Single-cell programmed cell death regulator patterns guide intercellular communication of cancer-associated fibroblasts that contribute to colorectal cancer progression
Highlight box
Key findings
• Among programmed cell death (PCD) mediated cancer-associated fibroblasts (CAFs), DDIT3+ CAFs associated with paraptosis exhibited the most pronounced influence on the prognosis of individuals with colorectal cancer (CRC).
What is known and what is new?
• CRC ranks as the third most prevalent cancer globally and the fourth highest contributor to cancer-related mortality, with increasing morbidity observed on a global scale.
• Our study reveals for the first time how PCD-mediated communication between CAFs regulates tumor growth in CRC patients and influences their prognosis.
What is the implication, and what should change now?
• This research presents a fresh viewpoint by elucidating the regulatory patterns of various PCDs in CAFs from a single-cell perspective. It also highlights the notable impact of paraptosis regulatory pattern in CAFs on the prognosis of CRC patients, thereby offering novel insights for CRC investigations. Additionally, we also found that DDIT3 could serve as a potential novel biomarker for CRC, warranting further exploration.
Introduction
Colorectal cancer (CRC) ranks as the third most prevalent cancer globally and the fourth highest contributor to cancer-related mortality, with increasing morbidity observed on a global scale (1). CRC progresses insidiously with subtle symptoms, often making early detection challenging. Consequently, the majority of patients are diagnosed at an advanced stage, complicating treatment efforts significantly (2). Despite significant breakthroughs in CRC surgery, radiotherapy, and chemotherapy, the prognosis for patients with advanced-stage disease remains disheartening (3). Thus, it is imperative to elucidate the molecular mechanisms underlying CRC and to explore more effective therapeutic strategies.
Cancer-associated fibroblasts (CAFs) are a significant component of the tumor microenvironment (TME), with pivotal involvement in various processes such as angiogenesis, tumor metastasis, immune modulation, metabolic reprogramming, and resistance to treatment. CAFs hold clinical significance and can serve as both prognostic indicators and potential therapeutic targets that influence the clinical treatment of CRC (4,5). In recent years, with the increasing research on the mechanism of action of CAFs on CRC, the important role of CAFs in CRC has become increasingly prominent. While CAFs can contribute to chemotherapy resistance in CRC (6) and promote tumor progression through certain gene upregulations (7), they are also linked to liver metastasis in CRC (8). However, despite the increasing clarity on the mechanisms of CAFs in CRC, it is recognized that distinct subpopulations of CAFs may exert varying functions. For instance, the ANGPTL2+ CAFs subpopulation is associated with liver metastasis in CRC (9), whereas the CD143+ CAFs subpopulation may suppress the development of CRC (10). Hence, investigating the functional roles of different CAFs subpopulations is imperative for advancing research on CRC.
Programmed cell death (PCD) is an important physiological process that maintains tissue homeostasis and removes damaged or abnormal cells, and plays a crucial role in the normal functioning of organisms. Up to now, 19 mechanisms of PCD have been identified, among which representative apoptosis, pyroptosis, autophagy, ferroptosis, cuproptosis, necroptosis, lysosome-dependent cell death, anoikis and paraptosis have been shown to be key processes in tumorigenesis with a growing body of evidence (11). Recent studies have demonstrated that PCD is integral to the regulation of CAFs and significantly influences their behavior within the TME. Various forms of PCD exert distinct effects on CAFs, impacting processes such as cancer proliferation, treatment resistance, and immune evasion (12). For instance, the activation of the apoptosis pathway may facilitate CAFs death by inhibiting the AKT signaling pathway, thereby enhancing the efficacy of anti-PD1 therapy (13). Pyroptosis, mediated by the NLRP3 inflammasome pathway, induces CAFs death and further promotes the growth and metastasis of breast cancer through the release of interleukin-1β (IL-1β) (14). CAFs also employ NUFIP1-dependent autophagy to secrete nucleotides, which support pancreatic tumor growth (15); conversely, targeting CAFs autophagy has the potential to augment the effects of immunotherapy (16). Furthermore, CAFs can inhibit tumor cell ferroptosis and contribute to drug resistance by modulating key molecules associated with ferroptosis (17,18). Other forms of PCD, including cuproptosis, necroptosis, anoikis, and paraptosis, similarly influence the activity and functionality of CAFs, thereby affecting tumor progression and treatment outcomes (19-22). Given the intricate relationship between PCD and CAFs, comprehensive research into the mechanisms by which PCD impacts CAFs in CRC is anticipated to yield novel insights for CRC research.
In this research, single-cell sequencing data from 65,362 cells of 23 CRC patients were employed to perform non-negative matrix factorization (NMF) clustering analysis on CAFs based on genes regulated by PCD. The analysis identified distinct subpopulations of CAFs regulated by PCD that exhibit significant interactions with tumor epithelial cells. These subpopulations are closely linked to metabolic pathways, transcriptional characteristics, and clinical outcomes. This investigation provides novel insights into the potential role of PCD in facilitating cellular crosstalk between CAFs and tumor cells, thereby impacting the progression of CRC. We present this article in accordance with the MDAR reporting checklist (available at https://tcr.amegroups.com/article/view/10.21037/tcr-24-1301/rc).
Methods
Data collection
The single-cell RNA sequencing (RNA-seq) dataset GSE132465 was downloaded from the Gene Expression Omnibus (GEO) database (http://www.ncbi.nlm.nih.gov/geo), comprising 23 tumor tissue samples and 10 adjacent normal tissue samples. Furthermore, transcriptome sequencing data and clinical details of 1,591 CRC patients were collected from The Cancer Genome Atlas (TCGA) Program (https://portal.gdc.cancer.gov/) [TCGA-colon adenocarcinoma (COAD), TCGA-rectum adenocarcinoma (READ)] and GEO databases (GSE17536, GSE29621, GSE39582, GSE72970). After literature search (23,24), a compilation of 19 pivotal regulatory genes associated with PCD was assembled, encompassing 580 apoptosis-related genes, 367 autophagy-related genes, 7 alkaliptosis-related genes, 338 anoikis-related genes, 19 cuproptosis-related genes, 15 entotic cell death-related genes, 88 ferroptosis-related genes, 34 immunogenic cell death- associated genes, 220 lysosomal cell death-associated genes, 220 genes related to lysosome-dependent cell death, 101 genes related to necroptosis, 8 genes related to netotic cell death, 24 NETosis-related genes, 5 oxeiptosis-related genes, 52 pyroptosis-related genes, 9 parthanatos-related genes, 8 methuosis-related genes, 23 entosis-related genes, 24 disulfidptosis-related genes, and 66 paraptosis-related genes (table available at https://cdn.amegroups.cn/static/public/10.21037tcr-24-1301-1.xlsx).
Screening for major PCD in CRC
In this study, the combined cohort TCGA-CRC, merged by TCGA-COAD and TCGA-READ, was utilized to detect differentially expressed genes (DEGs) between cancerous and adjacent normal tissues using the R package “limma” [|log2 fold change (FC)| >0.585, false discovery rate (FDR) <0.05]. Subsequently, the R package “VennDiagram” was employed to identify common genes between DEGs and genes associated with specific PCD. To investigate the involvement of diverse cell death mechanisms in CRC comprehensively, the random forest algorithm was applied to select the top 17 genes from various PCD gene sets that significantly impact the overall survival (OS) of CRC patients. PCD types with less than 17 common genes were deemed to have a minor role in CRC and were therefore excluded from the analysis.
Visualization of TME cell types and subtypes in CRC
We utilized the R package Seurat (version 5.0.1) to generate a Seurat object using the cell annotation data from the GSE132465 dataset. Subsequently, quality control measures were applied to the Seurat object, which included ensuring that the proportion of mitochondrial genes was below 25%, the proportion of ribosomal genes exceeded 3%, and the proportion of red blood cell genes was under 5%. Following quality control, we executed NormalizeData, FindVariableFeatures, ScaleData, and RunPCA functions to compute principal components (PCs) based on the Seurat objects. The t-distributed stochastic neighbor embedding (t-SNE) technique was then employed to reduce the dimensionality for enhanced visualization of the primary PCs. Lastly, leveraging the provided cell annotation data, we identified and visually represented the major TME cell types and their respective subtypes.
