Establishment and validation of predictive models by mutational and transcriptional factors for the prognosis of stage IV colorectal cancer patients with liver metastasis who undergo palliative surgery on primary tumors
Highlight box
Key findings
• Age, APC2 mutations and ADRB1 transcription were independent risk factors for the overall survival (OS). KRAS, PTPRF mutations and RFX8, MYEOV transcription were independent risk factors for the progression-free survival (PFS).
What is known and what is new?
• The prognosis of stage IV colorectal cancer patients with liver metastasis has been investigated and some clinicopathological factors, conventional markers and new molecular markers have been revealed as prognostic factors.
• Models for predicting the OS and PFS were successfully established with the independent risk factors and validated by internal and external validations.
What is the implication, and what should change now?
• The models established in this study may help to establish the personalized therapeutic strategies before treatment.
Introduction
Distant metastases are found in approximately 20–30% of colorectal cancer (CRC) patients at the time of their first diagnosis, and about 50% of these metastases occur in the liver (1). Due to the difficulties for curative treatment, the 5-year survival rate for stage IV CRC is less than 10% (1,2), and patients with CRC liver metastases have a worse prognosis than CRC patients without metastases. The current treatment for stage IV CRC patients with liver metastases is mainly systematic therapy, including chemotherapy, radiotherapy, targeted therapy, immunotherapy or multi-approach combined therapy (3,4). Palliative surgery combined with postoperative systematic therapy is also a common treatment method for such patients. The goal of surgery is to achieve remission by removing the cancer lesions and increase the disease maintenance likelihood for further adjuvant therapy (5,6), although the tumor cannot be radically removed for large majority of patients. Therefore, the purpose of the combined therapy is not to achieve radical treatment, but to relieve symptoms and related complications, extend survival time and improve quality of life.
The prognosis of stage IV CRC patients with liver metastasis has been investigated and some clinicopathological factors, conventional markers and new molecular markers have been revealed as prognostic factors. For example, pattern of distant metastases and primary tumor location were suggested as prognostic factors for the survival of CRC patients (7,8). Conventional serum protein markers, such as carcinoembryonic antigen (CEA), carbohydrate antigen 19-9 (CA199), heat shock protein 90 alpha and complement C5, were also suggested as diagnostic and prognostic markers for stage IV CRC with liver metastasis (9,10). More recently, methylation markers, such as methylated SEPT9, were also suggested as prognostic markers for CRC (11,12). Furthermore, prognostic models involving clinicopathological factors (13), competing endogenous RNA (ceRNA) network (14), and transcriptomic profiling (15) were developed for the prognosis of metastatic CRC.
Despite great advances in surgical techniques and new strategies in subsequent systemic therapy in recent years, patient relapse rates remain high for these patients (16,17). Due to the lack of effective biomarkers at mutational and transcriptional levels, it is currently difficult to predict prognosis and recurrence in these patients by genetic information, as the relationship between clinicopathological or genetic factors and disease prognosis is not fully understood in this specific group of patients. At the same time, there is currently no clear stratification of patient prognosis and risk of recurrence by genetic factors, which may lead to under-treatment or over-treatment. To identify factors that influence prognosis and recurrence in this specific population and to establish prognostic models, we for the first time combined clinicopathological factors with mutational and transcriptional factors. We enrolled patients with CRC liver metastases who underwent palliative primary tumor resection followed by adjuvant therapy. By whole-exome sequencing (WES) and transcriptome sequencing of the primary tissue, mutations and transcriptional variations of the primary tissue were obtained. Combined with clinicopathological data, significant genetic factors affecting the prognosis and recurrence of the specific group of patients were determined. Using these significant factors, we developed prognostic and recurrence prediction models and validated the models internally and externally. We hope that the establishment of the models can help patients to understand the risk and prognosis before treatment, and help doctors to choose appropriate treatment strategies and manage patient expectations. We present this article in accordance with the TRIPOD reporting checklist (available at https://tcr.amegroups.com/article/view/10.21037/tcr-24-1183/rc).
Methods
Ethics, patients and samples
This study is a database study combined with a retrospective cohort study. The study was conducted in accordance with the Declaration of Helsinki (as revised in 2013). The study was approved by the ethics committee of The Fourth Affiliated Hospital of Soochow University and conducted in accordance with the hospital’s guiding principles [approval number: (2021)-042; approval date: July, 2021]. The waiver of informed consent was granted by the hospital Ethics Committee due to the retrospective use of clinical samples in this study. Sample size estimation was performed with estimated AUC of 0.85 in internal and external validation for both overall survival (OS) and progression-free survival (PFS) prediction models, with type I error (alpha) set at 0.10 and type II error (beta) set at 0.20 and null hypothesis value at 0.70. An estimated sample size of 30 for internal and external validation cohort was obtained. For the training cohort, WES and ribonucleic acid sequencing (RNAseq) data were downloaded for 45 patients with stage IV CRC and liver metastasis who underwent primary cancer resection with adjuvant therapy. Data of patient OS and PFS was also collected from the database. For the external validation cohort, 30 stage IV CRC patients with liver metastasis from our hospital were retrospectively recruited. These patients underwent palliative surgery on primary CRC lesions and post-surgical adjuvant therapy. Clinicopathological information and follow-up information was also collected (Table 1). The duration of OS and PFS of patients in the validation cohort was matched to those in the training cohort as much as possible. Formalin fixed paraffin embedded (FFPE) tissues from the primary lesions of these patients were sent for WES and RNAseq sequencing. Patient information was kept anonymous for confidentiality throughout the study.
