Prognostic development and validation of a prediction model based on major histocompatibility complex-related differentially expressed genes in stomach adenocarcinoma
Highlight box
Key findings
• We screened five key major histocompatibility complex-related differentially expressed genes. A prediction model was constructed based on these five genes.
What is known and what is new?
• Stomach adenocarcinoma is a prevalent and deadly form of cancer, and the role of major histocompatibility complex in its development is unclear and requires more research.
• We identified five key genes and combined them with clinical information to construct a clinical prognosis prediction model.
What is the implication, and what should change now?
• Clinical prognostic prediction models have shown good predictive results and further experiments are needed to demonstrate differential gene expression.
Introduction
Stomach adenocarcinoma (STAD) is the fifth most common cancer worldwide and the third leading cause of cancer-related deaths (1,2). Despite a steady decline in morbidity and mortality in recent years, the world continues to experience more than 1 million new cases each year (3,4). Due to a lack of effective diagnostic and therapeutic strategies, STAD typically exhibits a poor prognosis with elevated mortality rates (5). Patients with STAD and peritoneal metastasis experience a median survival time of 4–12 months, with a 5-year survival rate below 5% (6). Serum tumor markers have become an option to diagnose and monitor cancer progression and are closely associated with the occurrence, recurrence, and metastasis of STAD. However, their clinical significance remains controversial due to issues with diagnostic sensitivity and specificity (7). Based on the development of next-generation sequencing technologies, more and more genes and RNAs with mechanisms of action in tumors have been identified and investigated, making the search for a sensitive STAD early diagnostic and prognostic marker imperative (8,9). Similarly, immunotherapy plays a crucial role in the treatment of advanced STAD, despite its expanding clinical use, with some patients yet to experience significant survival benefits (10). There is an urgent need to discover effective diagnostic markers and novel potential therapeutic targets.
The biological function of the major histocompatibility complex (MHC) is to deliver protein antigens to T cells, and human leukocyte antigen (HLA) is the expression product of MHC. The HLA gene complex is located on the short arm of human chromosome 6, 6p21.31, with a total length of 3,600 kb, and a total of 224 gene seats, of which 128 are functional genes (with product expression) and 96 are pseudogenes (11). Based on the location and function of the genes, HLA genes could be classified into three types: class I, II, and III gene regions (12). HLA-I molecules are located on the surface of nucleated cells and are mainly involved in the processing and presentation of endogenous antigens; HLA-II molecules are located on antigen-presenting cells (APCs) and are mainly engaged in the processing and presentation of the exogenous antigen; and HLA-III genes encode mainly for the complement components, including tumor necrosis factor (TNF), heat shock protein (HSP), etc. (13,14). Although HLA plays an important role in the diagnosis and treatment of STAD, the specific functions and modes of action are still unclear, and further systematic studies are needed (15).
HLA molecules could play a role in regulating tumor cell metastasis and suppressing anti-tumour immunity in various ways (16). Studies demonstrated that in EBV-associated STAD, Epstein-Barr virus (EBV) could mediate the process of immune evasion by down-regulating the expression of HLA-I molecules on the cell surface, thereby avoiding the immune surveillance by cytotoxic T-lymphocytes and natural killer cells (17,18). A study by Ghasemi et al. also illustrated that compared to other STAD subtypes, almost all MHC-II genes were significantly up-regulated and showed better prognostic outcomes (19). In addition, soluble human leukocyte antigen E (HLA-E), which is immunotolerant, has been shown to promote the immune escape of gastric cancer (GC) cells and might be a potential prognostic biomarker (20).
However, most of the previous bioinformatics studies only focused on individual differentially expressed genes (DEGs) and prognosis and did not consider MHC-related genes as a whole, nor constructed a complete prognostic model and evaluation system, or lacked experimental validation. We developed a nomogram clinical prognosis prediction model based on the results of one-way Cox regression analysis and evaluated the effect. We also performed immunogold-run analysis, gene mutation analysis, drug sensitivity analysis, and experimental validation to further explore its clinical application. We present this article in accordance with the TRIPOD reporting checklist (available at https://tcr.amegroups.com/article/view/10.21037/tcr-24-707/rc).
Methods
Data download
We used the R package TCGAbiolinks (21) from The Cancer Genome Atlas (TCGA) (https://portal.gdc.cancel.gov/) to download the expression matrix of the STAD dataset, and obtained count sequencing data of 408 STAD samples with clinical information. A total of 375 cases of STAD samples (cancer group) and 32 cases of para-cancer samples (normal group) were included in this study. The study was conducted in accordance with the Declaration of Helsinki (as revised in 2013).
We also downloaded STAD-related datasets GSE26899 (22) and GSE113255 (23) from the Gene Expression Omnibus (GEO) database (24) through the R package GEOquery. Among them, GSE26899 included microarray gene expression profile data of 96 GC patients and 12 adjacent normal tissue samples. GSE113255 included microarray gene expression profile data of 130 GC patients and 10 adjacent normal tissue samples. All samples were included in this study. Our STAD datasets GSE26899 and GSE113255 were merged, and the R package sva package was used to remove the batch effect, then the limma package was used to standardize the combined datasets to obtain the STAD GEO dataset. The corresponding GPL (Gene Expression Omnibus Platform) files were used for the probe names annotation of the datasets, and both GEO datasets were used as validation sets. The data set information is shown in Table 1.
Table 1
TCGA-STAD | GSE26899 | GSE113255 | |
---|---|---|---|
Platform | – | GPL6947 | GPL18573 |
Species | Homo sapiens | Homo sapiens | Homo sapiens |
Samples in Normal group | 32 | 12 | 10 |
Samples in STAD group | 375 | 96 | 130 |
STAD, stomach adenocarcinoma; TCGA, The Cancer Genome Atlas.
Major histocompatibility complex-related genes (MHCRGs) were collected through the GeneCards database and a total of 1,591 MHCRGs were obtained. See table available online: https://cdn.amegroups.cn/static/public/10.21037tcr-24-707-1.xlsx. We obtained 47 immune checkpoint genes (ICGs) in the published literature on the PubMed website (25), and the specific gene names are shown in table available online: https://cdn.amegroups.cn/static/public/10.21037tcr-24-707-2.xlsx. At the same time, we obtained data from the GeneCards (26) database were searched with “Human Leukocyte Antigen” as the keyword. A total of 34 HLA family genes were obtained, and the specific gene names are shown in table available online: https://cdn.amegroups.cn/static/public/10.21037tcr-24-707-3.xlsx.
In addition, we downloaded the somatic mutation (SM) data of the TCGA-STAD dataset from the TCGA official website, including single nucleotide polymorphism (SNP) and other data, and used R package maftools (27) to visualize the data. To analyze the copy number variation (CNV) of STAD patients, we used the R package TCGAbiolinks to download the “Copy Number Variation” data of the TCGA-STAD dataset. Then, the data were integrated and analyzed by GISTIC 2.0, and the default settings were used for analysis parameters. Subsequently, we downloaded the Tumor Immune Dysfunction and Exclusion (TIDE) score evaluated by the TIDE algorithm from the TIDE website.
STAD-related DEGs
We used the limma package for conducting a differential analysis on the TCGA-STAD dataset, aiming to identify DEGs between distinct groups (Normal/STAD) within the dataset.
Gene Ontology (GO) and Kyoto Encyclopedia of Genes and Genomes (KEGG) of DEGs
Functional enrichment analysis and pathway enrichment analysis of major histocompatibility complex-related differentially expressed genes (MHCRDEGs) were performed using the R package clusterProfiler. Item screening criteria of P<0.05 and false discovery rate (FDR) value (q.value) <0.05 were considered statistically significant.
Gene set enrichment analysis (GSEA) and Gene Set Variation Analysis (GSVA) enrichment analysis
The R package clusterProfiler was used to perform enrichment analysis of all genes related to the phenotype. The screening criteria for significant enrichment were P<0.05 and FDR value (q.value) <0.25.
The gene set “h.all.v7.4.symbols.gmt” was obtained from the MSigDB database and GSVA (28) was performed on the TCGA-STAD dataset to calculate the difference of functional enrichment between the Normal group and STAD patients in the dataset.
Prediction modeling and evaluation
To obtain a prognostic model of MHCRGs and screen out MHCRGs with prognostic significance, we used univariate Cox regression analysis to screen MHCRGs in the TCGA-STAD dataset by combining overall survival (OS) and overall survival time (OS.time). Then we included these genes with P<0.05 in the multivariate Cox regression analysis and constructed the model to obtain the RiskScore of the model.
Based on the results of univariate Cox regression analysis, a nomogram containing five key genes was created using the rms package of R to predict the survival of STAD patients at 1-, 3- and 5-year. Evaluation of 1-, 3- and 5-year survival outcomes of STAD patients in the TCGA-STAD dataset using the R package ggDCA to construct a decision curve analysis (DCA) assessment nomogram model.
