Construction and validation of a prognostic signature using WGCNA-identified key genes in osteosarcoma for treatment evaluation
Highlight box
Key findings
• Key genes associated with osteosarcoma (OS) prognosis and progression were identified, and a predictive model for OS patients was constructed based on these genes to evaluate the effectiveness of chemotherapy and immunotherapy strategies.
What is known and what is new?
• Currently, most prognostic models are constructed based on specific biological functions, which limits their predictive capabilities.
• Prognostic genes that play key roles in OS development and progression were found to construct the predictive model, aiming to achieve more accurate and comprehensive predictions.
What is the implication, and what should change now?
• A broadly applicable prognostic model for various OS patients was constructed, suggesting that CKMT2 and CGREF1 may serve as potential prognostic targets for OS. The model indicates that immunotherapy is more effective in low-risk individuals, and the sensitivity of patients at different risk levels to various chemotherapy drugs has also been evaluated.
Introduction
Osteosarcoma (OS) is an essential threatening bone tumor that’s commonly found in children and adolescents (1). Patients with OS often face a poor prognosis, especially in metastatic cases, where the 10-year survival rate hovers around just 20–30%, due to its high invasiveness, rapid proliferation, and early propensity for metastasis (2,3). Given these challenges, creating viable prognostic models for OS patients is vital for precisely predicting disease progression and survival, as well as distinguishing novel molecular indicators and novel treatment targets that can improve patient outcomes. These models utilize transcriptomic data to recognize prognostically significant biomarkers and stratify patients based on their risk profiles. This assists clinicians in forecasting patient outcomes, directing personalized treatment strategies, and possibly upgrading survival rates for those suffering from this challenging disease.
Currently, numerous studies have focused on developing prognostic models for OS. These models typically depend on genes known to participate in specific cellular functions, such as immune infiltration, oxidative phosphorylation, and endoplasmic reticulum stress (4-6). Others are based on genes associated with tumor-specific characteristics, such as those involved in cell migration (7). These prognostic models not as it were given extra therapeutic strategies for OS patients, but also enhance our understanding of the relationship between OS and these cellular functions or disease characteristics. However, OS progression is influenced by various biological processes (BPs), and depending solely on one set of genes might not fully capture the disease’s complexity. As a result, prognostic models that focus solely on genes associated with particular cellular functions or disease characteristics might overlook other essential BPs and clinical factors. Therefore, further refinement is necessary to enhance predictive accuracy. It is crucial to strive for more comprehensive prognostic models for OS.
The weighted gene co-expression network analysis (WGCNA) could be an effective method for developing gene co-expression networks to find modules of highly correlated genes and distinguish key genes related to particular biological states (8). This approach helps identify core genes that may play crucial roles in critical aspects of specific BPs or diseases. Recently, numerous studies have combined WGCNA analysis with the development of prognostic models, utilizing it to pinpoint key genes in tumors for model construction (9,10). Given the complex relationships between these genes and disease progression, prognostic models developed from these pivotal disease-related genes exhibit greater robustness and universality.
In this study, we started by using the WGCNA method to find key genes linked to OS. After that, we carried out survival analysis on these genes to determine which ones are important for prognosis. Based on these prognostic genes, we built and validated a solid prognostic model for OS. We present this article in accordance with the TRIPOD reporting checklist (available at https://tcr.amegroups.com/article/view/10.21037/tcr-24-1398/rc).
Methods
Data collection
All data were sourced from the Gene Expression Omnibus (GEO) database (https://www.ncbi.nlm.nih.gov/gds/) and the Therapeutically Applicable Research to Generate Effective Treatments (TARGET) database (https://www.cancer.gov/ccg/research/genome-sequencing/target). Initially, we selected the GSE16088 and GSE19276 datasets for WGCNA analysis. After merging, we obtained RNA sequencing (RNA-seq) data from a total of 72 OS patients, including 14 normal tissue samples and 58 tumor tissue samples. Subsequently, we chose the TARGET dataset to establish the risk model. We found that this dataset contained both RNA-seq and clinical information for 85 tumor tissue samples from patients. In addition, the study was conducted in accordance with the Declaration of Helsinki (as revised in 2013).
Screening differentially expressed genes (DEGs) in OS and performing enrichment analysis
We utilized the GSE16088 and GSE19276 datasets to identify DEGs between OS cancerous tissues and healthy tissues using the “limma” utility in R (version 4.3.1), with criteria set at |log2 fold change (log2FC)| ≥1 and P<0.05. Subsequently, we used the “pheatmap” and “ggplot2” tools for visualization of these genes. To examine the pathways and biological activities that are regulated by these DEGs, we conducted Kyoto Encyclopedia of Genes and Genomes (KEGG) and gene set enrichment analysis (GSEA) using the “clusterProfiler” tool. The GSEA datasets utilized in this study were obtained from the GSEA database (https://www.gsea-msigdb.org/gsea/index.jsp).
