Development and validation of a prediction model based on two-dimensional dose distribution maps fused with computed tomography images for noninvasive prediction of radiochemotherapy resistance in non-small cell lung cancer
Highlight box
Key findings
• This study found that dosiomics features extracted from two-dimensional (2D) radiation dose distribution maps can be used as a novel imaging marker to predict radiochemotherapy resistance in non-small cell lung cancer (NSCLC).
• The 2D dosiomics model can better predict radiochemotherapy resistance than clinical and traditional radiomics models.
• Combining radiomics, dosiomics, and clinical parameters can improve radiochemotherapy efficacy and prognosis prediction in NSCLC.
What is known and what is new?
• Patients with NSCLC are prone to local treatment failure after radiochemotherapy, but the mechanism of action is complex, and existing models have limitations in their predictive ability.
• In this study, a new prediction tool is introduced to integrate radiomics, dosiomics, and clinical parameters to establish a prediction model for improving the prediction accuracy of resistance to and prognosis of radiochemotherapy.
What is the implication, and what should change now?
• This study combines radiomics, 2D dosiomics, and clinical indicators to provide a new method for predicting radiochemotherapy resistance in NSCLC and stratifying the prognostic risk in patients with NSCLC, guiding clinicians in early detection and treatment regimen adjustment, which is, in turn, expected to improve patients’ outcomes. This might pave the way for more tailored NSCLC treatment.
Introduction
Background
Despite recent improvements in mortality rates, lung cancer is still a leading cause of cancer-related fatalities globally (1). Non-small cell lung cancer (NSCLC) accounts for roughly 85% of all lung cancers (2). Most patients with NSCLC are diagnosed in advanced stages, making surgery unsuitable due to the spread of cancer cells (3). Radiochemotherapy stands as the standard treatment for locally advanced NSCLC and plays a crucial role in the comprehensive treatment of lung cancer (4). Although advances in radiotherapy (RT) techniques and refinement of treatment strategies have improved lung cancer prognosis, the prognosis varies even at the same disease stage owing to tumor heterogeneity (5).
Rationale and knowledge gap
Most NSCLC cases demonstrate locoregional treatment failure after radiochemotherapy (6), underscoring the critical need for early identification of patients prone to radiochemotherapy resistance. Initial treatment response can predict overall survival (OS), and early acquisition of treatment responses predicts favorable outcomes (7). Identifying patients at risk for progression and making timely treatment adjustments may reduce disease progression and associated mortality.
The issue of drug resistance is particularly prominent in the treatment of NSCLC. Currently, some biomarkers have been used to predict drug resistance in NSCLC treatment, such as circulating tumor DNA (ctDNA), tumor microenvironment (especially hypoxic environment), and abnormal expression of microRNAs (8-10). However, their clinical application is still not ideal. On the other hand, NSCLC exhibits high heterogeneity, and the genetic background, pathological type, and treatment history of different patients may lead to varying expressions of the same biomarkers in different patients (11). Moreover, most predictive models rely on invasive methods such as tissue biopsies or liquid biopsies, which may pose additional risks and discomfort to patients (12). Therefore, finding less invasive predictive methods is crucial. The development of radiomics and machine learning technologies has provided a non-invasive, efficient, and cost-effective new method for predicting tumor efficacy. Conventional radiomics can predict tumor response and prognosis using information from medical image data, including models from [18F]-fluorodeoxyglucose positron emission tomography (PET)/computed tomography (CT) (13-17). Radiomics development has improved the efficiency of assessing prognosis and treatment response; however, the aforementioned radiomics studies have focused on exploring the relationship between imaging data and clinical outcomes, ignoring the potential impact of radiation dose distribution.
The local control rate of radiochemotherapy is significantly correlated with radiation dose (18), and dose distribution features affect RT outcomes. Dosiomics features from dose distribution images are mostly used to predict radiation pneumonitis, expanding radiomics development to predict RT toxicity (19,20). Dosiomics models are better than radiomics models, and their combination shows improved predictive performance (21,22). CT imaging can vividly show the anatomical structure of the human body, but it lacks contrast in soft tissues. The dose distribution map can depict the spread of radiation throughout the biological body; however, it lacks spatial localisation information. In RT, by fusing the dose distribution map with the CT image, we can compensate for the shortcomings of both, allowing us to more comprehensively understand the response of the tumour and surrounding tissues to RT, thus enabling more precise localization of lesions and better evaluation of treatment effects. Therefore, this combination may improve the prediction of radiochemotherapy response. The potential dosomics predictors of RT response are limited to dosimetry parameters (23-25); however, simple dosimetry parameters (dose-volume histograms and biologically effective doses) are limited in predicting treatment response. Therefore, new predictive biomarkers to accurately identify radiochemotherapy resistant cancer are urgently needed.
Objective
This study introduced an imaging marker to predict RT response in NSCLC. A two-dimensional (2D) dosiomics model of different regions was constructed using radiation dose distribution images from patients at three independent centers. The predictive performance of this model in NSCLC radiochemotherapy resistance was compared to conventional radiomics. The value of predicting radiochemotherapy resistance was explored by combining radiomics, 2D dosiomics, and clinical models. Risk stratification of patients with NSCLC and assessment of prognostic value based on the combined model will facilitate individualized treatment strategies. We present this article in accordance with the TRIPOD reporting checklist (available at https://tcr.amegroups.com/article/view/10.21037/tcr-24-1897/rc).
