Diagnostic value of a lactylation-related gene signature in hepatocellular carcinoma
Highlight box
Key findings
• Three lactylation-related diagnostic gene signatures predicted the prognosis and response to immunotherapy in hepatocellular carcinoma (HCC) patients.
What is known and what is new?
• The lactylation-related genes are associated with HCC.
• We confirmed the diagnostic gene signature associated with lactylation.
What is the implication, and what should change now?
• Lactylation-related gene signature is closely related to the diagnosis, prognosis, drug sensitivity and immunotherapy of HCC patients.
Introduction
Hepatocellular carcinoma (HCC) is the most common primary liver cancer and has a high mortality rate (1,2). Unfortunately, the majority of HCC patients are diagnosed at an advanced stage, and existing treatment options, including surgical resection, liver transplantation, and non-surgical therapies, have limited efficacy. This results in a low 5-year survival rate and poor prognosis for HCC patients (3-5). Improving the early diagnosis and identification of HCC can significantly enhance treatment outcomes and prognosis (6,7). Currently, imaging, pathology, and serological examinations are widely used in the diagnosis and treatment of HCC (8-10). However, due to the invasive nature and economic constraints of imaging and pathological techniques, there is significant value in exploring new and accurate biomarkers, in combination with clinical prediction models, for early diagnosis and treatment of tumors.
In recent years, metabolic reprogramming has gradually become recognized as a hallmark of cancer, capable of regulating tumor cell behavior and immunity, thereby influencing tumor progression (11). The reprogramming of metabolic processes within HCC cells may exert direct or indirect alterations on the microenvironment, as well as modulating the functional characteristics of heterogeneous immune cell populations. This could consequently enable tumor cells to elude immune surveillance (12). Predicated on the Warburg effect, tumors exhibit preferential energy metabolism toward anaerobic glycolysis even in the presence of oxygen, rather than utilizing oxidative phosphorylation (OXPHOS) (13). Lactate has long been regarded as a byproduct and metabolic waste of glycolysis. However, recent studies have shown that lactate can offer a favorable condition for tumor growth by forming an acidic immune microenvironment and promoting lactylation (14,15). The process of lactylation, which involves a lactyl group added to the histone lysine residues, was initially identified in tumor environments where there is an accumulation of lactate (16,17). As a novel contributor to the epigenetic landscape, lactylation is closely related to tumorigenesis. Evidence has shown that lactylation is beneficial to tumorigenesis (18), immune evasion (19), and drug resistance (20). Although lactylation has become a focus of research, there are currently no reliable lactylation-related molecular markers to facilitate the early diagnosis and prognosis of HCC.
With the continuous development of research, clinical prediction model, including diagnostic model and prognostic model, is becoming an important part of clinical diagnosis and prognosis. Here, we gathered all of the currently published lactylation-related genes and constructed a lactylation-related genes model based on The Cancer Genome Atlas (TCGA) database. In addition, we validated the prognostic performance of the diagnostic model in the Gene Expression Omnibus (GEO) data. The expression of diagnosis-related lactylation-genes and cluster analysis about HCC was also performed. The workflow of this study is presented in Figure 1. Our objective was to identify a gene signature that could be employed for diagnostic applications. Our study extended to analyze the implications of our diagnostic model in modulating drug responsiveness and guiding strategies for immunotherapy. We present this article in accordance with the TRIPOD reporting checklist (available at https://tcr.amegroups.com/article/view/10.21037/tcr-24-1023/rc).
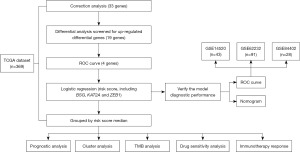
Methods
TCGA data collection and processing
This study was conducted in accordance with the Declaration of Helsinki (as revised in 2013). The Institutional Review Committee of the Human Research Ethics Committee of the Eighth Affiliated Hospital, Sun Yat-sen University approved the study. The IRB approval number of this study is ZB-KYIRB-AF/SC-08/01.0.
RNA-sequencing data and clinical profiles for a cohort of 369 HCC specimens, as well as 50 matched adjacent normal tissues, were retrieved from TCGA (https://portal.gdc.cancer.gov/) dataset. These datasets were then standardized by converting them into the transcripts per million (TPM) format, enabling a uniform framework for subsequent comparative analyses. The difference of gene expression between HCC and para-carcinoma tissue was compared and presented by bar graph. Exclude any individuals that contain missing data.
