Development and validation of a prognostic model based on disulfidptosis-related ferroptosis genes: DRD4 and SLC2A3 as biomarkers for predicting prognosis in colon cancer
Highlight box
Key findings
• This study developed a prognostic model for colon cancer (CC) based on disulfidptosis-related ferroptosis genes (DRFGs). The model performed well in predicting the prognosis of patients with CC. The important roles of the model genes DRD4 and SLC2A3 in CC were analyzed in terms of clinical features and immune characteristics. The model was validated using clinical samples to improve the utility of this study.
What is known and what is new?
• Disulfidptosis and ferroptosis are programmed cell death modalities that play an important role in tumor development.
• This study established a DRFGs-related prognostic model that can predict the prognosis of patients with CC and guide clinical treatment.
What is the implication, and what should change now?
• This study constructed and validated a prognostic model for CC based on DRFGs and systematically analyzed its value in clinical applications. Further studies remain warranted to explore the regulatory mechanisms of DRD4 and SLC2A3 in CC.
Introduction
Colon cancer (CC) accounts for approximately 6% of all cancers diagnosed globally each year, with over 114,000 new cases and 570,000 deaths reported worldwide in 2020 alone (1). As a major health challenge, CC poses a substantial challenge for patients and healthcare systems globally. More concerning is the increasing trend of younger individuals being diagnosed with CC, with a growing occurrence observed in those under the age of 50 years (2,3). With the advancements in medicine, the standard treatment approach for CC includes targeted therapy, chemotherapy, radiotherapy, and surgery (4). While surgical resection is preferred by most patients with stages I and II CC, approximately 49% of those with stage IV opt for a combination therapy plan involving surgery along with radiotherapy or chemotherapy to reduce the risk of recurrence (5). However, chemoresistance in CC cells typically impedes the efficacy of therapy (6). In addition to resistance commonly observed with conventional chemotherapeutic agents, such as 5-fluorouracil, oxaliplatin, and doxorubicin, resistance to targeted agents, such as bevacizumab and cetuximab, is also encountered (7,8). Therefore, it is important to identify new prognosis-related signatures and potential treatment targets that can provide more options for the clinical management of CC.
Ferroptosis, an iron-based form of programmed cell death (PCD) characterized by excessive accumulation of reactive oxygen species (ROS) (9), plays a multifaceted role in tumor proliferation and invasion, as well as in therapeutic interventions (10,11). Ferroptosis-related biomarkers possess prognostic capabilities for some tumor types, including hepatocellular carcinoma, thyroid cancer, prostate cancer, and lung adenocarcinoma (12-15). SLC7A11 is a vital solute carrier transporter protein that exerts influence during ferroptosis. SLC7A11 overexpression in cancer cells promotes tumor growth by inhibiting ferroptosis through enhanced cystine uptake (16). In contrast, Liu et al. found that cells expressing high levels of SLC7A11 could undergo a distinct form of cell death, known as disulfidptosis, during glucose starvation (17).
Disulfidptosis is PCD associated with disulfide overaccumulation (18). A study has shown that aberrant accumulation of cystine disulfides may cause disulfide stress, causing significant damage to cellular activity (19). NADPH produced through the pentose phosphate pathway can reduce cystine to cysteine, thereby aiding cell viability; however, during glucose starvation, the balance of this redox reaction is disrupted. Disulfide stress occurs when intracellular NADPH is depleted in large quantities, leading to the disruption of the actin network and allowing disulfidptosis to occur. Recent promising findings regarding disulfidptosis as a new mechanism of cell death provide further insights into tumor treatment (20,21). It has been shown that genes associated with disulfidptosis are likely to serve as prognostic markers for CC (22). Furthermore, studies indicate that the ferroptosis mechanism may have a modulatory influence on CC in therapy (23,24). However, there are presently few studies investigating the effects of combined disulfidptosis and ferroptosis on CC.
CC is one of the leading causes of cancer-related deaths worldwide, with approximately 1.8 million new cases and 900,000 deaths annually. Given its high incidence and heterogeneity, accurate prognosis prediction and selection of appropriate treatment strategies are critical for improving patient outcomes. Over the past few decades, several biomarkers and prognostic models have been proposed to better stratify patients based on their risk profiles. Among the well-established prognostic biomarkers in CC are KRAS, BRAF, and TP53 mutations, as well as microsatellite instability (MSI) and chromosomal instability (CIN). These biomarkers play significant roles in tumorigenesis and have been used clinically to predict patient outcomes and guide therapy. For instance, KRAS mutations are present in approximately 40% of CC cases and are associated with resistance to anti-EGFR therapies such as cetuximab and panitumumab (25). However, despite its predictive value in guiding targeted therapies, the presence of KRAS mutations alone does not fully explain variations in survival among patients, limiting its utility as a standalone prognostic marker (26). Similarly, BRAF mutations, particularly the V600E variant, occur in around 8–12% of CC patients and are associated with poor prognosis and reduced overall survival (OS) (27). Nonetheless, the occurrence of BRAF mutations is relatively low, limiting its applicability across the broader CC population. MSI-high status, which is found in about 15% of CC patients, is linked to a favorable response to immunotherapy and a better overall prognosis, particularly in early-stage disease (28). However, MSI-high tumors are less common in advanced stages of CC, further limiting the broad application of this marker (29). While these biomarkers offer insights into the molecular underpinnings of CC, their predictive accuracy and generalizability across different patient subsets remain limited. Furthermore, current clinical applications often rely on a combination of these biomarkers, yet even in combination, they may not capture the full complexity of tumor biology, especially the role of the tumor microenvironment (TME) and metabolic alterations.
In addition to individual biomarkers, various prognostic models have been developed to improve patient stratification. One of the most notable is the Consensus Molecular Subtypes (CMS) classification, which categorizes CC into four distinct subtypes based on transcriptomic profiling: CMS1 (immune), CMS2 (canonical), CMS3 (metabolic), and CMS4 (mesenchymal) (30). Each subtype is associated with different clinical outcomes, with CMS1 exhibiting better responses to immunotherapy and CMS4 associated with worse OS due to its invasive and aggressive nature (31). While the CMS model offers a more nuanced approach to patient classification, it requires complex molecular testing and lacks consideration of the dynamic interplay between tumor metabolism and the immune microenvironment, which are increasingly recognized as critical factors influencing prognosis and treatment response. Another challenge with existing models is the focus on static genetic alterations, such as mutations or copy number variations, which do not fully account for the metabolic reprogramming or immune evasion strategies employed by tumors during progression. Therefore, integrating biomarkers that reflect both genetic and functional changes in cancer cells is crucial for enhancing the predictive power of prognostic models.