Analysis of cellular communication between subtypes/clusters of cells
CellChat (25) is an R package that specifically analyzes inter-cellular communication networks in single-cell RNA-seq data labeled with different cell populations, and contains a database of ligand-receptor interactions in humans and mice. Firstly, CellChatDB.human was used to evaluate the main signal input and output of each cell subtype/group. Then, by using the netVisual_circle function, the cell-cell communication network between each cell subtype/population is displayed, reflecting the intensity of communication from the target cell subtype/population to different cell subtypes/populations. Finally, using the netVisual_bubble function, we generated a bubble diagram to show the key ligand-receptor interactions between the target cell subtype/population and other cell subtypes/populations. Visualization of intercellular receptor-ligand interactions primarily involves a statistical analysis of the quantity of receptor-ligand pairs and the strength of communication between distinct cell populations. The thickness of the connecting lines represents the intensity of the interaction, while the color of the lines and the corresponding points indicates the source cell, with the opposite end representing the target cell.
Gene set enrichment analysis (GSEA) analysis of cancer signature gene sets
Fifty feature gene sets were obtained from the Molecular Signatures Database (MSigDB; http://software.broadinstitute.org/gsea/msigdb/) (26). The scoring methodology for these gene features was detailed in a previous publication (27). Specifically, the gene feature score for individual cells was determined by averaging the expression levels of all genes within the feature. To detect significant alterations in gene feature scores between normal and tumor samples, the “limma” package was used to compute the score variances between normal and tumor cells within each cell subtype. These differences were illustrated using a bubble plot, where the bubble size corresponded to −log10 FDR and the bubble color indicated log2 FC values.
Non‑negative matrix factorization of PCD regulators in CAFs
To delve deeper into the impact of PCD-mediated regulatory factor expression on CAFs from tumor tissue, we employed the NMF algorithm to decrease the dimensionality of the expression data pertaining to pivotal regulatory factors in CAFs from tumor tissue. Subsequently, utilizing the single-cell RNA (scRNA) expression matrix, distinct cell subtypes were discerned for these cellular entities. These analytical procedures were conducted in accordance with the methodology outlined in prior research studies (28,29).
Pseudotime trajectory analysis of PCD regulators for CAFs
To investigate the correlation between cell pseudotime trajectories and critical regulators of PCD, we utilized the “Monocle R” package to analyze single-cell RNA-seq data of CAFs in CRC as reported in a previous study (30). The selection criteria for highly variable genes were defined as having an average expression level of 0.1 or higher, and a dispersion value at least twice the fitted dispersion value. Subsequently, we utilized the “plot_pseudotime_heatmap” function to generate a heatmap illustrating the evolving expression profiles of key PCD regulators in CAFs within CRC along the proposed temporal trajectories.
Identification of malignant epithelial cells
Our research utilized the R package “inferCNV” (https://github.com/broadinstitute/inferCNV) to detect malignant epithelial cells. In summary, we assessed copy number variation (CNV) across all cell types using single-cell RNA-seq data, with T cells, B cells, myeloid cells, and mast cells chosen as reference cell populations. Following the completion of the inferCNV analysis, we identified malignant cells. Initially, we employed the k-means algorithm to partition cells into 20 clusters by integrating expression data and cell annotation information within the inferCNV object. Subsequently, these clustering outcomes were amalgamated and organized alongside cell type information. To visually represent the CNV profiles of cells within distinct clusters, we randomly selected 100 cells from each cluster and generated a detailed heat map using R package “ComplexHeatmap”. Furthermore, we validated the identification of malignant cells by computing the CNV score. This involved converting and squaring the expression data, calculating CNV scores for each cell, and correlating these scores with the clustering results.
Single-cell regulatory network inference and clustering (SCENIC) analysis of PCD-associated CAFs subpopulations
SCENIC method was employed to investigate the transcriptional regulatory network at the single-cell level. By utilizing gene locus rankings from the RcisTarget (https://www.bioconductor.org/packages/release/bioc/html/RcisTarget.html) database, the hg19-tss-centered-10 kb and hg19-500 bp-upstream rankings, transcription start sites were identified within the single-cell transcriptome data of CRC. Subsequently, a gene regulatory network was constructed to elucidate alterations in cellular states and mechanisms of transcriptional regulation.
Functional enrichment and metabolic activity analysis for PCD-associated CAFs subpopulations
To identify the specific biological pathways associated with PCD-related cell subtypes, we initially utilized the FindAllMarkers function to detect DEGs from single-cell data. We set the logfc.threshold to 0.25 and the minimum percentage (min.pct) to 0.25. Subsequently, we conducted Kyoto Encyclopedia of Genes and Genomes (KEGG) enrichment analysis for each cell subtype based on the DEGs, and generated a heat map by ggplot2 to visualize the top three significantly enriched KEGG pathways in each cell subtype. Assessment of metabolic activity was performed using the scMetabolism package (31). Notably, particular emphasis was placed on the KEGG metabolic pathway, enabling the determination of the activity score for each metabolic pathway in every CAFs subpopulation.
Survival analysis of PCD-associated subpopulation features in public datasets
Gene profiles for various PCD cell subtypes were created utilizing the Seurat R package’s FindAllmarker function. These profiles were subsequently employed to compute gene profile scores across all accessible CRC datasets through the gene set variation analysis (GSVA) function. The association between PCD cell subtypes and OS was investigated using log-rank test and Cox proportional hazard regression analyses.
Ethical statement
The study was conducted in accordance with the Declaration of Helsinki (as revised in 2013). The study was approved by the Ethics Committee of Bengbu Medical University (No. 2023243). All participants provided written informed consent before the study, and we obtained informed consent from the parents and/or legal guardians of patients under the age of 18 years.
Immunohistochemistry
The tumor tissue sections (5 µm) from the patients, who were from The First Affiliated Hospital of Bengbu Medical University, were subjected to dewaxing using xylene and a series of gradient alcohols, followed by rinsing in phosphate buffer saline (PBS) with a pH of 7.2 for 10 minutes. To restore the antigenicity, the sections were heated in a 1.0% citric acid solution at pH 6.0 for 2 minutes and then boiled for an additional 10 minutes to block endogenous peroxidase activity. Subsequently, the sections were washed with PBS, treated with goat serum for 20 minutes at room temperature, and then incubated overnight at 4 ℃ with rabbit monoclonal anti-DDIT3 (1:250, A20987, ABclonal, Wuhan, China). Finally, the sections were exposed to the corresponding secondary antibody-horseradish peroxidase (HRP) conjugate, subjected to 3,3'-diaminobenzidine (DAB) coloration, hematoxylin restaining, alcohol dehydration, sealed with neutral gum, and air-dried before examination.
Statistical analysis
All statistical analyses were conducted utilizing the R 4.3.2 software. Various statistical tests such as the log-rank test, Wilcoxon rank-sum test, analysis of variance (ANOVA), and Kruskal-Wallis test were employed to assess disparities in continuous or categorical variables across distinct cell groups. A significance level of P<0.05 was deemed statistically significant.
Results
Distribution of key regulators of PCD in CRC TME cells
We explored the distribution of PCD regulators in CRC TME cells using single-cell transcriptomic data from the GSE132465 dataset (Figure 1A), which contains TME cells from 33 samples from 23 CRC patients. We annotated major cell subpopulations including epithelial cells, mast cells, myeloid cells, stromal cells, T cells and B cells (Figure 1B). In addition, based on the expression levels of typical markers, we confirmed the accuracy of the annotation (Figure 1C). To identify the key regulatory genes for PCD in CRC, we subjected cancer tissues from the TCGA-CRC cohort to differential gene expression analysis with paracancerous tissues (Figure 1D) and took the intersection of DEGs with PCD-related genes (Figure S1A). The results showed a large disparity in the number of intersections of different kinds of PCD genes with DEGs. We used the random forest algorithm to select the top 17 genes with more significant effects on OS of CRC patients from a variety of PCD-related genes for subsequent studies (Figure S1B). If the number of intersecting genes for a PCD type was less than 17, we assumed that these PCD types did not play a major role in CRC and therefore did not include them in this study. Finally, we screened eight PCD mechanisms that play important roles in CRC, which are apoptosis, pyroptosis, ferroptosis, autophagy, lysosome-dependent cell death, necroptosis, anoikis, and paraptosis. By performing cell communication analyses, we further identified diverse and unique modes of interactions among these cell subpopulations (Figure 1E). To investigate the changes in the regulatory networks of these cellular subpopulations, we used a set of 50 marker gene sets from MsigDB and analysed the changes in the pathways between epithelial, mast, myeloid, stromal, T and B cells between adjacent normal and tumor tissues (Figure 1F). Finally, we demonstrated the expression of eight major PCD-regulated genes in different cellular subpopulations by heatmap (Figure 1G).