GEO datasets
GSE14520 (n=43), GSE84402 (n=28), and GSE62232 (n=91) were used for the external validation of the gene model in this study. The expression values at the probe level (probe ID) were transformed into the associated gene symbol based on their annotation files, without subsequent standardization. For genes represented by multiple probes, the gene expression values were consolidated as the average of the derived measurements. Finally, a total of 162 samples with liver cancer were enrolled for analysis. What’s more, receiver operating characteristic (ROC) curves provided the assessment framework for gauging the diagnostic precision of the risk score. Exclude any individuals that contain missing data.
Construction of the risk score formula
Survival information for TCGA-HCC patients was extracted, and a binary classification (1 for tumor, 0 for normal) was performed on 419 samples. Logistic regression analysis using SPSS provided partial regression coefficients (B) and P values. Using the derived equation, risk score for each patient were calculated based on the mRNA expression levels of basigin (BSG), lysine acetyltransferase 2A (KAT2A), and zinc finger E-box-binding homeobox 1 (ZEB1). A similar approach was applied to the GEO validation data.
Real-time quantitative polymerase chain reaction (RT-PCR)
The TRIzol lysis procedure was utilised to extract total RNA. Following this, a PrimeScript™ RT reagent Kit with gDNA Eraser (Takara, Beijing, China) was employed for conversion of the RNA into complementary DNA (cDNA). Subsequently, the TB Green® Premix Ex Taq™ (Takara, Beijing, China) was used to conduct RT-PCR as per the manufacturer’s guidelines.
Nomogram construction
In order to enhance the diagnostic potential, a nomogram was formulated employing lactylation-related signatures and risk score. A ROC analysis was performed to demonstrate survival at 1-, 3-, and 5-year intervals.
Cluster analysis
The HCC cohort was stratified using the “ConsensusClusterPlus” package to ascertain if there was an association between lactylation-related gene expression and HCC. For k=2, the intragroup correlation was robust while the intergroup correlation was moderate. Utilizing heatmap analyses, we correlated the expression profiles of lactylation-associated genes with the clinical parameters of patients across diverse subtypes of HCC. The expression of immune cells in different HCC types was quantified using the single-sample gene set enrichment analysis (ssGSEA) technique, followed by data representation using box plots.
Immunotherapy response
In order to predict the outcomes of immunotherapy within patient cohorts stratified by high and low risk score, we calculated a range of immunological parameters including Tumor Immune Dysfunction and Exclusion (TIDE), expression of programmed death-ligand 1 (CD274 or PD-L1), activity of interferon-gamma (IFNG), prevalence of myeloid-derived suppressor cells (MDSC), and the immunophenoscore (IPS).
Survival analysis
In order to scrutinize the prognostic implications of BSG, KAT2A, ZEB1 and risk score mRNA on HCC samples, the influence of these factors on overall survival (OS) in HCC patients was assessed using the “survival” software package. Additionally, log-rank P values were depicted.
Drug sensitivity analysis
In order to discern the relationship between drug susceptibility and lactylation-related signatures, the half-maximal inhibitory concentrations (IC50) of various drugs were computed through the usage of the “oncoPredict” R package. Comparisons between the IC50 values of high risk score and low risk score HCC group were made using t-tests.
Statistical analysis
All statistical evaluations were conducted with version 4.3.1 of the R programming language. To examine variations in patient survival times across the two risk score groups, we executed a Kaplan-Meier (KM) analysis in conjunction with a log-rank test, facilitated through the R “survival” package. All statistical investigations were two-tailed, and a P value that was less than 0.05 denoted a notable variance.
Results
Gene selection
To screen the genes that are associated with HCC lactylation, correlation analysis was performed to analyze the coefficient of correlation between 33 genes. Then, by dividing the training set and the test set, the weights of 33 genes of 419 samples were analyzed with the random forest algorithm (Figure 2A). For the two genes with a correlation greater than 0.3, the one with the higher weight was selected to be used in the following analysis (Table S1). Additionally, RNA-Seq was used to analyze the differentially expressed genes between 369 HCC tissues and 50 control tissues from patients, 12 significant genes were identified. To filter diagnosis-related lactylation-genes, the ROC curve was employed. The area under the curve (AUC) for KAT2A, BSG, ZEB1 and predicted model was 0.974 [95% confidence interval (CI): 0.9595–0.9895], 0.959 (95% CI: 0.9365–0.9807), 0.664 (95% CI: 0.6058–0.7219), and 0.989 (95% CI: 0.9801–0.9979), respectively (Figure 2B). The three lactylation-genes (BSG, KAT2A, and ZEB1) were selected to construct a signature for the logistic regression model (Table 1). The following equation was utilized to compute the risk score:
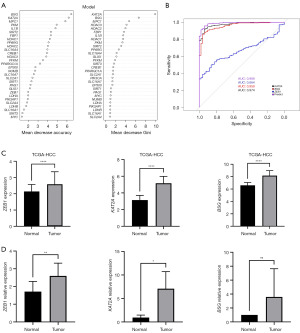
Table 1
Variables | β | S.E. | Wald | OR | P |
---|---|---|---|---|---|
BSG | 0.079 | 0.021 | 13.535 | 1.082 | 0.001 |
KAT2A | 0.730 | 0.170 | 18.370 | 2.075 | 0.001 |
ZEB1 | 1.190 | 0.539 | 4.882 | 3.289 | 0.03 |
Constant | −9.469 | 1.760 | 28.957 | 0.000 | 0.001 |
TCGA, The Cancer Genome Atlas; S.E., standard error, OR, odds ratio.