Emerging evidence suggests that the incorporation of genes related to novel cell death pathways, such as disulfidptosis and ferroptosis, may provide more accurate and comprehensive prognostic information. Disulfidptosis and ferroptosis are forms of regulated cell death that are triggered by oxidative stress and disulfide accumulation, processes closely linked to cancer metabolism and immune evasion (32). Genes involved in these pathways, such as DRD4 (dopamine receptor D4) and SLC2A3 (solute carrier family 2, member 3), have shown potential as prognostic biomarkers due to their roles in regulating key biological processes in cancer progression. DRD4 is a G-protein-coupled receptor involved in modulating cancer cell proliferation, migration, and apoptosis. Recent studies have demonstrated that DRD4 expression is upregulated in various cancers, including CC, and is associated with worse clinical outcomes (33,34). Inhibition of DRD4 has been shown to suppress tumor growth and enhance the sensitivity of cancer cells to apoptosis-inducing agents, suggesting its potential as a therapeutic target (35). SLC2A3, also known as GLUT3, is a glucose transporter that facilitates glucose uptake in tumor cells, particularly under hypoxic conditions. This transporter plays a crucial role in maintaining the high glycolytic rates characteristic of cancer cells, a phenomenon known as the Warburg effect (36). Elevated SLC2A3 expression has been correlated with increased tumor aggressiveness, poor prognosis, and resistance to chemotherapy in multiple cancers, including CC (37). The importance of SLC2A3 in cancer metabolism makes it a promising biomarker for prognosis, especially in metabolic reprogramming-driven tumors. By incorporating DRD4 and SLC2A3 into a prognostic model, we aim to capture both the proliferative and metabolic dimensions of tumor biology, providing a more comprehensive tool for patient stratification. High expression of these genes is indicative of poor prognosis, advanced clinical stage, and increased resistance to standard therapies. Therefore, the development of a DRD4 and SLC2A3-based prognostic model could offer enhanced accuracy in predicting patient outcomes and guiding personalized treatment strategies in CC.
The present study systematically investigated the effects of disulfidptosis-related ferroptosis genes (DRFGs) expression levels on the prognosis, immune microenvironment, and drug sensitivity of patients with CC, and established and verified a model for CC prognosis associated with DRFGs, specifically DRD4 and SLC2A3. DRD4 and SLC2A3 are potential targets for CC therapy. This study also investigated how the expression of these genes may be associated with the prognosis of patients with CC. We present this article in accordance with the TRIPOD reporting checklist (available at https://tcr.amegroups.com/article/view/10.21037/tcr-24-1177/rc).
Methods
Sources and collation of data
Transcriptomic data from 476 CC samples and 41 normal colon samples were acquired from The Cancer Genome Atlas (TCGA) (https://portal.gdc.cancer.gov/) database. Clinical data on 452 CC patients were similarly obtained from the TCGA database. These clinical data included patient age, gender, pathological stage, and survival data. After excluding patients with incomplete information regarding survival status and survival time, a total of 448 patients with CC were enrolled and randomly allocated to the training or testing groups.
The datasets GSE17538 (n=213) and GSE38832 (n=162) were obtained from the Gene Expression Omnibus (GEO) database as external validation datasets. We removed samples with incomplete clinical data and compiled a list disulfidptosis-related genes from relevant literature (17,19); genes associated with ferroptosis were collected from the FerrDb database (38). Pearson correlation analyses were performed with P value <0.05 and |Cor| >0.3 as filtering criteria; 196 DRFGs were obtained. The “limma” R package was utilized to examine DRFGs with differential expression between normal colon and CC tissues (39).
The primary prognosis outcomes considered were OS and progression-free survival (PFS). OS was defined as the time from the date of diagnosis to the date of death from any cause. PFS was defined as the time from diagnosis to the first occurrence of disease progression or recurrence, or to death if no progression was recorded. For patients without these events, the data were censored at the last follow-up. Kaplan-Meier survival analysis and the log-rank test were used to compare survival between high-risk (HR) and low-risk (LR) groups, while Cox proportional hazards regression was employed to estimate the hazard ratios for OS and PFS.
Protein-protein interaction (PPI) network
The STRING (https://cn.string-db.org/) database was utilized for the analysis of the PPI network of DRFGs. In this PPI network, genes with a confidence level >0.9 were displayed, and genes that did not interact were hidden.
Construction and validation of a prognostic model related to DRFGs
The prognostic model was constructed using the training group samples and tested for accuracy with the testing group. First, univariate Cox regression analyses were applied to identify DRFGs associated with prognosis. Subsequently, the least absolute shrinkage and selection operator (LASSO) regression analyses were used to prevent overfitting. LASSO, a regression analysis method that improves prediction accuracy by penalizing the absolute size of the regression coefficients, was used to avoid overfitting and select significant predictors for the prognostic model. Finally, multifactorial Cox regression analyses were used to output risk score formulas to construct prognostic models. The risk-scoring formula was as follows:
where exp(DRFGs) represents the normalized expression levels of DRD4 and SLC2A3, and coef(DRFGs) represents the regression coefficients of these two model genes.
According to the risk score, we classified the patients with CC into HR and LR groups and used principal component analysis (PCA) to assess the discriminatory power of the risk score. The characteristics of the HR and LR groups in terms of OS and PFS were evaluated using Kaplan-Meier curves. The accuracy of this prognostic model was evaluated using receiver operating characteristic (ROC) curves. We combined the risk score with the clinical traits of the patients by creating a nomogram, which allowed us to assess the 1-, 3-, and 5-year survival of patients with CC.
In constructing the nomogram, we first conducted univariate Cox regression analysis to identify genes significantly associated with OS in CC patients. Genes with a P value less than 0.05 were selected as potential candidates for further analysis. To refine this list and prevent overfitting, we applied the LASSO Cox regression. LASSO helps in selecting the most relevant predictors by shrinking the coefficients of less important variables to zero. This process identified DRD4 and SLC2A3 as the most significant prognostic genes. Next, we incorporated DRD4 and SLC2A3 into a multivariate Cox proportional hazards model, along with clinical factors such as age, gender, and tumor stage, which were also independently assessed for their prognostic value. The regression coefficients generated from this multivariate analysis were used to build the nomogram. The nomogram provides a visual representation of the contribution of each predictor, where each variable (gene expression and clinical factors) is assigned a score. The total score for an individual patient is used to estimate their 1-, 3-, and 5-year OS probabilities.
