A predictive nomogram for predicting liver metastasis in early-onset colon cancer: a population-based study
Highlight box
Key findings
• Histological type, T stage, N stage, and carcinoembryonic antigen (CEA) level were independent risk factors for liver metastasis (LM) in patients with early-onset colon cancer identified through univariate and multivariate analyses.
What is known and what is new?
• Early-onset colorectal cancer (EO-CRC) is diagnosed before age 50. Since the early 1990s, EO-CRC rates have nearly doubled due to increased screening, while overall colorectal cancer (CRC) incidence has decreased. The LM rate in CRC patients ranges from 17% to 26.5%, and it is the primary site of metastasis for those with EO-CRC.
• The incidence of LM in patients with early-onset colon cancer was 18.4% according to our study. This is higher than the results of previous studies that focused on creating a predictive model for LM in individuals with CRC.
• The nomogram developed in our study can objectively and accurately predict the risk of LM in patients with early-onset colon cancer.
What is the implication, and what should change now?
• It is important to assess risk factors to evaluate the presence of LM in early-onset colon cancer, thereby deciding the optimal treatment before further therapy, which may improve the quality of life in patients with early-onset colon cancer.
Introduction
Background
Colorectal cancer (CRC) is a prevalent malignant disease that ranks as the third most frequent tumor and the second most fatal form of cancer (1). Early-onset CRC (EO-CRC) is defined as a CRC diagnosed before the age of 50 years (2). The rate of EO-CRC has almost doubled since the early 1990s with the widespread use of screening tests, leading to a decrease in overall CRC incidence (3,4). The liver metastasis (LM) rate among patients with CRC generally ranges from 17% to 26.5% (5,6). The liver is also the primary site of metastasis in patients with EO-CRC compared to CRC. A recent study using the same Surveillance, Epidemiology, and End Results (SEER) database indicates an LM rate of 14% in patients with EO-CRC, while another study shows that approximately 11.9% of newly diagnosed patients in the same population have LM (7,8). Early detection of early-onset colon cancer at high risk for LM can aid clinicians in initiating tailored screening and individualized therapy, resulting in more accurate patient selection, decreased use of unnecessary imaging exams, minimized treatment interruptions, reduced diagnostic uncertainties, and ultimately lowered healthcare costs.
Rationale and knowledge gap
Previous research has established a prognostic nomogram for patients with EO-CRC and LM, and another study has developed a predictive nomogram for patients with EO-CRC and lymphatic metastasis (9,10). However, there is limited literature on the prediction of LM in patients with early-onset colon cancer, and a lack of understanding regarding the clinicopathological risk factors associated with LM in this patient population. Considering the distinct histological and pathological characteristics of rectal and colon cancers, this study focused on patients with early-onset colon cancer. The tumor node metastasis (TNM) staging system is the established method for clinically classifying malignancies and is widely utilized in the prognostic evaluation of cancer, indicating the size and extent of the primary tumor, regional lymph nodes, and distant metastasis. However, it does not encompass all risk factors associated with specific organ metastases, such as LM. Therefore, it is essential to identify risk factors for early-onset colon cancer patients with LM and develop a quantitative prediction nomogram.
Objective
In this study, we aimed to develop a nomogram using data from the SEER database to predict the occurrence of LM in patients with early-onset colon cancer. This approach helps to identify individuals at a high risk of LM, providing valuable insights that can optimize treatment strategies in clinical practice for patients with early-onset colon cancer and LM. We present this article in accordance with the TRIPOD reporting checklist (available at https://tcr.amegroups.com/article/view/10.21037/tcr-24-1159/rc).