Box plot showed the expression of lactylation-related genes in normal samples and HCC samples in TCGA database (Figure 2C) and GEO dataset (Figure S1). Furthermore, we conducted an examination of BSG, KAT2A, and ZEB1 expression levels in a cohort comprising 10 HCC and corresponding normal liver tissues. The results of RT-PCR analysis revealed a significant upregulation of BSG, KAT2A, and ZEB1 expression in the HCC specimens compared to their paired normal liver counterparts (Figure 2D). Besides, the association between BSG or KAT2A or ZEB1 and risk score was presented in Figure S2.
Validation
To verify the diagnostic efficiency of the signature, ROC analysis showed that in the GSE14520 dataset (Table S2), the AUC was 0.797 (95% CI: 0.6598–0.9333); in the GSE84402 dataset (Table S3), the AUC was 0.699 (95% CI: 0.4887–0.9093); in the GSE62232 dataset (Table S4), the AUC was 0.886 (95% CI: 0.7834–0.9895), revealing that the diagnostic ability of the signature was fine (Figure 3A-3C). Then, gender, age and risk score were integrated into the nomogram (Figure 3D). In the 1-year ROC analysis of the TCGA cohort, the AUC for the lactylation-based diagnostic model registered at 0.673, decreasing to 0.624 at 3 years and further to 0.541 at 5 years (Figure 3E).
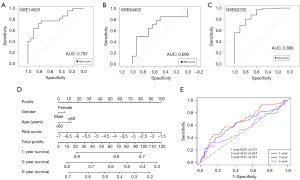
Prognostic analysis
Based on the expression levels of BSG, KAT2A, ZEB1, and the risk score, the TCGA patient cohort was stratified into high risk score and low risk score groups, as illustrated in Figure 4A. This stratification revealed that elevated levels of BSG, KAT2A, ZEB1, and the risk score were correlated with a poorer prognosis. Further investigation into the clinical significance of this signature revealed associations between the risk score and clinical parameters such as age, gender, T stage, N stage, M stage, and pathologic stage. Notably, higher risk score was observed at T3 compared to T1, at N1 compared to N0, and at pathologic stage III compared to I (P<0.001, P=0.03, P<0.001, respectively) (Figure 4B). The patients’ clinical characteristics are listed in Tables 2,3.
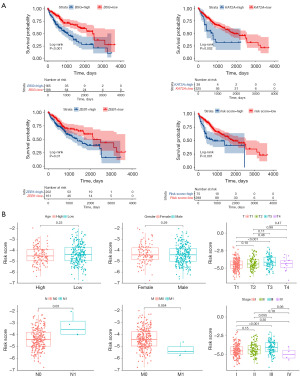
Table 2
Sample type | TCGA-LIHC (n=419) | GSE14520 (n=43) | GSE84402 (n=28) | GSE62232 (n=91) |
---|---|---|---|---|
Tumor | 369 | 22 | 14 | 81 |
Normal | 50 | 21 | 14 | 10 |
TCGA, The Cancer Genome Atlas; GEO, Gene Expression Omnibus; LIHC, liver hepatocellular carcinoma.
Table 3
Parameters | TCGA-LIHC (n=363) |
---|---|
Age (years) | |
≤65 | 136 |
>65 | 227 |
Survival status | |
Death | 129 |
Alive | 234 |
Gender | |
Male | 245 |
Female | 118 |
Stage | |
I | 170 |
II | 84 |
III | 81 |
IV | 4 |
Pathologic_M | |
M0 | 262 |
M1 | 3 |
MX | 98 |
Pathologic_N | |
N0 | 246 |
N1 | 4 |
NX | 113 |
Pathologic_T | |
T1 | 180 |
T2 | 91 |
T3 | 76 |
T4 | 13 |
TX | 1 |
TCGA, The Cancer Genome Atlas; LIHC, liver hepatocellular carcinoma.