Methods
Patients enrollment
Figure 1 and Figure 2 show the criteria for patient enrollment and the flow of the radiomic analysis. Overall, 783 patients with NSCLC receiving radiochemotherapy or RT at three medical institutions (The Second Affiliated Hospital of Guizhou Medical University; Guiyang Public Health Clinical Center; Qiandongnan Prefecture People’s Hospital) were initially screened. The inclusion criteria were histologically confirmed NSCLC, pretreatment planning CT scans, Karnofsky Performance Scale (KPS) score ≥70, and standard radiochemotherapy [including concurrent chemoradiotherapy (CCRT), sequential chemoradiotherapy, and RT]. The exclusion criteria were radical surgical treatment before treatment, incomplete clinical information combined with other malignant tumors, RT of non-lung primary lesions, and poor image quality. Eventually, 220 patients met the inclusion criteria, including 148 from The Second Affiliated Hospital of Guizhou Medical University (April 2018–May 2023), 42 from Guiyang Public Health Clinical Center (October 2017–December 2022), and 30 from Qiandongnan Prefecture People’s Hospital (February 2017–December 2022). All data from the multicenter cohort were integrated and randomly separated into training (n=154) and validation (n=66) cohorts.
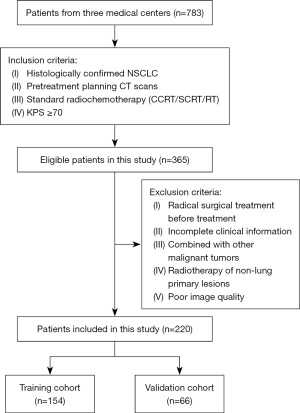
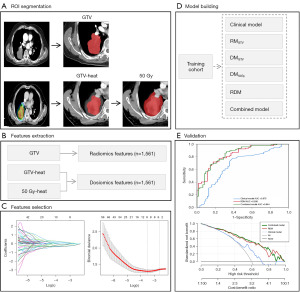
Research objectives and definitions
The main objective of the study was to assess the effectiveness of local radiochemotherapy. Efficacy was evaluated by an experienced radiation oncologist using the single-diameter measurement method in the response evaluation criteria in solid tumors (RECIST) (Version 1.1) guidelines, comparing CT images before and after radiochemotherapy. Patients with complete and partial remission were categorized into the radiochemotherapy-sensitive group, while those with disease stability and progression were categorized into the radiochemotherapy-resistant group. The progression-free survival (PFS) and OS were the study endpoints. PFS refers to the time from initial therapy to disease progression, death, or last follow-up, while OS is the time from first treatment to death or final follow-up. This study was conducted in accordance with the Declaration of Helsinki (as revised in 2013). The study was approved by the Institutional Review Board of The Second Affiliated Hospital of Guizhou Medical University (2020-LS-03), and prior notice was given to Qiandongnan Prefecture People’s Hospital and Guiyang Public Health Clinical Center, with consent obtained from both parties. Due to the retrospective nature of the study, written informed consent was not required.
Collection of clinical data
Baseline clinical information was obtained by reviewing the case system, including sex, age, nationality (minority or non-minority nationality), KPS score, carcinoembryonic antigen (CEA) level, hemoglobin (Hb) level, smoking status, diabetes status, hepatitis B virus (HBV) status, Child-Pugh score, T stage, N stage, M stage, tumor-node-metastasis (TNM) stage, histology (squamous carcinoma, adenocarcinoma, other), metastatic status (oligometastasis, polymetastasis), RT technique [three-dimensional conformal radiation therapy (3D-CRT), intensity-modulated radiation therapy (IMRT), image-guided radiotherapy (IGRT)], treatment mode [RT, short-course radiotherapy (SCRT), CCRT], and prescribed dose. CCRT included chemotherapy and RT simultaneously; SCRT was defined as chemotherapy followed by RT, while RT was radiation therapy only.
Estimation of sample size
In the field of radiomics, the robustness of predictive classifier models depends on the availability of sufficient data. Research data suggests that for machine learning algorithms, the sample size should be no less than 80 for the root mean square error to be less than 0.01 and no less than 30 for the research data to be sufficient to support any statistical model. Empirical data suggests that for binary classifier models, the recommended feature selection is about one-tenth of the sample size (26). Based on the above research basis, the number of features and sample size of this study basically meet the requirements, and the overfitting problem caused by too many features or insufficient sample size can be avoided.
CT and dose distribution image acquisition
All patients underwent large-aperture CT (Philips Electronics, GE Medical Systems, Eindhoven, the Netherlands) within one week before RT to obtain the planned CT images. The scanning range was from the submandibular margin to the subhepatic margin. During scanning, a high-pressure injector injects 2.5–3.0 mL/s of contrast agent (iohexol or iopamidol) into the elbow vein. Scanning was delayed by 25 s after injection completion to obtain arterial-phase CT, which was transmitted to the Varian Eclipse/Elekta planning system to produce an RT plan. The CT scanning systems and parameters of the three medical centers are listed in Table S1.
Selected 50 Gy dose distribution images of the largest lesion were downloaded in PNG format from the RT planning system and converted to DICOM format. These planning CT images were used to investigate the differences between the original 2D CT and radiation dose distribution images in predicting radiochemotherapy resistance.
Region of interest (ROI) segmentation
The ROIs of all images were segmented from the 2D planned CT images of the largest cross-section and radiation dose distribution images. The three ROIs were the gross tumor volume (GTV), GTV heat, and 50 Gy heat. The GTV was defined as the tumor region on the 2D planned CT images, GTV heat was defined as the tumor region covered by the 2D radiation dose distribution images, and 50 Gy heat was defined as the region covered by the 50 Gy absolute dose on the 2D radiation dose distribution images. All ROIs were manually drawn by an experienced radiologist using ITK-SNAP version 4.0.1 in the mediastinal window along the tumor edges of the 2D planned CT image of the largest lesion, the tumor edges of the 2D radiation dose distribution image, and the edges of the 50 Gy absolute dose area of the 2D radiation dose distribution image, respectively, avoiding the pleural wall, bronchi, and large blood vessels. Finally, 2D tumor segmentation of the three regions was generated, and manual correction was performed by radiation oncology professionals with substantial clinical and imaging experience.