WGCNA for identifying key genes associated with OS
In our study, we employed the “WGCNA” package to analyze DEGs in OS. Initially, we used the “WGCNA” package to select samples and features. The “goodSamplesGenes” function was employed to check and filter samples and genes, ensuring the reliability and consistency of our analysis. Subsequently, “the hclust” function was used to generate a sample clustering tree to assess the similarity between samples. Following this, we constructed a gene co-expression network. The “pickSoftThreshold” function was used to ascertain an optimal soft threshold, while the “adjacency” function calculated the adjacency matrix. The “TOMsimilarity” function was then employed to estimate the topological overlap among genes. Module identification and analysis, identifying gene sets with similar expression patterns, were conducted using the “cutreeDynamic” function. Lastly, the “cor” function was performed to explore relationships between modules and biological features, revealing associations between these modules and specific biological states or disease processes. After intersecting the DEGs with the key genes identified through WGCNA, we obtained the critical OS-related genes (OSRGs).
Filtering prognosis-associated OSRGs
To further explore the potential roles of OSRGs in patient prognosis and construct an OSRG-related prognostic risk model, we performed a Gene Ontology (GO) study to understand the biological functions of these genes. We also constructed a prognostic network using the “igraph”, “psych”, “reshape2”, and “RColorBrewer” packages to investigate their interactions in prognosis. Following this, we performed survival analysis using the “survival” and “survminer” packages to identify OSRGs with significant prognostic associations (P<0.05). Based on these prognostically significant genes, we proceeded with subsequent model construction.
Analysis of consensus clustering for OSRGs
According to the expression of OSRGs, we employed the “ConsensusClusterPlus” tool to do cluster analysis. We applied the k-means technique to determine the best number of clusters, ranging from 2 to 9, by conducting 1,000 tests. The “pheatmap” tool was used to visualize the expression levels of OSRGs across these clusters. Kaplan-Meier (KM) curves were generated to assess disparities in overall survival between the clusters via the “survival” tool. Gene set variation analysis (GSVA) was used to investigate biological functions using the “c2.cp.kegg.Hs.symbols.gmt” and “c5.go.symbols.gmt” datasets from the Molecular Signatures Database (MSigDB) database (https://www.gsea-msigdb.org/gsea/msigdb).
Development of the OSRG-related risk model
The “limma” package was employed to identify DEGs within specific clusters, with thresholds of false discovery rate (FDR) <0.05 and |log2FC| <0.585. The biological functions and pathways associated with these DEGs (P<0.05) were investigated through analyses of the KEGG and GO enrichment, which were conducted using the “clusterProfiler” tool. Based on these DEGs, we constructed gene clusters and separately analyzed the expression of these DEGs and OSRGs within each cluster. Additionally, we examined the biological functions associated with each gene cluster. Univariate Cox regression was employed to further isolate prognostic genes from the DEGs (P<0.05).
Using prognostic-related DEGs, we developed a risk model in R based on packages such as “caret”, “glmnet”, “survival”, “survminer”, and “timeROC”. Initially, the samples were randomly divided into two equal sets: one for training to establish the OSRG-related risk model and the other for testing to validate it. In order to reduce gene overfitting, we implemented least absolute shrinkage and selection operator (LASSO) regression analysis, which was subsequently followed by cross-validation. The candidate genes for the prognostic risk model were ultimately discovered using multivariate Cox regression analysis. The formula for computing risk scores for individuals is risk score = the sum of (Expi × coefi), where coefi represents the regression coefficient and Expi represents the gene expression value for each gene.
Subsequently, based on the median score of the training set, all samples were stratified into high and low-risk groups. A Sankey diagram was constructed using the “dplyr” tool to visually represent the distribution of OSRG-related and gene clusters across various risk groups and survival statuses. Additionally, we constructed a nomogram for further validation of the risk model by “rms” package.
Validation of the OSRG-related risk model
In order to verify the precision of the prognostic model we developed, we made prognostic risk predictions for each of the testing, training, and all patient groups separately. Initially, survival analyses were conducted within each of these three groups to compare the survival times. Following this, we used the “pheatmap” tool to examine the expression patterns of the prognostically significant genes in the constructed model. Additionally, we utilized the “survival” tool to display the correlation between survival status and survival times.
Immune infiltration-related analysis
The “GSEABase” tool was employed to investigate the differences in immune cell infiltration levels and immune function enrichment between high- and low-risk groups in order to investigate the relationship between risk score and immune infiltration. The “estimate” tool was employed to investigate the tumour microenvironment scores of high- and low-risk groups, which included Immune Score, Estimation of Stromal and Immune cells in Tumor microEnvironment (ESTIMATE) Score, and Stromal Score. The “ggExtra” tool was employed to perform the visualization. At last, we identified nine common immune checkpoint genes from the previous studies and conducted an analysis of their differential expression between high-risk and low-risk groups (11-13).
Drug sensitivity analysis
In addition, we used the “pRophetic” tool to evaluate the effectiveness of frequently used chemotherapeutic drugs in different risk groups by computing the half maximum inhibitory concentration (IC50) values.