Subsequent to the observed survival disparities between the high risk score and low risk score groups in HCC within the TCGA dataset, additional subgroup analyses were performed to affirm the predictive proficiency of the signature. The results showed a significantly better prognosis in low risk score HCC than high risk score HCC in the subgroups of age ≤65 years, age >65 years, male, T3–4, N0, M0, and pathologic stage III–IV (P=0.001, P=0.006, P<0.001, P<0.001, P=0.01, P=0.02, and P=0.002, respectively) (Figure 5).
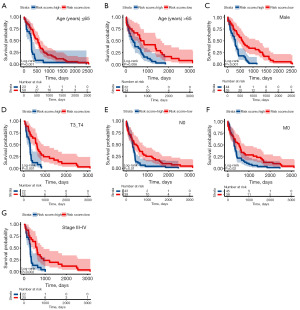
Cluster analysis
HCC specimens were classified into two distinct groups through a cluster analysis of genes associated with lactylation (Figure 6A-6D). Survival analysis methodologies were employed to evaluate the disparities in survival outcomes between two distinct clusters. It was observed that Cluster 2 exhibited a significantly reduced survival rate in comparison to Cluster 1 (Figure 6E). We also calculated the proportions of 22 varied immune cells in the two HCC clusters. There was significant variation in 11 types of immune cells between the two HCC clusters (Figure 6F).
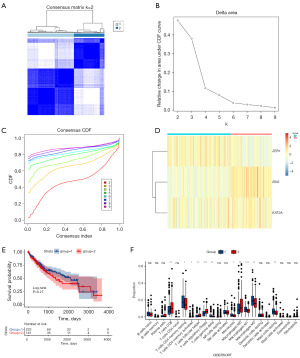
Tumor mutation burden (TMB) between high risk score and low risk score groups
In recent years, TMB has garnered significant attention in the research of immune checkpoint inhibitor (ICI)-related biomarkers: research shows that patients with a high tumor mutational burden (TMB-H) are more likely to benefit from ICI treatment (21). A higher TMB could generate more neoantigens, thereby increasing the chance of specific recognition by T cells. ICI-mediated tumor immunotherapy can eliminate the immune evasion of cancer cells. Initially, we analyzed the variations between high and low risk score groups in the top twenty genes that had the highest mutation frequencies in HCC (Figure 7A). We also compiled the variant classification using stacked bar plots and box plots to depict variant types and their quantities (Figure 7B). The most common type of variation was the missense mutation, and the occurrence of single nucleotide polymorphism (SNP) far exceeded other mutation types in HCC. Furthermore, the KM survival analysis indicated that patients with lower TMB had a longer OS compared to those with high TMB scores (Figure 7C). The mutation analysis data inferred that patients with high risk score could be more compatible for immunotherapy.
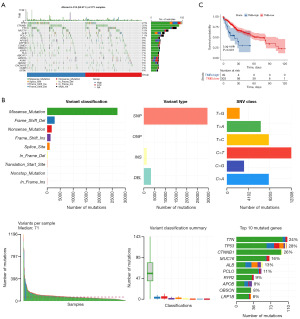
Drug sensitivity in high risk score and low risk score HCC
The sensitivity to various common drugs was forecasted by conducting drug analysis for high risk score and low risk score HCC (Figure 8). AZD2014, PF-4708671, irinotecan and nutlin-3a (−) showed higher sensitivity in low risk score group (P<0.001, P=0.02, P=0.01, P=0.04, respectively). However, pictilisib, 5-fluorouracil, cytarabine, sorafenib and vinblastine showed higher sensitivity in high risk score group (P<0.001, P=0.03, P=0.01, P=0.006, P<0.001, respectively).

Immunotherapy response
The TIDE computational framework was applied to determine the prospective clinical impact of immunotherapy across divergent risk score categories. The cohort with lower risk score exhibited decreased TIDE scores, intimating an increased probability of beneficial immunotherapeutic outcomes in patients with HCC within this subgroup (P<0.05) (Figure 9A). Moreover, the high risk score group exhibited a higher score for CD274, Exclusion, IFNG, and MDSC (Figure 9B-9E). However, the dysfunction and microsatellite instability (MSI) scores were less in the high risk score group (Figure 9F,9G). We observed varying immune therapy responses between the high risk score and low risk score groups. This may be due to the high risk score group typically having an inhibitory immune microenvironment, including suppressive immune cells and immune checkpoint molecules with high PD-L1 expression. In contrast, the low risk score group contains more immune effector cells, such as CD8+ T cells, resulting in a more effective immune therapy response.