CNV, SNP analysis
To analyze the CNVs of STAD samples, the “Copy Number Segment” data of STAD samples were downloaded using the R package TCGAbiolinks. Then, we performed a GISTIC 2.0 analysis on the downloaded and processed CNV segments.
Through TCGA, the TCGA website selected “Masked Somatic Mutation” data as the SM data of STAD samples, and preprocessed the data using VarScan software. Finally, the R package maftools (27) was used to visualize the SM situation.
Immune infiltration analysis
The CIBERSORT (29) algorithm combined with the LM22 feature gene matrix was used to calculate the immune cell infiltration matrix based on the TCGA-STAD dataset. Subsequently, employing the TCGA-STAD dataset’s STAD group and the control (Normal) group, we utilized the R package ggplot2 to generate a group comparison plot for CIBERSORT immune infiltration analysis. This plot effectively illustrates the contrasting expression outcomes of immune cells within the TCGA-STAD dataset.
STAD TIDE, tumor mutational burden (TMB), immune checkpoint, HLA analysis
We calculated the group differences of TMB scores and TIDE Immune scores in the STAD group and the control (Normal) group of the TCGA-STAD dataset by Mann-Whitney U test (WilCoxon Rank Sum test).
We analyzed the expression differences of 47 ICGs between the STAD group and the control (Normal) group in the TCGA-STAD dataset and plotted group comparison diagrams.
Drug sensitivity analysis of key genes
Drug sensitivity analysis of key genes based on their expression levels and drug data in Genomics of Drug Sensitivity in Cancer (GDSC) (30), Cancer Cell Line Encyclopedia (CCLE) (31), and CellMiner (32) databases was performed and the results were presented.
Construction and evaluation of clinical prediction models based on MHCs
The single-sample gene-set enrichment analysis (ssGSEA) algorithm assesses the relative abundance of each gene within dataset samples. Using the R package GSVA, we computed major histocompatibility complex score (MHCs) for each sample in the STAD subgroup of the TCGA-STAD dataset, based on its expression matrix. Subsequently, we stratified the STAD samples into high and low MHCs groups (MHCs_High, MHCs_Low) using the median MHCs.
To demonstrate that MHCs combined with clinicopathological features could be used to assess the prognosis of STAD patients, we constructed a clinical prediction column-line graph based on TCGA-STAD expression profiling data using multifactorial Cox regression with selected MHCs combined with Stage clinicopathological features included in the model using R’s rms package. Calibration curves were generated to assess the performance of the column-line plots by comparing the predicted values of the column-line plots with the observed actual survival rates.
Quantitative validation of key genes using reverse transcription-polymerase chain reaction
The expression of MKI67, MYB, SERPINE1, TRIM31, and HAVCR1 in the MGC-803 GC cell line and GSE-1 gastric mucosal epithelial cell line was detected by quantitative reverse transcription-polymerase chain reaction (qRT-PCR). The MGC-803 cell line was obtained from Procell Company (Wuhuan, China) and the GSE-1 cell line was obtained from Cellverse Bioscience Technology Company (Shanghai, China). Quantitative fluorescence analysis was performed using an ExicyclerTM 96 fluorescence quantifier manufactured by BIONEER, Korea. Relative gene expression levels were analyzed using the 2−ΔΔCT method and β-actin was used as an internal reference gene.
Statistical analysis
All data processing and analysis in this article relied on R software (Version 4.1.2). To assess the statistical significance of normally distributed variables in the comparison of two groups of continuous variables, an independent Student t-test was employed. For variables that did not follow a normal distribution, differences were analyzed using the Mann-Whitney U test (Wilcoxon rank sum test). The Kruskal-Wallis test was used for the comparison of three or more groups. The chi-square test or Fisher’s exact test was used to compare and analyze statistical significance between the two groups of categorical variables. The R survival package was employed for conducting survival analysis. Kaplan-Meier (KM) survival curves were utilized to illustrate survival disparities, and the log-rank test was employed to evaluate the significance of differences in survival time between the two groups. All statistical P values are two-sided unless otherwise specified, and significance was attributed to P values less than 0.05.
Results
Technology roadmap
The technology roadmap for this study is shown in Figure 1.
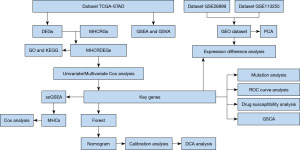
Data collection and correction
Firstly, we used the R package sva package to remove the batch effect on the two STAD datasets GSE26899 and GSE113255 to obtain the GEO dataset. Then the limma package was used to standardize the GEO dataset (Figure 2A,2B). The results showed that after removing the batch, the batch effect of samples from different sources in the GEO dataset was eliminated. The GEO dataset included 226 cases of STAD and 22 cases of Normal control samples.
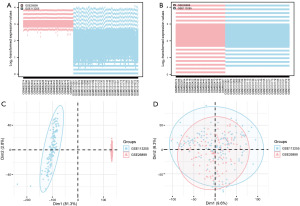
Following the removal of batch effects based on the sample source, we conducted principal component analysis (PCA) on the expression matrix of the GEO dataset both before and after the batch effect removal to assess its impact (Figure 2C,2D). The findings indicated that the elimination of batch effects resulted in a substantial reduction of the batch effect associated with samples from different sources.
Analysis of DEGs associated with STAD
We have analyzed and obtained the results of the differential genes in the STAD dataset, and the results are as follows: the number of genes in TCGA-STAD dataset that satisfy the thresholds of |logFC| >2 and P<0.05 threshold, there are 1,440 genes, and under this threshold, the number of highly expressed (low expression in normal group, logFC >2, up-regulated genes) in the STAD group is 1,102, and the number of low-expressed (high expression in normal group, logFC <0, down-regulated genes) in the STAD group is 338, and we plotted a volcano based on the results obtained from the differential analysis of this dataset map (Figure 3A).
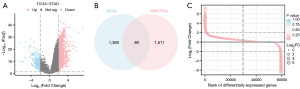
To obtain MHCRDEGs, we took the intersection of all DEGs and MHCRGs obtained from TCGA-STAD datasets. DEGs and MHCRGs were taken to intersect, and a total of 80 MHCRDEGs were obtained and plotted in a Venn diagram (Figure 3B).
We analyzed the differences in the expression of MHCRDEGs among different subgroups (STAD/Normal) in the TCGA-STAD dataset and plotted the differences in ordering diagrams (Figure 3C) to show the results of the analysis.
Functional enrichment analysis (GO) and pathway enrichment (KEGG) analysis of MHCRGS
To analyze the biological process, molecular function, cell component, biological pathway, and relationship with STAD of 80 MHCRDEGs, we first performed GO (Table 2) and KEGG (Table 3) enrichment analysis for MHCRDEGs. The criteria for screening enriched entries involved considering P<0.05 and a FDR value (q-value) <0.05 as statistically significant. The outcomes of both GO functional enrichment analysis and KEGG enrichment analysis were visually represented through a bubble diagram (Figure 4A,4B), a ring network diagram (Figure 4C,4D), and a bar diagram (Figure 4E,4F).
Table 2
Ontology | ID | Description | Gene ratio | Background ratio | P | P.adjust |
---|---|---|---|---|---|---|
BP | GO:0032496 | Response to lipopolysaccharide | 14/76 | 333/18,800 | <0.001 | 1.38e−07 |
BP | GO:0002237 | Response to molecule of bacterial origin | 14/76 | 354/18,800 | <0.001 | 1.55e−07 |
BP | GO:0071222 | Cellular response to lipopolysaccharide | 9/76 | 217/18,800 | <0.001 | <0.001 |
BP | GO:0071219 | Cellular response to molecule of bacterial origin | 9/76 | 229/18,800 | <0.001 | <0.001 |
BP | GO:0071216 | Cellular response to biotic stimulus | 9/76 | 256/18,800 | <0.001 | <0.001 |
CC | GO:0009897 | External side of plasma membrane | 9/79 | 455/19,594 | <0.001 | 0.01 |
CC | GO:0071682 | Endocytic vesicle lumen | 3/79 | 23/19,594 | <0.001 | 0.01 |
CC | GO:0062023 | Collagen-containing extracellular matrix | 8/79 | 429/19,594 | <0.001 | 0.02 |
CC | GO:0098636 | Protein complex involved in cell adhesion | 3/79 | 43/19,594 | <0.001 | 0.03 |
CC | GO:0034362 | Low-density lipoprotein particle | 2/79 | 12/19,594 | 0.001 | 0.03 |
MF | GO:0005125 | Cytokine activity | 11/76 | 235/18,410 | <0.001 | 6.54e−07 |
MF | GO:0008201 | Heparin binding | 9/76 | 168/18,410 | <0.001 | 3.05e−06 |
MF | GO:0048018 | Receptor ligand activity | 13/76 | 489/18,410 | <0.001 | 5.58e−06 |
MF | GO:0030546 | Signaling receptor activator activity | 13/76 | 496/18,410 | <0.001 | 5.58e−06 |
MF | GO:0005539 | Glycosaminoglycan binding | 9/76 | 234/18,410 | <0.001 | 2.06e−05 |
GO, Gene Ontology; MHCRDEGs, major histocompatibility complex-related differentially expressed genes; BP, biological process; CC, cellular component; MF, molecular function.