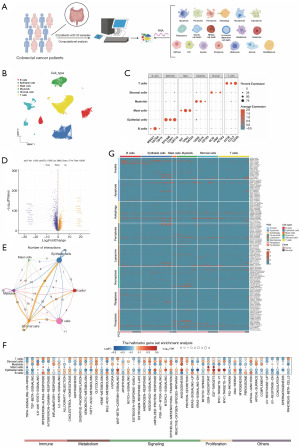
Novel apoptosis-mediated CAFs have an impact on TME in CRC
In recent years, CAFs have been recognised as key regulatory cells in TME (32). Therefore, we classified tumor tissue stromal cells into fibroblasts and endothelial cells based on classical markers of CAFs (Figure S2A). Moreover, it has been shown in the literature that CAFs are no longer only regarded as the physical support of mutant epithelial cells, but they are also important regulators and even drivers of tumor pathogenicity (33). To explore the regulatory network between CAFs and tumor epithelial cells, we classified the epithelial cells of tumor tissues into malignant and non-malignant epithelial cells by inferCNV method (Figure S2B,S2C) and did enrichment analysis of the two subpopulations (Figure S2D). Then, seven apoptosis-associated isoforms were identified among CAFs by NMF clustering, which were CLU+ CAFs-C1, P2RX4+ CAFs-C2, TRAP1+ CAFs-C3, SNAI1+ CAFs-C4, HSPA1A+ CAFs-C5, GGCT+ CAFs-C6 and non-apop-CAFs-C7 (Figure 2A). Based on pseudotime trajectory analysis (Figure 2B), we found that apoptosis-important regulators were expressed at all periods of CAFs development. For instance, nitric oxide synthase (NOS3), inhibin subunit beta B (INHBB), and gamma-glutamylcyclotransferase (GGCT) are associated with the initial development of CAFs, whereas nucleolar protein 3 (NOL3), purinergic receptor P2X 4 (P2RX4), and TNF receptor-associated protein 1 (TRAP1) are linked to the subsequent stages of CAF development (Figure 2C). We analyzed cellular communication between malignant and non-malignant epithelia in tumor tissues. Interestingly, our study revealed a higher frequency of interactions between CAFs and non-malignant epithelial cells compared to malignant epithelial cells. Additionally, we identified three subpopulations of CAFs, CLU+ CAFs-C1, P2RX4+ CAFs-C2, and SNAI1+ CAFs-C4, that may exert a significant influence based on the observed communication patterns with tumor epithelial cells (Figure 2D,2E). It is noteworthy that these subpopulations, which play important roles in cellular communication, have higher activities in many metabolic pathways (Figure 2F). In addition, KEGG enrichment analysis showed that the DEGs of these important subgroups were mainly associated with pathways such as proteoglycan and glutathione metabolism in cancer and protein processing in the endoplasmic reticulum (ER) (Figure 2G).
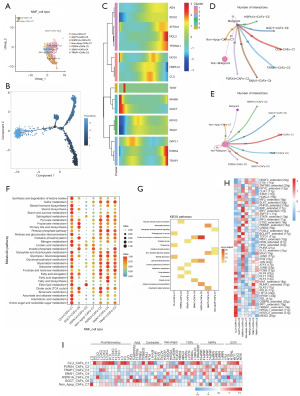
Next, gene regulatory network analysis revealed differences in transcription factors (TFs) among these major subgroups. Among them, the activities of ELK3, NFKB1, REL, HMGA1_extended, NFE2L1_extended, NFATC2_extended, and MEIS2 TFs were significantly elevated in the subpopulation of CLU+ CAFs-C1 (Figure 2H). In addition, we collected key CAFs phenotypic marker surface protein genes and compared their expression levels in apoptosis-mediated CAFs subpopulations. The results showed that most of them were highly expressed in CLU+ CAFs-C1 (Figure 2I).
Novel pyroptosis-mediated CAFs have an impact on TME in CRC
Based on NMF clustering, we identified 11 pyroptosis-associated subpopulations in CAFs, which are IL1B+ CAFs-C1, CHMP2B+ CAFs-C2, BAK1+ CAFs-C3, IL6+ CAFs-C4, CASP5+ CAFs-C5, CHMP6+ CAFs-C6, PLCG1+ CAFs-C7, CYCS+ CAFs-C8, PRKACA+ CAFs-C9, unclear-pyro-CAFs-C10 and non-pyro-CAFs-C11 (Figure 3A). After pseudotime trajectory analysis (Figure 3B), we found that the main regulators of pyroptosis were expressed at all developmental periods of CAFs (Figure 3C). CHMP6 and PLCG1 are highly expressed mainly in the early stages of CAFs development, whereas PRKACA is highly expressed mainly in the late stages of CAFs development. Analysis of cell communication indicated that the identified CAFs exhibited a higher level of communication with non-malignant epithelial cells compared to malignant epithelial cells. Notably, the number of interactions between these subpopulations and tumor epithelial cells, specifically IL1B+ CAFs-C1, CHMP2B+ CAFs-C2, BAK1+ CAFs-C3, CHMP6+ CAFs-C6, and PRKACA+ CAFs-C9, suggests that these five subpopulations may exert a significant influence (Figure 3D,3E). Interestingly, the CHMP6+ CAFs-C6 subpopulation, which communicates with the highest number of tumor epithelial cells, has higher activity in many metabolic pathways (Figure 3F). The results of KEGG enrichment analysis based on the differential genes of each cellular subpopulation showed that CHMP6+ CAFs-C6 was mainly associated with lysosomal and cytosolic actions (Figure 3G). In addition, the IL6+ CAFs-C4 subpopulation was significantly enriched in the tumor necrosis factor (TNF), interleukin-17 (IL-17) and hypoxia-inducible factor-1 (HIF-1) pathways.
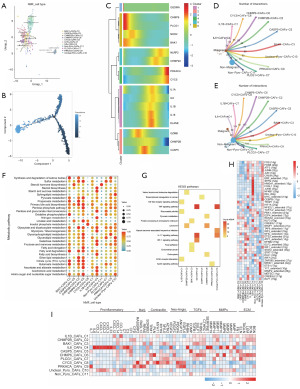
Gene regulatory network analysis revealed differences in TFs between these subpopulations (Figure 3H), with the most significant differences being in the IL6+ CAFs-C4 and PRKACA+ CAFs-C9 subpopulations. In the IL6+ CAFs-C4 subpopulation, the activities of SFPQ, HMGA1_extended, FOSL1, JUND, NFKB1, and REL were significantly elevated; NR3C1_extended, ETS1, YY1_extended, NR2F2_extended and MEF2C activities were significantly elevated in the PRKACA+ CAFs-C9 subpopulation. In addition, gene expression analysis of CAFs phenotypic marker surface proteins revealed that inflammatory response-associated proteins were significantly overexpressed in the IL6+ CAFs-C4 subpopulation (Figure 3I).
Novel ferroptosis-mediated CAFs have an impact on TME in CRC
We obtained nine subpopulations of ferroptosis-related CAFs, which were SLC1A5+ CAFs-C1, ZEB1+ CAFs-C2, CRYAB+ CAFs-C3, HMOX1+ CAFs-C4, NCOA4+ CAFs-C5, PRNP+ CAFs-C6, CD44+ CAFs-C7, unclear-ferro-CAFs-C8, non-ferro-CAFs-C9 (Figure 4A). Pseudotime trajectory analysis (Figure 4B) revealed that NCOA4, HMOX1, ACSF2, and AKR1C3 are characteristic proteins of CAFs in early development; PROM2 and CYBB are characteristic proteins of CAFs in late development. It is worth mentioning that HMOX1, although significantly highly expressed in early development, was significantly under-expressed in late development (Figure 4C). In addition to this, there were also other proteins that were significantly underexpressed in late development, such as CD44, ACACA, CRYAB, and SLC7A11. Similarly, the number of these CAFs communicating with non-malignant epithelial cells was still higher than that with malignant epithelial cells, and SLC1A5+ CAFs-C1, ZEB1+ CAFs-C2, HMOX1+ CAFs-C4, and non-ferro-CAFs-C9, the four subpopulations, may play a dominant role (Figure 4D,4E). The metabolic pathway activity of SLC1A5+ CAFs-C1 was significantly upregulated in all cellular subpopulations (Figure 4F). KEGG enrichment analysis of differential genes in each cellular subpopulation showed that the HMOX1+ CAFs-C4 subpopulation was mainly associated with ferroptosis and IL-17 signaling pathway (Figure 4G). It is worth mentioning that the activity of fatty acid biosynthesis metabolism pathway was significantly elevated in the HMOX1+ CAFs-C4 subpopulation (Figure 4F), which further verified that the HMOX1+ CAFs-C4 subpopulation might be closely related to the occurrence of ferroptosis.