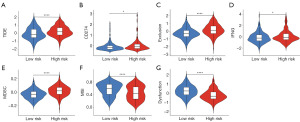
Discussion
The impact of a single gene on the prognosis of HCC has been previously identified (22). Lately, diagnostic models that combine gene expression and clinical features have become increasingly significant with the advancement of sequencing technology (4). In this context, we established a diagnostic model based on lactylation-related genes, which revealed a noticeable difference in OS between high risk score and low risk score subgroups. The 1-, 3-, and 5-year AUC, as indicated by ROC curves in the TCGA cohort, demonstrated high accuracy. Diagnostic-related lactylation-genes have the potential to be valuable prognostic biomarkers for HCC, providing support for targeted therapies related to lactylation-related genes. The TIDE score, which reflects the potential for immune surveillance evasion, could predict the response to immunotherapy (23). Patients in the low risk score group had lower TIDE scores, suggesting that individuals with a low risk score are likely to benefit more from ICI therapy. This may be due to the higher level of lactylation in the high risk score group, and the accumulation of lactate leads to the enrichment of immunosuppressive cells in the immune microenvironment, which weakens the function of effector T cells, resulting in poor immunotherapy response in the high risk score group. Typically, a higher TIDE score forecasts poorer patient outcomes, a finding that aligns with our survival analysis. In the future, this model can be applied to predict immunotherapy response and personalized medicine for patients. Additionally, we evaluated drug sensitivity, revealing that high risk score and low risk score HCC patients reacted differently to various anticancer drugs.
Lactylation has an effect on tumor development. In esophageal squamous cell carcinoma, hypoxia induces lactylation of histone H3K9 (H3K9la), thereby enhancing the transcription of laminin subunit gamma 2 (LAMC2) (24). In colorectal cancer, tumor-derived lactate promotes lysine lactylation of histone H3 at position 18 (H3K18la), enhancing the expression of the autophagy-related protein RUBCNL to promote tumor resistance (20). In addition, lactylation is closely related to immunity inhibition. Inhibition of MCT4 activates macrophage repair genes, thereby protecting against atherosclerosis through histone H3K18la (25). Studies have shown that lactylation is closely related to tumor immune escape. Zhang et al. demonstrated that H3K18la enhanced the immune evasion of non-small cell lung cancer (NSCLC) cells by activating the POM121/MYC/PD-L1 pathway (26). Intracellular lactate-driven histone lactation promotes the expression of IL-10, which is necessary to inhibit T cell activity (27). Lactate in TME promotes the dynamic lactylation of H3K18la, leading to transcriptional activation of VCAM1 in gastric cancer (GC) cells and up-regulation of CXCL1 expression, thereby promoting recruitment and infiltration of M2 macrophages (28). In summary, lactylation helps tumor cells to escape immune by affecting cell function in TME, suggesting that Kla may be a tumor therapeutic target. Targeting lactylation-related signaling pathways may offer new therapeutic targets and mechanisms for HCC treatment by altering tumor metabolism, regulating gene expression, and modulating the TME. For example, histone lactation promotes endometrial cancer progression by targeting the PI3K/AKT/HIF-1α signaling pathway (29). Histone lactylation promotes colorectal tumorigenesis by activating the TRAF6-IL-6-STAT3 signaling pathway (30). However, several drawbacks must be considered. Targeting cell proliferation-related signaling pathways may interfere with normal cellular functions, and cancer cells might evade inhibition by reprogramming their metabolism, leading to resistance in HCC treatment. Although lactylation pathways show potential in cancer therapy, it is crucial to address these potential off-target effects and limitations to develop safer and more effective treatments for HCC.