Methods
Study population and criteria for inclusion and exclusion
The SEER database, which includes data from over a quarter of the US population, is considered one of the most valuable cancer registry databases globally (11-13). Data on patients diagnosed with colon cancer from 2010 to 2015 were obtained using SEER*Stat version 8.4.3, focusing on the following primary site codes: C18.0 (cecum), C18.2 (ascending colon), C18.3 (hepatic flexure), C18.4 (transverse colon), C18.5 (splenic flexure), C18.6 (descending colon), and C18.7 (sigmoid colon). The histology codes utilized from SEER included: 8140–8147, 8210–8211, 8220–8221, 8260–8263, 8480–8481, and 8490. For each patient, data collected encompassed age, race, gender, histological type, TNM stage according to the seventh edition of the American Joint Committee on Cancer guidelines, tumor location and size, serum carcinoembryonic antigen (CEA) levels as well as lymph metastasis status. The criteria for inclusion were: (I) confirmation of primary colon cancer via microscopic examination; (II) age under 50 years; and (III) diagnosis of only primary colon cancer without other malignancies present. Exclusion criteria consisted of: (I) presence of multiple primary tumors; (II) cases documented solely through autopsy reports or death certificates; (III) incomplete or missing information regarding any inclusion criterion; and (IV) individuals younger than 18 years old. Following these parameters led to a total of 4,890 patients being part of this study (Figure 1). And we utilized R software’s sample function to randomly allocate early-onset colon cancer patients with LM in a 7:3 ratio to a training cohort (n=3,423) and a validation cohort (n=1,467) for the construction and validation of the nomogram (Table 1) (10,14). The validation set population was utilized to perform internal validation of the developed nomogram. This research relied on publicly accessible data from the SEER database, with all patient information anonymized prior to collection. As a result, approval from an ethics committee and informed consent from patients were not necessary. The study was conducted in accordance with the Declaration of Helsinki (as revised in 2013).
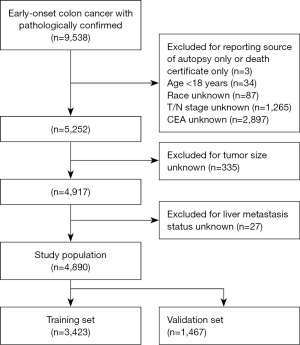
Table 1
Characteristics | Training set (N=3,423) | Validation set (N=1,467) | χ2 | P |
---|---|---|---|---|
Age, n (%) | 0.34964 | 0.55 | ||
<40 years | 895 (26.1) | 371 (25.3) | ||
40–50 years | 2,528 (73.9) | 1,096 (74.7) | ||
Sex, n (%) | 0.0038968 | 0.95 | ||
Female | 1,675 (48.9) | 720 (49.1) | ||
Male | 1,748 (51.1) | 747 (50.9) | ||
Race, n (%) | 5.1907 | 0.07 | ||
Black | 525 (15.3) | 260 (17.7) | ||
Others | 414 (12.1) | 186 (12.7) | ||
White | 2,484 (72.6) | 1,021 (69.6) | ||
Histological type, n (%) | 0.33351 | 0.56 | ||
Adenocarcinoma | 2,611 (76.3) | 1,107 (75.5) | ||
Others | 812 (23.7) | 360 (24.5) | ||
T stage, n (%) | 2.6509 | 0.45 | ||
T1 | 197 (5.8) | 102 (7.0) | ||
T2 | 327 (9.6) | 142 (9.7) | ||
T3 | 2,041 (59.6) | 863 (58.8) | ||
T4 | 858 (25.1) | 360 (24.5) | ||
N stage, n (%) | 4.5309 | 0.10 | ||
N0 | 1,427 (41.7) | 633 (43.1) | ||
N1 | 1,039 (30.4) | 467 (31.8) | ||
N2 | 957 (28.0) | 367 (25.0) | ||
Tumor size, n (%) | 0.49361 | 0.48 | ||
≤50 mm | 1,872 (54.7) | 819 (55.8) | ||
>50 mm | 1,551 (45.3) | 648 (44.2) | ||
Tumor location, n (%) | 2.9345 | 0.82 | ||
Ascending colon | 508 (14.8) | 227 (15.5) | ||
Cecum | 552 (16.1) | 222 (15.1) | ||
Descending colon | 338 (9.9) | 150 (10.2) | ||
Hepatic flexure | 157 (4.6) | 62 (4.2) | ||
Sigmoid colon | 1,385 (40.5) | 612 (41.7) | ||
Splenic flexure | 137 (4.0) | 61 (4.2) | ||
Transverse colon | 346 (10.1) | 133 (9.1) | ||
CEA, n (%) | 0.80055 | 0.37 | ||
Negative | 1,932 (56.4) | 849 (57.9) | ||
Positive | 1,491 (43.6) | 618 (42.1) | ||
Liver metastasis, n (%) | 1.0132 | 0.31 | ||
No | 2,780 (81.2) | 1,210 (82.5) | ||
Yes | 643 (18.8) | 257 (17.5) |
CEA, carcinoembryonic antigen.