Extraction and selection of features
A total of 1,561 features, including 14 shape features, 306 first-order statistical features, and 1,241 texture features, were extracted from the GTV, GTV-heat, and 50 Gy-heat using pyradiomics. Before feature selection, maximal and minimal normalization was applied to all radiomics and 2D dosiomics features to eliminate variances between features. The t-test or U test was used to exclude features not related to radiochemotherapy resistance. Finally, the least absolute shrinkage and selection operator (LASSO) regression method was employed to select radiomics and 2D dosiomics features that could predict optimal efficacy.
Model construction and validation
We developed six predictive models [clinical, GTV radiomics model (RMGTV), GTV dosiomics model (DMGTV), 50 Gy dosiomics model (DM50Gy), radiomics-dosiomics model (RDM), and combined model] using binary logistic regression based on clinical and selected features of three independent regions. The predictive performance of these models was assessed using receiver operating characteristic (ROC) curve analysis, with accuracy, sensitivity, and specificity computed. In addition, we tested the model’s performance with a validation cohort. Decision curve analysis (DCA) was utilized to determine the clinical utility of various thresholds in patients with NSCLC.
Statistical analysis
The statistical analysis was performed using IBM SPSS (version 26.0), Python software (version 3.9.13), and the R language (version 4.3.1). The comparison of numerical variables was performed with a t-test or non-parametric test. Statistical analysis of categorical variables was carried out using Chi-squared tests or Fisher’s exact tests. Clinical variables linked with radiochemotherapy resistance were identified using univariate and multivariate analyses. Radiomic and 2D dosiomics characteristics were screened using LASSO regression in R, followed by the creation of a logistic regression model in SPSS, the display of ROC curves, and the computation of area under the curve (AUC) values (in general, the larger the AUC value, the better the categorization and the more accurate the prediction of efficacy). The clinical applicability of the model was evaluated using DCA. The Kaplan-Meier method was used to compare survival estimates. Statistical significance was set at P<0.05 (two-sided).
Results
Patient features
The clinical features and distributions of the 220 individuals enrolled in this study are reported in Table 1. In the training (n=154) and validation (n=66) groups, 88 (57.1%) and 36 (54.5%) patients were minority nationality, respectively; 91 (59.1%) and 38 (57.6%) had a pathological classification of squamous carcinoma; 80 (51.9%) and 35 (53.0%) had stage III disease; and 64 (41.6%) and 26 (39.4%) had stage IV disease. Among these cases, 65 (42.2%) and 25 (37.9%) were metastatic at baseline, and 44 (67.7%) and 18 (72.0%) were oligometastatic. In addition, 100 (65.0%) and 40 (60.6%) patients received CCRT, and 98 (63.6%) and 43 (65.2%) patients in both groups were treated with IGRT. The training and validation cohorts showed no significant difference in clinical features such as sex, age, nationality, KPS score, CEA, Hb, smoking, diabetes, HBV, Child-Pugh score, T stage, N stage, M stage, TNM stage, histology, metastatic status, RT technique, treatment mode, and prescribed dose (P>0.05).
Table 1
Variables | Categories | Training | Validation | P value |
---|---|---|---|---|
Age (years) | – | 60.3±9.4 | 61.2±8.8 | 0.50 |
Sex | Male | 125 (81.2) | 55 (83.3) | 0.70 |
Female | 29 (18.8) | 11 (16.7) | ||
Nationality | Non-minority | 66 (42.9) | 30 (45.5) | 0.72 |
Minority | 88 (57.1) | 36 (54.5) | ||
Child-Pugh | A | 150 (97.4) | 62 (93.9) | 0.25 |
B | 4 (2.6) | 4 (6.1) | ||
Smoking | Yes | 94 (61.0) | 45 (68.2) | 0.31 |
No | 60 (39.0) | 21 (31.8) | ||
Diabetes | Yes | 14 (9.1) | 9 (13.6) | 0.31 |
No | 140 (90.9) | 57 (86.4) | ||
HBV | Yes | 10 (6.5) | 3 (4.5) | 0.76 |
No | 144 (93.5) | 63 (95.5) | ||
Tuberculosis | Yes | 16 (10.4) | 13 (19.7) | 0.06 |
No | 138 (89.6) | 53 (80.3) | ||
T stage | T1–2 | 45 (29.2) | 17 (25.8) | 0.60 |
T3–4 | 109 (70.8) | 49 (74.2) | ||
N stage | N0–1 | 39 (25.3) | 14 (21.2) | 0.51 |
N2–3 | 115 (74.7) | 52 (78.8) | ||
M stage | M0 | 90 (58.4) | 40 (60.6) | 0.77 |
M1 | 64 (41.6) | 26 (39.4) | ||
TNM stage | I–II | 10 (4.5) | 5 (7.6) | 0.93 |
III | 80 (51.9) | 35 (53.0) | ||
IV | 64 (41.6) | 26 (39.4) | ||
Metastatic status | Oligometastasis | 44 (67.7) | 18 (72.0) | 0.69 |
Polymetastasis | 21 (32.3) | 7 (28.0) | ||
Histology | Squamous carcinoma | 91 (59.1) | 38 (57.6) | 0.96 |
Adenocarcinoma | 57 (37.0) | 25 (37.9) | ||
Other | 6 (3.9) | 3 (4.5) | ||
RT technique | IMRT | 46 (29.9) | 16 (24.2) | 0.46 |
IGRT | 98 (63.6) | 43 (65.2) | ||
3D-CRT | 10 (6.5) | 7 (10.6) | ||
Treatment mode | RT | 33 (21.4) | 22 (33.3) | 0.08 |
SCRT | 21 (13.6) | 4 (6.1) | ||
CCRT | 100 (65.0) | 40 (60.6) | ||
KPS | – | 80.0 (80.0, 90.0) | 80.0 (80.0, 90.0) | 0.38 |
CEA (ng/mL) | – | 3.1 (1.8, 8.1) | 3.7 (1.6, 19.8) | 0.26 |
Hb (g/L) | – | 121.8±17.3 | 122.8±17.8 | 0.70 |
Prescribed dose (Gy) | – | 63.0 (60.0, 66.0) | 62.0 (60.0, 66.0) | 0.60 |
Continuous variables that follow a normal distribution are represented by the mean ± standard deviation, while numerical variables that do not follow a normal distribution are represented by the median (interquartile range). Categorical variables are represented by frequency (percentage). 3D-CRT, three-dimensional conformal radiotherapy; CCRT, concurrent chemoradiotherapy; CEA, carcinoembryonic antigen; Hb, hemoglobin; HBV, hepatitis B virus; IGRT, image-guided radiotherapy; IMRT, intensity-modulated radiation therapy; KPS, Karnofsky Performance Status; RT, radiotherapy; SCRT, sequential chemoradiotherapy; TNM, tumor-node-metastasis.
