021KY103) and the requirement for individual consent for this retrospective analysis was waived.
Open Access Statement: This is an Open Access article distributed in accordance with the Creative Commons Attribution-NonCommercial-NoDerivs 4.0 International License (CC BY-NC-ND 4.0), which permits the non-commercial replication and distribution of the article with the strict proviso that no changes or edits are made and the original work is properly cited (including links to both the formal publication through the relevant DOI and the license). See: https://creativecommons.org/licenses/by-nc-nd/4.0/.
References
- Sung H, Ferlay J, Siegel RL, et al. Global Cancer Statistics 2020: GLOBOCAN Estimates of Incidence and Mortality Worldwide for 36 Cancers in 185 Countries. CA Cancer J Clin 2021;71:209-49. [Crossref] [PubMed]
- Byers LA, Rudin CM. Small cell lung cancer: where do we go from here? Cancer 2015;121:664-72. [Crossref] [PubMed]
- Ganti AKP, Loo BW, Bassetti M, et al. Small Cell Lung Cancer, Version 2.2022, NCCN Clinical Practice Guidelines in Oncology. J Natl Compr Canc Netw 2021;19:1441-64. [Crossref] [PubMed]
- Xia C, Dong X, Li H, et al. Cancer statistics in China and United States, 2022: profiles, trends, and determinants. Chin Med J (Engl) 2022;135:584-90. [Crossref] [PubMed]
- Proctor MJ, Morrison DS, Talwar D, et al. An inflammation-based prognostic score (mGPS) predicts cancer survival independent of tumour site: a Glasgow Inflammation Outcome Study. Br J Cancer 2011;104:726-34. [Crossref] [PubMed]
- Tong YS, Tan J, Zhou XL, et al. Systemic immune-inflammation index predicting chemoradiation resistance and poor outcome in patients with stage III non-small cell lung cancer. J Transl Med 2017;15:221. [Crossref] [PubMed]
- Yu Y, Wang L, Cao S, et al. Pre-radiotherapy lymphocyte count and platelet-to-lymphocyte ratio may improve survival prediction beyond clinical factors in limited stage small cell lung cancer: model development and validation. Transl Lung Cancer Res 2020;9:2315-27. [Crossref] [PubMed]
- Yuan X, Feng H, Huang H, et al. Systemic immune-inflammation index during treatment predicts prognosis and guides clinical treatment in patients with nasopharyngeal carcinoma. J Cancer Res Clin Oncol 2023;149:191-202. [Crossref] [PubMed]
- Zhang B, Xu T. Prognostic significance of pretreatment systemic immune-inflammation index in patients with prostate cancer: a meta-analysis. World J Surg Oncol 2023;21:2. [Crossref] [PubMed]
- Zhang R, Hu C, Zhang J, et al. Prognostic significance of inflammatory and nutritional markers in perioperative period for patients with advanced gastric cancer. BMC Cancer 2023;23:5. [Crossref] [PubMed]
- Zhou Y, Dai M, Zhang Z. Prognostic Significance of the Systemic Immune-Inflammation Index (SII) in Patients With Small Cell Lung Cancer: A Meta-Analysis. Front Oncol 2022;12:814727. [Crossref] [PubMed]
- Baek JM, Cha H, Moon Y, et al. A Systemic Immune Inflammation Index and PD-L1 (SP142) Expression as a Potential Combined Biomarker of the Clinical Benefit of Chemo-Immunotherapy in Extensive-Stage Small-Cell Lung Cancer. J Clin Med 2024;13:1521. [Crossref] [PubMed]
- Yao JH, Shao Y, Wang JJ, et al. Evaluation of diagnostic and predictive values of the serum VEGF-A level and systemic immune-inflammation index in small cell lung cancer. J Cancer 2021;12:1356-64. [Crossref] [PubMed]
- Kim DH, Min EJ, Kim B, et al. RECIST 1.1, mRECIST, and Choi criteria for evaluating treatment response and survival outcomes in hepatocellular carcinoma patients treated with atezolizumab plus bevacizumab. Eur Radiol 2024; Epub ahead of print. [Crossref] [PubMed]
- Contal C, O'Quigley J. An application of changepoint methods in studying the effect of age on survival in breast cancer. Comput Stat Data Anal 2019;30:253-70. [Crossref]
- Dell'Aquila E, Cremolini C, Zeppola T, et al. Prognostic and predictive role of neutrophil/lymphocytes ratio in metastatic colorectal cancer: a retrospective analysis of the TRIBE study by GONO. Ann Oncol 2018;29:924-30. [Crossref] [PubMed]
- Howard R, Kanetsky PA, Egan KM. Exploring the prognostic value of the neutrophil-to-lymphocyte ratio in cancer. Sci Rep 2019;9:19673. [Crossref] [PubMed]
- Guo D, Zhang J, Jing W, et al. Prognostic value of systemic immune-inflammation index in patients with advanced non-small-cell lung cancer. Future Oncol 2018;14:2643-50. [Crossref] [PubMed]