Table 1
Clinicopathological factors | Training cohort | Validation cohort |
---|---|---|
Total (stage IV) | 45 (100.0) | 30 (100.0) |
Sex | ||
Male | 24 (53.3) | 17 (56.7) |
Female | 21 (46.7) | 13 (43.3) |
Age (years, median: 66) | ||
≤66 | 23 (51.1) | 15 (50.0) |
>66 | 22 (48.9) | 15 (50.0) |
Location | ||
Colon sigmoideum | 15 (33.3) | 10 (33.3) |
Ceceum | 11 (24.4) | 9 (30.0) |
Colon ascendens | 9 (20.0) | 3 (10.0) |
Flexura lienalis | 4 (8.9) | 2 (6.7) |
Colon transversum | 3 (6.7) | 4 (13.3) |
Flexura hepatica | 2 (4.4) | 2 (6.7) |
Rectosigmoideum | 1 (2.2) | 0 (0.0) |
Adjuvant treatment | ||
None | 18 (40.0) | 13 (43.3) |
Yes | 27 (60.0) | 17 (56.7) |
PFS (days) | 264 [23–4,699] | 272 [23–2,259] |
OS (days) | 823 [23–4,699] | 980 [23–2,380] |
Data are presented as n (%) or median [range]. PFS, progression-free survival; OS, overall survival.
The inclusion criteria included: (I) age ≥18 years, both male and female; (II) stage IV CRC patients with confirmed liver metastasis; (III) patients who had resection of primary cancer lesions and received subsequent adjuvant therapy; (IV) patients with available FFPE samples of primary CRC lesions; (V) patients whose clinicopathological and follow-up information was complete. The exclusion criteria included: (I) patients who did not receive surgery with adjuvant therapy; (II) patients who had a history of non-CRC cancers; (III) samples were not available for sequencing test.
As a result, a total of 75 patients were available for sequencing analysis based on the above criteria, including a training cohort with 45 patients from the cBioportal database (http://www.cbioportal.org/) and an independent validation cohort with 30 patients from our hospital (Table 1). FFPE samples from primary cancer lesions were collected and sent for sequencing, using the paracancerous normal tissues as the control. The demographic and clinicopathological information for all patients involved in training and validation cohorts is summarized in Table 1.
WES and data processing
Deoxyribonucleic acid (DNA) from FFPE samples of cancer tissues and paracancerous normal tissues was extracted using QIAamp DNA FFPE Tissue Kit (Qiagen Shanghai, Shanghai, China). Sample processing, quality control, library construction and sequencing procedures were performed as described previously (18). Data were generated and processed and analysis was performed as described previously (18).
RNA sequencing (RNAseq) and data processing
Ribonucleic acid (RNA) from FFPE samples of cancer tissues and paracancerous normal tissues was extracted using the TruSeq Targeted RNA Expression Library Prep Kit (Illumina China, Shanghai, China). Sample processing, quality control, library construction and sequencing were performed as described previously (19). RNA-seq and data processing were performed and data were analyzed as described previously (19).
Statistical analysis
Mutational alterations and significant mRNA alterations obtained from sequencing experiments (Figures 1,2), combined with demographic and clinicopathological factors, were correlated with patient prognosis (OS and PFS) in univariate and multivariate analyses (Tables 2,3). Kaplan-Meier survival analysis was performed with individual demographic, clinicopathological, mutational and transcriptional factors (Figures 3,4). Significant factors from multivariate analysis were used to establish prognostic models regarding OS and PFS, and Nomograms were plotted to illustrate the models. Receiver operating characteristics (ROCs) were used to assess the performance of the models (Figures 5,6).