Analysis of genes related to the prognostic model
The survival analysis was initially performed on creatine kinase, mitochondrial 2 (CKMT2) and cell growth regulator with EF-hand domain 1 (CGREF1) using the “survival” and “survminer” packages. Next, we accessed the Search Tool for the Retrieval of Interacting Genes/Proteins (STRING) database (https://string-db.org/), setting the medium confidence level as a criterion and limiting the number of first and second shell interactors to a maximum of 20. We identified proteins associated with CKMT2 and CGREF1, along with their interacting partners. Utilizing Cytoscape 3.7.2, we visualized the protein networks related to CKMT2 and CGREF1 separately. Finally, we employed the “clusterProfiler” tool to conduct KEGG analysis on the proteins associated with CKMT2 and CGREF1, aiming to elucidate the biological pathways and functions in which they may have been involved.
Statistical analysis
The significance threshold was established at P<0.05, and all statistical analyses were conducted by R software (version 4.3.1) and its associated tools.
Results
Differential expression analysis and functional enrichment in OS
To identify key genes related to the pathogenesis and progression of OS, we first screened for DEGs in OS. Including 64 downregulated and 214 upregulated genes, totally 278 DEGs were found (Figure 1A), with the visualization results shown in Figure 1B. These DEGs may be closely associated with disease occurrence and progression, prompting further pathway enrichment analysis. KEGG analysis revealed that these DEGs play roles in various critical BPs, including the cell cycle, cell death, metabolism, immune response, and disease-related processes (Figure 1C). GSEA analysis indicated that these DEGs are primarily up-regulated the immune and inflammation-related functions (Figure 1D).
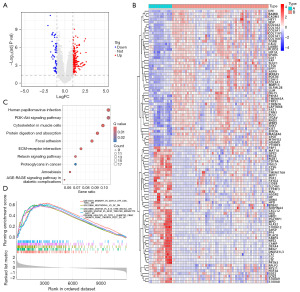
WGCNA network construction and screening of prognostic genes associated with OS
We conducted WGCNA analysis on 72 samples, initially calculating the standard deviation for each gene and filtering out those with a standard deviation less than 0.7, resulting in 2,219 genes with high variability. Subsequently, we constructed a weighted gene co-expression network by selecting a soft threshold to build an adjacency matrix, ensuring the network adheres to scale-free topology. We determined power =8 as the optimal threshold (Figure 2A). Using the topological overlap matrix, genes were clustered, and gene modules were identified using the dynamic tree cut algorithm with a minimum module size set to 60. Similar modules were merged, and module eigengenes were computed, resulting in six modules (Figure 2B). We assessed the correlation and significance of each module with clinical traits, presenting the relationships using a heatmap (Figure 2C). The red and blue modules showed significant associations with OS (P<0.05). Therefore, we subsequently computed the module membership (MM) of each gene in the red and blue modules, along with their gene significance (GS) to the trait. Genes were filtered based on MM >0.8 and GS >0.5, identifying key genes within each module. We identified 41 key genes in the blue module and 1 key gene in the red module. By merging genes from both modules and intersecting them with DEGs in OS, we identified a final set of 36 key genes relevant to OS (Figure 2D; Table S1).
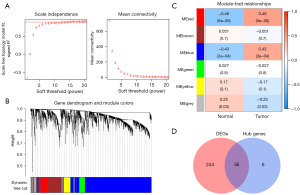
To further filter genes associated with OS prognosis for building the prognostic model, we initially performed a GO analysis on these genes to explore the primary BPs predominantly regulated by them, presented in Figure 3A. Subsequently, a prognostic network was constructed among these 36 genes, revealing correlations involving 35 genes (Figure 3B). Lastly, we performed survival analysis on the 36 genes choosing those with a P<0.05 significant level for additional investigation (Figure 3C). Following screening, 14 genes demonstrated significant correlation with OS patient survival. Due to the lack of clinical information in the GSE16088 and GSE19276 datasets, we utilized the TARGET dataset for constructing the prognostic model.
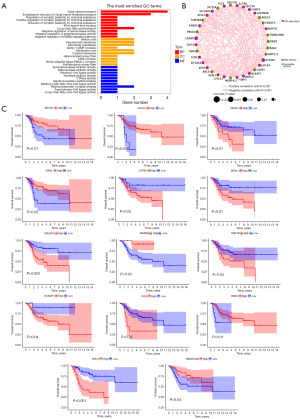
Construction of OSRG-related clusters in OS
We conducted consensus clustering analysis to identify separate clusters of OS patients based on the differential expression of 14 OSRGs. The optimal partition of the samples into cluster A and cluster B was determined to be k=2 (Figure 4A). The Kaplan-Meier analysis in Figure 4B revealed that cluster B exhibited better overall survival outcome compared to cluster A (P=0.08). The expression patterns of these 14 OSRGs were also compared between the two clusters. A heatmap was generated to graphically depict the associations among gene expression, clinical characteristics, and the clusters (Figure 4C). To get a more profound comprehension of the biological distinctions among these clusters, the GSVA enrichment analyses were employed. The enrichment of biological function and pathways for each cluster are shown in a heatmap, as shown in Figure 4D,4E.
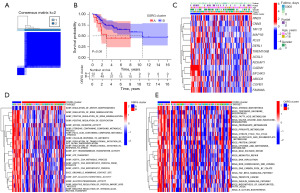
DEGs between OSRG-related clusters and construction of gene clusters
Based on the two OSRG-related clusters we created, we identified 167 DEGs for further study. To investigate the enhancement of biological activities and pathways in these DEGs, we conducted both GO and KEGG analyses. We focused on the 15 most enriched pathways in KEGG and the top 10 functions in each of the BP, cellular component (CC), and molecular function (MF) categories in the GO analysis, ranked by P values (Figure 5A,5B).