In our study, the lactylation-related gene signature includes three genes: BSG, KAT2A, and ZEB1. BSG, a transmembrane glycoprotein, plays a key role in the survival of cancer cells, primarily via the regulation of lactate transport through its liaison with monocarboxylate transporters (MCTs) like MCT1 (also termed as SLC16A1) and MCT4 (also referred to as SLC16A4) (31). It has been reported that the increased glycolysis activity of esophageal cancer is related to the increased expression levels of MCTs and CD147 (32). These cells also demonstrated an increased glycolytic rate in comparison to normal human melanocytes (33). This may enhance lactate transport, subsequently promoting the occurrence of lactylation modifications. The general control non-repressed 5 protein (GCN5), produced by the mammalian gene KAT2A, is the pioneer histone acetyltransferase found to associate histone acetylation with transcriptional activation (34). According to a study, as an upstream regulatory component, GCN5 controls the histone lactylation in monocytes and the downstream expression of reparative genes after myocardial infection (35). Upregulated of GCN5 is strongly associated with malignant features of HCC as well as a potential molecular target for HCC diagnosis (36). ZEB1, a constituent of the ZEB transcription factor family, is recognized for its role as a transcriptional regulator, which can promote tumorigenesis and metastasis in HCC (37). by regulating the expression of PHGDH. Reports suggest that ZEB1 regulates the expression of several key glycolytic enzymes through transcription, for example, GLUT ZEB1 activation promotes GLUT3 expression, enhancing glycolysis in ovarian cancer (OvCa) and facilitating tumor progression and metastasis (38). In this process, lactate accumulation mediated histone lactation lactylation enhances chromatin accessibility and cellular plasticity, thereby promoting neuroendocrine prostate cancer development (39).
This study developed a lactylation-related signature and demonstrated its effective diagnostic and prognostic capabilities. Additionally, the lactylation score can serve as a tool for evaluating responses to ICI therapy and drug sensitivity. Our novel lactylation-related model may potentially be applied for targeted HCC therapy in the future. However, there are some limitations to this study. First, this study is based on public databases (GEO and TCGA) and lacks external validation with clinical samples. In the future, we aim to collect sufficient clinical samples for model validation to ascertain the representativeness and clinical value of the diagnostic model. Second, using a limited set of samples for signature development may lead to models that perform well on TCGA dataset but poorly on GEO datasets. This often arises from using too many features without sufficient validation samples to ensure robustness. We plan to conduct model performance analysis, subgroup analysis, and statistical significance analysis through an independent validation cohort to ensure the clinical application potential of the diagnostic model. In order to enhance the robustness of the model, this study will be validated in multiple external cohorts. Furthermore, the detailed mechanisms of lactylation-related genes (BSG, KAT2A, and ZEB1) in the occurrence and development of HCC have not been fully elucidated. Future research needs to explore the biological functions and pathogenic mechanisms of these genes in HCC at the cellular and animal levels.
Conclusions
In conclusion, this research developed a lactylation-related signature and demonstrated its effective diagnostic and prognostic capabilities. Furthermore, the lactylation score could serve as a tool for evaluating responses to ICI treatment and drug sensitivity. Our novel lactylation-related model may potentially be applied for targeted HCC therapy in the future.
Acknowledgments
None.
Footnote
Reporting Checklist: The authors have completed the TRIPOD reporting checklist. Available at https://tcr.amegroups.com/article/view/10.21037/tcr-24-1023/rc
Peer Review File: Available at https://tcr.amegroups.com/article/view/10.21037/tcr-24-1023/prf
Funding: This paper was supported by
Conflicts of Interest: All authors have completed the ICMJE uniform disclosure form (available at https://tcr.amegroups.com/article/view/10.21037/tcr-24-1023/coif). The authors have no conflicts of interest to declare.
Ethical Statement: The authors are accountable for all aspects of the work in ensuring that questions related to the accuracy or integrity of any part of the work are appropriately investigated and resolved. The study was conducted in accordance with the Declaration of Helsinki (as revised in 2013). The Institutional Review Committee of the Human Research Ethics Committee of the Eighth Affiliated Hospital, Sun Yat-sen University approved the study. The IRB approval number of this study is ZB-KYIRB-AF/SC-08/01.0.
Open Access Statement: This is an Open Access article distributed in accordance with the Creative Commons Attribution-NonCommercial-NoDerivs 4.0 International License (CC BY-NC-ND 4.0), which permits the non-commercial replication and distribution of the article with the strict proviso that no changes or edits are made and the original work is properly cited (including links to both the formal publication through the relevant DOI and the license). See: https://creativecommons.org/licenses/by-nc-nd/4.0/.
References
- Luo X, He X, Zhang X, et al. Hepatocellular carcinoma: signaling pathways, targeted therapy, and immunotherapy. MedComm (2020) 2024;5:e474.