Feature selection and associated analysis of clinical factors
There were 1,561 features extracted from the three ROIs. First, to exclude features not significantly related to radiochemotherapy resistance, we employed the t-test or Mann-Whitney U test to assess normally and non-normally distributed radiomics features, respectively. After excluding features with P≥0.05, 19, 19, and 181 features were included from GTV, GTV-heat, and 50 Gy-heat, respectively. Subsequently, to optimize the model, we employed LASSO regression and 10-fold cross-validation to identify critical features. Finally, feature screening resulted in the identification of eight, nine, and eight radiomics and 2D dosiomics features significantly associated with radiochemotherapy resistance (Figure 3). Tables 2-4 list the significant characteristics together with their weight coefficients. In univariate analysis, Hb level was substantially linked with radiochemotherapy resistance (P=0.03) (Table S2). Following the univariate analysis results, multivariate analysis of clinical variables with P<0.1 revealed Hb level and T stage as independent risk factors for radiochemotherapy resistance [odds ratio (OR) and 95% confidence interval (CI): 0.975 (0.956–0.996), 0.432 (0.199–0.934)].
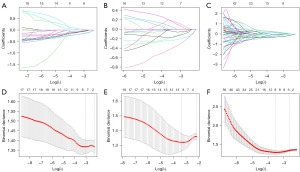
Table 2
Feature name (GTV) | Coefficient |
---|---|
[0] (Intercept) | 0.394 |
[1] exponential_glcm_Correlation | −0.0504 |
[2] lbp.3D.k_glszm_SizeZoneNonUniformity | −0.163 |
[3] square_gldm_LargeDependenceLowGrayLevelEmphasis | 0.014 |
[4] wavelet.HHH_firstorder_Range | −0.064 |
[5] wavelet.HHL_glcm_Correlation | −0.057 |
[6] wavelet.HLH_firstorder_Mean | −0.145 |
[7] wavelet.HLH_glszm_SizeZoneNonUniformityNormalized | 0.028 |
[8] wavelet.LHH_glcm_DifferenceVariance | −0.035 |
GTV, gross tumor volume.
Table 3
Feature name (GTV-heat) | Coefficient |
---|---|
[0] (Intercept) | 0.038 |
[1] lbp.3D.k_glszm_GrayLevelNonUniformity | 0.016 |
[2] lbp.3D.k_glszm_GrayLevelNonUniformityNormalized | −0.039 |
[3] logarithm_firstorder_Skewness | 0.017 |
[4] squareroot_firstorder_Kurtosis | −0.023 |
[5] wavelet.HLH_gldm_DependenceEntropy | 0.015 |
[6] wavelet.HLH_gldm_DependenceNonUniformityNormalized | −0.005 |
[7] wavelet.LHH_firstorder_Range | −0.016 |
[8] wavelet.LHH_glrlm_GrayLevelNonUniformityNormalized | 0.004 |
[9] wavelet.LHH_glrlm_GrayLevelVariance | −2.269e−16 |
GTV, gross tumor volume.
Table 4
Feature name (50 Gy-heat) | Coefficient |
---|---|
[0] (Intercept) | 0.389 |
[1] squareroot_firstorder_InterquartileRange | −0.227 |
[2] squareroot_firstorder_RobustMeanAbsoluteDeviation | −0.295 |
[3] wavelet.HHH_glszm_SmallAreaHighGrayLevelEmphasis | 0.239 |
[4] wavelet.HHH_glszm_ZonePercentage | 0.127 |
[5] wavelet.HHL_gldm_LargeDependenceHighGrayLevelEmphasis | −0.08 |
[6] wavelet.HLH_firstorder_90Percentile | 0.197 |
[7] wavelet.HLH_glrlm_ShortRunEmphasis | −0.19 |
[8] wavelet.LLL_glcm_InverseVariance | −0.317 |
Logistic regression analysis of clinical risk factors affecting radiochemotherapy resistance
Using the screened features, radiomics and 2D dosiomics models (RMGTV, DMGTV, and DM50Gy) were constructed to explore the predictive value of 2D radiation dose distribution images on radiochemotherapy resistance in NSCLC and the differences between them and conventional CT images. In both cohorts, the DM50Gy AUC [0.764 (95% CI: 0.687–0.841) and 0.729 (95% CI: 0.568–0.889)] were better than those of DMGTV (AUC 0.746 and 0.683) and RMGTV (AUC 0.694 and 0.613). Therefore, the 2D dosiomics features extracted from radiation dose distribution images displayed promising predictive capabilities for radiochemotherapy efficacy, outperforming the 2D radiomics model based on planned CT images. Particularly, the 2D dosiomics model extracted from the 50 Gy absolute dose distribution images demonstrated the strongest performance. In addition, the RDM combining radiomics and each 2D dosiomics feature showed even better predictive performance in both cohorts [AUC 0.836 (95% CI: 0.773–0.899) and 0.748 (95% CI: 0.617–0.879), respectively] (Table S3).