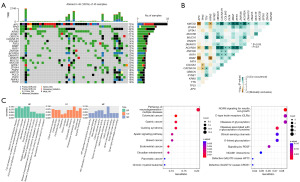
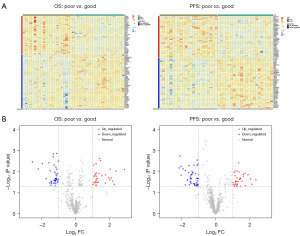
Table 2
Variables | Number [%] or range | Univariate | Multivariate | |||
---|---|---|---|---|---|---|
HR (95% CI) | P value | HR (95% CI) | P value | |||
Age (years) | 26–87 | 1.03 (1.00, 1.06) | 0.06 | 1.04 (1.01, 1.07) | 0.008 | |
APC2 | 9.41 (3.11, 28.46) | 11.87 (3.17, 44.41) | <0.001 | |||
Wild-type | 39 [87] | |||||
Mutation | 6 [13] | |||||
MRPL23-AS1 | 0.39 (0.19, 0.78) | 0.008 | 0.48 (0.20, 1.17) | 0.11 | ||
Low expression | 23 [51] | |||||
High expression | 22 [49] | |||||
ADRB1 | 2.45 (1.22, 4.89) | 0.01 | 2.41 (1.06, 5.49) | 0.04 | ||
Low expression | 23 [51] | |||||
High expression | 22 [49] | |||||
PDZK1 | 0.38 (0.19, 0.76) | 0.005 | 0.55 (0.23, 1.29) | 0.17 | ||
Low expression | 23 [51] | |||||
High expression | 22 [49] | |||||
ASTL | 2.33 (1.16, 4.69) | 0.02 | 0.72 (0.29, 1.82) | 0.49 | ||
Low expression | 23 [51] | |||||
High expression | 22 [49] |
HR, hazard ratio; CI, confidence interval.
Table 3
Variables | Number [%] | Univariate | Multivariate | |||
---|---|---|---|---|---|---|
HR (95% CI) | P value | HR (95% CI) | P value | |||
KRAS | 2.03 (1.04, 3.96) | 0.04 | 3.26 (1.53, 6.97) | 0.002 | ||
Wild-type | 27 [60] | |||||
Mutation | 18 [40] | |||||
PTPRF | 13.10 (3.66, 46.94) | <0.001 | 14.59 (1.88, 112.97) | 0.01 | ||
Wild-type | 39 [87] | |||||
Mutation | 6 [13] | |||||
FREM2 | 4.89 (1.71, 14.03) | 0.003 | 1.84 (0.44, 7.75) | 0.41 | ||
Wild-type | 40 [89] | |||||
Mutation | 5 [11] | |||||
CLOCK | 8.54 (2.83, 25.82) | <0.001 | 1.73 (0.25, 12.22) | 0.58 | ||
Wild-type | 40 [89] | |||||
Mutation | 5 [11] | |||||
LY6H | 0.45 (0.23, 0.87) | 0.02 | 0.41 (0.15, 1.15) | 0.09 | ||
Low expression | 23 [51] | |||||
High expression | 22 [49] | |||||
TMEM163 | 0.37 (0.19, 0.72) | 0.004 | 0.59 (0.26, 1.35) | 0.21 | ||
Low expression | 23 [51] | |||||
High expression | 22 [49] | |||||
RFX8 | 0.41 (0.21, 0.80) | 0.009 | 0.38 [0.17, 0.88) | 0.02 | ||
Low expression | 23 [51] | |||||
High expression | 22 [49] | |||||
ARHGDIG | 0.42 (0.22, 0.80) | 0.009 | 0.43 (0.17, 1.09) | 0.08 | ||
Low expression | 23 [51] | |||||
High expression | 22 [49] | |||||
TECTA | 0.32 (0.16, 0.64) | 0.001 | 0.56 (0.21, 1.5) | 0.25 | ||
Low expression | 23 [51] | |||||
High expression | 22 [49] | |||||
MYEOV | 1.91 (1.01, 3.62) | 0.049 | 5.82 (1.98, 17.05) | 0.001 | ||
Low expression | 23 [51] | |||||
High expression | 22 [49] |
HR, hazard ratio; CI, confidence interval.

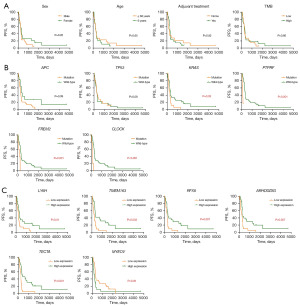
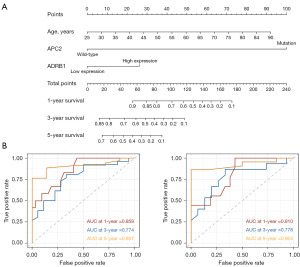
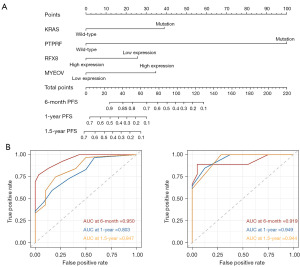
Data analyses were performed and figures were plotted using the R software (https://www.r-project.org/). The mutational landscape was generated and plotted using the ‘Complex Heatmaps’ package (Figure 1). The transcriptional data were analyzed using the “DESeq2” package, and the volcano plot and heatmap were generated (Figure 2). Enrichment analyses were conducted using the “clusterProfiler” package of the R software (Figure 2). The Kaplan-Meier survival analysis was performed using the “survival” and “survminer” packages (Figures 3,4). Univariate and multivariate regression analyses were performed using the R software (Tables 2,3). The Nomogram model was established with the corresponding package of the R software. The “survivalROC” package of the R software was used to plot the ROC curve and calculate the area under the curve (AUC) (Figures 5,6). Two-sided P values were calculated for univariate and multivariate analyses and survival analysis. All P values were corrected by Benjamini & Hochberg (BH) method. P<0.05 was considered statistically significant.