Table 3
Ontology | ID | Description | Gene ratio | Background ratio | P | P.adjust |
---|---|---|---|---|---|---|
KEGG | hsa05323 | Rheumatoid arthritis | 7/55 | 93/8,164 | <0.001 | <0.001 |
KEGG | hsa04657 | IL-17 signaling pathway | 7/55 | 94/8,164 | <0.001 | <0.001 |
KEGG | hsa04060 | Cytokine-cytokine receptor interaction | 11/55 | 295/8,164 | <0.001 | <0.001 |
KEGG | hsa05322 | Systemic lupus erythematosus | 6/55 | 136/8,164 | <0.001 | 0.009 |
KEGG | hsa04061 | Viral protein interaction with cytokine and cytokine receptor | 5/55 | 100/8,164 | <0.001 | 0.01 |
KEGG, Kyoto Encyclopedia of Genes and Genomes; MHCRDEGs, major histocompatibility complex-related differentially expressed genes; BgRatio, Background Ratio; GeneRatio, Gene Ratio.
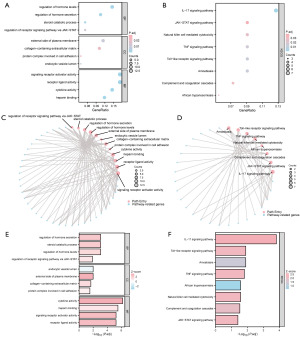
The results showed that 80 MHCRDEGs were mainly enriched in the regulation of receptor signaling pathway via JAK-STAT, steroid catabolic process, regulation of hormone secretion, regulation of hormone levels, and another biological process.
The results of KEGG enrichment analysis were mainly in Toll-like receptor signaling pathway, TNF signaling pathway, and amoebiasis. The results of KEGG enrichment analysis were mainly in Toll-like receptor signaling pathway, TNF signaling pathway, amoebiasis, natural killer cell-mediated cytotoxicity, African trypanosomiasis, complement and coagulation cascades, JAK-STAT signaling pathway, IL-17 signaling pathway, and other pathways.
GSEA and GSVA enrichment analysis of the STAD dataset
To determine the impact of gene expression levels on STAD, GSEA was performed. The results showed that the GSEA enrichment analysis of the dataset TCGA-STAD was dominated by five main biological characteristics (Figure 5A). The results showed that all the genes in TCGA-STAD were significantly enriched in the IL-12 pathway (Figure 5B), Wnt pathway (Figure 5C), TP53 pathway (Figure 5D), MAPK pathway (Figure 5E), and the expression levels of genes in the STAD group were significantly higher than those in the control group (P values are shown in Table 4). The results of enrichment analysis of TGFbeta pathway and other pathways were shown in Figure 5F and Table 4.
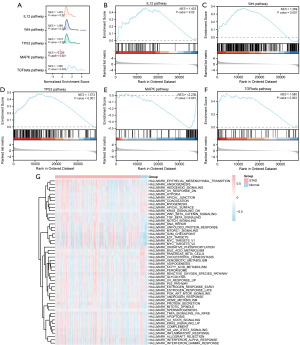
Table 4
ID | SetSize | EnrichmentScore | NES | P value | P.adjust | Q value |
---|---|---|---|---|---|---|
WP_TGFBETA_RECEPTOR_SIGNALING | 55 | 0.525934209 | 1.579612072 | 0.003 | 0.021659335 | 0.016098463 |
REACTOME_FCERI_MEDIATED_MAPK_ACTIVATION | 87 | −0.494121388 | −2.236060575 | <0.001 | 2.21635E−06 | 1.64732E−06 |
REACTOME_REGULATION_OF_TP53_ACTIVITY | 160 | 0.482214464 | 1.57331104 | <0.001 | 0.000429137 | 0.00031896 |
REACTOME_SIGNALING_BY_WNT | 330 | 0.378780691 | 1.269252069 | 0.007 | 0.040081452 | 0.02979084 |
PID_IL12_2PATHWAY | 62 | 0.468561699 | 1.423090481 | 0.02 | 0.077837181 | 0.057853068 |
REACTOME_SCAVENGING_OF_HEME_FROM_PLASMA | 69 | −0.7462940 | −3.217517 | <0.001 | 8.08e−09 | 6.01e−09 |
REACTOME_CD22_MEDIATED_BCR_REGULATION | 61 | −0.7226036 | −3.079387 | <0.001 | 8.08e−09 | 6.01e−09 |
KEGG_METABOLISM_OF_XENOBIOTICS_BY_CYTOCHROME_P450 | 69 | −0.6320480 | −2.724965 | <0.001 | 8.08e−09 | 6.01e−09 |
KEGG_DRUG_METABOLISM_CYTOCHROME_P450 | 71 | −0.6038146 | −2.610274 | <0.001 | 8.08e−09 | 6.01e−09 |
REACTOME_ROLE_OF_LAT2_NTAL_LAB_ON_CALCIUM_MOBILIZATION | 71 | −0.5976158 | −2.583477 | <0.001 | 8.08e−09 | 6.01e−09 |
PID_PLK1_PATHWAY | 46 | 0.7666399 | 2.237805 | <0.001 | 8.08e−09 | 6.01e−09 |
WP_RETINOBLASTOMA_GENE_IN_CANCER | 90 | 0.7098569 | 2.226083 | <0.001 | 8.08e−09 | 6.01e−09 |
WP_DNA_REPLICATION | 42 | 0.7690904 | 2.213133 | <0.001 | 8.08e−09 | 6.01e−09 |
REACTOME_MITOTIC_SPINDLE_CHECKPOINT | 111 | 0.6724397 | 2.149378 | <0.001 | 8.08e−09 | 6.01e−09 |
REACTOME_RESOLUTION_OF_SISTER_CHROMATID_COHESION | 126 | 0.6537886 | 2.100586 | <0.001 | 8.08e−09 | 6.01e−09 |
GSEA, Gene Set Enrichment Analysis; TCGA, The Cancer Genome Atlas; STAD, stomach adenocarcinoma; NES, Normalized Enrichment Score.
To explore the difference between the TCGA-STAD dataset in STAD samples (group: STAD) and the corresponding Normal samples (group: Normal), we performed GSVA on the TCGA-STAD dataset (Figure 5G). The results showed that the Hedgehog pathway, PI3K/AKT pathway, and other gene sets showed differences between STAD samples and corresponding normal samples in the TCGA-STAD dataset (Table 5).
Table 5
Pathway | logFC | AveExpr | t | P | adj.P.Val |
---|---|---|---|---|---|
HALLMARK_E2F_TARGETS | 0.666802328 | −0.006395071 | 7.893191541 | <0.001 | 5.75E−13 |
HALLMARK_G2M_CHECKPOINT | 0.605973947 | −0.013187441 | 7.791756326 | <0.001 | 7.79E−13 |
HALLMARK_MYC_TARGETS_V2 | 0.639056248 | −0.020777814 | 7.450440732 | <0.001 | 6.07E−12 |
HALLMARK_MYC_TARGETS_V1 | 0.516101743 | −0.025592043 | 6.239768317 | <0.001 | 7.28E−09 |
HALLMARK_MITOTIC_SPINDLE | 0.358702771 | −0.043958178 | 5.644492583 | <0.001 | 1.84E−07 |
HALLMARK_UNFOLDED_PROTEIN_RESPONSE | 0.336477924 | −0.024973598 | 4.790868529 | <0.001 | 1.13E−05 |
HALLMARK_DNA_REPAIR | 0.295298442 | −0.037306524 | 4.337264441 | <0.001 | 8.11E−05 |
HALLMARK_MTORC1_SIGNALING | 0.301767786 | −0.025387216 | 4.228558902 | <0.001 | 0.000109779 |
HALLMARK_NOTCH_SIGNALING | 0.237162271 | −0.053165046 | 3.650612952 | <0.001 | 0.000860513 |
HALLMARK_ANGIOGENESIS | 0.266343133 | −0.043240286 | 3.600000013 | <0.001 | 0.000931244 |
HALLMARK_PROTEIN_SECRETION | 0.240593031 | −0.042305908 | 3.570838782 | <0.001 | 0.000986166 |
HALLMARK_TGF_BETA_SIGNALING | 0.172916132 | −0.049946496 | 2.634959306 | <0.001 | 0.017414631 |
HALLMARK_WNT_BETA_CATENIN_SIGNALING | 0.161001688 | −0.054055378 | 2.422299172 | 0.02 | 0.03042244 |
GSVA, Gene Set Variation Analysis; TCGA, The Cancer Genome Atlas; STAD, stomach adenocarcinoma; FC, fold change; AveExpr, average expression; adj.P.Val, adjusted P value.