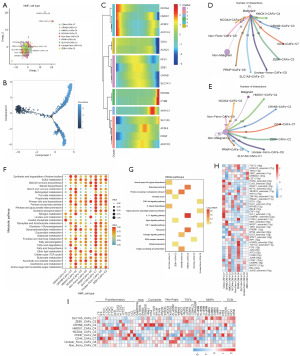
In the gene expression regulatory network analysis, we also noted that in the HMOX1+ CAFs-C4 subpopulation, the activities of ELK3_extended, ETS2_extended, and ELF2_extended, in addition to the activity of HIF-1A, were significantly elevated (Figure 4H), suggesting a possible relationship between these TFs and ferroptosis. Some inflammation-related factors such as CXCL12 and IL33 were significantly highly expressed in the HMOX1+ CAFs-C4 subpopulation (Figure 4I).
Novel autophagy-mediated CAFs have an impact on TME in CRC
Using NMF clustering, we defined six subpopulations of autophagy-associated CAFs, which are CLU+ CAFs-C1, DRAM1+ CAFs-C2, OPTN+ CAFs-C3, LZTS1+ CAFs-C4, HSPA8+ CAFs-C5, and non-auto-CAFs-C6 (Figure 5A). Through pseudotime trajectory analysis by Monocle we found (Figure 5B) that autophagy genes that play an important role in CRC are mainly expressed in CAFs during early and late development, with PINK1, LZTS1, OPTN, TMEM59, ATP6V1C2, and HSPB8 being early developmental markers; and MTM1, CLU, TRIM27, MAPK15, DAPK1, and SH3BP4 being late developmental markers (Figure 5C). Cell communication analysis revealed that these six subpopulations of autophagy-associated CAFs communicated with non-malignant epithelial cells in greater numbers (Figure 5D,5E). And, based on the number of communications between these subpopulations and tumor epithelial cells, three subpopulations, CLU+ CAFs-C1, DRAM1+ CAFs-C2 and LZTS1+ CAFs-C4, were dominant in CAFs cellular communications. In addition, metabolic pathway activity analysis revealed that the three cellular subpopulations that dominated cellular communication had higher metabolic pathway activity compared with other cellular subpopulations (Figure 5F). KEGG enrichment analysis showed that these cellular subpopulations were mainly associated with human diseases such as Ruan viral disease, Parkinson’s disease, hepatocellular carcinoma, etc. (Figure 5G).
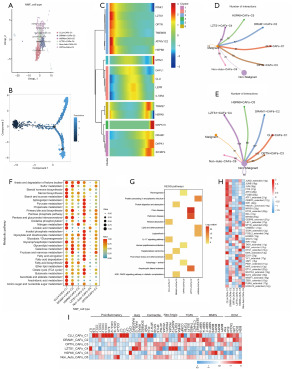
Gene expression regulatory network analysis showed that most of the TFs were highly active in CLU+ CAFs-C1 and DRAM1+ CAFs-C2 subpopulations (Figure 5H). In addition, gene expression of key CAFs phenotypic marker surface proteins showed that the CLU+ CAFs-C1 subpopulation of inflammatory factors possessed higher expression (Figure 5I).
Novel necroptosis-mediated CAFs have an impact on TME in CRC
The NMF clustering results showed that we newly defined a total of 11 subpopulations of CAFs: CHMP6+ CAFs-C1, BID+ CAFs-C2, TNFRSF10B+ CAFs-C3, BIRC3+ CAFs-C4, CHMP2B+ CAFs-C5, CYLD+ CAFs-C6, IL1B+ CAFs-C7, SMPD1+ CAFs-C8, TNFRSF1A+ CAFs-C9, IRF9+ CAFs-C10 and non-necro-CAFs-C11 (Figure 6A). By pseudotime trajectory analysis (Figure 6B), we found that necroptosis major regulators were expressed at all periods of CAFs development, and that SMPD1 and CHMP6 were characteristic genes in early development; TRAF5, TNFASF1A, and PGAM5 were characteristic genes in late development (Figure 6C). Cellular communication analysis revealed that these 11 subpopulations of necroptosis-associated CAFs communicated more with non-malignant epithelial cells compared to malignant epithelial cells (Figure 6D,6E). And, based on the number of communications between these subpopulations and tumor epithelial cells, CHMP6+ CAFs-C1, BID+ CAFs-C2, TNFRSF10B+ CAFs-C3, and CHMP2B+ CAFs-C5, were dominant in CAFs cellular communications. Metabolic pathway activity analysis revealed that the four cellular subpopulations dominating in cellular communication had higher metabolic pathway activity (Figure 6F). By KEGG enrichment analysis we found that these cell subpopulations were mainly associated with focal adhesion, platelet activation, rheumatoid arthritis, mineral absorption, p53 signaling pathway, and lysosomes (Figure 6G). Notably, the BIRC3+ CAFs-C4 subpopulation was mainly associated with inflammation-related signaling pathways such as TNF, nuclear factor kappa-B (NF-κB) and IL-17.
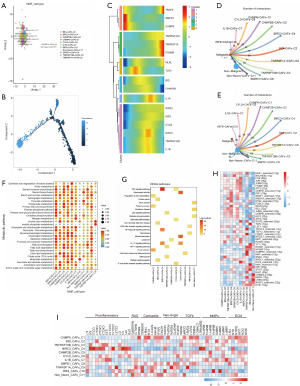
TF activities such as TCF4, TCF7L2_extended, FOXO1_extended, GABPB1_extended and MAF_extended were found to be higher in all cell subtypes by gene expression regulatory network analysis (Figure 6H). Notably, key CAFs phenotypic markers for surface protein gene expression showed that the BIRC3+ CAFs-C4 subpopulation not only exhibited enrichment of inflammatory pathways, but also relatively high expression of inflammatory factors (Figure 6I).
Novel lysosome-dependent cell death-mediated CAFs have an impact on TME in CRC
Based on NMF clustering, we obtained nine subgroups of lysosome-dependent cell death-associated CAFs, CLU+ CAFs-C1, SMPD1+ CAFs-C2, CTSH+ CAFs-C3, GNPTAB+ CAFs-C4, GLA+ CAFs-C5, IL13RA2+ CAFs-C6, HSPA8+ CAFs-C7, FES+ CAFs-C8 and non-lyso-CAFs-C9 (Figure 7A). Through Monocle (Figure 7B), we identified the specific highly expressed genes SMPD1, GNPTAB and the specific low expressed genes AP1S3, HSPA8 in the early phase of CAFs development and the specific highly expressed gene PIK3CG and the specific low expressed genes IDUA, CTSH in the late phase of CAFs development (Figure 7C). By cellular communication analysis we found that these nine subpopulations of lysosomal-dependent cell death-associated CAFs communicated with non-malignant epithelial cells in greater numbers compared to malignant epithelial cells (Figure 7D,7E). Based on the number of communications between these subpopulations and tumor epithelial cells, it was evident that five subpopulations, CLU+ CAFs-C1, SMPD1+ CAFs-C2, CTSH+ CAFs-C3, GNPTAB+ CAFs-C4, and IL13RA2+ CAFs-C6, were predominant in CAFs cellular communications. Metabolic pathway activity analysis revealed higher metabolic pathway activity in the CLU+ CAFs-C1, IL13RA2+ CAFs-C6 subpopulations among the nine lysosome-dependent cell death-associated CAFs subpopulations (Figure 7F). KEGG enrichment analysis based on the differential expressed genes of each cellular subpopulation revealed that these cellular subpopulations were mainly associated with kaposi sarcoma-associated herpesvirus infection, cellular senescence, carbon metabolism, transforming growth factor beta (TGF-beta), and NOD-like receptor signaling pathways (Figure 7G).
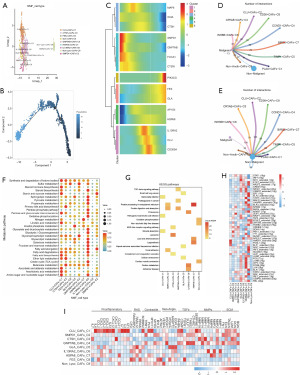
In addition, SCENIC analysis showed that lysosome-dependent cell death-associated CAFs subpopulations activated potential TFs to different degrees (Figure 7H). Notably, the expression of key CAF phenotypic marker surface protein genes was also very different for different cellular subpopulations, whereas CLU+ CAFs-C1 predominantly expressed inflammatory factors (Figure 7I).