- Mo Y, Zou Z, Chen E. Targeting ferroptosis in hepatocellular carcinoma. Hepatol Int 2024;18:32-49. [Crossref] [PubMed]
- Xia T, Zhao B, Li B, et al. MRI-Based Radiomics and Deep Learning in Biological Characteristics and Prognosis of Hepatocellular Carcinoma: Opportunities and Challenges. J Magn Reson Imaging 2024;59:767-83. [Crossref] [PubMed]
- Chan YT, Zhang C, Wu J, et al. Biomarkers for diagnosis and therapeutic options in hepatocellular carcinoma. Mol Cancer 2024;23:189. [Crossref] [PubMed]
- Wang S, Wang X, Yin X, et al. Differentiating HCC from ICC and prediction of ICC grade based on MRI deep-radiomics: Using lesions and their extended regions. Phys Med 2024;120:103322. [Crossref] [PubMed]
- Colapietro F, Maisonneuve P, Lytvyak E, et al. Incidence and predictors of hepatocellular carcinoma in patients with autoimmune hepatitis. J Hepatol 2024;80:53-61. [Crossref] [PubMed]
- Urquijo-Ponce JJ, Alventosa-Mateu C, Latorre-Sánchez M, et al. Present and future of new systemic therapies for early and intermediate stages of hepatocellular carcinoma. World J Gastroenterol 2024;30:2512-22. [Crossref] [PubMed]
- Jiang H, Zuo M, Li W, et al. Multimodal imaging-based prediction of recurrence for unresectable HCC after downstage and resection-cohort study. Int J Surg 2024;110:5672-84. [Crossref] [PubMed]
- Wang W, Zhou Y, Li W, et al. Claudins and hepatocellular carcinoma. Biomed Pharmacother 2024;171:116109. [Crossref] [PubMed]
- Wang F, Numata K, Funaoka A, et al. Construction of a nomogram combining CEUS and MRI imaging for preoperative diagnosis of microvascular invasion in hepatocellular carcinoma. Eur J Radiol Open 2024;13:100587. [Crossref] [PubMed]
- Wang Q, Liu J, Chen Z, et al. Targeting metabolic reprogramming in hepatocellular carcinoma to overcome therapeutic resistance: A comprehensive review. Biomed Pharmacother 2024;170:116021. [Crossref] [PubMed]
- Lin J, Rao D, Zhang M, et al. Metabolic reprogramming in the tumor microenvironment of liver cancer. J Hematol Oncol 2024;17:6. [Crossref] [PubMed]
- Lao Y, Cui X, Xu Z, et al. Glutaryl-CoA dehydrogenase suppresses tumor progression and shapes an anti-tumor microenvironment in hepatocellular carcinoma. J Hepatol 2024;81:847-61. [Crossref] [PubMed]
- Li LN, Li WW, Xiao LS, et al. Lactylation signature identifies liver fibrosis phenotypes and traces fibrotic progression to hepatocellular carcinoma. Front Immunol 2024;15:1433393. [Crossref] [PubMed]
- Song F, Hou C, Huang Y, et al. Lactylome analyses suggest systematic lysine-lactylated substrates in oral squamous cell carcinoma under normoxia and hypoxia. Cell Signal 2024;120:111228. [Crossref] [PubMed]
- Yu X, Yang J, Xu J, et al. Histone lactylation: from tumor lactate metabolism to epigenetic regulation. Int J Biol Sci 2024;20:1833-54. [Crossref] [PubMed]
- Wang J, Wang Z, Wang Q, et al. Ubiquitous protein lactylation in health and diseases. Cell Mol Biol Lett 2024;29:23. [Crossref] [PubMed]
- Hu Y, He Z, Li Z, et al. Lactylation: the novel histone modification influence on gene expression, protein function, and disease. Clin Epigenetics 2024;16:72. [Crossref] [PubMed]
- Dai E, Wang W, Li Y, et al. Lactate and lactylation: Behind the development of tumors. Cancer Lett 2024;591:216896. [Crossref] [PubMed]
- Li W, Zhou C, Yu L, et al. Tumor-derived lactate promotes resistance to bevacizumab treatment by facilitating autophagy enhancer protein RUBCNL expression through histone H3 lysine 18 lactylation (H3K18la) in colorectal cancer. Autophagy 2024;20:114-30. [Crossref] [PubMed]
- Qin Y, Huo M, Liu X, et al. Biomarkers and computational models for predicting efficacy to tumor ICI immunotherapy. Front Immunol 2024;15:1368749. [Crossref] [PubMed]