Results
Identification of prognostic factors by characterization of mutational and transcriptional alterations in stage IV CRC
To identify the genetic factors related to patient prognosis, the mutational and transcriptional alterations and their features were first characterized. Figure 1 shows the mutational landscape and results of enrichment analysis for stage IV CRC patients in this study. The mutational landscape in Figure 1A shows that genes with the highest mutational frequency included APC, TP53, TTN, KRAS and SYNE1. Missense mutation was the predominant mutational type, while frameshift, nonsense and multiple hit mutations were also present. Further analysis in Figure 1B shows the landscape of co-mutations and mutually exclusive mutations. KMT2D and ACVR2A showed the most prominent co-mutations with OBSCN, CACNA1H, FAT4, BRAF, RYR1 and ZNF536, etc., while APC and KRAS showed mutually exclusive mutations with KMT2D and BRAF. This analysis revealed intrinsic correlations between mutations in stage IV CRC patients. Enrichment analyses in Figure 1C show comprehensive aberrant functions or pathways. Gene Ontology (GO) enrichment revealed aberrancies in membrane functions, membrane protein and ion channel functions. Kyoto Encyclopedia of Genes and Genomes (KEGG) and Reactome enrichments revealed abnormalities in neurodegeneration pathways, neural cell adhesion molecule (NCAM) signaling, glycosylation, and a series overlaps with other cancers, including gastric, breast and endometrial cancer.
The transcriptional analysis was performed by comparing the transcriptional differences between patients with good prognosis and poor prognosis (OS and PFS). Heatmap and volcano plot in Figure 2 show the characteristics in transcriptional alterations. Many upregulated and downregulated genes can be found in patients with good prognosis or poor prognosis in terms of OS and PFS (Figure 2A). Among these genes, significantly upregulated and downregulated genes can be found with volcano plot in Figure 2B, using −log10(P value) =1.3 (P=0.050) and |log2FC| =1 as the thresholds, including some genes with significant stratification of OS in later analysis, such as ADRB1, ASTL, MRPL23-AS1 and PDZK1 (Figure 3), and some genes with significant stratification of PFS in later analysis, such as LY6H, TMEM163, RFX8, ARHGDIG, TECTA and MYEOV (Figure 4).
The stratification on patient OS by clinicopathological, mutational and transcriptional factors was then investigated. It can be seen from Figure 3 that age, APC2 mutational status, and the transcriptional status of ADRB1, ASTL, MRPL23-AS1 and PDZK1 significantly stratified patient OS, while other factors, including sex, adjuvant therapy, TMB and the mutational status of APC, TP53 and KRAS could not stratify the patient OS. The stratification on patient PFS by clinicopathological, mutational and transcriptional factors was also investigated. Figure 4 shows that the mutational status of KRAS, PTPRF, FREM2, CLOCK and the transcription status of LY6H, TMEM163, RFX8, ARHDGDIG, TECTA and MYEOV were significant stratifying factors, while other factors could not significantly stratify the patient PFS.
Establishment and validation of OS and PFS models enable the prediction of prognosis in stage IV CRC patients
To identify the risk factors for patient prognosis, which is represented by OS and PFS, univariate analysis and subsequent multivariate analysis were performed, and corresponding models were established and validated for OS and PFS prediction, respectively. For the training cohort, patients with OS up to 4,699 days (median at 823 days, range, 23–4,699 days) were recruited. For the validation cohort, patients with OS up to 2,380 days (median at 980 days, range, 23–2,380 days) were recruited. Table 2 shows the results of univariate and multivariate analyses of risk factors for OS. Age, APC2 mutational status and the transcriptional status of MRPL23-AS1, ADRB1, PDZK1 and ASTL were risk factors from univariate analysis, while only age, APC2 mutational status and ADRB1 transcriptional status were independent risk factors from multivariate analysis. These significant risk factors were used to establish a Cox regression risk prediction model for OS, illustrated by the Nomogram diagram in Figure 5A, in which the probability of 1-, 3- and 5-year OS can be calculated. Internal and external validation were performed to examine the model efficacy (Figure 5B). The 1-, 3- and 5-year AUC reached 0.858, 0.774 and 0.907 in internal validation, respectively, and reached 0.810, 0.778 and 0.924 in external validation, respectively.