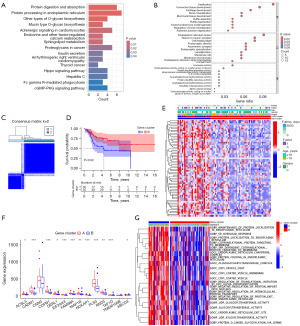
The OS samples were categorized into two gene clusters based on the expression of DEGs using the consensus clustering technique (Figure 5C). The Kaplan-Meier survival plot showed a significant difference in overall survival between the two gene clusters (P=0.02). Patients in gene cluster A were more likely to experience poorer outcomes (Figure 5D). The heatmap illustrated the differential expression of prognostic DEGs between the two gene groups (Figure 5E). Additionally, the levels of OSRG expression were examined in these two gene clusters, revealing notable disparities in 12 genes between the clusters (Figure 5F). We also performed GO analysis on the two gene clusters and found significant differences in the enriched biological functions between the two gene clusters (Figure 5G).
OSRG-related prognostic model construction and validation
To determine the prognostic significance of these DEGs, we performed univariate Cox analysis, identifying 62 DEGs with prognostic value at a statistical significance of P<0.05. After identifying prognostic DEGs, we developed an OSRG-related risk model that was based on these genes. All samples were arbitrarily assigned to either the training or testing group. LASSO regression and Cox multivariate regression analysis were employed to identify the most effective prognostic factors (Figure 6A). Using LASSO regression and minimum partial likelihood deviance, we selected four OS-related genes for inclusion in the Cox multivariate regression analysis to establish the prognostic model. Individual risk scores are calculated using the following formula: Risk score = (0.0289 × expression of CKMT2) + (0.0277 × expression of CGREF1).
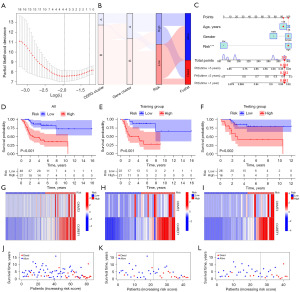
Based on the median risk score from the training group, we categorized all samples into two groups: a low-risk group consisting of 48 samples and a high-risk group consisting of 37 samples (Table S2). A Sankey diagram was used to illustrate the relationships between OSRG clusters, gene clusters, risk score groups, and survival status (Figure 6B). Additionally, we developed a personalized score nomogram for comprehensive risk assessments of patients based on three clinical features: age, gender, and risk score (Figure 6C).
To demonstrate the clinical applicability of the risk model, we compared the distribution of risk scores, survival status, and the expression of genes used in model construction between distinct groups. In both the training and testing groups, patients with higher risk scores exhibited stronger associations with poorer prognoses (Figure 6D-6F). The expression levels of CKMT2 and CGREF1 increased with higher risk scores (Figure 6G-6I). Furthermore, as disease progression and risk scores increased, susceptibility to mortality also increased (Figure 6J-6L). These findings underscore the robust prognostic accuracy of the risk model and its suitability for future research.
Risk groups and immune infiltration
Using our constructed prognostic model, we investigated the differences in immune infiltration between high-risk and low-risk groups. First, we evaluated the infiltration levels of stromal and immune cells in tumor samples from different risk groups using the ESTIMATE algorithm. The results indicated significant differences in immune infiltration scores between the two risk groups for both stromal and immune cells (Figure 7A). Subsequently, we analyzed the specific types of immune cells and immune functions enriched in the high- and low-risk groups, as shown in Figure 7B,7C. Finally, we examined the expression differences of reported immune checkpoints between the two risk groups. Our analysis revealed that, with the exception of ICOSLG, the majority of immune checkpoints exhibited significantly higher expression in patients with lower risk scores (Figure 7D).
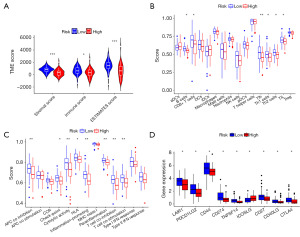
Risk groups and chemotherapeutic treatment analysis
To investigate how OS patients’ risk scores, as calculated by our model, correlate with the effectiveness of chemotherapeutic treatments, we conducted evaluations. Our findings indicated that treatments like elesclomol, AZD8055, GDC0941, and nilotinib were more effective in the low-risk group, while JNJ.26854165, JNK Inhibitor VIII, LFM-A13, and shikonin showed greater efficacy in the high-risk group (Figures 8A-8H).