- Yu B, Ma W. Biomarker discovery in hepatocellular carcinoma (HCC) for personalized treatment and enhanced prognosis. Cytokine Growth Factor Rev 2024;79:29-38. [Crossref] [PubMed]
- Chen JJ, Jin ZC, Zhong BY, et al. Locoregional therapies for hepatocellular carcinoma: The current status and future perspectives. United European Gastroenterol J 2024;12:226-39. [Crossref] [PubMed]
- Zang Y, Wang A, Zhang J, et al. Hypoxia promotes histone H3K9 lactylation to enhance LAMC2 transcription in esophageal squamous cell carcinoma. iScience 2024;27:110188. [Crossref] [PubMed]
- Zhang Y, Jiang H, Dong M, et al. Macrophage MCT4 inhibition activates reparative genes and protects from atherosclerosis by histone H3 lysine 18 lactylation. Cell Rep 2024;43:114180. [Crossref] [PubMed]
- Zhang C, Zhou L, Zhang M, et al. H3K18 Lactylation Potentiates Immune Escape of Non-Small Cell Lung Cancer. Cancer Res 2024;84:3589-601. [Crossref] [PubMed]
- De Leo A, Ugolini A, Yu X, et al. Glucose-driven histone lactylation promotes the immunosuppressive activity of monocyte-derived macrophages in glioblastoma. Immunity 2024;57:1105-1123.e8. [Crossref] [PubMed]
- Zhao Y, Jiang J, Zhou P, et al. H3K18 lactylation-mediated VCAM1 expression promotes gastric cancer progression and metastasis via AKT-mTOR-CXCL1 axis. Biochem Pharmacol 2024;222:116120. [Crossref] [PubMed]
- Wei S, Zhang J, Zhao R, et al. Histone lactylation promotes malignant progression by facilitating USP39 expression to target PI3K/AKT/HIF-1α signal pathway in endometrial carcinoma. Cell Death Discov 2024;10:121. [Crossref] [PubMed]
- Li XM, Yang Y, Jiang FQ, et al. Histone lactylation inhibits RARγ expression in macrophages to promote colorectal tumorigenesis through activation of TRAF6-IL-6-STAT3 signaling. Cell Rep 2024;43:113688. [Crossref] [PubMed]
- Sugiyama K, Shimano H, Takahashi M, et al. The Use of Carboxyfluorescein Reveals the Transport Function of MCT6/SLC16A5 Associated with CD147 as a Chloride-Sensitive Organic Anion Transporter in Mammalian Cells. J Pharm Sci 2024;113:1113-20. [Crossref] [PubMed]
- Afonso J, Barbosa A, Aguiar Pastrez PR, et al. Clinical and Prognostic Impact of the Warburg Effect in Esophageal Carcinoma: Monocarboxylate Transporters as Candidates for Therapeutic Targeting. Pathobiology 2023;90:251-69. [Crossref] [PubMed]
- Kanekura T. CD147/Basigin Is Involved in the Development of Malignant Tumors and T-Cell-Mediated Immunological Disorders via Regulation of Glycolysis. Int J Mol Sci 2023;24:17344. [Crossref] [PubMed]
- Han Y, Zhao H, Li G, et al. GCN5 mediates DNA-PKcs crotonylation for DNA double-strand break repair and determining cancer radiosensitivity. Br J Cancer 2024;130:1621-34. [Crossref] [PubMed]
- Wang N, Wang W, Wang X, et al. Histone Lactylation Boosts Reparative Gene Activation Post-Myocardial Infarction. Circ Res 2022;131:893-908. [Crossref] [PubMed]
- Zhu Y, Lu F. Astragaloside IV inhibits cell viability and glycolysis of hepatocellular carcinoma by regulating KAT2A-mediated succinylation of PGAM1. BMC Cancer 2024;24:682. [Crossref] [PubMed]
- Liang J, Yao N, Deng B, et al. GINS1 promotes ZEB1-mediated epithelial-mesenchymal transition and tumor metastasis via β-catenin signaling in hepatocellular carcinoma. J Cell Physiol 2024;239:e31237. [Crossref] [PubMed]
- Lin F, Ma L, Yu S, et al. GLUT3 transcriptional activation by ZEB1 fuels the Warburg effect and promotes ovarian cancer progression. Biochim Biophys Acta Mol Cell Res 2024;1871:119715. [Crossref] [PubMed]
- Wang D, Du G, Chen X, et al. Zeb1-controlled metabolic plasticity enables remodeling of chromatin accessibility in the development of neuroendocrine prostate cancer. Cell Death Differ 2024;31:779-91. [Crossref] [PubMed]