Construction of the prediction model
A predictive model was developed utilizing the independent risk factors and the training set through the “glm” function in R. The resulting model was visually represented as a nomogram using the “regplot” function.
Statistical analysis
All analyses were conducted using R software (version 4.1.1). The Chi-squared test was employed to evaluate differences in categorical variables. Variables that showed significant differences (P<0.05) during the univariate analysis were incorporated into a multivariate logistic regression analysis to determine independent risk factors for lymphatic metastasis. A nomogram was utilized to assess individual risk for LM, and its ability to discriminate was measured by the area under the receiver operating characteristic (ROC) curve (AUC). The predictive nomogram’s accuracy and clinical relevance were examined through calibration and decision curve analyses, respectively. A significance level of P<0.05 was established for all observed differences.
Results
Baseline characteristics of the study population
Among the 4,890 patients analyzed for early-onset colon cancer diagnosis, 900 (18.4%) had LM, whereas 3,990 (81.6%) did not. The training and validation sets showed no significant differences in baseline characteristics, such as age, sex, race, histological type, T stage, N stage, tumor size, tumor location, CEA level, and LM (Table 1), as confirmed using the Chi-squared test (all P>0.05). Further analysis revealed that 643 (18.8%) of the 3,423 patients and 257 (17.5%) of the 1,467 patients in the training and validation sets, respectively, presented with LM. There was no statistically significant difference in the LM rates between the training and validation sets (P=0.31).
Univariate and multivariate analyses of risk factors for LM in patients with early-onset colon cancer
Univariate logistic analysis revealed that histological type, T stage, N stage, tumor location, and CEA level were correlated with LM in patients with early-onset colon cancer (all P<0.05). Conversely, age, sex, race, and tumor size were not associated with LM (all P>0.05) (Table 2). The multivariate analysis subsequently included significant factors identified in the univariate analysis. The results indicated that histological type, T stage, N stage, and CEA level were independent risk factors for LM in patients with early-onset colon cancer (all P<0.05) (Table 2).
Table 2
Characteristics | Univariate analysis | Multivariate analysis | |||||
---|---|---|---|---|---|---|---|
OR | 95% CI | P | OR | 95% CI | P | ||
Age | |||||||
<40 years | Reference | ||||||
40–50 years | 1.067 | 0.904–1.262 | 0.45 | ||||
Sex | |||||||
Female | Reference | ||||||
Male | 0.988 | 0.855–1.142 | 0.87 | ||||
Race | |||||||
Black | Reference | ||||||
Others | 0.863 | 0.658–1.129 | 0.29 | ||||
White | 0.839 | 0.693–1.021 | 0.08 | ||||
Histological type | |||||||
Adenocarcinoma | Reference | Reference | |||||
Others | 0.603 | 0.498–0.725 | <0.001 | 0.593 | 0.479–0.731 | <0.001 | |
T stage | |||||||
T1 | Reference | Reference | |||||
T2 | 0.419 | 0.261–0.667 | <0.001 | 0.312 | 0.185–0.521 | <0.001 | |
T3 | 1.083 | 0.789–1.517 | 0.63 | 0.358 | 0.245–0.530 | <0.001 | |
T4 | 2.000 | 1.442–2.828 | <0.001 | 0.493 | 0.332–0.740 | <0.001 | |
N stage | |||||||
N0 | Reference | Reference | |||||
N1 | 2.469 | 2.029–3.012 | <0.001 | 2.144 | 1.726–2.671 | <0.001 | |
N2 | 4.709 | 3.899–5.706 | <0.001 | 3.734 | 3.011–4.645 | <0.001 | |
Tumor size | |||||||
≤50 mm | Reference | ||||||
>50 mm | 1.118 | 0.967–1.292 | 0.13 | ||||
Tumor location | |||||||
Ascending colon | Reference | Reference | |||||
Cecum | 1.130 | 0.860–1.487 | 0.38 | 1.053 | 0.776–1.431 | 0.74 | |
Descending colon | 1.300 | 0.961–1.755 | 0.09 | 1.290 | 0.920–1.805 | 0.14 | |
Hepatic flexure | 1.107 | 0.730–1.648 | 0.62 | 1.223 | 0.770–1.915 | 0.39 | |
Sigmoid colon | 1.394 | 1.114–1.757 | 0.004 | 1.223 | 0.936–1.559 | 0.15 | |
Splenic flexure | 1.379 | 0.916–2.044 | 0.12 | 1.163 | 0.739–1.807 | 0.51 | |
Transverse colon | 1.011 | 0.734–1.386 | 0.95 | 0.954 | 0.670–1.354 | 0.79 | |
CEA | |||||||
Negative | Reference | Reference | |||||
Positive | 9.421 | 7.845–11.379 | <0.001 | 8.342 | 6.902–10.140 | <0.001 |
OR, odds ratio; CI, confidence interval; CEA, carcinoembryonic antigen.