Novel anoikis-mediated CAFs have an impact on TME in CRC
We newly defined nine subpopulations of anoikis-associated CAFs by NMF clustering, PLAU+ CAFs-C1, CLU+ CAFs-C2, CRYAB+ CAFs-C3, CCND1+ CAFs-C4, TIMP1+ CAFs-C5, INHBB+ CAFs-C6, BIRC3+ CAFs-C7, CD36+ CAFs-C8 and non-anoik-CAFs-C9 (Figure 8A). We found that loss-of-nest apoptosis major regulatory genes were highly expressed during late CAF development (Figure 8B,8C). Cellular communication analysis revealed that these nine subpopulations of anoikis-associated CAFs communicated more with non-malignant epithelial cells compared to malignant epithelial cells (Figure 8D,8E). Based on the number of communications between these subpopulations and tumor epithelial cells, it is evident that the CLU+ CAFs-C2 and INHBB+ CAFs-C6 subpopulations dominate CAFs cellular communication. Metabolic pathway activity analysis revealed that the metabolic pathway activity of CLU+ CAFs-C2 and CD36+ CAFs-C8 subpopulations was higher among the nine anoikis-associated CAFs subpopulations (Figure 8F). KEGG enrichment analysis revealed that CLU+ CAFs-C2 was mainly associated with TNF signaling pathway, proteoglycans and cancers; whereas CD36+ CAFs-C8 was mainly associated with Nguyen virus disease, Parkinson’s disease and Huntington’s disease, among other human diseases (Figure 8G).
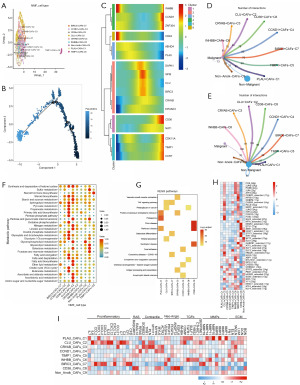
SCENIC analysis showed that subpopulations of anoikis-associated CAFs were differentially activated by potential TFs (Figure 8H). In addition, the expression of key CAFs phenotypic markers surface protein genes was distinctly different in different cell subpopulations, and CLU+ CAFs-C2 predominantly expressed inflammatory factors (Figure 8I).
Novel paraptosis-mediated CAFs have an impact on TME in CRC
We used NMF clustering to newly define 11 subpopulations of paraptosis-associated CAFs (Figure 9A), and they are LPAR1+ CAFs-C1, MYLK+ CAFs-C2, CFD+ CAFs-C3, DDIT3+ CAFs-C4, PDCD6IP+ CAFs-C5, CDK4+ CAFs-C6, CTDSP2+ CAFs-C7, HSPB8+ CAFs-C8, CSF1+ CAFs-C9, unclear-para-CAFs-C10, and non-para-CAFs-C11. After pseudotime trajectory analysis (Figure 9B), we found that the major regulatory genes of paraptosis were expressed at all periods of CAFs development (Figure 9C) and that the genes characteristic of CAFs in early development: TNK2, CASP7, CTDSP2, HSPB8; and genes characteristic of late development: CDKN3, PDCD6IP, MCU. By cellular communication analysis we found that these 11 subpopulations of paraptosis-associated CAFs communicated with non-malignant epithelial cells in greater numbers compared to malignant epithelial cells (Figure 9D,9E). Based on the number of communications between these subpopulations and tumor epithelial cells, it was seen that the LPAR1+ CAFs-C1, CFD+ CAFs-C3, DDIT3+ CAFs-C4, and unclear-para-CAFs-C10 subpopulations were dominant in CAFs cellular communications. Metabolic pathway activity analysis revealed that the LPAR1+ CAFs-C1, CFD+ CAFs-C3, and unclear-para-CAFs-C10 subpopulations had higher metabolic pathway activities among the 11 subpopulations of paraptosis-associated CAFs (Figure 9F). It is noteworthy that all of these cellular subpopulations dominated cellular communication. Furthermore, through the KEGG enrichment analysis of genes exhibiting variances across different subpopulations (Figure 9G), it was observed that the 11 CAFs subpopulations linked to paraptosis were connected with processes such as protein processing in ER, protein digestion and absorption, TNF and IL-17 signaling pathways, extracellular matrix (ECM) receptor interaction. Notably, two specific cell subpopulations, namely DDIT3+ CAFs-C4 and CDK4+ CAFs-C6, were found to be associated with ferroptosis.
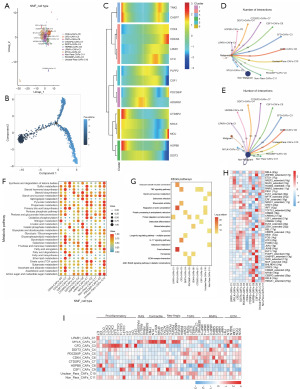
SCENIC analysis showed that subpopulations of paraptosis-associated CAFs were differentially activated by potential TFs (Figure 9H). In addition, the expression of key CAFs phenotypic markers surface protein genes was distinctly different in different cellular subpopulations (Figure 9I).
Various PCD-mediated CAFs have an impact on the prognosis of CRC
After comprehending the roles of eight PCD-mediated CAFs, an investigation was conducted to assess the influence of these cellular subpopulations on the prognosis of CRC patients. This involved a reassessment of the differential genes associated with these cell subpopulations in order to elucidate their distinct characteristics. Subsequently, we employed the GSVA method to compute the scores for each set of DEGs, and then conducted a univariate Cox analysis to investigate the correlation between these genes and the prognosis of CRC patients. As illustrated in Figure 10A, the subpopulations of CAFs characterized by apoptosis had a relatively constrained impact on the prognosis of patients with CRC. The most notable among these subpopulations of CAFs was the HSPA1A+ CAFs, identified as a risk factor impacting the prognosis of CRC patients in only two cohorts (TCGA-CRC, GSE39582). The pyroptosis-mediated subpopulations of CAFs collectively influenced the prognosis of patients with CRC. However, the PRKACA+ CAFs subpopulation, which was the most notable, also served as a risk factor in just two cohorts (GSE39582, GSE17536) (Figure 10B). The ferroptosis-mediated subpopulations of CAFs exhibited a diminished impact on patients with CRC, acting as a potential risk factor in just one of the cohorts analyzed (GSE17536) (Figure 10C). The various subpopulations of autophagy-mediated CAFs exhibit a significant prognostic influence on CRC patients, with each subpopulation serving as a risk factor in one to two cohorts. Notably, in the GSE17536 cohort, CLU+ CAFs, DRAM1+ CAFs, OPTN+ CAFs, and LZTS1+ CAFs were identified as risk factors, while HSPA8+ CAFs were found to be risk factors in the GSE39582 and TCGA-CRC cohorts (Figure 10D). The necroptosis-mediated subpopulation of CAFs had a limited influence on the prognosis of patients with CRC, serving as a risk factor in just one cohort (TCGA-CRC or GSE17536) (Figure 10E). The lysosome-dependent cell death-mediated CAFs subpopulation collectively influenced the prognosis of CRC patients, with the most notable subpopulations being HSPA8+ CAFs and non-lyso-CAFs (Figure 10F). Conversely, the impact of the anoikis-mediated CAFs subpopulation on CRC patient prognosis was less pronounced, with only the CRYAB+ CAFs subpopulation identified as a risk factor in both cohorts (TCGA-CRC, GSE17536) (Figure 10G). Among the various PCD mechanisms studied, the paraptosis-mediated CAFs subpopulation had the most significant effect on CRC patient prognosis, particularly the DDIT3+ CAFs subpopulation, which was identified as a risk factor in three cohorts (TCGA-CRC, GSE39582, GSE17536) (Figure 10H). Furthermore, the MYLK+ CAFs and HSPB8+ CAFs subpopulations were also identified as risk factors in two cohorts.