The predictive performance of the nomogram was evaluated using calibration curves, which compared predicted survival probabilities with actual outcomes. Additionally, the model’s discrimination ability was assessed using the concordance index (C-index), which achieved a value of 0.75, indicating a strong ability to predict patient outcomes. To further validate the robustness of the model, it was externally tested using independent cohorts from the GEO database, confirming its predictive accuracy.
Biological characteristic enrichment analysis
Using the “clusterProfiler” R package (40), we conducted Gene Ontology (GO) and Kyoto Encyclopedia of Genes and Genomes (KEGG) analysis of differentially expressed genes (DEGs) between HR and LR groups. The biological functions of the risk groups were examined through gene set enrichment analysis (GSEA) (41).
TME analysis and immune cell infiltration analysis
The TME characteristics of patients with CC were assessed using the “ESTIMATE” R package (42) and the abundance of immune cells in both risk groups was evaluated using the CIBERSORT algorithm (43).
Somatic mutation analysis and drug sensitivity analysis
Somatic mutation data for patients with CC were obtained from the TCGA database. The visualization maps of somatic mutation characteristics of the HR and LR groups were created using the “maftools” R package (44). Using the “pRRophetic” R package, analysis was conducted on half maximal inhibitory concentrations (IC50) of various chemical drugs in the HR and LR groups.
Single-cell RNA sequencing (scRNA-seq) analysis
The expression and distribution of genes in the prognostic model were analyzed at the single-cell level using the Tumor Immune Single-cell Hub 2 (TISCH2, http://tisch.comp-genomics.org/) website (45). Using this website, we analyzed the GSE166555 dataset and visualized the expression and distribution of model genes using t-distributed stochastic neighbor embedding (t-SNE) and violin plots.
External validation
External validation of the prognostic model was performed using independent validation cohorts GSE17538 and GSE38832. The discriminatory and predictive power of the model was validated in the independent cohorts using PCA and ROC curves. The characteristics of the HR and LR groups in terms of OS and disease-specific survival (DSS) were also assessed using Kaplan-Meier curves.
Clinical tissue samples and immunohistochemistry
Six CC samples and paired paracancerous tissues were collected from patients undergoing surgical treatment at the Affiliated Hospital of Nanjing University of Traditional Chinese Medicine in Nanjing, China, from May 2022 to December 2023. A microtome was used to cut 5-µm-thick sections of embedded colon tissues. Tissue sections were soaked in xylene for 15 min three times to dewax, hydrated using graded levels of ethanol (100%, 90%, 80%, and 70%), and placed in a sodium citrate antigen retrieval solution for antigen repair using the microwave heating method. For endogenous peroxidase blocking, sections were incubated with 3% hydrogen peroxide for 25 min at room temperature, protected from light. Serum closure was performed using 5% bovine serum albumin to evenly cover the tissue and incubated for 30 min at room temperature. Sections were incubated overnight at 4 ℃ with DRD4 (1:500, TD4866M, Abmart, Shanghai, China) or SLC2A3 (1:200, CSB-PA868995, Cusabio, Wuhan, China) antibodies. After removing the excess primary antibody, the tissue sections were incubated with the corresponding secondary antibody for 1 h. The study was conducted in accordance with the Declaration of Helsinki (as revised in 2013). The study was approved by the Ethics Committee of Affiliated Hospital of Nanjing University of Chinese Medicine (No. 2022NL-KS086) and individual consent for this retrospective analysis was waived.
As secondary antibodies, the Goat anti-Rabbit IgG UltraPolymer antibody (GU101019, Abmart, Shanghai, China) was used for both DRD4 and SLC2A3 antigens. Diaminobenzidine was used to develop the color before hematoxylin (H9627; Sigma-Aldrich, MO, USA) re-staining. Finally, the results were statistically analyzed using ImageJ software (version 1.51).
Statistical analysis
Data were analyzed using the R software (version 4.22). A paired t-test was used to compare the protein expression levels of DRD4 and SLC2A3 in CC tissues and paired paracancerous tissues. P<0.05 was considered statistically significant.
Results
Confirmation of DRFGs
Through Pearson correlation analysis, we obtained 196 DRFGs from the CC transcriptome data from the TCGA database. Figure 1A shows the PPI network, illustrating the interconnections between the proteins encoded by these genes. In total, 95 differentially expressed DRFGs were discovered between normal and tumorous CC tissues (Figure 1B,1C).
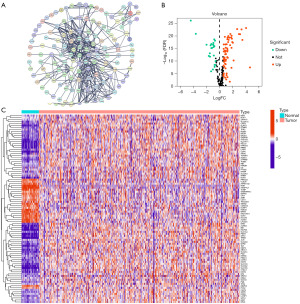
Building of prognostic models
Using univariate Cox analysis, we obtained four DRFGs associated with CC prognosis (Figure 2A). We then performed LASSO analysis (Figure 2B,2C) and constructed a two-gene risk score model via multifactor Cox analysis. The following model was achieved: risk score for each patient = (0.6445 × DRD4 expression level) + (0.2795 × SLC2A3 expression level). Through PCA, we determined that the risk model exhibited a more pronounced discriminatory ability in distinguishing between patients in the HR and LR groups than the DRFGs (Figure 2D,2E). In the training group, the HR group had a significantly lower chance of survival than the LR group (Figure 2F, P=0.003); the testing group also demonstrated this trend (Figure 2G, P=0.004). The HR group exhibited significantly poorer PFS than the LR group (Figure 2H, P<0.001).
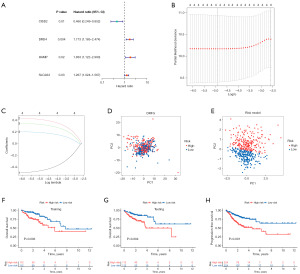
Evaluation of the DRFG-based prediction model for CC
Patients diagnosed with CC were segregated into two different groups, HR and LR, based on their median risk score. Heatmaps showed that in both training and testing groups, DRD4 and SLC2A3 were highly expressed in the HR group (Figure 3A,3B). The mortality rate exhibited an upward trend as the risk score escalated within the training group (Figure 3C,3D). The ROC curves demonstrated a commendable level of precision in forecasting survival outcomes for the training group, as confirmed by the area under the curve values at 1, 3, and 5 years (Figure 3E). Additionally, similar to that in the training group, the mortality rate was higher in the HR group in the testing group than in the LR group (Figure 3F,3G). The model also provided satisfactory precision in predicting survival in the testing group (Figure 3H).