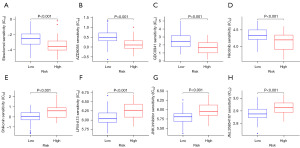
The functional analysis of CGREF1 and CKMT2 in OS
Since the prognostic model was constructed based on the expression of CGREF1 and CKMT2, it was crucial to further investigate the significance of these proteins in OS. Based on survival analysis, we found that OS patients with high expression levels of CGREF1 and CKMT2 often face poorer prognoses (Figure 9A,9B). This conclusion aligns with our earlier finding that the risk score is positively correlated with expression levels of CGREF1 and CKMT2. Proteins typically work synergistically through interactions to regulate cellular functions and physiological processes. To further analyze the regulatory roles of CGREF1 and CKMT2 in biological functions, we first constructed protein-protein interaction (PPI) networks for CGREF1 and CKMT2. To gain a deeper understanding of the biological functions associated with these two genes and the proteins that interact with them, we simultaneously screened both first and second shell interactors. Through PPI analysis, we identified 26 proteins associated with CGREF1 and 40 proteins associated with CKMT2 (Figure 9C,9D). Among these, CGREF1 had 6 first shell interactors and 20 second shell interactors, while CKMT2 had 20 first and second shell interactors each. After visualizing the networks using Cytoscape, second shell interactors were marked in gray, while first shell interactors were color-coded from red to yellow based on their degree values. Finally, we conducted KEGG analysis on the nodes of the interaction networks for both proteins to explore the related pathways regulated by CGREF1 and CKMT2. The results indicated that CGREF1 and its associated proteins primarily participate in the regulation of various tumor-related pathways, whereas CKMT2 and its associated proteins play significant roles in multiple metabolic processes (Figure 9E,9F).
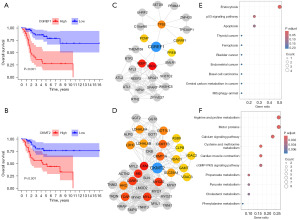
Discussion
As a highly malignant tumor, OS frequently presents considerable uncertainty regarding disease progression and treatment responses, resulting in poor prognostic outcomes for patients. Identifying new biomarkers with therapeutic or diagnostic potential and conducting prognostic predictions based on the clinical characteristics of patients play a crucial role in improving the treatment efficacy and quality of life for OS patients. Currently, numerous studies have constructed OS-related prognostic models based on genes associated with specific functions or disease characteristics (14,15). However, constructing OS models based on key genes involved in the pathogenesis and progression of OS may offer broader applicability to various patient groups, although such predictive models remain relatively rare in OS research. Therefore, this study aims to construct a reliable and universally applicable prognostic model based on OS-related genes.
We employed the WGCNA method to identify key modules associated with OS progression and obtained crucial genes related to OS. Initially, we screened for DEGs between OS and normal tissues, followed by KEGG and GSEA analyses of these genes. The functions regulated by these genes primarily encompass deoxyribonucleic acid (DNA) repair, cell cycle regulation, mechanisms of cell death, and various metabolic pathways. These functions influence one another, resulting in a complex network. Under normal circumstances, DNA repair maintains genomic stability and facilitates the orderly progression of the cell cycle. However, when this repair fails, it can disrupt the cell cycle, subsequently triggering cell death mechanisms to avert potential tumor development (16). Tumor cells frequently disrupt cell cycle regulation by inactivating DNA repair pathways, promoting rapid cell division without addressing genetic damage. Furthermore, alterations in cell cycle regulatory factors enable tumor cells to bypass standard division checkpoints, thus avoiding activation of cell death mechanisms and evading immune system surveillance (17,18). Additionally, these cells can reprogram their metabolic pathways to meet the energy demands of rapid proliferation and enhance their antioxidant capacity to counteract stress induced by cell cycle abnormalities and DNA damage (19). The interplay among these mechanisms allows tumor cells to survive, proliferate, and develop resistance to therapeutic interventions. Furthermore, these genes were linked to increased immune system activity and responsiveness in OS patients, including the activation of immune cells, enhanced inflammatory responses, and infection responses. Overall, the functions enriched by these genes suggest that mechanisms such as cell death, cellular metabolism, and immune response play crucial roles in the occurrence and progression of OS.
Subsequently, we conducted WGCNA analysis based on the expression of all genes in OS patients, identifying two key modules—red and blue—associated with OS, totaling 42 key genes. By intersecting these 42 genes with DEGs in OS, we ultimately identified 36 key genes related to OS using the WGCNA method. To enhance the construction of the prognostic model, we further investigated the 36 genes and ultimately selected 14 prognosis-related OSRGs for the construction of the model. Among these 14 genes, five have already been reported to play regulatory roles in OS. CADM1 and CNN3 are involved in regulating the proliferation, migration, and invasion of OS cells, while DERL1 is implicated in inducing autophagy in these cells (20-22). Bioinformatics analysis suggests that TAF1D and FAM98A may serve as new biomarkers for OS, although their specific mechanisms require further investigation (23,24). Future research could delve into the specific roles of TAF1D and FAM98A, while also validating the regulatory functions of CADM1, CNN3, and DERL1 in the progression of OS, aiming to provide new targets and strategies for OS treatment and prognosis. Given that all 14 genes are considered closely related to OS prognosis, other unreported genes also warrant further attention.