Development and validation of a prediction model for LM in patients with early-onset colon cancer
The diagnostic prediction model was developed using independent risk factors, such as histological type, T stage, N stage, and CEA level. These factors were used to create a nomogram for graphical presentation (Figure 2). Each risk factor was assigned a corresponding score based on patient-specific information, and the total score was calculated by summing all risk factors. The total score was used to predict the probabilistic risk of LM in each patient by projecting it onto the corresponding risk axis of LM. The ROC curves of the prediction model for diagnosis were plotted using the R software, resulting in AUCs of 0.812 [95% confidence interval (CI): 0.795–0.829] and 0.809 (95% CI: 0.784–0.834) for the training and validation sets, respectively. This indicated that the prediction model had high diagnostic and discriminatory power, with a C-index of 0.812 and 0.809 for the training and validation sets, respectively (Figure 3A,3B).
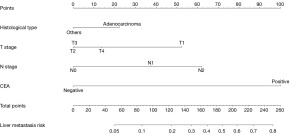
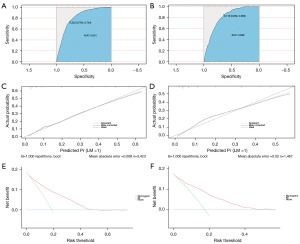
The calibration curves for both the training and validation sets revealed a strong correlation between the observed and predicted probabilities (see Figure 3C,3D). The decision curve analysis indicated that the diagnostic prediction model created in this study possesses significant clinical utility, confirming that the nomogram serves as an effective tool for assessing LM risk in patients with early-onset colon cancer (Figure 3E,3F).
Discussion
In recent decades, there has been an approximately two-fold increase in the incidence of early-onset colon cancer since the early 1990s (3,4), drawing increased attention to this condition. Based on previous studies, LM of colon cancer is more prevalent than metastases at other sites, such as the brain or bone (15,16). LM is a significant contributor to poor outcomes in colon cancer; therefore, accurately predicting its occurrence in early-onset cases is crucial for guiding clinical decision-making. Previous studies have investigated prognostic factors and developed prediction models for patients with early-onset colon cancer; however, there is limited information available regarding the risk factors associated with developing LM in this patient population (7,9,17-20). We developed a predictive nomogram to quantitatively assess the risk of developing LM in patients with early-onset colon cancer. This tool can assist clinicians in making more informed and personalized treatment decisions for individual patients, thereby reducing unnecessary examination expenses.
In this study, we identified independent risk factors, including histological type, T stage, N stage, and CEA levels. Furthermore, a predictive nomogram was developed for patients with early-onset colon cancer and LM based on these risk factors, and it demonstrated an excellent discriminatory ability. However, these findings are not entirely consistent with those of previous research (8,21-23), as none of the histological types were identified as significant independent risk factors in those studies. This discrepancy could be due to the differing categorizations of subgroups within the histological type between our study and previous studies. Notably, in our research, CEA was the primary risk factor associated with the presence of LM, followed by N stage, consistent with the findings of a previous report (21). This finding was not unexpected, given that previous research indicates that CEA may facilitate the development of LM (24). Based on the odds ratio values, T2, T3, and T4 also appeared to be protective factors; however, according to the nomogram, there was a significant association between T4/T1 and LM development. These findings are consistent with those of a previous study (23). This phenomenon may be due to the route of metastasis of colon cancer to the liver, as the drainage of the colon veins into the portal vein system facilitates their subsequent reach to the liver, thus rendering such progression plausible. However, additional research such as modeling with larger populations or molecular-level studies is necessary to clarify the underlying mechanisms governing this process. In addition, our study revealed that within the cohort of 4,890 patients assessed for early-onset colon cancer, 900 (18.4%) were identified as having LM. This is higher than the results of previous studies that focused on creating a predictive model for LM in individuals with CRC (21-23). This suggests a potentially increased susceptibility to LM in individuals with early-onset colon cancer and that distant metastasis is more prevalent in these patients than in those with late-onset colon cancer (25,26). This emphasizes the need for prospective studies involving larger cohorts and additional molecular-level investigations to validate our findings.