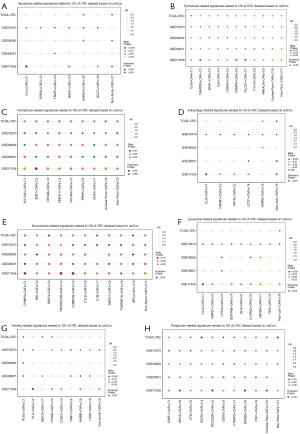
DDIT3 may affect the prognosis of CRC patients
In order to identify critical genes that influence the prognosis of CRC patients, we conducted an analysis of subpopulations of CAFs that are significantly associated with prognosis, specifically focusing on DDIT3+ CAFs, HSPB8+ CAFs, and MYLK+ CAFs. Initially, patients were categorized into high-score and low-score groups based on the median GSVA scores within each cohort, followed by the generation of Kaplan-Meier survival curves. The findings indicated that in the cohorts GSE17536 (Figure 11A), GSE39582 (Figure 11B), and TCGA-CRC (Figure 11C), there were notable differences in survival outcomes between the high and low score groups of CRC patients with DDIT3+ CAFs. Conversely, HSPB8+ CAFs (Figure S3A,S3B) and MYLK+ CAFs (Figure S3C,S3D) exhibited significant survival differences in only two of the cohorts analyzed. Furthermore, within the TCGA-CRC cohort, we constructed Kaplan-Meier survival curves based on the median expression levels of the three characteristic genes associated with the subpopulations, revealing that only the survival difference between the high and low expression groups of DDIT3 was statistically significant (Figure 11D, Figure S3E,S3F). We further analyzed the expression of DDIT3 in various types of cells and showed the differential expression between cancer tissues and adjacent non-cancer tissues. The results indicated significant differential expression of DDIT3 in B cells, T cells, epithelial cells, and stromal cells (Figure 11E), and in the TCGA-CRC cohort, DDIT3 was significantly higher expressed in cancer tissue cells compared to adjacent non-cancer tissues (Figure 11F). It is noteworthy that there is a positive correlation between the T-stage (Figure 11G), N-stage (Figure 11H), M-stage (Figure 11I), and stage (Figure 11J) with the expression of DDIT3 (Figure 11G-11J). Besides, immunohistochemical analysis also showed high expression of DDIT3 in CRC tissue (Figure 11K). To explore the clinical relevance of DDIT3 in CRC, a study was conducted analyzing the association between DDIT3 protein levels and clinicopathological characteristics in 19 CRC patients (Table 1). The results indicated a significant correlation between DDIT3 expression and tumor node metastasis (TNM) stage (P=0.008) as well as OS (P=0.04) (Table 2). These findings align with data obtained from bulk-seq analysis, suggesting a potential prognostic impact of DDIT3 on CRC patients.
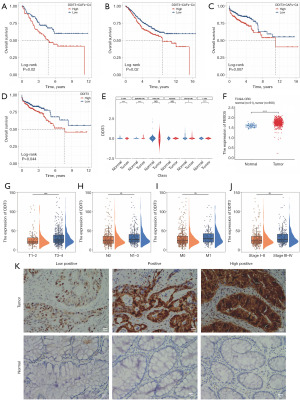
Table 1
ID | Gender | Age (years) | TNM | LNM | OS | DDIT3 IHC score |
---|---|---|---|---|---|---|
201838158 | Female | 66 | II | Yes | Yes | High positive |
201814697 | Male | 64 | II | Yes | Yes | High positive |
201809418 | Female | 58 | II–III | Yes | Yes | Positive |
201816826 | Male | 65 | II–III | Yes | Yes | High positive |
201833176 | Female | 43 | II–III | Yes | Yes | Positive |
201835370 | Male | 72 | I | No | Yes | Positive |
201833323 | Female | 58 | II | No | Yes | Low positive |
201815661 | Female | 70 | II–III | No | Yes | Positive |
201816484 | Male | 82 | II–III | No | Yes | Positive |
201846696 | Male | 72 | II–III | Yes | Yes | High positive |
201840608 | Male | 55 | II–III | No | Yes | Positive |
201831504 | Female | 65 | II–III | Yes | Yes | Positive |
201807722 | Male | 73 | I–II | No | No | Low positive |
201810330 | Male | 54 | I | Yes | No | Positive |
201838988 | Male | 70 | I | Yes | No | Positive |
201850118 | Male | 49 | I | Yes | No | Low positive |
201812559 | Female | 68 | I | No | No | Low positive |
201824151 | Female | 54 | I | Yes | No | Positive |
201822781 | Male | 64 | I–II | No | No | Low positive |
CRC, colorectal cancer; TNM, tumor-node-metastasis; LNM, lymph node metastasis; OS, overall survival; DDIT3 IHC score, immunohistochemical score of DDIT3.
Table 2
Patients’ characteristics | High positive (n=4) | Low positive (n=5) | Positive (n=10) | P value |
---|---|---|---|---|
Gender | 0.83 | |||
Female | 1 (25.0) | 2 (40.0) | 5 (50.0) | |
Male | 3 (75.0) | 3 (60.0) | 5 (50.0) | |
Age (years) | 0.32 | |||
<60 | 0 (0.0) | 2 (40.0) | 5 (50.0) | |
≥60 | 4 (100.0) | 3 (60.0) | 5 (50.0) | |
TNM | 0.008 | |||
I | 0 (0.0) | 2 (40.0) | 4 (40.0) | |
I–II | 0 (0.0) | 2 (40.0) | 0 (0.0) | |
II | 2 (50.0) | 1 (20.0) | 0 (0.0) | |
II–III | 2 (50.0) | 0 (0.0) | 6 (60.0) | |
LNM | 0.06 | |||
No | 0 (0.0) | 4 (80.0) | 4 (40.0) | |
Yes | 4 (100.0) | 1 (20.0) | 6 (60.0) | |
OS | 0.04 | |||
No | 0 (0.0) | 4 (80.0) | 3 (30.0) | |
Yes | 4 (100.0) | 1 (20.0) | 7 (70.0) |
CRC, colorectal cancer; TNM, tumor-node-metastasis; LNM, lymph node metastasis; OS, overall survival.
Discussion
Up to date, there is limited research on the involvement of PCD regulatory genes in cancer progression, particularly in CRC (34,35). This study is the first to extensively investigate the expression of crucial PCD regulatory genes in CRC-CAFs and to elucidate the interactions between various PCD-mediated CAFs subpopulations and cancer cells. This unique approach provides insights into how different CAFs subpopulations influence the prognosis of CRC patients.
During cell differentiation, there is a notable variation in gene expression between cells before and after differentiation (36). Our study utilizing pseudotime analysis revealed alterations in the expression of critical regulators of PCD during the differentiation process of CAFs, leading to the identification of distinct genes associated with early and late-stage CAFs development. Notably, certain characteristic genes identified in this study have been found to have specific functions in CRC cells. For instance, TRAP1, an apoptosis-related gene, has been shown to modulate the response of CRC cells to hypoxia (37,38), while HMOX1, a gene related to ferroptosis, enhances the growth, tolerance, and survival of CRC cells under oxidative stress (39). Additionally, PINK1, an autophagy-related gene, has been found to reprogram metabolism by activating p53, thereby reducing acetyl-CoA production and inhibiting colon tumor growth (40). These findings underscore the significance of investigating key genes involved in the regulation of PCD.
Epithelial cells play a crucial role in tumor development as they are the primary component of tumor tissue. The heterogeneity of cancer epithelial cells influences a patient’s response to treatment and prognosis. Our study utilized the inferCNV algorithm to determine the CNV score of cancer epithelial cells, categorizing them into malignant and relatively non-malignant cells. Through differential gene functional enrichment analysis of cell subpopulations, relatively non-malignant cells exhibited higher enrichment in energy metabolism and pathways related to cancer formation. Research suggests that tumor progression is not solely driven by genetic mutations but is also influenced by the TME (41). Recent literature highlights the significance of CAFs as key regulators in tumor pathogenesis (42). Communication analysis revealed a higher number of interactions between all PCD-mediated CAFs subpopulations and relatively non-malignant cells compared to malignant cells. Furthermore, differential gene enrichment analysis of CAFs subpopulations, such as IL1B+ CAFs and IL6+ CAFs, suggests their involvement in ECM receptor signaling and HIF-1 pathways, potentially contributing to CRC development (43,44). These findings lead us to hypothesize that CAFs may promote malignant tumor growth, and the identification of distinct CAFs subpopulations may aid in further elucidating their role in tumor progression.
In order to investigate the cell-specific gene regulatory network, an analysis of TFs at the single-cell level was conducted. Each subpopulation of CAFs exhibited distinct characteristics in terms of TF expression. The process of epithelial-to-mesenchymal transition (EMT) in CRC cells has been identified to enhance metastasis (45,46), consequently impacting patient prognosis. Previous research has indicated that heightened activity of TF families such as Snail, ZEB, and Twist can facilitate EMT in tumor cells (47). Our study revealed that specific subgroups like ZEB1+ CAFs, DRAM1+ CAFs, and CTSH+ CAFs exhibited a notable increase in ZEB1 transcriptional activity. ZEB1 was observed to influence YAP1 activity in diverse contexts and regulate the YAP1 pathway in CAFs (48), thereby modulating the ECM through upregulation of various cytoskeletal regulatory factors (e.g., ANLN and DIAPH3) and control of MYL9 protein levels (49). Consequently, ZEB1 can directly and indirectly impact the organization and remodeling of extracellular fibers by transmitting tension through adhesion. Ultimately, pivotal regulatory genes associated with PCD may influence specific TF regulatory systems, consequently contributing to the metastasis of CRC cells.