Prediction modeling and evaluation
We used univariate Cox regression analysis to screen MHCRDEGs in the TCGA-STAD dataset combined with OS and OS.time. Clinical information on the relevant samples is given in table available online: https://cdn.amegroups.cn/static/public/10.21037tcr-24-707-4.xlsx. We select five key genes: MKI67 (P=0.04), MYB (P=0.01), SERPINE1 (P=0.001), TRIM31 (P=0.04), HAVCR1 (P=0.005) for our subsequent study and the forest diagram (Figure 6A) to display the results. Then we included these key genes in the multivariate Cox regression analysis and constructed a model to obtain the risk score RiskScore of the model. The samples of the TCGA-STAD dataset were divided into high and low-risk groups with the median RiskScore (low-risk group: low; high-risk group: high) (Table 6).
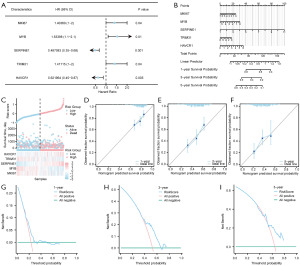
Table 6
Characteristics | Multivariate analysis | P value | ||
---|---|---|---|---|
HR | Low CI | High CI | ||
MKI67 | 0.931263856708635 | 0.755608564019992 | 1.14775349580195 | 0.51 |
MYB | 0.891988845718735 | 0.734639134929742 | 1.08304072442687 | 0.24 |
SERPINE1 | 1.22624250589404 | 1.09176728802698 | 1.37728131237444 | <0.001 |
TRIM31 | 0.95552081718245 | 0.852496781353976 | 1.07099528354689 | 0.43 |
HAVCR1 | 1.13005465080264 | 0.973818570476436 | 1.31135670700538 | 0.11 |
TCGA, The Cancer Genome Atlas; STAD, stomach adenocarcinoma; HR, hazard ratio; CI, confidence interval.
We then performed a nomogram analysis to determine the prognostic power of the Cox regression model and developed the nomogram (Figure 6B). The results showed that the expression level of Model Genes SERPINE1 was significantly higher than other variables, while MYB was significantly lower than other variables for the STAD diagnostic model.
The grouping of risk factors from the multivariate Cox prognostic model was visualized in a risk factor plot (Figure 6C), which showed that there were five key genes in the multivariate Cox prognostic model we constructed.
In addition, we performed a Calibration analysis of the nomogram of the multivariate Cox prognostic model at 1 year (Figure 6D), 3 years (Figure 6E), and 5 years (Figure 6F) and drew a calibration curve (Figure 6D-6F). We found that the survival predicted by the model was consistent with the actual survival of patients.
We then used DCA to evaluate and present the clinical utility of the constructed Cox regression prognostic model at 1-year (Figure 6G), 3-year (Figure 6H), and 5-year (Figure 6I). The results showed that the utility of the clinical prediction model: was 5-year > 3-year > 1-year.
At the same time, we combined the OS and OS.time corresponding to samples in the RiskScore and TCGA-STAD datasets to draw the survival KM curve (Figure 7A). The results showed that the risk scores of the Cox regression model were highly statistically significant in the prediction of patient OS in the TCGA-STAD dataset (P<0.001), and the prognosis of patients in the high group was worse.

We used the Mann-Whitney U test (Wilcoxon rank sum test) to analyze the RiskScore of the Cox regression model between groups for OS in the TCGA-STAD dataset and plotted group comparisons (Figure 7B). P<0.001 was considered to indicate statistical significance.
We performed time-dependent area under curve (AUC) (1-, 3-, and 5-year) analysis of the RiskScore of the Cox regression model in the TCGA-STAD dataset and presented the results (Figure 7C). Based on the time-dependent AUC curve, the RiskScore of the Cox regression model showed low accuracy in predicting the occurrence of STAD at 1-, 3-, and 5-year (0.5< AUC <0.7).
Ultimately, we conducted time-dependent receiver operating characteristic (ROC) curve analysis (1-, 3-, and 5-year) for the RiskScore derived from the Cox regression model in the TCGA-STAD dataset, and the outcomes are illustrated in Figure 7D. According to the time-dependent ROC curve, the accuracy of the Cox regression model’s RiskScore in predicting the occurrence of STAD was relatively low at 1-, 3-, and 5-year intervals (0.5< AUC <0.7) (AUC value >0.7 is generally considered indicative of robust predictive performance, 0.7> AUC values <0.6 suggest moderate accuracy, AUC values <0.6 are typically associated with poorer predictive efficacy).
Expression analysis of key genes in TCGA-STAD dataset and GEO
A violin plot (Figure 8A) was used to show the different analysis results of the expression levels of five key genes between different groups in the STAD dataset TCGA. The results showed that the expression levels of five key genes were significantly different between different groups in the TCGA-STAD dataset (P<0.001).
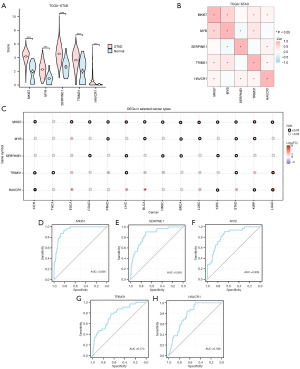
Based on the complete expression matrix of the five key genes in the TCGA-STAD dataset, correlation analysis was performed and a correlation heat map was drawn (Figure 8B). The results showed that there was a certain positive correlation between key genes MKI67 and MYB, MYB, and TRIM31. The key genes MYB and SERPINE1 were negatively correlated.
The expression patterns of five key genes were assessed later using the Gene Set Cancer Analysis (GSCA) online tool in 14 cancer types with at least three matched tumors and normal samples (Figure 8C, table available online: https://cdn.amegroups.cn/static/public/10.21037tcr-24-707-5.xlsx). The results showed that key genes such as MKI67, MYB, and SERPINE1 were upregulated in most of the tumor samples.
Then, the ROC curves of the five key genes in the TCGA-STAD dataset were drawn and the results were presented (Figure 8D-8H). The ROC curve showed that the expression difference of the key gene MKI67 in the TCGA-STAD dataset showed high accuracy (AUC >0.9) among different groups, and the key genes (MYB, SERPINE1, TRIM31, HAVCR1) in the TCGA-STAD dataset showed a certain accuracy among different groups (0.7< AUC <0.9).
The violin plot of group comparison (Figure 9A) was used to show the difference analysis results of the expression levels of five key genes among different groups (STAD/Normal) in the GEO dataset. A total of four key genes (MKI67, MYB, SERPINE1, TRIM31) were obtained after excluding key genes that did not exist in the data set. The results showed that the expression of three key genes (MKI67, MYB, SERPINE1) was significantly different between different groups in the GEO dataset (P<0.001).
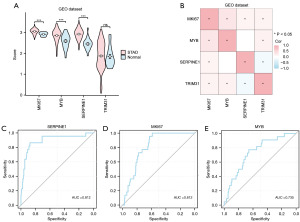
Based on the complete expression matrix of the five key genes in the TCGA-STAD dataset, the key genes that did not exist in the dataset were excluded for correlation analysis and the correlation heat map was drawn (Figure 9B). The results showed that there was a certain positive correlation between key genes MKI67 and MYB, MYB, and TRIM31. There was a negative correlation between TRIM31 and SERPINE1.
Then, the ROC curves of the four key genes between different groups (STAD/Normal) in the GEO dataset (Figure 9C-9E) were drawn, and the results with AUC values less than 0.6 were excluded for display. The results showed that these three key genes showed certain accuracy among different groups in dataset GSE29431 (0.7< AUC <0.9).
CNV, SNP analysis
First, to analyze the SMs of 1,591 MHCRGs in STAD samples of the TCGA-STAD dataset, we counted the mutation analysis results of 1,591 MHCRGs in STAD samples and visualized them through the R package map tools (Figure 10A). The results showed that there were nine major SM in MHCRGs, of which Missense mutations accounted for the majority.
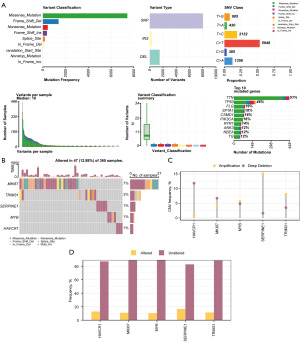
In addition, the mutation type of 1,591 MHCRGs in STAD samples was mainly SNP. In addition, C to T mutation was the most common SNP in STAD samples.
Then, we also analyzed the SM status of five key genes in STAD samples and ranked them according to mutation frequency from high to low to visualize the five key genes (Figure 10B). The results showed that the key gene MKI67 had the highest mutation rate (7%).