The risk factors for PFS were also investigated by univariate analysis and subsequent multivariate analysis. For the training cohort, patients with PFS up to 4,699 days (median at 264 days, range, 23–4,699 days) were recruited. For the validation cohort, patients with PFS up to 2,259 days (median at 272 days, range, 23–2,259 days) were recruited. Table 3 shows a series of significant risk factors in univariate analysis, including the mutational status of four genes and the transcriptional status of six genes. However, only the mutational status of KRAS and PTPRF and the transcriptional status of RFX8 and MYEOV were independent risk factors in multivariate analysis. These significant risk factors were used to establish a Cox regression risk prediction model for PFS, illustrated by the Nomogram diagram in Figure 6A, in which the probability of 6 months, 1-year and 1.5-year PFS can be calculated. Internal and external validation were performed to examine the model efficacy (Figure 6B). The 6 months, 1-year and 1.5-year AUC reached 0.950, 0.803 and 0.847 in internal validation, respectively, and reached 0.919, 0.949 and 0.944 in external validation, respectively.
Discussion
Palliative surgery and postoperative adjuvant therapy are one of the methods for the treatment of primary CRC with liver metastasis. Palliative surgery is not a cure, but can be used as palliative care to relieve cancer-related symptoms and complications, resulting in longer survival and improved quality of life (5,6). However, prognostic factors are not clear for stage IV CRC patients with liver metastases who undergo palliative surgery and postoperative systemic therapy. In this study, we examined clinicopathological, mutational, and transcriptional factors that may influence patient survival and predict recurrence. By looking for significant factors related to prognosis and recurrence, prognosis and recurrence prediction models were established and validated, which are expected to be used clinically to predict the survival and recurrence of such patients, and establish a basis for treatment strategies and reasonable expectations.
Mutational profiles of primary cancer and liver metastases associated with stage IV CRC patients have been widely reported, and most studies support the idea that stage IV CRC is mutationally similar to stage I–III CRC (20). In this study, we also found that driver genes such as APC, TP53, TTN, KRAS and BRAF are high-frequency mutations in stage IV CRC patients, and we also identified a series of characteristic co-mutations and mutually exclusive mutations, including several major driver genes. For example, APC and KRAS were mutually exclusive to BRAF, and BRAF was significantly co-mutated with KMT2D. Significant co-mutations or mutually exclusive mutations between these driver genes suggest that stage IV CRC presented highly heterogeneous molecular typing and that the clonal evolution was sufficiently advanced, and these characteristics may serve as predictors of therapeutic response and prognosis (20). We also noted that patients with stage IV CRC showed high frequency mutations in ion channels, especially calcium channels, including CACNA1H, RYR1, and RYR2, which were intensively studied in a recent report on ion channels in cancer (21).
APC2 mutations show a strong prognostic effect in the current patient cohort. Patients with APC2 mutations showed poor OS, suggesting that patients with APC2 mutations may represent a molecular subtype with poor prognosis. The APC2 gene is a member of the APC gene family and plays an important role in regulating the WNT signaling pathway (22). APC2 encodes a highly conserved protein that facilitates the assembly of multiprotein complexes. These multiprotein complexes recruit, phosphorylate and ubiquitinate β-catenin and degrade proteasome (23). Therefore, APC2 plays an important role in reducing cytoplasmic β-catenin levels and further reducing the activation of Wnt target genes, which play important roles in CRC pathogenesis. The protein encoded by APC2 is closely related to the APC tumor suppressor protein and has similar tumor suppressive effects (22). It also plays a role in actin assembly, intercellular adhesion, and microtubule network formation through interactions with cytoskeletal proteins (23). Therefore, the dysfunction of the corresponding proteins caused by gene mutations of APC2 may lead to the abnormal regulation of Wnt signaling pathway, promote the occurrence and progression of CRC, and may be associated with poor prognosis. Therefore, it is understandable that patients with APC2 gene mutation exhibited worse OS in this study.
In contrast, KRAS, PTPRF, FREM2 and CLOCK mutations were significantly associated with patients’ recurrence. It is worth noting that patients with wild type had better PFS than those with mutations, indicating that patients with these mutations had earlier recurrence. For example, KRAS is a known important driver gene for CRC. In this study, the mutation frequency of KRAS in the population reached 40%, and the high-frequency mutation mainly occurred in G12, which is a clearly targeted drug resistance mutation associated with poor prognosis (24). This study found that patients with KRAS mutations had worse PFS than those with wild type, indicating that abnormal activation of KRAS caused by mutation may promote the occurrence and development of tumors, resulting in patients with KRAS mutation being more likely to relapse. Previous studies have shown that RAS mutations were a negative prognostic factor affecting the overall and relapse-free survival in patients with stage IV CRC with resectable or unresectable liver metastases, and BRAF mutations were also strongly associated with poorer prognosis (25), while TP53 and PIK3CA mutations do not affect long-term prognosis (26). In this study, we confirm these observations. However, conclusions from individual studies may differ due to different populations, mutations, and treatments. The effect of mutations in the other three genes (PTPRF, FREM2, and CLOCK) on CRC recurrence was not found in previous reports, and further studies on the role of these gene mutations in prognosis and recurrence prediction in different CRC populations are warranted. Since WES method was used to analyze the mutation landscape of primary cancer tissue in this study, the number of genes and the exon region were highly covered, which ensured high confidence and low bias, and the role of some mutations that had not been reported before may be found.