Utilizing Cox regression analysis and Kaplan-Meier survival analysis of DEGs within distinct subpopulations, insights have been garnered regarding the influence of these subpopulations on patient prognosis. Notably, the paraptosis-mediated DDIT3+ CAFs subpopulation emerged as particularly impactful on the prognosis of CRC patients. A recent study has reported that genes mediating paraptosis in CAFs promote tumor development (22). At the same time, inducing paraptosis in CRC cells is gradually being recognized as a potential therapeutic approach. Interventions targeting this mechanism may open new avenues for the treatment of CRC (50-52). Intriguingly, enrichment analysis of DEGs within the paraptosis-related DDIT3+ CAFs subpopulation unveiled an association with ferroptosis. Additionally, research has demonstrated that DDIT3 can trigger ferroptosis in conditions such as ankylosing spondylitis and non-small cell lung cancer (53,54). A previous review has explored the interplay between ferroptosis and apoptosis via ER stress (55). Likewise, paraptosis processes mediated by the ER may intersect with ferroptosis through this organelle, warranting further investigation in forthcoming studies.
In order to delve deeper into the association between the DDIT3 gene and CRC, it was observed that DDIT3 exhibits elevated expression levels in cancerous tissues, impacting the TNM staging and OS rates of CRC patients. A review of existing literature revealed that DDIT3 functions as a versatile TF within the ER stress response (56,57). Its pivotal role involves responding to diverse cellular stressors, leading to cell cycle arrest and apoptosis upon ER stress induction. Despite its established functions in cellular stress responses, the involvement of DDIT3 in cancer, particularly in CRC, remains relatively underexplored. Consequently, future research endeavors are encouraged to delve into the specific mechanisms through which DDIT3 operates in cancer, with the potential to unveil novel insights for targeted therapeutic interventions in oncology.
The primary limitations of our preliminary analysis stem from the shallow depth of single-cell sequencing and inadequate sample size, necessitating further validation of our findings through additional basic and clinical experiments. Given that reliance on a singular random forest algorithm may impose constraints on the identification of prognostic-related genes, it is advisable to employ multiple machine learning techniques in future research endeavors aimed at screening for these genes. Furthermore, the unique characteristics of the NMF algorithm pose constraints on our study, as it tends to offer only partial data representation, potentially introducing bias into the clustering methods employed. Moreover, the limited sample size constrains the interpretative strength of the DDIT3 immunohistochemistry findings. Nonetheless, this research presents a fresh viewpoint by elucidating the regulatory patterns of various PCDs in CAFs from a single-cell perspective. It also highlights the notable impact of paraptosis regulatory pattern in CAFs on the prognosis of CRC patients, thereby offering novel insights for CRC investigations.
Conclusions
In this research, we characterized distinct subtypes of CAFs influenced by eight key regulators of PCD that are crucial in CRC. Furthermore, our findings suggest that PCD-related intercellular signaling mechanisms may be involved in the control of malignant tumor progression and could impact patient outcomes. Notably, DDIT3+ CAFs associated with paraptosis exhibited the most pronounced influence on the prognosis of individuals with CRC.
Acknowledgments
None.
Footnote
Reporting Checklist: The authors have completed the MDAR reporting checklist. Available at https://tcr.amegroups.com/article/view/10.21037/tcr-24-1301/rc
Data Sharing Statement: Available at https://tcr.amegroups.com/article/view/10.21037/tcr-24-1301/dss
Peer Review File: Available at https://tcr.amegroups.com/article/view/10.21037/tcr-24-1301/prf
Funding: This study was supported by
Conflicts of Interest: All authors have completed the ICMJE uniform disclosure form (available at https://tcr.amegroups.com/article/view/10.21037/tcr-24-1301/coif). The authors have no conflicts of interest to declare.
Ethical Statement: The authors are accountable for all aspects of the work in ensuring that questions related to the accuracy or integrity of any part of the work are appropriately investigated and resolved. The study was conducted in accordance with the Declaration of Helsinki (as revised in 2013). The study was approved by the Ethics Committee of Bengbu Medical University (No. 2023243). All participants provided written informed consent before the study, and we obtained informed consent from the parents and/or legal guardians of patients under the age of 18 years.
Open Access Statement: This is an Open Access article distributed in accordance with the Creative Commons Attribution-NonCommercial-NoDerivs 4.0 International License (CC BY-NC-ND 4.0), which permits the non-commercial replication and distribution of the article with the strict proviso that no changes or edits are made and the original work is properly cited (including links to both the formal publication through the relevant DOI and the license). See: https://creativecommons.org/licenses/by-nc-nd/4.0/.
References
- Patel SG, Karlitz JJ, Yen T, et al. The rising tide of early-onset colorectal cancer: a comprehensive review of epidemiology, clinical features, biology, risk factors, prevention, and early detection. Lancet Gastroenterol Hepatol 2022;7:262-74.
- Tariq K, Ghias K. Colorectal cancer carcinogenesis: a review of mechanisms. Cancer Biol Med 2016;13:120-35.
- Bray F, Ferlay J, Soerjomataram I, et al. Global cancer statistics 2018: GLOBOCAN estimates of incidence and mortality worldwide for 36 cancers in 185 countries. CA Cancer J Clin 2018;68:394-424.
- Biffi G, Tuveson DA. Diversity and Biology of Cancer-Associated Fibroblasts. Physiol Rev 2021;101:147-76.
- Chen Y, McAndrews KM, Kalluri R. Clinical and therapeutic relevance of cancer-associated fibroblasts. Nat Rev Clin Oncol 2021;18:792-804.
- Ren J, Ding L, Zhang D, et al. Carcinoma-associated fibroblasts promote the stemness and chemoresistance of colorectal cancer by transferring exosomal lncRNA H19. Theranostics 2018;8:3932-48.
- Kasashima H, Duran A, Martinez-Ordoñez A, et al. Stromal SOX2 Upregulation Promotes Tumorigenesis through the Generation of a SFRP1/2-Expressing Cancer-Associated Fibroblast Population. Dev Cell 2021;56:95-110.e10.
- Zhang C, Wang XY, Zhang P, et al. Cancer-derived exosomal HSPC111 promotes colorectal cancer liver metastasis by reprogramming lipid metabolism in cancer-associated fibroblasts. Cell Death Dis 2022;13:57.
- Liu X, Qin J, Nie J, et al. ANGPTL2+cancer-associated fibroblasts and SPP1+macrophages are metastasis accelerators of colorectal cancer. Front Immunol 2023;14:1185208.
- Chen S, Fan L, Lin Y, et al. Bifidobacterium adolescentis orchestrates CD143(+) cancer-associated fibroblasts to suppress colorectal tumorigenesis by Wnt signaling-regulated GAS1. Cancer Commun (Lond) 2023;43:1027-47.
- Peng F, Liao M, Qin R, et al. Regulated cell death (RCD) in cancer: key pathways and targeted therapies. Signal Transduct Target Ther 2022;7:286.
- Chen C, Liu J, Lin X, et al. Crosstalk between cancer-associated fibroblasts and regulated cell death in tumors: insights into apoptosis, autophagy, ferroptosis, and pyroptosis. Cell Death Discov 2024;10:189.
- Tang H, You T, Ge H, et al. Anlotinib may enhance the efficacy of anti-PD1 therapy by inhibiting the AKT pathway and promoting the apoptosis of CAFs in lung adenocarcinoma. Int Immunopharmacol 2024;133:112053.
- Ershaid N, Sharon Y, Doron H, et al. NLRP3 inflammasome in fibroblasts links tissue damage with inflammation in breast cancer progression and metastasis. Nat Commun 2019;10:4375.
- Yuan M, Tu B, Li H, et al. Cancer-associated fibroblasts employ NUFIP1-dependent autophagy to secrete nucleosides and support pancreatic tumor growth. Nat Cancer 2022;3:945-60.
- Zhang X, Lao M, Yang H, et al. Targeting cancer-associated fibroblast autophagy renders pancreatic cancer eradicable with immunochemotherapy by inhibiting adaptive immune resistance. Autophagy 2024;20:1314-34.
- Qi R, Bai Y, Li K, et al. Cancer-associated fibroblasts suppress ferroptosis and induce gemcitabine resistance in pancreatic cancer cells by secreting exosome-derived ACSL4-targeting miRNAs. Drug Resist Updat 2023;68:100960.