To analyze the CNV of the five key genes in the TCGA-STAD dataset, the CNV data of the TCGA-STAD dataset were downloaded and merged, and then analyzed by GISTIC2.0. A total of five key genes had CNV in STAD samples, and then five key genes were shown (Figure 10C,10D).
STAD TIDE, TMB, immune infiltration, immune checkpoint, HLA analysis
Given the current important role of immunotherapy in tumors, we evaluated the sensitivity of the TCGA-STAD dataset to immunotherapy by the TIDE algorithm, and then we presented the specific analysis results by group comparison plot (Figure 11A). The findings revealed a highly statistically significant difference in the TIDE immunotherapy score between the STAD group and the control (Normal) group in the TCGA-STAD dataset (P<0.001), with the STAD group exhibiting a lower score compared to the control (Normal) group.
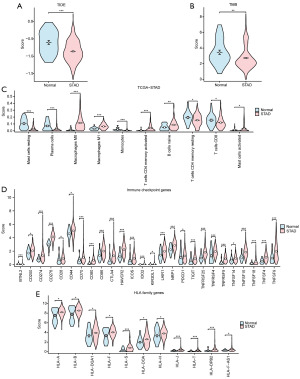
We then analyzed the difference in TMB score between the STAD group and the control (Normal) group in the TCGA-STAD dataset. The results of the TMB score group comparison plot (Figure 11B) show that the TMB score of the TCGA-STAD dataset was highly statistically significant between the STAD group and the control (Normal) group (P=0.002), and the STAD group was lower than the control (Normal) group.
Subsequently, the CIBERSORT algorithm was employed to compute the abundance and correlation of 22 immune cells in STAD samples obtained from the TCGA-STAD dataset. We filtered immune cells based on a P<0.05 and illustrated the variation in immune cell infiltration abundance among different groups using a group comparison plot. The group comparison plot (Figure 11C) showed that 10 immune cells were statistically significant (P values are shown in table available online: https://cdn.amegroups.cn/static/public/10.21037tcr-24-707-6.xlsx).
Subsequently, we also obtained the ICGs and HLA family genes from the published literature and GeneCards database. After the intersection with the genes in the TCGA-STAD dataset, we obtained the ICG and HLA family genes (HLA family genes) from the published literature and GeneCards database. A matrix containing 47 ICG and their respective expression levels was acquired, as depicted in table available online: https://cdn.amegroups.cn/static/public/10.21037tcr-24-707-2.xlsx. Additionally, a matrix encompassing 34 HLA family genes along with their corresponding expression levels was obtained, as illustrated in table available online: https://cdn.amegroups.cn/static/public/10.21037tcr-24-707-3.xlsx.
Then, we combined the grouping of the TCGA-STAD dataset and used the Mann-Whitney U test to explore the statistical difference in ICGs between the STAD group and the control (Normal) group (Figure 11D). The results showed that: ICGs BTNL2, CD200, CD274, CD276, CD28, CD44, CD70, CD80, CD86, CTLA4, HAVCR2, ICOS, IDO2, KIR3DL1, LAIR1, NRP1, PDCD1, TIGIT, TNFRSF25, TNFRSF4, TNFRSF9, TNFSF14, TNFSF15, TNFSF18, TNFSF4 and TNFSF9 were statistically significant between STAD and control (Normal) group of TCGA-STAD dataset (P values are shown in table available online: https://cdn.amegroups.cn/static/public/10.21037tcr-24-707-7.xlsx).
Finally, we used the Mann-Whitney U test to explore the statistical difference of HLA family genes between the STAD group and control the (Normal) group by combining the grouping of the STAD (TCGA-STAD) data set (Figure 11E). The results showed that HLA-A, HLA-B, HLA-DQA1, HLA-F, HLA-S, HLA-DOA, HLA-H, HLA-J, HLA-T, HLA-DPB2, HLA-F-AS1 was statistically significant between the STAD group and the control (Normal) group in the STAD (TCGA-STAD) data set (P values are shown in table available online: https://cdn.amegroups.cn/static/public/10.21037tcr-24-707-8.xlsx).
Drug sensitivity analysis
We used the cancer drug susceptibility genomics CellMiner database, GDSC database, and CCLE database including the mRNA expression profile and drug activity data of key genes, using the prophetic algorithm, according to the five key genes and TCGA gene expression profile used to construct ridge regression model to predict the sensitivity of key genes to common anticancer drugs by IC50 value. Finally, we combined key genes with the CellMiner database (Figure 12A), the correlation of small molecules in the GDSC database (Figure 12B) and CCLE database (Figure 12C) were visualized respectively.
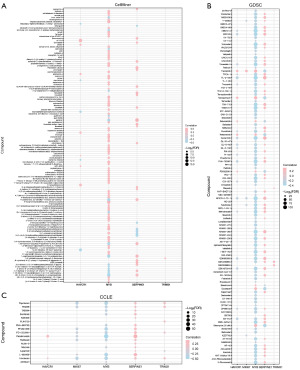
The results showed that there was a certain positive correlation between key genes MYB and most drug small molecules in the CellMiner database. key genes SERPINE1 and drug small molecule 2-(1-(4-(2-(2-hydroxyethyl) ethyl) piperazine)) naphthazarin, key genes HAVCR1 and drug small molecule 1, 2, 4, There were negative correlations among 5-tetrazine, 3,6-bis (1-azetidine), etc.
In the GDSC database, the key genes MYB and most of the drug small molecules have a certain negative correlation. The key gene SERPINE1 is positively correlated with most of the drug’s small molecules.
In the CCLE database, the key genes MYB and most drug small molecules have a certain negative correlation, and the key genes SERPINE1 and most drug small molecules have a certain positive correlation.
Construction and evaluation of clinical prediction models based on MHCs
To analyze the differences in gene expression between High/Low groups of MHCs in samples from patients with TCGA-STAD disease in the dataset, Violin plot of group comparison (Figure 13A) was used to show the difference analysis results of the expression levels of 5 key genes between High and Low groups of MHCs in the samples of patients with disease in the data set. The results showed that the expression levels of four key genes (MKI67, MYB, TRIM31, HAVCR1) in the STAD patients with High/Low MHC scores in the data set TCGA-STAD were extremely statistically significant (P<0.001). The expression of the key gene SERPINE1 was highly statistically significant between MHC score high and low (P=0.007) in STAD patient samples in the data set TCGA-STAD. The co-expression heatmap of the five key genes was also shown (Figure 13B). Clinical information for samples related to MHCs is provided in table available online: https://cdn.amegroups.cn/static/public/10.21037tcr-24-707-9.xlsx.
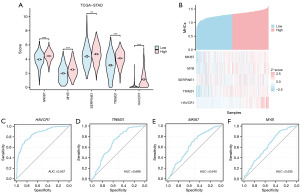
At the same time, we combined the corresponding OS and OS.time of samples in the MHCs and TCGA-STAD dataset to draw the survival KM curve (Figure S1).
Next, the ROC curves of five key genes were plotted between High/Low groups of MHCs in the samples of patients with TCGA-STAD disease in the dataset. Results with AUC values less than 0.6 were excluded from the presentation (Figure 13C-13F). The ROC curve showed that the key gene HAVCR1 showed a certain accuracy (0.7< AUC <0.9) between High and Low MHC groups in the data set TCGA-STAD disease patient samples. The key genes (MKI67, MYB, TRIM31) showed Low accuracy (0.5< AUC <0.7) between High and low MHC groups in the TCGA-STAD disease samples.
The results of Cox regression of MHCs were organized and presented in the form of a forest plot (Figure 14A). Finally, Hs was combined with stage to construct a predictive nomogram to evaluate the OS status of STAD patients (Figure 14B). The nomogram could calculate the overall score according to the patient’s MHCs and stage, and determine the probability of survival time less than 1, 3, and 5 years according to the score. The calibration curve of the nomogram was drawn. By comparing the estimated OS values of the patients at 1 year (Figure 14C), 3 years (Figure 14D), and 5 years (Figure 14E) of the calibration curve with the actual observed values of the patients, it was found that there was good consistency between the two (Figure 14C-14E), which proved that the accuracy of the model was good.
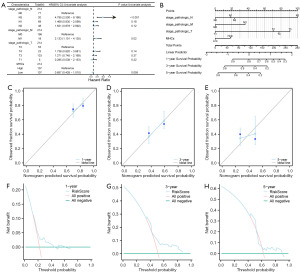
Subsequently, DCA was employed to assess and showcase the clinical utility of the developed clinical prediction model at 1-year (Figure 14F), 3-year (Figure 14G), and 5-year (Figure 14H). The findings indicated that the clinical utility of the 5-year model surpassed that of the 3-year model, while the clinical utility of the 1-year model exceeded that of the 3-year model.