The transcriptional analysis in this study directly compared the differences in the transcriptome of patients with different prognoses represented by the stratification in OS or PFS, which is conducive to directly searching for risk factors influencing prognosis. Since transcriptional changes were widespread in CRC, we observed more transcriptional changes than mutational changes that can stratify patient prognosis. However, not all transcriptional changes were decisive factors for prognosis, therefore, both univariate and multivariate analyses were necessary to identify independent risk factors for model establishment. The predictive model for OS identified ADRB1 transcription as an independent risk factor, and the model for PFS identified RFX8 and MYEOV transcription as independent risk factors. ADRB1 encodes the adrenoceptor β1 protein, which mediates the physiological effects of neurotransmitters, including norepinephrine and adrenaline. The β1 adrenergic receptor is primarily found in the heart, and polymorphisms of this gene have been shown to affect the resting heart rate and may be associated with heart failure (27). In terms of cancer, studies have reported that ADRB1 was associated with the development of cervical cancer (28) and breast cancer (29) etc., but no reports have been found in CRC. Regulatory factor X8 (RFX8) is suggested to be located in nucleus and is a part of chromatin. It may function as a DNA-binding transcription factor (30). Limited evidence suggested that RFX8 may promote the progression of hepatocellular carcinoma by upregulating DEAD-box helicase 24 (DDX24) expression (31). In contrast, myeloma overexpressed (MYEOV) overexpression was reported in many cancers, including myeloma (32), CRC (33), non-small cell lung cancer (34) and breast cancer (32), etc.
In this study, we established prediction models for OS and PFS by combining clinicopathological, mutational and transcriptional information. Multi-omics model for cancer prognosis prediction has become a hot spot in cancer research in recent years. Integration of information from multiple omics and clinicopathological factors and identification of markers with the best predictive efficacy help to optimize models and provide high performance. However, one should balance the number of omics or markers involved in a model with the performance. Too many markers may lead to overfitting in model training, and the best strategy is to only include those markers with sufficient weight and good efficacy (35). This ensures a model with minimal necessary markers and optimal performance. The models established in this study involved markers with significant predictive performance from multivariate analysis, which ensured both minimal marker numbers and performance.
The model established in this study has the potential to be used in clinical practice and to benefit patients. The tissue for mutation and transcriptome sequencing can be from preoperative or postoperative pathological tissue, which can be fresh tissue, frozen tissue or FFPE tissue. Next generation sequencing (NGS)-based WES and RNAseq can be completed with relatively low cost and identify the mutation and transcriptional abnormalities of CRC in patients in a short time. The alterations detected by NGS, combined with clinicopathological information, can be used to predict patients’ OS and PFS using the model of this study and give probabilities of survival and likelihood of recurrence. For patients with a greater risk of recurrence or poor OS, more aggressive therapies such as surgery plus combined therapy involving chemotherapy, targeted therapy or immunotherapy should be considered. Furthermore, preoperative neoadjuvant therapy may be an option for these patients, which may improve patient prognosis than direct surgery without neoadjuvant therapy. Since patients are tested for mutations, the test results can also be used to guide targeted therapy or immunotherapy, helping to choose the best therapeutic strategy.
The study had some limitations. First of all, different adjuvant treatments may affect the prognosis of patients. In subsequent studies, the prognosis can be studied in patients grouped by different adjuvant treatments. Second, although internal and external validation was performed in this study, the validation was retrospective, and future validation of the models can be considered in prospective populations.
Conclusions
Taken together, this study was the first to identify clinicopathological, mutational, and transcriptional factors that can be used to predict the prognosis and recurrence in stage IV CRC patients with liver metastases who underwent primary resection and subsequent adjuvant therapy. The models developed using these significant factors showed high performance in predicting patients’ OS and PFS. Internal validation and external validation ensured the validity of the model. In clinical practice, predication of patient prognosis using the models in this study may help to establish the personalized therapeutic strategies before treatment and manage physician and patient expectations of response and prognosis.
Acknowledgments
None.