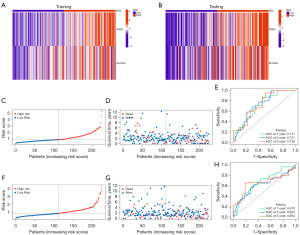
Independent prognostic factor analysis and nomogram development
To determine the independent prognostic significance of the DRFG-based risk score in CC, univariate and multivariate Cox regression analyses were performed. Leads from univariate (HR =1.693, P<0.001) and multivariate (HR =1.332, P=0.01) regression analyses showed that risk scores were relevant to the prognostic situation and could serve as an independent prognostic element (Figure 4A,4B). Subsequently, we established a nomogram incorporating gender, age, staging, and risk scores to forecast 1-, 3-, and 5-year survival in patients with CC (Figure 4C). Calibrated graphs show the excellent performance of the nomogram in predicting patient survival, with the highest accuracy for 1-year survival (Figure 4D). To assess the prognostic significance of the DRFG-based prognostic model at different stages of CC, we performed survival analyses of patients at stages I–II and III–IV. Survival analyses were also conducted to assess the prognosis of the HR and LR groups at various stages and, as previously hypothesized, those in the HR group had a substantially worse prognosis at both early and late stages (stages I–II, P=0.03; stages III–IV, P=0.006; Figure 4E,4F).
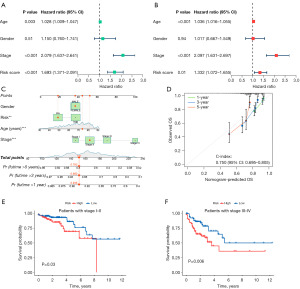
Biological characteristics enrichment analyses
To elucidate the heterogeneity of biological characteristics between different risk groups, GO and KEGG enrichment analyses were applied to the DEGs between HR and LR groups. In GO analysis, these DEGs were highly enriched for extracellular matrix (ECM)-associated cellular structures, endoplasmic reticulum lumen, and binding of glycosaminoglycans, sulfur compounds, heparin, and other components (Figure 5A). These DEGs were primarily related to the PI3K-Akt signaling pathway, PPAR signaling pathway, and other pathways, as identified using KEGG enrichment analysis (Figure 5B). In addition, GSEA showed that genes in samples from the HR group were predominantly enriched in pathways associated with cell adhesion molecules, cytokine-cytokine receptor interaction, ECM receptor interaction, focal pathways related to adhesion, and neuroactive ligand-receptor interaction. Genes in the samples from the LR group were significantly enriched for pathways related to drug metabolism, other enzymes, pentose and glucuronate interconversions, peroxisome, retinol metabolism, and ribosomes (Figure 5C,5D).
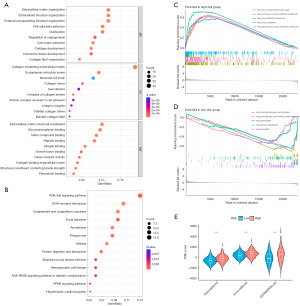
Evaluation of DRFGs in TME and immune infiltrates
The HR group had higher SromalScore, ImmuneScore, and ESTIMATEScore than the HR group (Figure 5E). Immune infiltration analysis was performed to investigate differences in TME status between HR and LR groups. The expression of memory CD4 T-cells was high in the LR group; however, T-cells regulatory (Tregs) and M0 macrophages were present at high levels in the HR group (Figure 6A). Additionally, the HR group scored significantly high on human leukocyte antigen (HLA), macrophages, T-cell co-stimulation, T-helper cells, and tumor infiltrating lymphocytes (TIL) (Figure 6B). We used waterfall plots to visually demonstrate somatic mutations in HR and LR groups; the probability of gene mutation was not significantly different between the two groups. In both groups, APC had the highest mutation frequency (71%) (Figure 6C,6D).
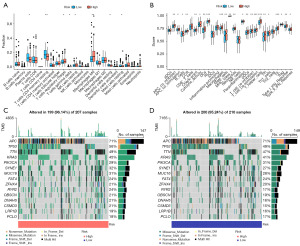
Drug sensitivity analysis
We used IC50 to assess the sensitivity of eight common chemotherapeutic agents used to treat CC, including AG-014699, foretinib, PF-4708671, and PI-103. The HR group exhibited low IC50 values for all eight classes of drugs, implying that they were more sensitive to these drugs than the LR group (Figure S1A). Furthermore, the IC50 values for all eight chemotherapeutic agents were negatively correlated with the risk scores in the prognostic model (Figure S1B).
Analysis of single-cell sequencing
The scRNA-seq data from GSE166555 were analyzed using the TISCH2 database to determine the expression of DRD4 and SLC2A3. Figure 7A shows a total of 13 cell types and their respective occupancies. We annotated all 13 cell types (Figure 7B). The distribution of DRD4 is shown in Figure 7C,7D. SLC2A3 was more frequently distributed in the B, CD4 conventional T cell (Tconv), CD8 T, dendritic cell (DC), endothelial, fibroblasts, mast, monocytes/macrophages, myofibroblasts, and proliferating T cell (Tprolif) cell types (Figure 7E,7F).
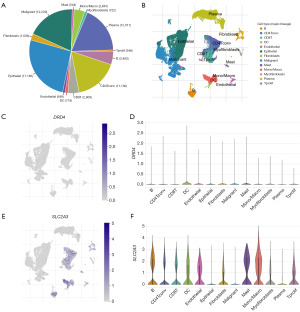
Clinical characteristics of DRD4 and SLC2A3 in CC
We investigated the effects of DRD4 and SLC2A3 expression on prognostic survival. Survival curves revealed that as the expression of these two genes increased, patients with CC exhibited a low chance of survival (Figure 8A,8B). Analysis of immune cell infiltration in high and low DRD4 and SLC2A3 expression groups indicated that the DRD4 high expression group had low memory CD4 T-cell scores and high Treg scores (Figure 8C). The SLC2A3 high expression group had high natural killer (NK) cell, M0 macrophage, and neutrophil scores and low plasma cell and Treg scores (Figure 8D). To further explore the potential effects of DRD4 and SLC2A3 on CC, we analyzed gene expression in relation to the clinical features of CC. The expression of DRD4 and SLC2A3 did not differ in patients of different ages or genders; however, expression levels correlated with the pathological stage of CC (Figures S2,S3).