- Li Y, Ma Z, Li W, et al. PDPN(+) CAFs facilitate the motility of OSCC cells by inhibiting ferroptosis via transferring exosomal lncRNA FTX. Cell Death Dis 2023;14:759.
- Li J, Chen S, Liao Y, et al. Arecoline Is Associated With Inhibition of Cuproptosis and Proliferation of Cancer-Associated Fibroblasts in Oral Squamous Cell Carcinoma: A Potential Mechanism for Tumor Metastasis. Front Oncol 2022;12:925743.
- Herbert A, Balachandran S. Z-DNA enhances immunotherapy by triggering death of inflammatory cancer-associated fibroblasts. J Immunother Cancer 2022;10:e005704.
- Weigel KJ, Jakimenko A, Conti BA, et al. CAF-secreted IGFBPs regulate breast cancer cell anoikis. Mol Cancer Res 2014;12:855-66.
- Dai YJ, Tang HD, Jiang GQ, et al. The immunological landscape and silico analysis of key paraptosis regulator LPAR1 in gastric cancer patients. Transl Oncol 2024;49:102110.
- Zou Y, Xie J, Zheng S, et al. Leveraging diverse cell-death patterns to predict the prognosis and drug sensitivity of triple-negative breast cancer patients after surgery. Int J Surg 2022;107:106936.
- Xu K, Li D, Qian J, et al. Single-cell disulfidptosis regulator patterns guide intercellular communication of tumor microenvironment that contribute to kidney renal clear cell carcinoma progression and immunotherapy. Front Immunol 2024;15:1288240.
- Jin S, Guerrero-Juarez CF, Zhang L, et al. Inference and analysis of cell-cell communication using CellChat. Nat Commun 2021;12:1088.
- Liberzon A, Birger C, Thorvaldsdóttir H, et al. The Molecular Signatures Database (MSigDB) hallmark gene set collection. Cell Syst 2015;1:417-25.
- Smillie CS, Biton M, Ordovas-Montanes J, et al. Intra- and Inter-cellular Rewiring of the Human Colon during Ulcerative Colitis. Cell 2019;178:714-730.e22.
- Chen YP, Yin JH, Li WF, et al. Single-cell transcriptomics reveals regulators underlying immune cell diversity and immune subtypes associated with prognosis in nasopharyngeal carcinoma. Cell Res 2020;30:1024-42.
- Gavish A, Tyler M, Greenwald AC, et al. Hallmarks of transcriptional intratumour heterogeneity across a thousand tumours. Nature 2023;618:598-606.
- Qiu X, Mao Q, Tang Y, et al. Reversed graph embedding resolves complex single-cell trajectories. Nat Methods 2017;14:979-82.
- Wu Y, Yang S, Ma J, et al. Spatiotemporal Immune Landscape of Colorectal Cancer Liver Metastasis at Single-Cell Level. Cancer Discov 2022;12:134-53.
- Fang Z, Meng Q, Xu J, et al. Signaling pathways in cancer-associated fibroblasts: recent advances and future perspectives. Cancer Commun (Lond) 2023;43:3-41.
- Gascard P, Tlsty TD. Carcinoma-associated fibroblasts: orchestrating the composition of malignancy. Genes Dev 2016;30:1002-19.
- Qin H, Abulaiti A, Maimaiti A, et al. Integrated machine learning survival framework develops a prognostic model based on inter-crosstalk definition of mitochondrial function and cell death patterns in a large multicenter cohort for lower-grade glioma. J Transl Med 2023;21:588.
- Zhang Y, Wang Y, Chen J, et al. A programmed cell death-related model based on machine learning for predicting prognosis and immunotherapy responses in patients with lung adenocarcinoma. Front Immunol 2023;14:1183230.
- Zhou F, Tan P, Liu S, et al. Subcellular RNA distribution and its change during human embryonic stem cell differentiation. Stem Cell Reports 2024;19:126-40.
- Maddalena F, Condelli V, Matassa DS, et al. TRAP1 enhances Warburg metabolism through modulation of PFK1 expression/activity and favors resistance to EGFR inhibitors in human colorectal carcinomas. Mol Oncol 2020;14:3030-47.
- Bruno G, Li Bergolis V, Piscazzi A, et al. TRAP1 regulates the response of colorectal cancer cells to hypoxia and inhibits ribosome biogenesis under conditions of oxygen deprivation. Int J Oncol 2022;60:79.
- Singhabahu R, Kodagoda Gamage SM, Gopalan V. Pathological significance of heme oxygenase-1 as a potential tumor promoter in heme-induced colorectal carcinogenesis. Cancer Pathog Ther 2024;2:65-73.
- Yin K, Lee J, Liu Z, et al. Mitophagy protein PINK1 suppresses colon tumor growth by metabolic reprogramming via p53 activation and reducing acetyl-CoA production. Cell Death Differ 2021;28:2421-35.
- Cai Z, Yao H, Chen J, et al. Schwann cells in pancreatic cancer: Unraveling their multifaceted roles in tumorigenesis and neural interactions. Cancer Lett 2024;587:216689.
- Peng Z, Ye M, Ding H, et al. Spatial transcriptomics atlas reveals the crosstalk between cancer-associated fibroblasts and tumor microenvironment components in colorectal cancer. J Transl Med 2022;20:302.
- Yamamoto Y, Kasashima H, Fukui Y, et al. The heterogeneity of cancer-associated fibroblast subpopulations: Their origins, biomarkers, and roles in the tumor microenvironment. Cancer Sci 2023;114:16-24.
- Xu H, Zhao J, Li J, et al. Cancer associated fibroblast-derived CCL5 promotes hepatocellular carcinoma metastasis through activating HIF1α/ZEB1 axis. Cell Death Dis 2022;13:478.
- Yang C, Dou R, Wei C, et al. Tumor-derived exosomal microRNA-106b-5p activates EMT-cancer cell and M2-subtype TAM interaction to facilitate CRC metastasis. Mol Ther 2021;29:2088-107.
- Dou R, Liu K, Yang C, et al. EMT-cancer cells-derived exosomal miR-27b-3p promotes circulating tumour cells-mediated metastasis by modulating vascular permeability in colorectal cancer. Clin Transl Med 2021;11:e595.
- Baulida J. Epithelial-to-mesenchymal transition transcription factors in cancer-associated fibroblasts. Mol Oncol 2017;11:847-59.
- Selth LA, Das R, Townley SL, et al. A ZEB1-miR-375-YAP1 pathway regulates epithelial plasticity in prostate cancer. Oncogene 2017;36:24-34.
- Calvo F, Ege N, Grande-Garcia A, et al. Mechanotransduction and YAP-dependent matrix remodelling is required for the generation and maintenance of cancer-associated fibroblasts. Nat Cell Biol 2013;15:637-46.
- Kim NY, Mohan CD, Sethi G, et al. Cannabidiol activates MAPK pathway to induce apoptosis, paraptosis, and autophagy in colorectal cancer cells. J Cell Biochem 2024;125:e30537.
- Rao X, Li Z, Zhang Q, et al. α-Hederin induces paraptosis by targeting GPCRs to activate Ca(2+)/MAPK signaling pathway in colorectal cancer. Cancer Med 2024;13:e7202.
- Zhao S, Meng Y, Cai W, et al. Docosahexaenoic Acid Coordinating with Sodium Selenite Promotes Paraptosis in Colorectal Cancer Cells by Disrupting the Redox Homeostasis and Activating the MAPK Pathway. Nutrients 2024;16:1737.
- Li Q, Chen Z, Yang C, et al. Role of ferroptosis-associated genes in ankylosing spondylitis and immune cell infiltration. Front Genet 2022;13:948290.
- Han S, Yang X, Zhuang J, et al. α-Hederin promotes ferroptosis and reverses cisplatin chemoresistance in non-small cell lung cancer. Aging (Albany NY) 2024;16:1298-317.
- Lee YS, Lee DH, Choudry HA, et al. Ferroptosis-Induced Endoplasmic Reticulum Stress: Cross-talk between Ferroptosis and Apoptosis. Mol Cancer Res 2018;16:1073-6.
- Yamaguchi H, Wang HG. CHOP is involved in endoplasmic reticulum stress-induced apoptosis by enhancing DR5 expression in human carcinoma cells. J Biol Chem 2004;279:45495-502.
- Ohoka N, Yoshii S, Hattori T, et al. TRB3, a novel ER stress-inducible gene, is induced via ATF4-CHOP pathway and is involved in cell death. EMBO J 2005;24:1243-55.