Construction and evaluation of the combined model
Based on the multivariate analysis results, a combined model was established using binary logistic regression. The combined model’s AUCs were 0.844 (95% CI: 0.781–0.907) in the training cohort and 0.753 (95% CI: 0.618–0.887) in the validation cohorts, indicating that the combined model integrating Hb and T stage had high diagnostic performance in predicting radiochemotherapy resistance. Figure 4 shows the ROC curves for all models. Figure 5 shows the DCA for the clinical, RDM, and combination models. The combined model demonstrated more accurate and reliable efficacy prediction in predicting radiochemotherapy resistance and slightly outperformed the RDM in most threshold ranges.
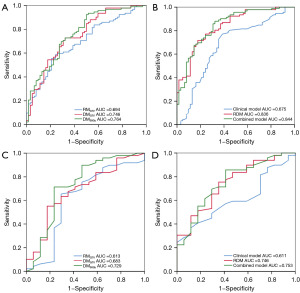
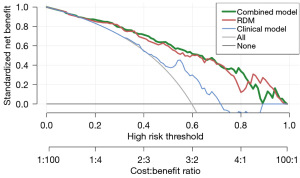
Survival risk stratification
The cut-off value (0.498) was calculated using the ROC curves of the combined model, which was used to group patients with NSCLC as low-risk and high-risk, allowing for the subsequent comparison in their survival prognosis. The log-rank test revealed significant differences in PFS and OS between the two groups, with high-risk patients having worse PFS and OS (Figure 6A,6B). Testing in the validation cohort using the same cutoff values yielded similar results (Figure 6C,6D). Further subgroup analyses revealed a worse prognosis in the radiochemotherapy-resistant group than the radiochemotherapy-sensitive group (Figure S1).
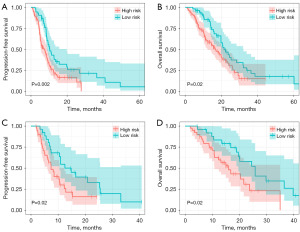
Discussion
Key findings
This study extracted dosiomics features from 2D dose distribution images, which can reflect the changes after radiochemotherapy, and provide new ideas for local control and prognostic evaluation of NSCLC after radiochemotherapy. Based on the pre-treatment CT image data and 50 Gy dose distribution image, we selected 2D data of the largest lesion and extracted the radiomics features and dosiomics features of different regions. The results showed that DM50Gy was superior to DMGTV, conventional radiology, Hb level, and T stage in predicting radiochemotherapy resistance. The combination of radiomics, dosiomics, and clinical parameters significantly improved the accuracy of prediction. Furthermore, we found that the cut-off values of the combined model successfully achieved risk stratification for patients with NSCLC, with worse PFS and OS for patients at a higher risk (P<0.05).
Strengths and limitations
This is the first research to integrate radiation dose distribution mapping with CT imaging, resulting in a novel method for predicting radiochemotherapy resistance in NSCLC. By merging multi-omics data to create a combined model, risk classification of NSCLC radiochemotherapy patients may be achieved, allowing for early detection of high-risk groups and prompt treatment plan adjustments. But our study had some limitations. First, due to its retrospective nature, it lacked an external test cohort; hence, more data are needed for validation to avoid model overfitting. Second, differences in image acquisition and quality across centers may affect the applicability of the model. Third, because of multicenter differences, we were unable to investigate the potential impact of radiation toxicity and normal organ radiation dose on radiochemotherapy efficacy and prognosis.
Comparison with similar research
Radiochemotherapy resistance is a key factor in the development of NSCLC, but there is no effective intervention. Therefore, early diagnosis and timely adjustment of the treatment regimen for patients with radiochemotherapy resistant NSCLC are urgent problems to be solved in clinical practice. Existing RECIST efficacy evaluation criteria are inadequate in the assessment of early response in tumors (27), and dynamic changes in the tumor diameter can be detected only when macroscopic changes in tumor volume occur. In clinical practice, it is highly inaccurate to rely solely on the change in the maximum diameter of the tumor to reflect the early response of the tumor. Therefore, the key to promoting individualized treatment finding is in finding new valuable predictors.