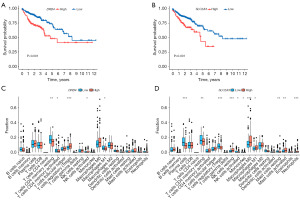
External validation and immunohistochemistry
To validate the prognostic model, we used the GSE17538 and GSE38832 external validation datasets. The PCA showed that our prognostic model distinguished well between patients in different risk groups (Figure 9A,9B). Kaplan-Meier analyses of OS and DSS revealed that patients in the HR group had a low likelihood of survival, which is concordant with our prior analyses (Figure 9C,9D). The ROC results suggest that the prognostic model has certain diagnostic efficacy (Figure 9E,9F). The results of immunohistochemistry experiments using six pairs of CC and paracancerous tissues are presented in Figure 9G-9I. Expression levels of DRD4 and SLC2A3 proteins in CC tissues were significantly upregulated compared with paracancerous tissues suggesting that they may influence CC.
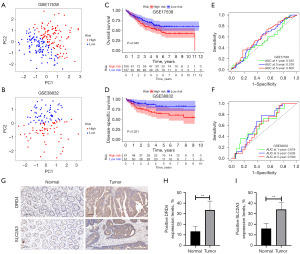
Discussion
CC is a typical malignant cancer of the gastrointestinal tract. Owing to high rates of metastasis and recurrence, the prognosis of patients with CC is very poor, with an overall five-year survival rate of approximately 20% (46). PCD plays a crucial role in tumor progression (47). Disulfidptosis and ferroptosis are important PCD modalities, and both are regulated by the key gene SLC7A11 (48). To date, biomarkers with good predictive power and therapeutic target potential have been identified in a wide range of tumors based on disulfidptosis and ferroptosis (49,50). Currently, few published studies have focused on the relationship between DRFGs and CC prognosis. Therefore, developing a prognostic model based on DRFGs that can aid in predicting prognosis and improving treatment in CC is warranted.
This study constructed a prognostic model comprising two genes based on the expression of DRFGs and survival data from patients with CC. Using the calculated risk scores, patients with CC were classified into HR and LR groups. Survival analysis demonstrated that patients in the HR group displayed a poor prognostic status. ROC analysis demonstrated that this model had a stable and effective ability to assess the prognostic status of patients with CC. Subsequently, through biological characteristic enrichment, immune infiltration, and TME analyses, we identified a strong association between this model and CC prognosis.
This prognostic model utilizes the expression of DRD4 and SLC2A3. DRD4 is a dopamine receptor belonging to the G protein-coupled receptor family. Recently, a study demonstrated that DRD4 regulates the proliferation, migration, and apoptosis of CC cells and exerts pro-tumorigenic effects (51). Chen et al. developed a 7-gene model (including DRD4) to assess OS in colorectal cancer patients (52). Furthermore, the inhibition of DRD4 has been shown to impede autophagic flux, as well as the proliferation and survival of glioblastoma stem cells (35). In esophageal squamous cell carcinoma and thyroid carcinoma, a prognostic model constructed with the participation of DRD4 has been shown to have a predictive value for patient survival (53,54). SLC2A3 belongs to the solute carrier 2A (SLC2A) family, has a high glucose affinity, and is involved in transmembrane glucose transport (55). Tumor cells can meet the demands of proliferation by increasing glucose consumption through aerobic glycolysis; this is known as the Warburg effect (56). It has been previously shown that SLC2A3 is associated with gastric, pancreatic, and prostate cancers and is involved in glycolysis in these tumors (57-59). Gao et al. reported that the knockdown of SLC2A3 inhibited the migration of colorectal cancer cells (37). Kim et al. reported that colorectal cancer patients with high expression of SLC2A3 had low disease-free survival rates (60). These previous reports suggest that the genes used in our model may have a considerable role in CC. Furthermore, through survival analyses, we found that patients with high DRD4 and SLC2A3 expression had poor OS and that the expression of DRD4 and SLC2A3 was related to the clinical stage of CC. These results suggest DRD4 and SLC2A3 as important targets with the potential to guide CC treatment.
Using the DEGs between HR and LR groups, we investigated the biological characteristics associated with the prognostic model and found that the DEGs were connected to pathways such as PI3K-Akt signaling and PPAR signaling. The inhibition of the PI3K-Akt signaling pathway has been reported to inhibit colorectal cancer metastasis (61). Furthermore, the PPAR signaling pathway promotes proliferation and inhibits apoptosis in colorectal cancer cells (62). Following this, we evaluated the TME, and the analysis showed higher stromal, immune, and ESTIMATE scores in the HR group than in the LR group. We observed that the infiltrating level of memory CD4 T-cells was increased in the LR group, whereas Treg cells and M0 macrophages were more abundant in the HR group than in the LR group. Treg cells can restrain antitumor immunity and are the key cells that help tumor cells escape host immunity, which may explain the poor prognosis of the HR group (63,64). Furthermore, we assessed the sensitivity of patients with CC in different risk groups to a range of anticancer drugs and showed that eight drugs, including foretinib, were preferable for patients with CC in the HR group. These findings provide more personalized treatment options for patients with CC in different risk groups, thereby improving patient prognosis.
Studies have shown that both disulfidptosis and ferroptosis are promising mechanisms in cancer therapy. Ferroptosis, characterized by iron-dependent lipid peroxidation, has been linked to tumor suppression in various cancers, including hepatocellular carcinoma and colorectal cancer (65,66). Ferroptosis-related genes, such as those involved in iron metabolism, have demonstrated prognostic value across several cancer types, and recent studies suggest that combining ferroptosis inducers with other therapeutic strategies could enhance treatment efficacy (67,68). For instance, a study in hepatocellular carcinoma identified a prognostic signature based on disulfidptosis-related genes, offering valuable insights for personalized treatment (69). Similarly, disulfidptosis, a recently identified form of PCD, has been shown to play a role in cancer progression and prognosis. The study by Xiao et al. demonstrated that disulfidptosis could be triggered by glucose starvation, leading to actin network disruption and cell death (70). Given that metabolic alterations, including enhanced glycolysis (the Warburg effect), are hallmarks of cancer, targeting disulfidptosis offers a novel therapeutic avenue. In this context, SLC2A3 plays a critical role in glucose metabolism by facilitating glucose uptake, further linking metabolic pathways to cancer progression and prognosis.