Validation of MKI67, MYB, SERPINE1, TRIM31, HAVCR1 by qRT-PCR
MGC-803 and GSE-1 cell lines were selected and validated by qRT-PCR (Table 7). The results showed that the expression levels of four key genes (MKI67, MYB, SERPINE1, TRIM31) in GC cell lines and gastric mucosal epithelial cell lines were statistically significant (MKI67: P=0.01, MYB: P=0.02, SERPINE1: P=0.02, TRIM31: P=0.02) (Figure 15A-15D), and the expression levels of HAVCR1 was extremely statistically significant (P<0.0001) (Figure 15E).
Table 7
Primer | Forward (5' to 3') | Reverse (5' to 3') |
---|---|---|
MKI67 | AGATGTGCTCTGGGTTAC | TTCTTCAGGACAGGTGG |
MYB | ATACCCAACTGTTCACGC | ATGTCATCTGCTCCTCCA |
SERPINE1 | CACTCTTGTACTGCCTGCCA | TGCACACTGTTTCTGGGGAG |
TRIM31 | GTCTTCACGGACCAGGTAG | TCTTCAGGGAATCAACGAG |
HAVCR1 | AGGGAGCAATAAGGAGA | AGCAAGAAGCACCAAGA |
β-actin | GGCACCCAGCACAATGAA | TAGAAGCATTTGCGGTGG |
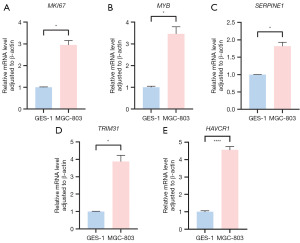
Discussion
STAD is one of the most common malignant tumors worldwide, and the prognosis of STAD is still poor despite the availability of various therapeutic options such as surgical treatment, chemotherapy, and immunotherapy (33). Therefore, it is crucial to explore effective diagnostic markers and new potential therapeutic targets.
Various prognostic biomarkers related to DNA, RNA, and exosomes have been identified in GC. Among these, tumor-associated oncogenes and tumor suppressor genes have been extensively studied and are well-established in terms of their clinical significance (7). These genes often exhibit specific expression alterations during tumor initiation and progression, which further influence the biological behavior of the tumor. Furthermore, these genes have been shown to provide valuable prognostic insights for patients. Research indicates that amplification of FGFR2 is associated with higher pT and pN stages as well as lymph node metastasis, and correlates with poorer OS. Regarding E-cadherin, patients with epigenetic and structural alterations in CDH1 have worse OS compared to those with unaltered CDH1 (34,35). Yu et al. (36) developed a prognostic prediction model for GC patients with peritoneal metastasis receiving immunotherapy. This model incorporated CDH1, ERBB3, HLA-DQB1, and naive CD4+ T cell infiltration, and achieved favorable results in external validation.
With the advent of high-throughput sequencing technologies, various non-coding RNAs, such as microRNAs (miRNAs), long non-coding RNAs (lncRNAs), and enhancer RNAs (eRNAs), have garnered increasing attention for their roles in modulating tumor-related gene expression and influencing tumor biology (37). Studies have demonstrated that high expression levels of multiple miRNAs in patients not undergoing chemotherapy are associated with shorter OS. lncRNAs also hold promise as biomarkers, with downregulation of AI364715, GACAT1, and GACAT2 identified as markers of poor prognosis (38). Han et al. (39) developed a predictive model related to tumor angiogenesis, termed ARLncs, which showed good predictive performance and a positive correlation between ARLncs risk scores and the expression of angiogenesis markers CD34 and CD105. Additionally, Gao et al. (40) constructed a prediction model based on eRNAs, suggesting that these genes are primarily involved in tumor-associated biological processes and that the model exhibits high accuracy.
In the present study, two clinical prediction models were established based on five key genes: MKI67, MYB, SERPINE1, TRIM31, and HAVCR1, also based on the key genes and their related MHCs. Among the prognostic prediction models based on key genes, the utility of the SERPINE1 diagnostic model was significantly higher than that of the other variables, whereas the utility of MYB was significantly lower than that of the other variables. In a recent study, SERPINE1 expression was found to be significantly increased in STAD samples, with the degree of expression closely correlating with immune cells in the immune microenvironment and synergizing with the immune checkpoints programmed death-1 (PD1) and programmed death-ligand 1 (PD-L1) (41). In addition, several studies have shown that high SERPINE1 expression is significantly associated with poor prognosis in STAD (42,43). The MKI67 gene is a gene that encodes the protein Ki-67, which is a cell cycle protein that is highly expressed mainly in the G1, S, G2, and M phases of the cell cycle (44). Ki-67 is usually associated with rapidly proliferating cancer cells and is therefore widely used to assess the malignancy and prognosis of tumors (45). A study has shown that high expression of the MKI67 gene is also associated with poor prognosis and decreased survival in STAD patients (46). These findings are consistent with our study. However, it has also been demonstrated that MYB could also participate in the miR-139-5p/MYB axis thereby regulating cancer cell proliferation and metastasis (47). Key genes showed some negative correlation between MYB and SERPINE1 in differential expression analysis in the TCGA dataset, but only studies in Saccharomyces cerevisiae have pointed out that the expression products of SERPINE1 could be inhibitors of MYB protein targets and thus related to the maintenance of ribosomes in a dormant state, which is not clear in the study of tumor mechanisms (48). Besides, it has also been pointed out that HAVCR1 could play a key role in GC by regulating the MEK/ERK signaling pathway; TRIM31 is significantly elevated in STAD tissues, which is closely associated with aggressive clinical outcomes and poor prognosis (49,50) All these results indicate that the key genes in this study have an important impact on the development of STAD, which is consistent with our analysis.
In this study, we screened 80 MHCRDEGs and further performed GO and KEGG enrichment analyses and GSEA and GSVA enrichment analysis on the differential genes to explore their overall changes in biological functions, signaling pathways, and gene sets. Subsequently, we further identified five prognostically significant MHCRDEGs: MKI67, MYB, SERPINE1, TRIM31, and HAVCR1, by Cox regression analysis, which were used to construct a prognostic prediction model and derive a risk score for the model, which in turn grouped the samples into high- low-risk groups. We found that the model prediction of patient survival was largely consistent with the actual patient survival, confirming the predictive effect of the model. Finally, we calculated the MHCs for each sample, incorporated the MHCs into a multifactorial Cox analysis in conjunction with the Stage clinicopathologic features, and constructed nomograms to predict patient survival, which showed good accuracy and the best clinical utility of the model at a 5-year prognosis.
In the GO and KEGG enrichment analyses in this study, the results suggested that MHCRDEGs were associated with a variety of biological processes or signaling pathways, including hormone secretion levels and the JAK-STAT signaling pathway. Hormones play an important role in the occurrence and development of STAD, and the pathogenesis of STAD is related to the metabolism of hormones such as gastrin and glucagon (51,52). There are complex interactions between hormones and the immune system, and MHC genes might be indirectly involved in processes associated with STAD by influencing the immune response. For example, T cell activity is regulated by MHC molecules, and T cells play a key role in the anti-cancer immune response. The JAK-STAT signaling pathway is an important signaling pathway that transmits information within cells and is involved in a wide range of biological processes, including cell proliferation, differentiation, immune regulation, and inflammatory responses (53). Over-activation of the JAK-STAT signaling pathway might lead to alterations in aberrant cell proliferation and anti-apoptotic mechanisms, thereby promoting tumor formation and progression (54). A recent study classified stage IV STAD into three subtypes based on immune characteristics, in which the JAK-STAT signaling pathway was enriched in the immune high-expression subtype and showed better prognostic outcomes (55). In GSEA, all the genes in TCGA-STAD were significantly enriched in the IL-12 pathway, Wnt pathway, and so on. Among them, the Wnt signaling pathway is a signaling pathway that plays a key role in many cancers, including STAD, and is associated with several biological processes, such as cell proliferation, cell differentiation, and apoptosis, whereas IL-12 affects the immune environment of STAD mainly by regulating the activity of immune cells and promoting the Th1 immune response (56). However, the current study did not find that the two act together with MHC genes in the development and progression of STAD, which needs to be further explored.
Next, we assessed the abundance of immune infiltration in the samples and its correlation by immune infiltration analysis, which screened for immune cells with significantly different expression in different subgroups, including macrophages, and CD 4T cells. It has been shown that macrophages could participate in the formation of a microenvironment that promotes tumor growth and also produces pro-inflammatory cytokines, which in turn stimulate tumor growth, angiogenesis, and distant metastasis and inhibit the anti-tumor immune response (57). In the face of tumor tissues, CD4 T cells secrete a variety of cytokines that activate and regulate other immune cells, which enhance the overall anti-tumor immune response to strengthen the attack on cancer cells (58). Studies also showed that the TIDE score STAD group was significantly lower than the control group (P<0.001), a result that may indicate a tendency towards immune escape or immune rejection in STAD, which may be related to its immune microenvironment, including overexpression of immune-suppressing factors, and insufficient T-cell infiltration (59,60). In the ICC analysis, CD274 and TNFSF9 were key genes regulating the immune response, and their expression in the TCGA group and the control group were significantly differently expressed, this difference in expression may be a marker of immune escape in STAD, enabling the tumor to evade the monitoring and attack of the immune system, suggesting that it may play an important role in STAD development and may serve as a potential therapeutic target (61).