Footnote
Reporting Checklist: The authors have completed the TRIPOD reporting checklist. Available at https://tcr.amegroups.com/article/view/10.21037/tcr-24-1183/rc
Data Sharing Statement: Available at https://tcr.amegroups.com/article/view/10.21037/tcr-24-1183/dss
Peer Review File: Available at https://tcr.amegroups.com/article/view/10.21037/tcr-24-1183/prf
Funding: None.
Conflicts of Interest: Both authors have completed the ICMJE uniform disclosure form (available at https://tcr.amegroups.com/article/view/10.21037/tcr-24-1183/coif). The authors have no conflicts of interest to declare.
Ethical Statement: The authors are accountable for all aspects of the work in ensuring that questions related to the accuracy or integrity of any part of the work are appropriately investigated and resolved. The study was conducted in accordance with the Declaration of Helsinki (as revised in 2013). This research was approved by the ethics committee of The Fourth Affiliated Hospital of Soochow University and conducted in accordance with the hospital’s guiding principles [approval number: (2021)-042; approval date: July, 2021]. The waiver of informed consent was granted by the hospital Ethics Committee due to the retrospective use of clinical samples in this study.
Open Access Statement: This is an Open Access article distributed in accordance with the Creative Commons Attribution-NonCommercial-NoDerivs 4.0 International License (CC BY-NC-ND 4.0), which permits the non-commercial replication and distribution of the article with the strict proviso that no changes or edits are made and the original work is properly cited (including links to both the formal publication through the relevant DOI and the license). See: https://creativecommons.org/licenses/by-nc-nd/4.0/.
References
- Engstrand J, Nilsson H, Strömberg C, et al. Colorectal cancer liver metastases - a population-based study on incidence, management and survival. BMC Cancer 2018;18:78. [Crossref] [PubMed]
- Kelly ME, Spolverato G, Lê GN, et al. Synchronous colorectal liver metastasis: a network meta-analysis review comparing classical, combined, and liver-first surgical strategies. J Surg Oncol 2015;111:341-51. [Crossref] [PubMed]
- Zhou H, Liu Z, Wang Y, et al. Colorectal liver metastasis: molecular mechanism and interventional therapy. Signal Transduct Target Ther 2022;7:70. [Crossref] [PubMed]
- Shin AE, Giancotti FG, Rustgi AK. Metastatic colorectal cancer: mechanisms and emerging therapeutics. Trends Pharmacol Sci 2023;44:222-36. [Crossref] [PubMed]
- Costi R, Leonardi F, Zanoni D, et al. Palliative care and end-stage colorectal cancer management: the surgeon meets the oncologist. World J Gastroenterol 2014;20:7602-21. [Crossref] [PubMed]
- Akgül Ö, Çetinkaya E, Ersöz Ş, et al. Role of surgery in colorectal cancer liver metastases. World J Gastroenterol 2014;20:6113-22. [Crossref] [PubMed]
- Lian L, Xu XF, Shen XM, et al. Pattern of distant metastases and predictive nomograms in colorectal mucinous adenocarcinoma: a SEER analysis. J Gastrointest Oncol 2021;12:2906-18. [Crossref] [PubMed]
- Yin H, Li H, Xu J, et al. Primary tumor location impacts survival in colorectal cancer patients after primary resection: a population‑based propensity score matching cohort study. J Gastrointest Oncol 2023;14:886-99. [Crossref] [PubMed]
- Ding Q, Sun Y, Zhang J, et al. Utility and specificity of plasma heat shock protein 90 alpha, CEA, and CA199 as the diagnostic test in colorectal cancer liver metastasis. J Gastrointest Oncol 2022;13:2497-504. [Crossref] [PubMed]
- Chang H, Jin L, Xie P, et al. Complement C5 is a novel biomarker for liver metastasis of colorectal cancer. J Gastrointest Oncol 2022;13:2351-65. [Crossref] [PubMed]
- Song L, Chen Y, Gong Y, et al. Opportunistic screening and survival prediction of digestive cancers by the combination of blood mSEPT9 with protein markers. Ther Adv Med Oncol 2020;12:1758835920962966. [Crossref] [PubMed]
- Song L, Yu H, Jia J, et al. A systematic review of the performance of the SEPT9 gene methylation assay in colorectal cancer screening, monitoring, diagnosis and prognosis. Cancer Biomark 2017;18:425-32. [Crossref] [PubMed]
- Dou X, Xi J, Zheng G, et al. A nomogram was developed using clinicopathological features to predict postoperative liver metastasis in patients with colorectal cancer. J Cancer Res Clin Oncol 2023;149:14045-56. [Crossref] [PubMed]
