Analysis and assessment of ferroptosis-related gene signatures and prognostic risk models in skin cutaneous melanoma
Highlight box
Key findings
• We constructed a prognostic risk model and a nomogram with twelve ferroptosis-related genes (NOX4, FH, ARNTL, EGFR, GJA1, SESN2, KIF20A, TRIM21, SUV39H1, TMSB4X, USP35, CYBB). The risk model was validated using a validation group, and nine ferroptosis-related genes were validated in the Human Protein Atlas (HPA) database.
• Protein-protein interaction network, univariate Cox regression analysis, and multiple stepwise Cox regression were used to identify prognostically relevant ferroptosis-related genes. The optimal algorithm was selected to construct an artificial intelligence-derived prognostic risk model and nomogram.
• The study showed that the abnormal expression of ferroptosis-related genes significantly affects the prognosis of skin cutaneous melanoma (SKCM) patients, providing a reference for treating SKCM.
What is known and what is new?
• Increasing evidence suggests that ferroptosis is crucial in SKCM development.
• In this study, we newly identified 12 ferroptosis-related genes associated with the prognosis of SKCM, and constructed a prognostic risk model and nomogram.
What is the implication, and what should change now?
• This study successfully constructed a prognostic risk model and a nomogram of ferroptosis-related genes, which can more accurately predict SKCM patient prognosis, providing potential targets and a theoretical basis for treating SKCM.
Introduction
Skin cutaneous melanoma (SKCM) is the most severe form of skin cancer and is highly aggressive and lethal, accounting for more than 80% of all skin cancer deaths (1). The incidence of SKCM is increasing yearly, especially in the white population, and can be as high as 60/100,000 in Europe and the United States (2). The common cause is that long-term ultraviolet radiation causes gene mutations in melanocytes, and with the accumulation of gene mutations, disorders occur in cell proliferation, differentiation, apoptosis, and other functions, which ultimately lead to cancer (3). Most patients with early SKCM have a favorable prognosis after surgical treatment (4). However, advanced melanomas, especially those with distant metastases, can be fatal for patients. The treatment effect of advanced SKCM is highly unsatisfactory, ordinary chemotherapy is ineffective (5). In recent years, with the advent of immune checkpoint inhibitor (ICI) therapy and BRAF-targeted therapies, the prognosis for patients with stage III and IV SKCM has improved significantly, but unfortunately, the response is far from guaranteed (6). The search for reliable and accurate prognostic markers is critical to assess SKCM patients’ progression and improve their clinical prognosis. Traditional clinical and histopathological predictors do not apply to all patients with SKCM because of the significant heterogeneity and instability of the SKCM genome (7). The above study suggests that searching for potential prognostic markers at the gene expression level can help identify SKCM patients and improve the prognosis prediction ability to provide SKCM patients with more sensitive and specific risk level classification and more optimized individual-level treatment strategies.
Ferroptosis, a new form of regulatory cell death, is an emerging research hotspot. Unlike the common forms of autophagy, apoptosis, pyroptosis, and necrosis, ferroptosis is a cell death produced by excessive intracellular iron deposition and lipid peroxidation. Excessive intracellular iron deposition can lead to an increase in reactive oxygen species (ROS) or trigger lipid peroxidation, which can cause dysfunction of enzymes in the cell membrane, ultimately leading to alterations in the fluidity and permeability of the cell membrane (8,9). Tumor cells were found to evade other forms of cell death but maintain a persistent sensitivity to ferroptosis (10). For example, triple-negative breast cancer (TNBC) is the most difficult-to-treat breast cancer subtypes, with significant clinical and biological heterogeneity. However, it was found that TNBC maintains a high sensitivity to Ferroptosis-induced cell death, and Ferroptosis was found to promote tumor cell death through gene transcription levels and cellular metabolism (11). In addition, the ferroptosis sensitivity of non-small cell lung cancer (NSCLC) with epidermal growth factor receptor (EGFR) mutations was increased by inhibiting SLC7A11 expression or decreasing cystine levels (12). Therefore, there is an increasing interest in killing tumor cells by modulating key targets of ferroptosis, an area full of potential.