In the context of model evaluation, we employed Cox regression analysis and time-dependent ROC curve analysis to assess the accuracy of a clinical prediction model based on MHC scoring. Specifically, we first used Cox regression analysis to evaluate the primary factors influencing survival outcomes in STAD patients, include hazard ratios (HRs), 95% confidence intervals (CIs), and corresponding P values for various clinical features. By generating nomogram graph, we combined MHC score and clinical staging information for predicting patients’ 1-, 3-, and 5-year survival probabilities. Finally, we further used DCA to assess the clinical utility of the model, comparing the net benefit at different prediction probabilities. Notably, of the two models, by calibration analysis with DCA, we found that the best clinical utility was found for the prognostic model in which both predicted patient survival to be essentially the same as the actual patient survival and both at 5 years. This might be because both models might have used a consistent training dataset five genes play a key role in the pathogenesis of the disease, and the expression levels of these genes have a higher correlation with survival prediction than even the second model constructed based on different methods might still yield similar survival prediction results under the combined assessment of MHCs and clinical staging. However, we cannot ignore the differences between the two models; although the MHCs were constructed based on five key genes, the MHCs might provide a more comprehensive assessment of gene expression and involve multiple gene interactions, thus better reflecting the status of the patient’s immune system. Moreover, information on clinical staging was introduced in the second model, which could provide the model with key information on disease severity and help predict patient survival more accurately.
However, there are some limitations in this study. Firstly, despite the use of a substantial sample size, our study samples were primarily sourced from specific databases, potentially introducing selection bias and limiting the generalizability of our findings. Secondly, while experimental validation was conducted, the selected key genes may not comprehensively cover the complex biological characteristics of gastric adenocarcinoma, potentially overlooking important factors. In bioinformatics analysis, although multiple statistical methods were employed to ensure the reliability of results, our analyses relied on existing datasets, which may restrict information availability and introduce potential biases. Furthermore, while our prognostic model demonstrated predictive capability upon evaluation, its reproducibility and clinical applicability require validation in larger independent cohorts. Lastly, treatment response and prognosis in gastric adenocarcinoma are influenced by numerous factors including individual patient variations, the tumor microenvironment, and other clinical features. Therefore, a single prognostic model may not fully capture the diversity and complexity of patients, underscoring the need for future efforts in developing more comprehensive predictive models.
Conclusions
In conclusion, we analyzed the expression and distribution of MHCRDEGs in STAD by various methods and then constructed a prognostic prediction model based on the five most critical key genes. The MHCs were then combined with clinical analysis to further predict the prognostic survival of STAD patients. This study highlights the diagnostic and prognostic role of MHCRDEGs in STAD and identifies potential diagnostic and prognostic markers for STAD.
Acknowledgments
None.
Footnote
Reporting Checklist: The authors have completed the TRIPOD reporting checklist. Available at https://tcr.amegroups.com/article/view/10.21037/tcr-24-707/rc
Peer Review File: Available at https://tcr.amegroups.com/article/view/10.21037/tcr-24-707/prf
Funding: This research was funded by
Conflicts of Interest: All authors have completed the ICMJE uniform disclosure form (available at https://tcr.amegroups.com/article/view/10.21037/tcr-24-707/coif). The authors have no conflicts of interest to declare.
Ethical Statement: The authors are accountable for all aspects of the work in ensuring that questions related to the accuracy or integrity of any part of the work are appropriately investigated and resolved. The study was conducted in accordance with the Declaration of Helsinki (as revised in 2013).
Open Access Statement: This is an Open Access article distributed in accordance with the Creative Commons Attribution-NonCommercial-NoDerivs 4.0 International License (CC BY-NC-ND 4.0), which permits the non-commercial replication and distribution of the article with the strict proviso that no changes or edits are made and the original work is properly cited (including links to both the formal publication through the relevant DOI and the license). See: https://creativecommons.org/licenses/by-nc-nd/4.0/.
References
- Smyth EC, Nilsson M, Grabsch HI, et al. Gastric cancer. Lancet 2020;396:635-48. [Crossref] [PubMed]
- Nevo Y, Ferri L. Current management of gastric adenocarcinoma: a narrative review. J Gastrointest Oncol 2023;14:1933-48. [Crossref] [PubMed]
- Bray F, Ferlay J, Soerjomataram I, et al. Global cancer statistics 2018: GLOBOCAN estimates of incidence and mortality worldwide for 36 cancers in 185 countries. CA Cancer J Clin 2018;68:394-424. [Crossref] [PubMed]
- Calderillo-Ruíz G, Díaz-Romero MC, Carbajal-López B, et al. Latin American young patients with gastric adenocarcinoma: worst prognosis and outcomes. J Gastrointest Oncol 2023;14:2018-27. [Crossref] [PubMed]
- Lordick F, Carneiro F, Cascinu S, et al. Gastric cancer: ESMO Clinical Practice Guideline for diagnosis, treatment and follow-up. Ann Oncol 2022;33:1005-20. [Crossref] [PubMed]
- Hu HM, Tsai HJ, Ku HY, et al. Survival outcomes of management in metastatic gastric adenocarcinoma patients. Sci Rep 2021;11:23142. [Crossref] [PubMed]
- Matsuoka T, Yashiro M. Biomarkers of gastric cancer: Current topics and future perspective. World J Gastroenterol 2018;24:2818-32. [Crossref] [PubMed]
- Wu C, Hou X, Li S, et al. Identification of a potential competing endogenous RNA (ceRNA) network in gastric adenocarcinoma. J Gastrointest Oncol 2023;14:1019-36. [Crossref] [PubMed]
- Huang H, Xie L, Feng X, et al. An integrated analysis of DNA promoter methylation, microRNA regulation, and gene expression in gastric adenocarcinoma. Ann Transl Med 2021;9:1414. [Crossref] [PubMed]
- Varadé J, Magadán S, González-Fernández Á. Human immunology and immunotherapy: main achievements and challenges. Cell Mol Immunol 2021;18:805-28. [Crossref] [PubMed]
- Krijgsman D, Roelands J, Hendrickx W, et al. HLA-G: A New Immune Checkpoint in Cancer? Int J Mol Sci 2020;21:4528. [Crossref] [PubMed]
- Barker DJ, Maccari G, Georgiou X, et al. The IPD-IMGT/HLA Database. Nucleic Acids Res 2023;51:D1053-60. [Crossref] [PubMed]
- Blees A, Januliene D, Hofmann T, et al. Structure of the human MHC-I peptide-loading complex. Nature 2017;551:525-8. [Crossref] [PubMed]
- Boegel S, Löwer M, Bukur T, et al. HLA and proteasome expression body map. BMC Med Genomics 2018;11:36. [Crossref] [PubMed]
- Liu Z, Derkach A, Yu KJ, et al. Patterns of Human Leukocyte Antigen Class I and Class II Associations and Cancer. Cancer Res 2021;81:1148-52. [Crossref] [PubMed]
- Liu DH, Mou FF, An M, et al. Human leukocyte antigen and tumor immunotherapy Int J Oncol 2023;62:68. (Review). [Crossref] [PubMed]
- Sadagopan A, Michelakos T, Boyiadzis G, et al. Human Leukocyte Antigen Class I Antigen-Processing Machinery Upregulation by Anticancer Therapies in the Era of Checkpoint Inhibitors: A Review. JAMA Oncol 2022;8:462-73. [Crossref] [PubMed]
- Gettinger S, Choi J, Hastings K, et al. Impaired HLA Class I Antigen Processing and Presentation as a Mechanism of Acquired Resistance to Immune Checkpoint Inhibitors in Lung Cancer. Cancer Discov 2017;7:1420-35. [Crossref] [PubMed]
- Ghasemi F, Tessier TM, Gameiro SF, et al. High MHC-II expression in Epstein-Barr virus-associated gastric cancers suggests that tumor cells serve an important role in antigen presentation. Sci Rep 2020;10:14786. [Crossref] [PubMed]
- Morinaga T, Iwatsuki M, Yamashita K, et al. Dynamic Alteration in HLA-E Expression and Soluble HLA-E via Interaction with Natural Killer Cells in Gastric Cancer. Ann Surg Oncol 2023;30:1240-52. [Crossref] [PubMed]
- Colaprico A, Silva TC, Olsen C, et al. TCGAbiolinks: an R/Bioconductor package for integrative analysis of TCGA data. Nucleic Acids Res 2016;44:e71. [Crossref] [PubMed]
- Oh SC, Sohn BH, Cheong JH, et al. Clinical and genomic landscape of gastric cancer with a mesenchymal phenotype. Nat Commun 2018;9:1777. [Crossref] [PubMed]
- Kim SK, Kim HJ, Park JL, et al. Identification of a molecular signature of prognostic subtypes in diffuse-type gastric cancer. Gastric Cancer 2020;23:473-82. [Crossref] [PubMed]
- Barrett T, Wilhite SE, Ledoux P, et al. NCBI GEO: archive for functional genomics data sets--update. Nucleic Acids Res 2013;41:D991-5. [Crossref] [PubMed]
- Xu D, Liu X, Wang Y, et al. Identification of immune subtypes and prognosis of hepatocellular carcinoma based on immune checkpoint gene expression profile. Biomed Pharmacother 2020;126:109903. [Crossref] [PubMed]
- Stelzer G, Rosen N, Plaschkes I, et al. The GeneCards Suite: From Gene Data Mining to Disease Genome Sequence Analyses. Curr Protoc Bioinformatics 2016;54:1.30.1-1.30.33.