Radiomics can capture small changes in tumor tissue during RT (28) and is important for evaluating patient response and prognosis (29,30). Based on the radiomics method, the 3D dose distribution generated by the dose calculation method can be used to extract the dosiomics characteristics, which can effectively predict the therapeutic effect of RT; this will greatly improve the predictive power of RT response (20,31). A recent meta-analysis delved into the relationship between radiomics and dosiomics data. The study found that the combination of the two has a synergistic effect, validating the effectiveness of integrating radiomics with dosiomics, marking a significant advancement in the field of radiation therapy (32). However, current research on dosiomics primarily relies on Anisotropic Analytical Algorithm (AAA) or Acuros XB (AXB) algorithms to describe the radiation dose distribution characteristics in RT plans. There are differences in the dose distributions produced by the AXB and AAA algorithms, with AXB being more accurate than AAA (33). Furthermore, the study indicated that changes in dose calculation grids, types, and versions can alter dosiomics features, and the effect of dose calculation algorithms on dose distributions may be more significant for tumor sites with greater tissue inhomogeneity, such as the lungs (34). To explore and solve this series of clinical problems, the real 2D dose distribution map of multicenter RT planning was used to extract the dosiomics characteristics of different regions for the first time, to explore the relationship between radiochemotherapy resistances. We constructed the models of DMGTV and DM50Gy radiochemotherapy resistance by extracting dose-group characteristics from GTV and 50 Gy radiation dose region. We found that DM50Gy outperformed DMGTV and the traditional radiomics model (RMGTV) in predicting radiochemotherapy resistance. This may be because the effective dosiomics features extracted from the 50 Gy dose area can more comprehensively reflect the relationship between the tumor and the microenvironment of adjacent tissues and the response to radiochemotherapy. After all, differences in the environmental biological characteristics of adjacent tumors can be reflected by radiomics (35). Although there are no previous studies on dosiomics models to predict NSCLC radiochemotherapy resistance, dosiomics models have been used to predict RT response in other solid tumors. Buizza et al. (36) studied 57 patients with skull-base chordoma (SBC) who received carbon-ion radiotherapy (CIRT). Magnetic resonance imaging (MRI) and CT radiomics features and dosiomics features based on dose maps were found to be associated with local control rates of RT. Thus, the combination of dosiomics and radiomics may be a promising factor affecting the local control of SBC. Kawahara et al. (37) showed that combining radiomics with dosiomics can improve the accuracy of predicting response in patients with esophageal cancer who receive radical RT. Similar to the above results, the 2D dosiomics model based on the radiation dose distribution image was superior to the traditional radiomics model in performance. In addition, the combination of the 2D radiomics model with the dosiomics model and the clinical model was more effective than either model alone. We considered not only the medical imaging data but also the effect of dose distribution in the RT plan on treatment outcomes. The combination of these three methods can evaluate the response of patients with NSCLC to radiochemotherapy and their prognosis more comprehensively, thus achieving more accurate prediction, and provide a basis for timely adjustment of treatment in the clinical setting.
Explanation of findings
We chose to segment the largest lesion on 2D, mainly considering the following factors. First, there is still considerable controversy regarding the use of 2D or 3D radiomics features in radiological analyses (38-41). Second, although 3D models can provide richer information on tumor biological characteristics, their fabrication process is more complex and often difficult to implement in a clinical setting (42). In contrast, 2D imaging data are simpler and faster in radiomics analysis, and therefore are preferred for clinical applications. Multiple studies have shown that the performance of 2D radiomics is comparable to that of 3D radiomics, and that 2D radiomics performs even better in assessing the accuracy of treatment responses (43-45). In general, the prediction model based on 2D radiomics features is feasible.
Implications and actions needed
We discovered that dosiomics features extracted from 2D dose distribution maps, combined with radiomics and clinical indicators, can more accurately predict NSCLC radiochemotherapy resistance and prognosis, providing a theoretical basis for the adjustment of treatment regimens in high-risk populations of NSCLC. However, in the emerging field of 2D dosiomics, the differences among 2D, 3D, or even multiple dosiomics characteristic sections need to be explored for studying radiochemotherapy resistance.
Conclusions
The combination of dosiomics and radiomics effectively predicted radiochemotherapy resistance in NSCLC. Additionally, the combined model with clinically independent predictors further improved the predictive efficacy and effectively stratified patients’ survival status. These findings are important references for personalized medicine, helping to identify high-risk populations in a timely manner and guide towards more effective treatment strategies.
Acknowledgments
None.
Footnote
Reporting Checklist: The authors have completed the TRIPOD reporting checklist. Available at https://tcr.amegroups.com/article/view/10.21037/tcr-24-1897/rc
Data Sharing Statement: Available at https://tcr.amegroups.com/article/view/10.21037/tcr-24-1897/dss
Peer Review File: Available at https://tcr.amegroups.com/article/view/10.21037/tcr-24-1897/prf
Funding: This work was supported by
Conflicts of Interest: All authors have completed the ICMJE uniform disclosure form (available at https://tcr.amegroups.com/article/view/10.21037/tcr-24-1897/coif). The authors have no conflicts of interest to declare.
Ethical Statement: The authors are accountable for all aspects of the work in ensuring that questions related to the accuracy or integrity of any part of the work are appropriately investigated and resolved. The study was conducted in accordance with the Declaration of Helsinki (as revised in 2013). The study was approved by the Institutional Review Board of The Second Affiliated Hospital of Guizhou Medical University (2020-LS-03), and prior notice was given to Qiandongnan Prefecture People’s Hospital and Guiyang Public Health Clinical Center, with consent obtained from both parties. Due to the retrospective nature of the study, written informed consent was not required.
Open Access Statement: This is an Open Access article distributed in accordance with the Creative Commons Attribution-NonCommercial-NoDerivs 4.0 International License (CC BY-NC-ND 4.0), which permits the non-commercial replication and distribution of the article with the strict proviso that no changes or edits are made and the original work is properly cited (including links to both the formal publication through the relevant DOI and the license). See: https://creativecommons.org/licenses/by-nc-nd/4.0/.