While disulfidptosis and ferroptosis are emerging as critical pathways in cancer biology, current prognostic models rarely integrate these cell death modalities. Our study adds to the growing body of literature by incorporating these mechanisms into a prognostic model for CC. Previous research has established ferroptosis as a viable target in colon adenocarcinoma, highlighting the therapeutic potential of modulating iron metabolism (66). Moreover, recent advancements in disulfidptosis research have underscored its potential as a target for personalized cancer therapies, particularly in tumors with metabolic vulnerabilities. The integration of both disulfidptosis- and ferroptosis-related genes into our model allows for a more comprehensive assessment of CC prognosis. By capturing the interplay between metabolic reprogramming and cell death pathways, the model provides insights not only into patient outcomes but also into potential therapeutic strategies. For example, patients with HR scores in our model showed increased sensitivity to specific chemotherapy agents, suggesting that the model could be used to tailor treatment regimens based on individual risk profiles.
Our study linked DRFGs to CC, constructing a reliable prognostic model and identifying a promising therapeutic target. However, the study was limited by reliance on public databases, highlighting the need for more patient data and further biological experiments to clarify the roles of the model genes. Despite these limitations, our findings underscore the clinical significance of disulfidptosis and ferroptosis in CC prognosis and treatment, paving the way for more precise and personalized cancer therapies.
Conclusions
Our study constructed and validated a DRFG-related prognostic model for the prognosis of patients with CC. This model can classify patients into HR and LR groups, aiding in the prediction of the prognosis of CC and guiding clinical treatment. In addition, high expression levels of DRD4 and SLC2A3 are associated with poor prognosis in patients with CC and are potential therapeutic targets for CC.
Acknowledgments
We thank TCGA and GEO databases for generously providing the data utilized in this study.
Footnote
Reporting Checklist: The authors have completed the TRIPOD reporting checklist. Available at https://tcr.amegroups.com/article/view/10.21037/tcr-24-1177/rc
Data Sharing Statement: Available at https://tcr.amegroups.com/article/view/10.21037/tcr-24-1177/dss
Peer Review File: Available at https://tcr.amegroups.com/article/view/10.21037/tcr-24-1177/prf
Funding: This research was supported by
Conflicts of Interest: All authors have completed the ICMJE uniform disclosure form (available at https://tcr.amegroups.com/article/view/10.21037/tcr-24-1177/coif). The authors have no conflicts of interest to declare.
Ethical Statement: The authors are accountable for all aspects of the work in ensuring that questions related to the accuracy or integrity of any part of the work are appropriately investigated and resolved. The study was conducted in accordance with the Declaration of Helsinki (as revised in 2013). The study was approved by the Ethics Committee of Affiliated Hospital of Nanjing University of Chinese Medicine (No. 2022NL-KS086) and individual consent for this retrospective analysis was waived.
Open Access Statement: This is an Open Access article distributed in accordance with the Creative Commons Attribution-NonCommercial-NoDerivs 4.0 International License (CC BY-NC-ND 4.0), which permits the non-commercial replication and distribution of the article with the strict proviso that no changes or edits are made and the original work is properly cited (including links to both the formal publication through the relevant DOI and the license). See: https://creativecommons.org/licenses/by-nc-nd/4.0/.
References
- Sung H, Ferlay J, Siegel RL, et al. Global Cancer Statistics 2020: GLOBOCAN Estimates of Incidence and Mortality Worldwide for 36 Cancers in 185 Countries. CA Cancer J Clin 2021;71:209-49. [Crossref] [PubMed]
- Siegel RL, Wagle NS, Cercek A, et al. Colorectal cancer statistics, 2023. CA Cancer J Clin 2023;73:233-54. [Crossref] [PubMed]
- Patel SG, Karlitz JJ, Yen T, et al. The rising tide of early-onset colorectal cancer: a comprehensive review of epidemiology, clinical features, biology, risk factors, prevention, and early detection. Lancet Gastroenterol Hepatol 2022;7:262-74. [Crossref] [PubMed]
- Schmoll HJ, Van Cutsem E, Stein A, et al. ESMO Consensus Guidelines for management of patients with colon and rectal cancer. a personalized approach to clinical decision making. Ann Oncol 2012;23:2479-516. [Crossref] [PubMed]
- Miller KD, Nogueira L, Devasia T, et al. Cancer treatment and survivorship statistics, 2022. CA Cancer J Clin 2022;72:409-36. [Crossref] [PubMed]
- Tan S, Shi H, Ba M, et al. miR-409-3p sensitizes colon cancer cells to oxaliplatin by inhibiting Beclin-1-mediated autophagy. Int J Mol Med 2016;37:1030-8. [Crossref] [PubMed]
- Temraz S, Mukherji D, Alameddine R, et al. Methods of overcoming treatment resistance in colorectal cancer. Crit Rev Oncol Hematol 2014;89:217-30. [Crossref] [PubMed]
- Zhang N, Hu X, Du Y, et al. The role of miRNAs in colorectal cancer progression and chemoradiotherapy. Biomed Pharmacother 2021;134:111099. [Crossref] [PubMed]
- Dixon SJ, Lemberg KM, Lamprecht MR, et al. Ferroptosis: an iron-dependent form of nonapoptotic cell death. Cell 2012;149:1060-72. [Crossref] [PubMed]
- Tang D, Chen X, Kang R, et al. Ferroptosis: molecular mechanisms and health implications. Cell Res 2021;31:107-25. [Crossref] [PubMed]