- Zhang J, Dai S. Prognostic and clinicopathological role of pretreatment systemic immune-inflammation index in patients with oral squamous cell carcinoma: a meta-analysis. Front Oncol 2024;13:1303132. [Crossref] [PubMed]
- Han W, Weng K, Zhang P, et al. Predictive value of systemic immune-inflammation index for pathological complete response in patients receiving neoadjuvant immunochemotherapy for locally advanced esophageal cancer. Front Surg 2023;9:1091601. [Crossref] [PubMed]
- De Larco JE, Wuertz BR, Furcht LT. The potential role of neutrophils in promoting the metastatic phenotype of tumors releasing interleukin-8. Clin Cancer Res 2004;10:4895-900. [Crossref] [PubMed]
- Bausch D, Pausch T, Krauss T, et al. Neutrophil granulocyte derived MMP-9 is a VEGF independent functional component of the angiogenic switch in pancreatic ductal adenocarcinoma. Angiogenesis 2011;14:235-43. [Crossref] [PubMed]
- Tan KW, Chong SZ, Wong FH, et al. Neutrophils contribute to inflammatory lymphangiogenesis by increasing VEGF-A bioavailability and secreting VEGF-D. Blood 2013;122:3666-77. [Crossref] [PubMed]
- Bambace NM, Holmes CE. The platelet contribution to cancer progression. J Thromb Haemost 2011;9:237-49. [Crossref] [PubMed]
- Lim JU, Yeo CD, Kang HS, et al. Prognostic value of platelet count and lymphocyte to monocyte ratio combination in stage IV non-small cell lung cancer with malignant pleural effusion. PLoS One 2018;13:e0200341. [Crossref] [PubMed]
- Labelle M, Begum S, Hynes RO. Direct signaling between platelets and cancer cells induces an epithelial-mesenchymal-like transition and promotes metastasis. Cancer Cell 2011;20:576-90. [Crossref] [PubMed]
- Shoji F, Morodomi Y, Akamine T, et al. Predictive impact for postoperative recurrence using the preoperative prognostic nutritional index in pathological stage I non-small cell lung cancer. Lung Cancer 2016;98:15-21. [Crossref] [PubMed]
- Hong X, Cui B, Wang M, et al. Systemic Immune-inflammation Index, Based on Platelet Counts and Neutrophil-Lymphocyte Ratio, Is Useful for Predicting Prognosis in Small Cell Lung Cancer. Tohoku J Exp Med 2015;236:297-304. [Crossref] [PubMed]
- Özcan P, Düzgün Ö. The Importance of Preoperative NLR, PLR, and MPV Values in Predicting the Risk of Complications in Colorectal Peritoneal Carcinomatosis. J Pers Med 2024;14:916. [Crossref] [PubMed]
- Koc DC, Mănescu IB, Mănescu M, et al. A Review of the Prognostic Significance of Neutrophil-to-Lymphocyte Ratio in Nonhematologic Malignancies. Diagnostics (Basel) 2024;14:2057. [Crossref] [PubMed]
- Geng Y, Shao Y, Zhu D, et al. Systemic Immune-Inflammation Index Predicts Prognosis of Patients with Esophageal Squamous Cell Carcinoma: A Propensity Score-matched Analysis. Sci Rep 2016;6:39482. [Crossref] [PubMed]
- Naggara O, Raymond J, Guilbert F, et al. Analysis by categorizing or dichotomizing continuous variables is inadvisable: an example from the natural history of unruptured aneurysms. AJNR Am J Neuroradiol 2011;32:437-40. [Crossref] [PubMed]
- Wang L, Wang C, Wang J, et al. A novel systemic immune-inflammation index predicts survival and quality of life of patients after curative resection for esophageal squamous cell carcinoma. J Cancer Res Clin Oncol 2017;143:2077-86. [Crossref] [PubMed]
- Xiong Q, Huang Z, Xin L, et al. Post-treatment neutrophil-to-lymphocyte ratio (NLR) predicts response to anti-PD-1/PD-L1 antibody in SCLC patients at early phase. Cancer Immunol Immunother 2021;70:713-20. [Crossref] [PubMed]
- Ma SJ, Yu H, Khan M, et al. Evaluation of Optimal Threshold of Neutrophil-Lymphocyte Ratio and Its Association With Survival Outcomes Among Patients With Head and Neck Cancer. JAMA Netw Open 2022;5:e227567. [Crossref] [PubMed]
- Hua T, Miao-miao L, Rong-san Z, et al. Effect of systemic immune-inflammation index on prognosis of patients with small cell lung cancer. Journal of Hebei Medical University 2021;42:886-890.
- Wang C, Jin S, Xu S, et al. High Systemic Immune-Inflammation Index (SII) Represents an Unfavorable Prognostic Factor for Small Cell Lung Cancer Treated with Etoposide and Platinum-Based Chemotherapy. Lung 2020;198:405-14. [Crossref] [PubMed]
- Zheng F, Meng Q, Zhang L, et al. Prognostic roles of hematological indicators for the efficacy and prognosis of immune checkpoint inhibitors in patients with advanced tumors: a retrospective cohort study. World J Surg Oncol 2023;21:198. [Crossref] [PubMed]