References
- Siegel RL, Miller KD, Fuchs HE, et al. Cancer Statistics, 2021. CA Cancer J Clin 2021;71:7-33. [Crossref] [PubMed]
- Rina A, Maffeo D, Minnai F, et al. The Genetic Analysis and Clinical Therapy in Lung Cancer: Current Advances and Future Directions. Cancers (Basel) 2024;16:2882. [Crossref] [PubMed]
- Sun Q, Li W, Liu T, et al. Individualized Treatment for Advanced Non-Small Cell Lung Cancer: A Case Report and Literature Review. Front Oncol 2022;12:916681. [Crossref] [PubMed]
- Ettinger DS, Wood DE, Aisner DL, et al. NCCN Guidelines® Insights: Non-Small Cell Lung Cancer, Version 2.2023. J Natl Compr Canc Netw 2023;21:340-50. [Crossref] [PubMed]
- Kang DH, Lee J, Im S, et al. Navigating the Complexity of Resistance in Lung Cancer Therapy: Mechanisms, Organoid Models, and Strategies for Overcoming Treatment Failure. Cancers (Basel) 2024;16:3996. [Crossref] [PubMed]
- Eisenberg M, Deboever N, Antonoff MB. Salvage surgery in lung cancer following definitive therapies. J Surg Oncol 2023;127:319-28. [Crossref] [PubMed]
- Peng J, Kang S, Ning Z, et al. Residual convolutional neural network for predicting response of transarterial chemoembolization in hepatocellular carcinoma from CT imaging. Eur Radiol 2020;30:413-24. [Crossref] [PubMed]
- Desai A, Lovly CM. Strategies to overcome resistance to ALK inhibitors in non-small cell lung cancer: a narrative review. Transl Lung Cancer Res 2023;12:615-28. [Crossref] [PubMed]
- Li H, Wang J, Jin Y, et al. Hypoxia upregulates the expression of lncRNA H19 in non-small cell lung cancer cells and induces drug resistance. Transl Cancer Res 2022;11:2876-86. [Crossref] [PubMed]
- Zhang D, Yang Y, Kang Y, et al. Dysregulated expression of microRNA involved in resistance to osimertinib in EGFR mutant non-small cell lung cancer cells. J Thorac Dis 2023;15:1978-93. [Crossref] [PubMed]
- Bourreau C, Treps L, Faure S, et al. Therapeutic strategies for non-small cell lung cancer: Experimental models and emerging biomarkers to monitor drug efficacies. Pharmacol Ther 2023;242:108347. [Crossref] [PubMed]
- Tang X, Li Y, Shen LT, et al. CT Radiomics Predict EGFR-T790M Resistance Mutation in Advanced Non-Small Cell Lung Cancer Patients After Progression on First-line EGFR-TKI. Acad Radiol 2023;30:2574-87. [Crossref] [PubMed]
- Abdollahi H, Chin E, Clark H, et al. Radiomics-guided radiation therapy: opportunities and challenges. Phys Med Biol 2022; [Crossref] [PubMed]
- Zheng X, Liu K, Li C, et al. A CT-based radiomics nomogram for predicting the progression-free survival in small cell lung cancer: a multicenter cohort study. Radiol Med 2023;128:1386-97. [Crossref] [PubMed]
- He X, Li K, Wei R, et al. A multitask deep learning radiomics model for predicting the macrotrabecular-massive subtype and prognosis of hepatocellular carcinoma after hepatic arterial infusion chemotherapy. Radiol Med 2023;128:1508-20. [Crossref] [PubMed]
- Peng J, Zou D, Zhang X, et al. A novel sub-regional radiomics model to predict immunotherapy response in non-small cell lung carcinoma. J Transl Med 2024;22:87. [Crossref] [PubMed]
- Peng J, Zou D, Han L, et al. A Support Vector Machine Based on Liquid Immune Profiling Predicts Major Pathological Response to Chemotherapy Plus Anti-PD-1/PD-L1 as a Neoadjuvant Treatment for Patients With Resectable Non-Small Cell Lung Cancer. Front Immunol 2021;12:778276. [Crossref] [PubMed]
- Viani GA, Gouveia AG, Louie AV, et al. Stereotactic ablative radiotherapy for locally advanced non-small cell lung cancer: A systematic review and meta-analysis. Radiother Oncol 2024;201:110439. [Crossref] [PubMed]
- Huang Y, Feng A, Lin Y, et al. Radiation pneumonitis prediction after stereotactic body radiation therapy based on 3D dose distribution: dosiomics and/or deep learning-based radiomics features. Radiat Oncol 2022;17:188. [Crossref] [PubMed]
- Kraus KM, Oreshko M, Bernhardt D, et al. Dosiomics and radiomics to predict pneumonitis after thoracic stereotactic body radiotherapy and immune checkpoint inhibition. Front Oncol 2023;13:1124592. [Crossref] [PubMed]
- Nie T, Chen Z, Cai J, et al. Integration of dosimetric parameters, clinical factors, and radiomics to predict symptomatic radiation pneumonitis in lung cancer patients undergoing combined immunotherapy and radiotherapy. Radiother Oncol 2024;190:110047. [Crossref] [PubMed]
- Bourbonne V, Da-Ano R, Jaouen V, et al. Radiomics analysis of 3D dose distributions to predict toxicity of radiotherapy for lung cancer. Radiother Oncol 2021;155:144-50. [Crossref] [PubMed]
- Avanzo M, Gagliardi V, Stancanello J, et al. Combining computed tomography and biologically effective dose in radiomics and deep learning improves prediction of tumor response to robotic lung stereotactic body radiation therapy. Med Phys 2021;48:6257-69. [Crossref] [PubMed]