There are some limitations in this study. First, the validation set was derived from the same SEER dataset as that for the training set, which could have led to spillover effects in the model. Second, as the SEER database only records synchronous LM and does not record cases that develop LM later in the disease course, the real incidence rate of total LM might have been underestimated. Third, the SEER program included only the US population; therefore, the prediction model in this study might be unsuitable for populations in other countries and regions. Finally, the research population and study factors included in this study were insufficient. Crucial factors, such as gene status, which may further improve the effectiveness of the nomogram, were not included. Therefore, external validation should be performed in future studies using patient data from localized cohorts, including clinical and pathological information.
Conclusions
This study demonstrated that the prevalence of LM in patients with early-onset colon cancer was 18.4% and identified the risk factors for LM development in this population. Based on these findings, a nomogram was developed to predict the risk of LM in patients with early-onset colon cancer, which demonstrated good calibration and discrimination. This nomogram can help clinicians make personalized predictions and provide specific treatment recommendations for patients.
Acknowledgments
None.
Footnote
Reporting Checklist: The authors have completed the TRIPOD reporting checklist. Available at https://tcr.amegroups.com/article/view/10.21037/tcr-24-1159/rc
Peer Review File: Available at https://tcr.amegroups.com/article/view/10.21037/tcr-24-1159/prf
Funding: This work was supported by grants from
Conflicts of Interest: All authors have completed the ICMJE uniform disclosure form (available at https://tcr.amegroups.com/article/view/10.21037/tcr-24-1159/coif). The authors have no conflicts of interest to declare.
Ethical Statement: The authors are accountable for all aspects of the work in ensuring that questions related to the accuracy or integrity of any part of the work are appropriately investigated and resolved
Open Access Statement: This is an Open Access article distributed in accordance with the Creative Commons Attribution-NonCommercial-NoDerivs 4.0 International License (CC BY-NC-ND 4.0), which permits the non-commercial replication and distribution of the article with the strict proviso that no changes or edits are made and the original work is properly cited (including links to both the formal publication through the relevant DOI and the license). See: https://creativecommons.org/licenses/by-nc-nd/4.0/.
References
- Bray F, Laversanne M, Sung H, et al. Global cancer statistics 2022: GLOBOCAN estimates of incidence and mortality worldwide for 36 cancers in 185 countries. CA Cancer J Clin 2024;74:229-63. [Crossref] [PubMed]
- Patel SG, Karlitz JJ, Yen T, et al. The rising tide of early-onset colorectal cancer: a comprehensive review of epidemiology, clinical features, biology, risk factors, prevention, and early detection. Lancet Gastroenterol Hepatol 2022;7:262-74. [Crossref] [PubMed]
- Montminy EM, Zhou M, Maniscalco L, et al. Contributions of Adenocarcinoma and Carcinoid Tumors to Early-Onset Colorectal Cancer Incidence Rates in the United States. Ann Intern Med 2021;174:157-66. [Crossref] [PubMed]
- Stoffel EM, Murphy CC. Epidemiology and Mechanisms of the Increasing Incidence of Colon and Rectal Cancers in Young Adults. Gastroenterology 2020;158:341-53. [Crossref] [PubMed]
- Reboux N, Jooste V, Goungounga J, et al. Incidence and Survival in Synchronous and Metachronous Liver Metastases From Colorectal Cancer. JAMA Netw Open 2022;5:e2236666. [Crossref] [PubMed]
- Ding Y, Han X, Zhao S, et al. Constructing a prognostic model for colorectal cancer with synchronous liver metastases after preoperative chemotherapy: a study based on SEER and an external validation cohort. Clin Transl Oncol 2024;26:3169-90. [Crossref] [PubMed]