Although we could directly construct a prognostic model using these 14 prognosis-related OSRGs, OS is a highly heterogeneous tumor (25). Considering only the average impact of OSRGs on prognosis across all patients may not adequately account for significant molecular differences among different patients, leading to inconsistent predictive performance of the model across different OS patient types. Therefore, we first classified patients based on these 14 prognosis-related OSRGs and subsequently screened for DEGs between the identified clusters. This ensures that the selected genes exhibit significant prognostic relevance in different clusters, thereby enhancing the predictive capability and accuracy of the prognostic model. Based on these 14 prognosis-related OSRGs, we classified all patients into two clusters. Despite cluster A patients having a poorer prognosis compared to cluster B, there was no significant difference in survival between these two subtypes. This is likely due to the considerably smaller number of patients in cluster A compared to cluster B. However, gene differential expression analysis and GO and KEGG enrichment analysis between the two clusters indicate significant differences in OSRGs expression and DEGs expression and enriched biological functions and pathways. In summary, we successfully stratified OS patients into two distinct clusters.
Afterwards, we screened for DEGs between two clusters. GO and KEGG analyses revealed that these genes play crucial roles in cell migration, invasion, bone and cartilage development, mineralization processes, protein synthesis, and modification. These biological functions and pathways are known to be pivotal in the pathogenesis of OS. Using these DEGs, we conducted single-factor Cox analysis to identify genes with prognostic value and classified patients based on their expression profiles. The results demonstrated significant differences between the clusters in terms of survival outcomes, biological functions, and the expression levels of the OSRGs and DEGs. This further highlights the importance of these DEGs, not only in predicting OS prognosis but also in effectively classifying OS patients.
Based on these DEGs, we constructed a prognostic model using LASSO regression and Cox multivariate regression. The final prognostic score formula includes CKMT2 and CGREF1 genes. CKMT2 encodes an enzyme crucial for cellular energy homeostasis. This enzyme facilitates the transfer of high-energy phosphate from mitochondria to creatine, forming phosphocreatine, a vital energy reserve (26). In several cancers, including colorectal and breast cancer, CKMT2 is implicated in promoting tumor growth and development (27,28). CGREF1, also known as CGRP receptor component protein, is a gene that encodes a protein containing an EF-hand domain, which typically binds calcium ions (29). This protein plays a role in cellular signaling pathways involved in regulating cell growth, differentiation, and survival (30). Although specific research on these genes’ mechanisms in OS patients is limited, both CKMT2 and CGREF1 have been implicated in constructing various prognostic models for OS. CKMT2 has been explored in models focused on oxidative stress-related genes, hypoxia-immune related genes, and mitochondria-related genes, whereas CGREF1 has been linked to models based on vasculogenic mimicry-associated and cuproptosis-related genes in OS (31-35). In this study, we conducted an initial investigation into the potential protein interactions and enriched pathways associated with CKMT2 and CGREF1. Our findings indicate that CKMT2 is primarily involved in mechanisms related to cellular metabolism, while CGREF1 is linked to various tumors and forms of cell death. These results underscore the critical roles that cellular metabolism and cell death mechanisms play in the development and progression of OS, and they suggest possible future research directions for CKMT2 and CGREF1 in OS-related studies.
After constructing the prognostic model, we validated it using a testing group. We observed that patients in the high-risk group consistently showed significantly reduced survival times across all patients, including those in the training and testing groups. Moreover, CKMT2 and CGREF1 were found to be highly expressed in the high-risk group. In conclusion, our constructed prognostic model demonstrates robust predictive efficacy.
Based on the prognostic model we developed, we assessed the relationship between risk scores, immune infiltration, and drug sensitivity. Immune infiltration refers to the process by which immune cells enter the tumor microenvironment (TME) and interact with tumor cells and other components of the microenvironment (36,37). Therefore, we initially conducted a TME scoring for high- and low-risk groups. The results showed that regardless of whether considering stromal cells, immune cells individually, or their combined assessment, the TME scores were consistently lower in the high-risk group compared to the low-risk group. Further analysis of the types of infiltrating cells and immune functions in these groups revealed that the majority of immune cells and immune functions scored higher in the low-risk group compared to the high-risk group. Overall, immune infiltration was significantly better in the low-risk group, suggesting these patients may be more sensitive to immunotherapy and potentially benefit more from immune-enhancing adjunct therapies (38). Immune checkpoints are a critical mechanism of immune resistance in cancer, as they typically mediate immune tolerance and reduce collateral tissue injury. The blockage of immune checkpoints is one of the most promising methods for activating therapeutic antitumor immunity (39,40). Therefore, we examined the expression levels of several reported immune checkpoints between different risk groups and found that most immune checkpoints were expressed at higher levels in the low-risk group. This suggests that low-risk patients are generally more sensitive to immune checkpoint-targeted therapies and may be more suitable for such treatments. In addition, drug sensitivity analysis indicated that our prognostic model enables personalized treatment strategies for OS patients. Patients in the high- and low-risk groups can select more effective drugs based on the predictions of our model, thereby enhancing treatment outcomes.
Finally, in this study, we successfully identified OSRGs through the WGCNA method and constructed a prognostic model for OS. Additional datasets may be used in the future to validate and analyze the created prognostic model, hence improving and optimizing it. Moreover, conducting more in vitro and in vivo experiments will deepen our understanding of the specific functions of potential biomarkers like CKMT2 and CGREF1 in OS.