- Yang F, Xiao Y, Ding JH, et al. Ferroptosis heterogeneity in triple-negative breast cancer reveals an innovative immunotherapy combination strategy. Cell Metab 2023;35:84-100.e8. [Crossref] [PubMed]
- Tang B, Zhu J, Li J, et al. The ferroptosis and iron-metabolism signature robustly predicts clinical diagnosis, prognosis and immune microenvironment for hepatocellular carcinoma. Cell Commun Signal 2020;18:174. [Crossref] [PubMed]
- Ren X, Du H, Cheng W, et al. Construction of a ferroptosis-related eight gene signature for predicting the prognosis and immune infiltration of thyroid cancer. Front Endocrinol (Lausanne) 2022;13:997873. [Crossref] [PubMed]
- Wang Y, Fan J, Chen T, et al. A novel ferroptosis-related gene prognostic index for prognosis and response to immunotherapy in patients with prostate cancer. Front Endocrinol (Lausanne) 2022;13:975623. [Crossref] [PubMed]
- Zhang W, Yao S, Huang H, et al. Molecular subtypes based on ferroptosis-related genes and tumor microenvironment infiltration characterization in lung adenocarcinoma. Oncoimmunology 2021;10:1959977. [Crossref] [PubMed]
- Koppula P, Zhuang L, Gan B. Cystine transporter SLC7A11/xCT in cancer: ferroptosis, nutrient dependency, and cancer therapy. Protein Cell 2021;12:599-620. [Crossref] [PubMed]
- Liu X, Nie L, Zhang Y, et al. Actin cytoskeleton vulnerability to disulfide stress mediates disulfidptosis. Nat Cell Biol 2023;25:404-14. [Crossref] [PubMed]
- Zheng T, Liu Q, Xing F, et al. Disulfidptosis: a new form of programmed cell death. J Exp Clin Cancer Res 2023;42:137. [Crossref] [PubMed]
- Liu X, Olszewski K, Zhang Y, et al. Cystine transporter regulation of pentose phosphate pathway dependency and disulfide stress exposes a targetable metabolic vulnerability in cancer. Nat Cell Biol 2020;22:476-86. [Crossref] [PubMed]
- Hadian K, Stockwell BR. The therapeutic potential of targeting regulated non-apoptotic cell death. Nat Rev Drug Discov 2023;22:723-42. [Crossref] [PubMed]
- Huang J, Zhang J, Zhang F, et al. Identification of a disulfidptosis-related genes signature for prognostic implication in lung adenocarcinoma. Comput Biol Med 2023;165:107402. [Crossref] [PubMed]
- Hu G, Yao H, Wei Z, et al. A bioinformatics approach to identify a disulfidptosis-related gene signature for prognostic implication in colon adenocarcinoma. Sci Rep 2023;13:12403. [Crossref] [PubMed]
- Zhang Z, Ji Y, Hu N, et al. Ferroptosis-induced anticancer effect of resveratrol with a biomimetic nano-delivery system in colorectal cancer treatment. Asian J Pharm Sci 2022;17:751-66. [Crossref] [PubMed]
- Wu J, Liu C, Wang T, et al. Deubiquitinase inhibitor PR-619 potentiates colon cancer immunotherapy by inducing ferroptosis. Immunology 2023;170:439-51. [Crossref] [PubMed]
- Coupez D, Hulo P, Touchefeu Y, et al. KRAS mutations in metastatic colorectal cancer: from a de facto ban on anti-EGFR treatment in the past to a potential biomarker for precision medicine. Expert Opin Biol Ther 2021;21:1325-34. [Crossref] [PubMed]
- Douillard JY, Oliner KS, Siena S, et al. Panitumumab-FOLFOX4 treatment and RAS mutations in colorectal cancer. N Engl J Med 2013;369:1023-34. [Crossref] [PubMed]
- Barras D. BRAF Mutation in Colorectal Cancer: An Update. Biomark Cancer 2015;7:9-12. [Crossref] [PubMed]
- Le DT, Durham JN, Smith KN, et al. Mismatch repair deficiency predicts response of solid tumors to PD-1 blockade. Science 2017;357:409-13. [Crossref] [PubMed]
- De' Angelis GL. Microsatellite instability in colorectal cancer. Acta Biomed 2018;89:97-101. [PubMed]
- Guinney J, Dienstmann R, Wang X, et al. The consensus molecular subtypes of colorectal cancer. Nat Med 2015;21:1350-6. [Crossref] [PubMed]
- Dienstmann R, Vermeulen L, Guinney J, et al. Consensus molecular subtypes and the evolution of precision medicine in colorectal cancer. Nat Rev Cancer 2017;17:79-92. [Crossref] [PubMed]
- Stockwell BR, Friedmann Angeli JP, Bayir H, et al. Ferroptosis: A Regulated Cell Death Nexus Linking Metabolism, Redox Biology, and Disease. Cell 2017;171:273-85. [Crossref] [PubMed]
- Rosas-Cruz A, Salinas-Jazmín N, Velázquez MAV. Dopamine Receptors in Cancer: Are They Valid Therapeutic Targets? Technol Cancer Res Treat 2021;20:15330338211027913. [Crossref] [PubMed]
- Wang H, Fang D, Zhu J, et al. Ferroptosis-related gene signature predicts prognosis and immune microenvironment in prostate cancer. Transl Androl Urol 2024;13:2092-109. [Crossref] [PubMed]
- Dolma S, Selvadurai HJ, Lan X, et al. Inhibition of Dopamine Receptor D4 Impedes Autophagic Flux, Proliferation, and Survival of Glioblastoma Stem Cells. Cancer Cell 2016;29:859-73. [Crossref] [PubMed]
- Pan J, Zang Y. LINC00667 Promotes Progression of Esophageal Cancer Cells by Regulating miR-200b-3p/SLC2A3 Axis. Dig Dis Sci 2022;67:2936-47. [Crossref] [PubMed]
- Gao H, Liang J, Duan J, et al. A Prognosis Marker SLC2A3 Correlates With EMT and Immune Signature in Colorectal Cancer. Front Oncol 2021;11:638099. [Crossref] [PubMed]
- Zhou N, Bao J. FerrDb: a manually curated resource for regulators and markers of ferroptosis and ferroptosis-disease associations. Database (Oxford) 2020;2020:baaa021. [Crossref] [PubMed]
- Ritchie ME, Phipson B, Wu D, et al. limma powers differential expression analyses for RNA-sequencing and microarray studies. Nucleic Acids Res 2015;43:e47. [Crossref] [PubMed]
- Yu G, Wang LG, Han Y, et al. clusterProfiler: an R package for comparing biological themes among gene clusters. OMICS 2012;16:284-7. [Crossref] [PubMed]
- Subramanian A, Tamayo P, Mootha VK, et al. Gene set enrichment analysis: a knowledge-based approach for interpreting genome-wide expression profiles. Proc Natl Acad Sci U S A 2005;102:15545-50. [Crossref] [PubMed]