Several prognostic models of SKCM have been identified in previous studies. A prognostic model constructed on the basis of 8 pyroptosis-related genes and clinical features demonstrated good prognostic predictive ability for SKCM patients (13). More conveniently and precisely, the prognosis of SKCM patients may be predicted using the prognostic model created by 7 autophagy-related genes (14). Shen et al. excellently predicted the prognostic survival of SKCM patients by using a prognostic risk model constructed by three immune-related lncRNAs (15). Recent relevant studies have also confirmed that prognostically relevant ferroptosis-related genes (PR-FRGs) screened in SKCM, which have superior predictive ability to clinical traits, can serve as valuable predictors to predict the prognosis of SKCM patients and have shown encouraging results in predicting therapeutic targets in SKCM (16-18). The search for independent predictors is important for SKCM identification, prognosis prediction, and targeted therapy. Gene expression profiles, nomograms, and other models have been used as risk prediction tools to predict the prognosis of SKCM patients (2). Some progress has been made in the study of ferroptosis in SKCM, but it is still in its infancy. Therefore, in-depth exploration of the potential mechanism of ferroptosis in SKCM is of great value for the identification, prognostic assessment, and individualized treatment of SKCM.
In this work, we used screened PR-FRGs to create a risk model and nomogram and evaluated their impact on patient outcomes in combination with relevant clinical traits. At the same time, value analysis and verification demonstrated the prognostic risk model’s dependability. The whole study will contribute to identifying potential prognostic biological targets of SKCM in ferroptosis, which will help to improve the identification of SKCM and improve the survival prognosis of SKCM patients. We present this article in accordance with the TRIPOD reporting checklist (available at https://tcr.amegroups.com/article/view/10.21037/tcr-24-1506/rc).
Methods
Data gathering and processing
This study was conducted in accordance with the Declaration of Helsinki (as revised in 2013). The 473 SKCM samples from the training group were downloaded from The Cancer Genome Atlas (TCGA) (https://portal.gdc.carnec.gov/repository) platform, including transcriptome information and clinical data. Samples with complete clinical information were retained for prognostic analysis. Since only one normal skin sample was available at TCGA, the GTEx platform (https://xenabrowser.net/datapages/) was used to collect 812 normal skin samples that were calibrated and merged with the TCGA data. The validation group GSE19234 dataset was received from the Gene Expression Omnibus (GEO, https://www.ncbi.nlm.nih.gov/geo/) platform and included transcriptional data as well as clinical information from 44 SKCM patients. FRGs were downloaded from the ferrDb database (http://www.zhounan.org/ferrdb) (19). Differentially expressed ferroptosis-related genes (DE-FRGs) between SKCM and normal skin tissues in the training group were obtained using the “limma” R package, with false discovery rate (FDR) <0.05 and |log2 fold change| ≥1 as the screening criterion (20).
Enrichment analysis
Gene Ontology (GO) and the Kyoto Encyclopedia of Genes and Genomes (KEGG) enrichment analyses were performed using the “clusterProfiler” R package (21) to characterize the biological functions and signaling pathways enriched by DE-FRGs. The criterion for analysis was defined as P<0.05.
Construct a protein-protein interaction (PPI) network
Through the STRING platform (http://www.string-db.org/) (22), we mapped the PPI network of all SKCM DE-FRGs and deleted the genes with node disconnection in the network diagram. Analysis and visualization of PPI networks using Cytoscape_v3.9.1 software (23).
Identification of SKCM prognostic risk model genes and model construction
Univariate Cox regression analysis and Kaplan-Meier analysis were conducted on DE-FRGs with a P<0.05 criterion in order to get the PR-FRGs of SKCM. We ended up with 35 PR-FRGs. We then conducted multiple stepwise Cox regression analysis for these 35 PR-FRGs to determine their independent prognostic predictive ability. Detailed results of the multiple stepwise Cox regression analysis and clinical data are provided in the supplementary materials (Tables S1, https://cdn.amegroups.cn/static/public/10.21037tcr-24-1506-1.xls). Ultimately, we obtained 12 optimal PR-FRGs as independent prognostic predictors for SKCM patients. We then calculated regression coefficients and risk scores for each gene to assess patient outcomes. The calculation formula is . coef is the gene coefficient, Expgene is the gene expression. SKCM patients in the training and validation groups were separated into high- and low-risk groups according to median values.