- Zhang X, Wu T, Zhou J, et al. Establishment and verification of prognostic model and ceRNA network analysis for colorectal cancer liver metastasis. BMC Med Genomics 2023;16:99. [Crossref] [PubMed]
- Moosavi SH, Eide PW, Eilertsen IA, et al. De novo transcriptomic subtyping of colorectal cancer liver metastases in the context of tumor heterogeneity. Genome Med 2021;13:143. [Crossref] [PubMed]
- Matias M, Casa-Nova M, Faria M, et al. Prognostic Factors after Liver Resection for Colorectal Liver Metastasis. Acta Med Port 2015;28:357-69. [Crossref] [PubMed]
- Kassahun WT. Unresolved issues and controversies surrounding the management of colorectal cancer liver metastasis. World J Surg Oncol 2015;13:61. [Crossref] [PubMed]
- He Y, Song L, Wang H, et al. Mutational Profile Evaluates Response and Survival to First-Line Chemotherapy in Lung Cancer. Adv Sci (Weinh) 2020;8:2003263. [Crossref] [PubMed]
- Cheng J, Li Y, Wang X, et al. Response Stratification in the First-Line Combined Immunotherapy of Hepatocellular Carcinoma at Genomic, Transcriptional and Immune Repertoire Levels. J Hepatocell Carcinoma 2021;8:1281-95. [Crossref] [PubMed]
- Guo TA, Wu YC, Tan C, et al. Clinicopathologic features and prognostic value of KRAS, NRAS and BRAF mutations and DNA mismatch repair status: A single-center retrospective study of 1,834 Chinese patients with Stage I-IV colorectal cancer. Int J Cancer 2019;145:1625-34. [Crossref] [PubMed]
- Wang F, Yu J, Lin P, et al. The ryanodine receptor mutational characteristics and its indication for cancer prognosis. Sci Rep 2022;12:16113. [Crossref] [PubMed]
- Meng F, Liu X, Lin C, et al. SMYD2 suppresses APC2 expression to activate the Wnt/β-catenin pathway and promotes epithelial-mesenchymal transition in colorectal cancer. Am J Cancer Res 2020;10:997-1011. [PubMed]
- Croy HE, Fuller CN, Giannotti J, et al. The Poly(ADP-ribose) Polymerase Enzyme Tankyrase Antagonizes Activity of the β-Catenin Destruction Complex through ADP-ribosylation of Axin and APC2. J Biol Chem 2016;291:12747-60. [Crossref] [PubMed]
- Zhu G, Pei L, Xia H, et al. Role of oncogenic KRAS in the prognosis, diagnosis and treatment of colorectal cancer. Mol Cancer 2021;20:143. [Crossref] [PubMed]
- Sanz-Garcia E, Argiles G, Elez E, et al. BRAF mutant colorectal cancer: prognosis, treatment, and new perspectives. Ann Oncol 2017;28:2648-57. [Crossref] [PubMed]
- Li AJ, Li HG, Tang EJ, et al. PIK3CA and TP53 mutations predict overall survival of stage II/III colorectal cancer patients. World J Gastroenterol 2018;24:631-40. [Crossref] [PubMed]
- Guerra LA, Lteif C, Arwood MJ, et al. Genetic polymorphisms in ADRB2 and ADRB1 are associated with differential survival in heart failure patients taking β-blockers. Pharmacogenomics J 2022;22:62-8. [Crossref] [PubMed]
- Zheng X, Xu S, Wu J. Cervical Cancer Imaging Features Associated With ADRB1 as a Risk Factor for Cerebral Neurovascular Metastases. Front Neurol 2022;13:905761. [Crossref] [PubMed]
- Wang J, Zhang X, Li J, et al. ADRB1 was identified as a potential biomarker for breast cancer by the co-analysis of tumor mutational burden and immune infiltration. Aging (Albany NY) 2020;13:351-63. [Crossref] [PubMed]
- Available online: https://www.ncbi.nlm.nih.gov/gene/731220
- Liu T, Gan H, He S, et al. RNA Helicase DDX24 Stabilizes LAMB1 to Promote Hepatocellular Carcinoma Progression. Cancer Res 2022;82:3074-87. [Crossref] [PubMed]
- Davidson BSA, Arcila-Galvis JE, Trevisan-Herraz M, et al. Evolutionarily conserved enhancer-associated features within the MYEOV locus suggest a regulatory role for this non-coding DNA region in cancer. Front Cell Dev Biol 2024;12:1294510. [Crossref] [PubMed]
- Lawlor G, Doran PP, MacMathuna P, et al. MYEOV (myeloma overexpressed gene) drives colon cancer cell migration and is regulated by PGE2. J Exp Clin Cancer Res 2010;29:81. [Crossref] [PubMed]
- Luo S, Luo Y, Wang Z, et al. Super-enhancer mediated upregulation of MYEOV suppresses ferroptosis in lung adenocarcinoma. Cancer Lett 2024;589:216811. [Crossref] [PubMed]
- Yang R, Wu Y, Qi Y, et al. A nomogram for predicting breast cancer specific survival in elderly patients with breast cancer: a SEER population-based analysis. BMC Geriatr 2023;23:594. [Crossref] [PubMed]