- Mayakonda A, Lin DC, Assenov Y, et al. Maftools: efficient and comprehensive analysis of somatic variants in cancer. Genome Res 2018;28:1747-56. [Crossref] [PubMed]
- Jansen A, Dieleman GC, Smit AB, et al. Gene-set analysis shows association between FMRP targets and autism spectrum disorder. Eur J Hum Genet 2017;25:863-8. [Crossref] [PubMed]
- Newman AM, Liu CL, Green MR, et al. Robust enumeration of cell subsets from tissue expression profiles. Nat Methods 2015;12:453-7. [Crossref] [PubMed]
- Yang W, Soares J, Greninger P, et al. Genomics of Drug Sensitivity in Cancer (GDSC): a resource for therapeutic biomarker discovery in cancer cells. Nucleic Acids Res 2013;41:D955-61. [Crossref] [PubMed]
- Nusinow DP, Szpyt J, Ghandi M, et al. Quantitative Proteomics of the Cancer Cell Line Encyclopedia. Cell 2020;180:387-402.e16. [Crossref] [PubMed]
- Tlemsani C, Pongor L, Elloumi F, et al. SCLC-CellMiner: A Resource for Small Cell Lung Cancer Cell Line Genomics and Pharmacology Based on Genomic Signatures. Cell Rep 2020;33:108296. [Crossref] [PubMed]
- Sjoquist KM, Zalcberg JR. Gastric cancer: past progress and present challenges. Gastric Cancer 2015;18:205-9. [Crossref] [PubMed]
- Betts G, Valentine H, Pritchard S, et al. FGFR2, HER2 and cMet in gastric adenocarcinoma: detection, prognostic significance and assessment of downstream pathway activation. Virchows Arch 2014;464:145-56. [Crossref] [PubMed]
- Corso G, Carvalho J, Marrelli D, et al. Somatic mutations and deletions of the E-cadherin gene predict poor survival of patients with gastric cancer. J Clin Oncol 2013;31:868-75. [Crossref] [PubMed]
- Yu P, Ding G, Huang X, et al. Genomic and immune microenvironment features influencing chemoimmunotherapy response in gastric cancer with peritoneal metastasis: a retrospective cohort study. Int J Surg 2024;110:3504-17. [Crossref] [PubMed]
- Xie S, Chang Y, Jin H, et al. Non-coding RNAs in gastric cancer. Cancer Lett 2020;493:55-70. [Crossref] [PubMed]
- Huang Z, Zhu D, Wu L, et al. Six Serum-Based miRNAs as Potential Diagnostic Biomarkers for Gastric Cancer. Cancer Epidemiol Biomarkers Prev 2017;26:188-96. [Crossref] [PubMed]
- Han C, Zhang C, Wang H, et al. Angiogenesis-related lncRNAs predict the prognosis signature of stomach adenocarcinoma. BMC Cancer 2021;21:1312. [Crossref] [PubMed]
- Gao L, Rong H. Potential mechanisms and prognostic model of eRNAs-regulated genes in stomach adenocarcinoma. Sci Rep 2022;12:16545. [Crossref] [PubMed]
- Zhai Y, Liu X, Huang Z, et al. Data mining combines bioinformatics discover immunoinfiltration-related gene SERPINE1 as a biomarker for diagnosis and prognosis of stomach adenocarcinoma. Sci Rep 2023;13:1373. [Crossref] [PubMed]
- Li L, Zhu Z, Zhao Y, et al. FN1, SPARC, and SERPINE1 are highly expressed and significantly related to a poor prognosis of gastric adenocarcinoma revealed by microarray and bioinformatics. Sci Rep 2019;9:7827. [Crossref] [PubMed]
- Zhao Q, Xie J, Xie J, et al. Weighted correlation network analysis identifies FN1, COL1A1 and SERPINE1 associated with the progression and prognosis of gastric cancer. Cancer Biomark 2021;31:59-75. [Crossref] [PubMed]
- Sun X, Kaufman PD. Ki-67: more than a proliferation marker. Chromosoma 2018;127:175-86. [Crossref] [PubMed]
- Xiong DD, Zeng CM, Jiang L, et al. Ki-67/MKI67 as a Predictive Biomarker for Clinical Outcome in Gastric Cancer Patients: an Updated Meta-analysis and Systematic Review involving 53 Studies and 7078 Patients. J Cancer 2019;10:5339-54. [Crossref] [PubMed]
- Wen S, Zhou W, Li CM, et al. Ki-67 as a prognostic marker in early-stage non-small cell lung cancer in Asian patients: a meta-analysis of published studies involving 32 studies. BMC Cancer 2015;15:520. [Crossref] [PubMed]
- Xie Y, Rong L, He M, et al. LncRNA SNHG3 promotes gastric cancer cell proliferation and metastasis by regulating the miR-139-5p/MYB axis. Aging (Albany NY) 2021;13:25138-52. [Crossref] [PubMed]
- Wells JN, Buschauer R, Mackens-Kiani T, et al. Structure and function of yeast Lso2 and human CCDC124 bound to hibernating ribosomes. PLoS Biol 2020;18:e3000780. [Crossref] [PubMed]
- Chen Y, Zhang R. Long non-coding RNA AL139002.1 promotes gastric cancer development by sponging microRNA-490-3p to regulate Hepatitis A Virus Cellular Receptor 1 expression. Bioengineered 2021;12:1927-38. [Crossref] [PubMed]
- Feng Q, Nie F, Gan L, et al. Tripartite motif 31 drives gastric cancer cell proliferation and invasion through activating the Wnt/β-catenin pathway by regulating Axin1 protein stability. Sci Rep 2023;13:20099. [Crossref] [PubMed]
- Hofland J, Zandee WT, de Herder WW. Role of biomarker tests for diagnosis of neuroendocrine tumours. Nat Rev Endocrinol 2018;14:656-69. [Crossref] [PubMed]
- Waldum HL, Fossmark R. Types of Gastric Carcinomas. Int J Mol Sci 2018;19:4109. [Crossref] [PubMed]
- Standing D, Feess E, Kodiyalam S, et al. The Role of STATs in Ovarian Cancer: Exploring Their Potential for Therapy. Cancers (Basel) 2023;15:2485. [Crossref] [PubMed]
- Ni Y, Low JT, Silke J, et al. Digesting the Role of JAK-STAT and Cytokine Signaling in Oral and Gastric Cancers. Front Immunol 2022;13:835997. [Crossref] [PubMed]
- Zhang X, Yang F, Wang Z. Tumor microenvironment characterization in stage IV gastric cancer. Biosci Rep 2021;41:BSR20201248. [Crossref] [PubMed]
- Nusse R, Clevers H. Wnt/β-Catenin Signaling, Disease, and Emerging Therapeutic Modalities. Cell 2017;169:985-99. [Crossref] [PubMed]
- Fu LQ, Du WL, Cai MH, et al. The roles of tumor-associated macrophages in tumor angiogenesis and metastasis. Cell Immunol 2020;353:104119. [Crossref] [PubMed]
- Qu Y, Wang X, Bai S, et al. The effects of TNF-α/TNFR2 in regulatory T cells on the microenvironment and progression of gastric cancer. Int J Cancer 2022;150:1373-91. [Crossref] [PubMed]
- Zhong J, Pan R, Gao M, et al. Identification and validation of a T cell marker gene-based signature to predict prognosis and immunotherapy response in gastric cancer. Sci Rep 2023;13:21357. [Crossref] [PubMed]
- Jiang Q, Sun J, Chen H, et al. Establishment of an Immune Cell Infiltration Score to Help Predict the Prognosis and Chemotherapy Responsiveness of Gastric Cancer Patients. Front Oncol 2021;11:650673. [Crossref] [PubMed]
- Shen K, Liu T. Comprehensive Analysis of the Prognostic Value and Immune Function of Immune Checkpoints in Stomach Adenocarcinoma. Int J Gen Med 2021;14:5807-24. [Crossref] [PubMed]