Value assessment and validation of the prognostic model
The training group was the TCGA dataset, while the validation group was GSE19234. R packages “survival” and “survminer” were used to draw the prognostic risk model’s survival curves and assess their prognostic value. Then, to assess how well this prognostic risk model predicted patient survival, we displayed the receiver operating characteristic (ROC) curves of the training and validation groups. Patients’ ROC curves over time (1, 3, and 5 years) were also plotted based on risk scores, and the area under the curve was calculated and used to predict patients’ overall survival (OS) over time. In addition, univariate Cox regression and multiple Cox regression analyses were performed on the clinical traits of patients in the training and validation groups, including age, gender, tumor stage, and risk score. Multivariate analysis was used to find independent clinical characteristics related to prognosis and to verify the predictive ability of the prognostic risk model for the prognosis of SKCM patients. At the same time, the ROC curve of clinical characteristics was drawn, and the accuracy of clinical traits as independent prognostic predictors was judged according to the area under the ROC curve (AUC) value of the curve. Nomogram can be used as an effective predictive tool for the prognosis of cancer or other health problems (24). Therefore, we constructed a nomogram based on the expression of the 12 PR-FRGs using “rms” and “survival” of the R package to predict the OS of SKCM patients at years 1, 3 and 5 and verified the stability of the nomogram by drawing calibration curves.
Analysis of expression levels of PR-FRGs
To determine the differences in mRNA expression of the 12 PR-FRGs in normal skin tissues and skin cancer tissues, we reanalyzed the raw data obtained from the GTEx and TCGA databases. Meanwhile, the protein expression levels of PR-FRGs between normal skin and skin cancer were evaluated by immunohistochemical analysis on the Human Protein Atlas (HPA) website.
Statistical analysis
Data were analyzed using the software package R v.4.1.3. The Wilcoxon test compared the two groups’ differentially expressed genes (DEGs). Cox regression analysis was used to determine independent PR-FRGs and clinical factors. Forest plots were plotted using the R packages “survival” and “survminer”. The R packages “timeROC” and “survivalROC” were used to draw ROC curves. The criterion for statistical significance was set at P<0.05.
Results
Confirmation of DE-FRGs
The design of this study is shown in the flow chart of Figure 1. We merged the TCGA data with the GTEx data to obtain the training group, including 813 normal skin tissues and 472 SKCM tissues. A total of 180 DE-FRGs were obtained by screening. Of these, 103 were up-regulated and 77 were down-regulated. Their expression and enrichment were visualized by heat map and volcano plot (Figure 2A,2B).
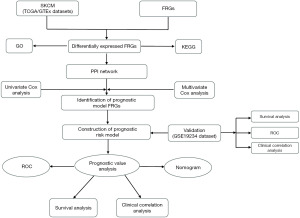
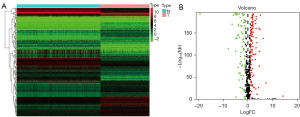
Enrichment analysis
KEGG and GO enrichment analyses were performed to understand the potential biological functions of these 180 DE-FRGs (Figure 3A,3B). KEGG results suggested that DE-FRGs were significantly enriched in lipid and atherosclerosis, chemical carcinogenesis-ROS, human cytomegalovirus infection, PPAR signaling pathway, ferroptosis, necroptosis, nod-like receptor signaling pathway, and other aspects. GO showed enrichment results in biological processes (BPs), such as fatty acid metabolic process, ROS metabolic process, long-chain fatty acid metabolic process, unsaturated fatty acid metabolic process, superoxide metabolic process, and superoxide anion generation. Regarding cellular components (CCs), it was enriched in apical part of cell, apical plasma membrane, peroxisome, microbody, organelle outer membrane, outer membrane, NADPH oxidase complex, etc. In terms of molecular functions (MFs), it was enriched in heme binding, tetrapyrrole binding, iron ion binding, oxidoreductase activity, acting on NAD(P)H, superoxide-generating NAD(P)H oxidase activity, oxidoreductase activity, acting on single donors with incorporation of molecular oxygen and so on. The above results suggest that DE-FRGs play a vital role in SKCM.