- Duan C, Chaovalitwongse WA, Bai F, et al. Sensitivity analysis of FDG PET tumor voxel cluster radiomics and dosimetry for predicting mid-chemoradiation regional response of locally advanced lung cancer. Phys Med Biol 2020;65:205007. [Crossref] [PubMed]
- Luo W, Xiu Z, Wang X, et al. A Novel Method for Evaluating Early Tumor Response Based on Daily CBCT Images for Lung SBRT. Cancers (Basel) 2023;16:20. [Crossref] [PubMed]
- Gillies RJ, Kinahan PE, Hricak H. Radiomics: Images Are More than Pictures, They Are Data. Radiology 2016;278:563-77. [Crossref] [PubMed]
- Wyatt AW, Litiere S, Bidard FC, et al. Plasma ctDNA as a Treatment Response Biomarker in Metastatic Cancers: Evaluation by the RECIST Working Group. Clin Cancer Res 2024;30:5034-41. [Crossref] [PubMed]
- Watson SS, Duc B, Kang Z, et al. Microenvironmental reorganization in brain tumors following radiotherapy and recurrence revealed by hyperplexed immunofluorescence imaging. Nat Commun 2024;15:3226. [Crossref] [PubMed]
- Lucia F, Louis T, Cousin F, et al. Multicentric development and evaluation of (18)FFDG PET/CT and CT radiomic models to predict regional and/or distant recurrence in early-stage non-small cell lung cancer treated by stereotactic body radiation therapy. Eur J Nucl Med Mol Imaging 2024;51:1097-108. [Crossref] [PubMed]
- Zhou C, Hou L, Tang X, et al. CT-based radiomics nomogram may predict who can benefit from adaptive radiotherapy in patients with local advanced-NSCLC patients. Radiother Oncol 2023;183:109637. [Crossref] [PubMed]
- Yap WK, Hsiao IT, Yap WL, et al. A Radiotherapy Dose Map-Guided Deep Learning Method for Predicting Pathological Complete Response in Esophageal Cancer Patients after Neoadjuvant Chemoradiotherapy Followed by Surgery. Biomedicines 2023;11:3072. [Crossref] [PubMed]
- Sheen H, Cho W, Kim C, et al. Radiomics-based hybrid model for predicting radiation pneumonitis: A systematic review and meta-analysis. Phys Med 2024;123:103414. [Crossref] [PubMed]
- Abdullah C, Farag H, El-Sheshtawy W, et al. Clinical impact of anisotropic analytical algorithm and Acuros XB dose calculation algorithms for intensity modulated radiation therapy in lung cancer patients. J Xray Sci Technol 2021;29:1019-31. [Crossref] [PubMed]
- Sun L, Smith W, Kirkby C. Stability of dosiomic features against variations in dose calculation: An analysis based on a cohort of prostate external beam radiotherapy patients. J Appl Clin Med Phys 2023;24:e13904. [Crossref] [PubMed]
- Perrone M, Raimondi E, Costa M, et al. Inflammatory Microenvironment in Early Non-Small Cell Lung Cancer: Exploring the Predictive Value of Radiomics. Cancers (Basel) 2022;14:3335. [Crossref] [PubMed]
- Buizza G, Paganelli C, D'Ippolito E, et al. Radiomics and Dosiomics for Predicting Local Control after Carbon-Ion Radiotherapy in Skull-Base Chordoma. Cancers (Basel) 2021;13:339. [Crossref] [PubMed]
- Kawahara D, Murakami Y, Awane S, et al. Radiomics and dosiomics for predicting complete response to definitive chemoradiotherapy patients with oesophageal squamous cell cancer using the hybrid institution model. Eur Radiol 2024;34:1200-9. [Crossref] [PubMed]
- Zhu Y, Yao W, Xu BC, et al. Predicting response to immunotherapy plus chemotherapy in patients with esophageal squamous cell carcinoma using non-invasive Radiomic biomarkers. BMC Cancer 2021;21:1167. [Crossref] [PubMed]
- Xie XJ, Liu SY, Chen JY, et al. Development of unenhanced CT-based imaging signature for BAP1 mutation status prediction in malignant pleural mesothelioma: Consideration of 2D and 3D segmentation. Lung Cancer 2021;157:30-9. [Crossref] [PubMed]
- Zhang X, Zhang G, Qiu X, et al. Radiomics under 2D regions, 3D regions, and peritumoral regions reveal tumor heterogeneity in non-small cell lung cancer: a multicenter study. Radiol Med 2023;128:1079-92. [Crossref] [PubMed]
- Wang MM, Li JQ, Dou SH, et al. Lack of incremental value of three-dimensional measurement in assessing invasiveness for lung cancer. Eur J Cardiothorac Surg 2023;64:ezad373. [Crossref] [PubMed]
- Lambin P, Rios-Velazquez E, Leijenaar R, et al. Radiomics: extracting more information from medical images using advanced feature analysis. Eur J Cancer 2012;48:441-6. [Crossref] [PubMed]
- Meng L, Dong D, Chen X, et al. 2D and 3D CT Radiomic Features Performance Comparison in Characterization of Gastric Cancer: A Multi-Center Study. IEEE J Biomed Health Inform 2021;25:755-63. [Crossref] [PubMed]
- Abdoli N, Zhang K, Gilley P, et al. Evaluating the Effectiveness of 2D and 3D CT Image Features for Predicting Tumor Response to Chemotherapy. Bioengineering (Basel) 2023;10:1334. [Crossref] [PubMed]
- He LN, Chen T, Fu S, et al. Tumor response assessment by measuring the single largest lesion per organ in advanced non-small cell lung cancer patients treated with PD-1/PD-L1 inhibitor. Ther Adv Med Oncol 2023;15:17588359231200463. [Crossref] [PubMed]