- Wu J, Lu L, Chen H, et al. Prognostic nomogram to predict the overall survival of patients with early-onset colorectal cancer: a population-based analysis. Int J Colorectal Dis 2021;36:1981-93. [Crossref] [PubMed]
- E Y. Prediction of liver and lung metastases in patients with early-onset colorectal cancer by nomograms based on heterogeneous and homogenous risk factors. Cancer Med 2023;12:20712-26. [Crossref] [PubMed]
- Ding X, Yang X, Wu D, et al. Nomogram predicting the cancer-specific survival of early-onset colorectal cancer patients with synchronous liver metastasis: a population-based study. Int J Colorectal Dis 2022;37:1309-19. [Crossref] [PubMed]
- Liu Y, Sun Z, Guo Y, et al. Construction and validation of a nomogram of risk factors and cancer-specific survival prognosis for combined lymphatic metastases in patients with early-onset colorectal cancer. Int J Colorectal Dis 2023;38:128. [Crossref] [PubMed]
- Blum KA, Keller FG, Castellino S, et al. Incidence and outcomes of lymphoid malignancies in adolescent and young adult patients in the United States. Br J Haematol 2018;183:385-99. [Crossref] [PubMed]
- Huang L, Jansen L, Balavarca Y, et al. Resection of pancreatic cancer in Europe and USA: an international large-scale study highlighting large variations. Gut 2019;68:130-9. [Crossref] [PubMed]
- Warren JL, Klabunde CN, Schrag D, et al. Overview of the SEER-Medicare data: content, research applications, and generalizability to the United States elderly population. Med Care 2002;40:IV-3-18. [Crossref] [PubMed]
- Chen B, Zeng Y, Liu B, et al. Risk Factors, Prognostic Factors, and Nomograms for Distant Metastasis in Patients With Newly Diagnosed Osteosarcoma: A Population-Based Study. Front Endocrinol (Lausanne) 2021;12:672024. [Crossref] [PubMed]
- Hackl C, Neumann P, Gerken M, et al. Treatment of colorectal liver metastases in Germany: a ten-year population-based analysis of 5772 cases of primary colorectal adenocarcinoma. BMC Cancer 2014;14:810. [Crossref] [PubMed]
- Lei S, Ge Y, Tian S, et al. Colorectal Cancer Metastases to Brain or Bone and the Relationship to Primary Tumor Location: a Population-Based Study. J Gastrointest Surg 2020;24:1833-42. [Crossref] [PubMed]
- Li D. Establishment and validation of a prognostic nomogram for patients with early-onset stage I-II colon cancer. World J Surg Oncol 2023;21:103. [Crossref] [PubMed]
- Li Y, Liu W, Zhou Z, et al. Development and validation of prognostic nomograms for early-onset locally advanced colon cancer. Aging (Albany NY) 2020;13:477-92. [Crossref] [PubMed]
- Liu J, Liu Z, Li J, et al. Personalizing prognostic prediction in early-onset Colorectal Cancer. J Cancer 2020;11:6727-36. [Crossref] [PubMed]
- Yu C, Zhang Y. Development and validation of a prognostic nomogram for early-onset colon cancer. Biosci Rep 2019;39:BSR20181781. [Crossref] [PubMed]
- Gaitanidis A, Machairas N, Alevizakos M, et al. Predictive Nomograms for Synchronous Liver and Lung Metastasis in Colon Cancer. J Gastrointest Cancer 2020;51:925-31. [Crossref] [PubMed]
- Liu Y, Wang Y, Zhang H, et al. Nomogram for predicting occurrence of synchronous liver metastasis in colorectal cancer: a single-center retrospective study based on pathological factors. World J Surg Oncol 2022;20:39. [Crossref] [PubMed]
- Tang M, Wang H, Cao Y, et al. Nomogram for predicting occurrence and prognosis of liver metastasis in colorectal cancer: a population-based study. Int J Colorectal Dis 2021;36:271-82. [Crossref] [PubMed]
- Lee JH, Lee SW. The Roles of Carcinoembryonic Antigen in Liver Metastasis and Therapeutic Approaches. Gastroenterol Res Pract 2017;2017:7521987. [Crossref] [PubMed]
- Himbert C, Figueiredo JC, Shibata D, et al. Clinical Characteristics and Outcomes of Colorectal Cancer in the ColoCare Study: Differences by Age of Onset. Cancers (Basel) 2021;13:3817. [Crossref] [PubMed]
- Watson R, Liu TC, Ruzinova MB. High frequency of KRAS mutation in early onset colorectal adenocarcinoma: implications for pathogenesis. Hum Pathol 2016;56:163-70. [Crossref] [PubMed]