- Yoshihara K, Shahmoradgoli M, Martínez E, et al. Inferring tumour purity and stromal and immune cell admixture from expression data. Nat Commun 2013;4:2612. [Crossref] [PubMed]
- Newman AM, Steen CB, Liu CL, et al. Determining cell type abundance and expression from bulk tissues with digital cytometry. Nat Biotechnol 2019;37:773-82. [Crossref] [PubMed]
- Mayakonda A, Lin DC, Assenov Y, et al. Maftools: efficient and comprehensive analysis of somatic variants in cancer. Genome Res 2018;28:1747-56. [Crossref] [PubMed]
- Han Y, Wang Y, Dong X, et al. TISCH2: expanded datasets and new tools for single-cell transcriptome analyses of the tumor microenvironment. Nucleic Acids Res 2023;51:D1425-31. [Crossref] [PubMed]
- Franke AJ, Skelton WP, Starr JS, et al. Immunotherapy for Colorectal Cancer: A Review of Current and Novel Therapeutic Approaches. J Natl Cancer Inst 2019;111:1131-41. [Crossref] [PubMed]
- Kari S, Subramanian K, Altomonte IA, et al. Programmed cell death detection methods: a systematic review and a categorical comparison. Apoptosis 2022;27:482-508. [Crossref] [PubMed]
- Ma X, Deng Z, Li Z, et al. Leveraging a disulfidptosis/ferroptosis-based signature to predict the prognosis of lung adenocarcinoma. Cancer Cell Int 2023;23:267. [Crossref] [PubMed]
- Zhang C, Xu T, Ji K, et al. Development and experimental validation of a machine learning-based disulfidptosis-related ferroptosis score for hepatocellular carcinoma. Apoptosis 2024;29:103-20. [Crossref] [PubMed]
- Xu L, Wang S, Zhang D, et al. Machine learning- and WGCNA-mediated double analysis based on genes associated with disulfidptosis, cuproptosis and ferroptosis for the construction and validation of the prognostic model for breast cancer. J Cancer Res Clin Oncol 2023;149:16511-23. [Crossref] [PubMed]
- Lee CJ, Baek B, Cho SH, et al. Machine learning with in silico analysis markedly improves survival prediction modeling in colon cancer patients. Cancer Med 2023;12:7603-15. [Crossref] [PubMed]
- Chen J, Wu S, Peng Y, et al. Constructing a cancer stem cell related prognostic model for predicting immune landscape and drug sensitivity in colorectal cancer. Front Pharmacol 2023;14:1200017. [Crossref] [PubMed]
- Song J, Liu Y, Guan X, et al. A Novel Ferroptosis-Related Biomarker Signature to Predict Overall Survival of Esophageal Squamous Cell Carcinoma. Front Mol Biosci 2021;8:675193. [Crossref] [PubMed]
- Shi J, Wu P, Sheng L, et al. Ferroptosis-related gene signature predicts the prognosis of papillary thyroid carcinoma. Cancer Cell Int 2021;21:669. [Crossref] [PubMed]
- Rodríguez-Enríquez S, Marín-Hernández A, Gallardo-Pérez JC, et al. Kinetics of transport and phosphorylation of glucose in cancer cells. J Cell Physiol 2009;221:552-9. [Crossref] [PubMed]
- Fukushi A, Kim HD, Chang YC, et al. Revisited Metabolic Control and Reprogramming Cancers by Means of the Warburg Effect in Tumor Cells. Int J Mol Sci 2022;23:10037. [Crossref] [PubMed]
- Chen D, Wang H, Chen J, et al. MicroRNA-129-5p Regulates Glycolysis and Cell Proliferation by Targeting the Glucose Transporter SLC2A3 in Gastric Cancer Cells. Front Pharmacol 2018;9:502. [Crossref] [PubMed]
- Liu Y, Jin A, Quan X, et al. miR-590-5p/Tiam1-mediated glucose metabolism promotes malignant evolution of pancreatic cancer by regulating SLC2A3 stability. Cancer Cell Int 2023;23:301. [Crossref] [PubMed]
- Li J, Fu F, Wan X, et al. Up-regulated miR-29c inhibits cell proliferation and glycolysis by inhibiting SLC2A3 expression in prostate cancer. Gene 2018;665:26-34. [Crossref] [PubMed]
- Kim E, Jung S, Park WS, et al. Upregulation of SLC2A3 gene and prognosis in colorectal carcinoma: analysis of TCGA data. BMC Cancer 2019;19:302. [Crossref] [PubMed]
- Wei J, Zheng Z, Hou X, et al. Echinacoside inhibits colorectal cancer metastasis via modulating the gut microbiota and suppressing the PI3K/AKT signaling pathway. J Ethnopharmacol 2024;318:116866. [Crossref] [PubMed]
- Wang R, Li J, Zhou X, et al. Single-cell genomic and transcriptomic landscapes of primary and metastatic colorectal cancer tumors. Genome Med 2022;14:93. [Crossref] [PubMed]
- Watson MJ, Vignali PDA, Mullett SJ, et al. Metabolic support of tumour-infiltrating regulatory T cells by lactic acid. Nature 2021;591:645-51. [Crossref] [PubMed]
- Itahashi K, Irie T, Nishikawa H. Regulatory T-cell development in the tumor microenvironment. Eur J Immunol 2022;52:1216-27. [Crossref] [PubMed]
- Liu JF, Huang L, Zhou XP, et al. Disulfidptosis and ferroptosis related genes predict prognosis and personalize treatment for hepatocellular carcinoma. Transl Cancer Res 2024;13:496-514. [Crossref] [PubMed]
- Liu X, An J, Wang Q, et al. Characterization and validation of a prognostic model for the N6-methyladenosine-associated ferroptosis gene in colon adenocarcinoma. Transl Cancer Res 2024;13:4389-407. [Crossref] [PubMed]
- Jiang M, Hu R, Yu R, et al. A narrative review of mechanisms of ferroptosis in cancer: new challenges and opportunities. Ann Transl Med 2021;9:1599. [Crossref] [PubMed]
- Yamaguchi Y, Kasukabe T, Kumakura S. Piperlongumine rapidly induces the death of human pancreatic cancer cells mainly through the induction of ferroptosis. Int J Oncol 2018;52:1011-22. [Crossref] [PubMed]
- Zhu J, Wu Y, Ji P, et al. Identification of a prognostic disulfidptosis-related gene signature in hepatocellular cancer. J Gastrointest Oncol 2024;15:1647-56. [Crossref] [PubMed]
- Xiao F, Li HL, Yang B, et al. Disulfidptosis: A new type of cell death. Apoptosis 2024;29:1309-29. [Crossref] [PubMed]