Conclusions
In summary, we used the WGCNA approach to identify genes associated with OS and selected prognostically relevant genes among them. Afterward, we categorized patients according to these OSRGs and discovered DEGs that are significant for prognosis amongst two clusters. As a result, we developed a reliable and widely applicable prognostic model. Subsequently, we verified the accuracy of the prognostic model to predict outcomes and performed investigations into immune infiltration and drug sensitivity based on the model. This allowed us to provide personalized treatment strategies for people with different levels of risk.
Acknowledgments
None.
Footnote
Reporting Checklist: The authors have completed the TRIPOD reporting checklist. Available at https://tcr.amegroups.com/article/view/10.21037/tcr-24-1398/rc
Peer Review File: Available at https://tcr.amegroups.com/article/view/10.21037/tcr-24-1398/prf
Funding: None.
Conflicts of Interest: All authors have completed the ICMJE uniform disclosure form (available at https://tcr.amegroups.com/article/view/10.21037/tcr-24-1398/coif). The authors have no conflicts of interest to declare.
Ethical Statement: The authors are accountable for all aspects of the work in ensuring that questions related to the accuracy or integrity of any part of the work are appropriately investigated and resolved. The study was conducted in accordance with the Declaration of Helsinki (as revised in 2013).
Open Access Statement: This is an Open Access article distributed in accordance with the Creative Commons Attribution-NonCommercial-NoDerivs 4.0 International License (CC BY-NC-ND 4.0), which permits the non-commercial replication and distribution of the article with the strict proviso that no changes or edits are made and the original work is properly cited (including links to both the formal publication through the relevant DOI and the license). See: https://creativecommons.org/licenses/by-nc-nd/4.0/.
References
- Hameed M, Dorfman H. Primary malignant bone tumors--recent developments. Semin Diagn Pathol 2011;28:86-101. [Crossref] [PubMed]
- Spalato M, Italiano A. The safety of current pharmacotherapeutic strategies for osteosarcoma. Expert Opin Drug Saf 2021;20:427-38. [Crossref] [PubMed]
- Beird HC, Bielack SS, Flanagan AM, et al. Osteosarcoma. Nat Rev Dis Primers 2022;8:77. [Crossref] [PubMed]
- Li J, Su L, Xiao X, et al. Development and Validation of Novel Prognostic Models for Immune-Related Genes in Osteosarcoma. Front Mol Biosci 2022;9:828886. [Crossref] [PubMed]
- Zhao Y, Gao J, Fan Y, et al. A risk score model based on endoplasmic reticulum stress related genes for predicting prognostic value of osteosarcoma. BMC Musculoskelet Disord 2023;24:519. [Crossref] [PubMed]
- Zhou P, Zhang J, Feng J, et al. Construction of an oxidative phosphorylation-related gene signature for predicting prognosis and identifying immune infiltration in osteosarcoma. Aging (Albany NY) 2024;16:5311-35. [Crossref] [PubMed]
- Zheng D, Xia K, Yu L, et al. A Novel Six Metastasis-Related Prognostic Gene Signature for Patients With Osteosarcoma. Front Cell Dev Biol 2021;9:699212. [Crossref] [PubMed]
- Langfelder P, Horvath S. WGCNA: an R package for weighted correlation network analysis. BMC Bioinformatics 2008;9:559. [Crossref] [PubMed]
- Li S, Han F, Qi N, et al. Determination of a six-gene prognostic model for cervical cancer based on WGCNA combined with LASSO and Cox-PH analysis. World J Surg Oncol 2021;19:277. [Crossref] [PubMed]
- Yin X, Wang P, Yang T, et al. Identification of key modules and genes associated with breast cancer prognosis using WGCNA and ceRNA network analysis. Aging (Albany NY) 2020;13:2519-38. [Crossref] [PubMed]
- Li A, Ji B, Yang Y, et al. Single-cell RNA sequencing highlights the role of PVR/PVRL2 in the immunosuppressive tumour microenvironment in hepatocellular carcinoma. Front Immunol 2023;14:1164448. [Crossref] [PubMed]
- Lai X, Liu N, Liu L, et al. Prognostic Prediction and Immunotherapy Analysis of Basement Membranes-Related Genes in Osteosarcoma Based on Bioinformatics. Available online: https://doi.org/
10.21203/rs.3.rs-2306174/v1 - Wu F, Xu J, Jin M, et al. Development and Verification of a Hypoxic Gene Signature for Predicting Prognosis, Immune Microenvironment, and Chemosensitivity for Osteosarcoma. Front Mol Biosci 2021;8:705148. [Crossref] [PubMed]
- Li J, Tang X, Du Y, et al. Establishment of an Autophagy-Related Clinical Prognosis Model for Predicting the Overall Survival of Osteosarcoma. Biomed Res Int 2021;2021:5428425. [Crossref] [PubMed]
- Zou J, Chen L, Xu H. Unveiling and validation of a disulfidptosis determined prognostic model for osteosarcoma: new insights from prognosis to immunotherapy and chemotherapy. Oncologie 2023;25:417-33.
- Branzei D, Foiani M. Regulation of DNA repair throughout the cell cycle. Nat Rev Mol Cell Biol 2008;9:297-308. [Crossref] [PubMed]
- Kastan MB, Bartek J. Cell-cycle checkpoints and cancer. Nature 2004;432:316-23. [Crossref] [PubMed]
- Kaufmann WK, Kaufman DG. Cell cycle control, DNA repair and initiation of carcinogenesis. FASEB J 1993;7:1188-91. [Crossref] [PubMed]
- Zhao Y, Ye X, Xiong Z, et al. Cancer Metabolism: The Role of ROS in DNA Damage and Induction of Apoptosis in Cancer Cells. Metabolites 2023;13:796. [Crossref] [PubMed]
- Dai F, Luo F, Zhou R, et al. Calponin 3 is associated with poor prognosis and regulates proliferation and metastasis in osteosarcoma. Aging (Albany NY) 2020;12:14037-49. [Crossref] [PubMed]
- Cai H, Miao M, Wang Z. miR-214-3p promotes the proliferation, migration and invasion of osteosarcoma cells by targeting CADM1. Oncol Lett 2018;16:2620-8. [Crossref] [PubMed]
- Ji Z, Chen W, Wan S, et al. MicroRNA-196-5p targets Derlin-1 to induce autophagy in human osteosarcoma cells. Acta Biochim Pol 2023;70:313-23. [Crossref] [PubMed]
- Chen Z, Li L, Li Z, et al. Identification of key serum biomarkers for the diagnosis and metastatic prediction of osteosarcoma by analysis of immune cell infiltration. Cancer Cell Int 2022;22:78. [Crossref] [PubMed]
- Man YN, Sun Y, Chen PJ, et al. TAF1D Functions as a Novel Biomarker in Osteosarcoma. J Cancer 2023;14:2051-65. [Crossref] [PubMed]
- Botter SM, Neri D, Fuchs B. Recent advances in osteosarcoma. Curr Opin Pharmacol 2014;16:15-23. [Crossref] [PubMed]
- Cai S, Xia Q, Duan D, et al. Creatine kinase mitochondrial 2 promotes the growth and progression of colorectal cancer via enhancing Warburg effect through lactate dehydrogenase B. PeerJ 2024;12:e17672. [Crossref] [PubMed]
Mamoor S. Differential expression of creatine kinase, mitochondrial 2 in cancers of the breast. 2021 . DOI: .- Zhuang B, Ni X, Min Z, et al. Long Non-Coding RNA CKMT2-AS1 Reduces the Viability of Colorectal Cancer Cells by Targeting AKT/mTOR Signaling Pathway. Iran J Public Health 2022;51:327-35. [Crossref] [PubMed]
- Girard F, Venail J, Schwaller B, et al. The EF-hand Ca(2+)-binding protein super-family: a genome-wide analysis of gene expression patterns in the adult mouse brain. Neuroscience 2015;294:116-55. [Crossref] [PubMed]
- Deng W, Wang L, Xiong Y, et al. The novel secretory protein CGREF1 inhibits the activation of AP-1 transcriptional activity and cell proliferation. Int J Biochem Cell Biol 2015;65:32-9. [Crossref] [PubMed]
- Hong X, Fu R. Construction of a 5-gene prognostic signature based on oxidative stress related genes for predicting prognosis in osteosarcoma. PLoS One 2023;18:e0295364. [Crossref] [PubMed]
- Zhang W, Lyu P, Andreev D, et al. Hypoxia-immune-related microenvironment prognostic signature for osteosarcoma. Front Cell Dev Biol 2022;10:974851. [Crossref] [PubMed]
- Zhang L, Wu S, Huang J, et al. A mitochondria-related signature for predicting immune microenvironment and therapeutic response in osteosarcoma. Front Oncol 2022;12:1085065. [Crossref] [PubMed]
- Yan L, Li R, Li D, et al. Development of a novel vasculogenic mimicry-associated gene signature for the prognostic assessment of osteosarcoma patients. Clin Transl Oncol 2023;25:3501-18. [Crossref] [PubMed]
- Li M, Song Q, Bai Y, et al. Comprehensive analysis of cuproptosis in immune response and prognosis of osteosarcoma. Front Pharmacol 2022;13:992431. [Crossref] [PubMed]
- Fridman WH, Galon J, Dieu-Nosjean MC, et al. Immune infiltration in human cancer: prognostic significance and disease control. Curr Top Microbiol Immunol 2011;344:1-24. [Crossref] [PubMed]
- Anderson NM, Simon MC. The tumor microenvironment. Curr Biol 2020;30:R921-5. [Crossref] [PubMed]
- Sokratous G, Polyzoidis S, Ashkan K. Immune infiltration of tumor microenvironment following immunotherapy for glioblastoma multiforme. Hum Vaccin Immunother 2017;13:2575-82. [Crossref] [PubMed]
- Pardoll DM. The blockade of immune checkpoints in cancer immunotherapy. Nat Rev Cancer 2012;12:252-64. [Crossref] [PubMed]
- Li B, Chan HL, Chen P. Immune Checkpoint Inhibitors: Basics and Challenges. Curr Med Chem 2019;26:3009-25. [Crossref] [PubMed]