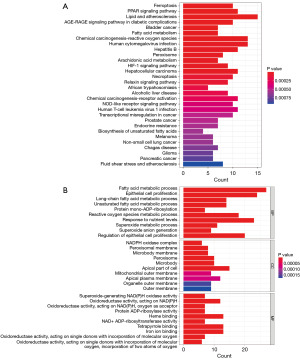
Selection of candidate prognosis-related genes and model construction
We acquired a total of 180 DE-FRGs. Next, the PPI network between DEGs was constructed to find the genes with interaction relationships. In this process, genes with disconnected interaction nodes were eliminated, and finally, a PPI network of 176 DE-FRGs was obtained, and a circle diagram was drawn. Interacting genes are connected by line segments (Figure 4A,4B). Next, we analyzed these 176 DE-FRGs by univariate Cox regression and obtained 35 PR-FRGs (Figure 5A). To obtain the optimal independent predictor genes associated with prognosis, we performed multiple Cox regression analysis and finally obtained 12 PR-FRGs (Figure 5B). A prognostic risk model was constructed using these 12 PR-FRGs. The model formula is: Risk score = (−0.15895*ExpNOX4) + (0.31321*ExpFH) + (−0.47352*ExpARNTL) + (0.26180*ExpEGFR) + (0.15689*ExpGJA1) + (0.25809*ExpSESN2) + (0.23066*ExpKIF20A) + (−0.26474*ExpTRIM21) + (0.35484*ExpSUV39H1) + (−0.29347*ExpTMSB4X) + (0.26953*ExpUSP35) + (0.15503*ExpCYBB). Finally, the median value of the risk score was used to separate the SKCM patients in the training and validation groups into high- and low-risk groups.
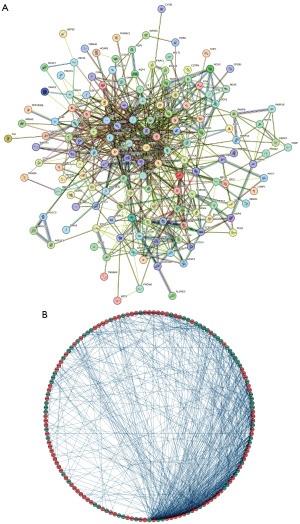
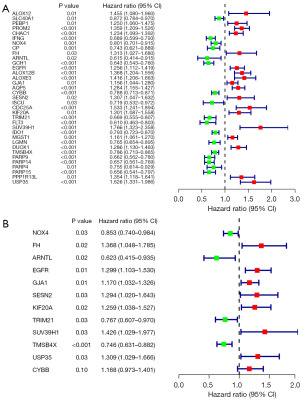
Prognostic value analysis and validation of risk model
Based on the risk scores, we plotted the risk curves of the patients in the training group and a scatterplot of survival status (Figure 6A,6B). As shown, the patient’s risk score rises in order from left to right. The number of patients who died gradually increased as their risk score increased. We also mapped the heat map of the expression of 12 PR-FRGs in the training group (Figure 6C) and discovered that while the expression of genes like NOX4, ARNTL, and CYBB was lowered in the high-risk group, the expression of genes like EGFR, USP35, and GJA1 was enhanced in this group. Figure 6D illustrates the survival curves for patients in the high and low-risk groups, with patients in the low-risk group having a higher survival rate. To observe the accuracy of the risk model in predicting patient survival, we constructed the ROC curve of the risk model, and the AUC value of the curve was 0.763 (Figure 6E). Based on the patient’s risk scores, we also plotted the ROC curves over time, which showed that the AUC values for the 1-, 3-, and 5-year curves were 0.759, 0.708, and 0.740, respectively (Figure 6F). This suggests that risk models have good accuracy in predicting patient survival.
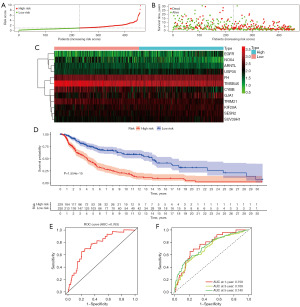
Next, to validate the accuracy of the risk model, we validated it with an external dataset, GSE19234, and obtained the same results. Low-risk SKCM patients had a superior survival prognosis (Figure 7A,7B). The heat map of the PR-FRGs expression trend in the validation group was similar to the results in the training group (Figure 7C). In the survival curve of the GSE19234 dataset, high-risk SKCM patients had lower survival rates than those at low-risk (Figure 7D). Meanwhile, the risk model constructed to predict the survival of the patients in the validation group had good accuracy, and the AUC value of the ROC curve obtained was 0.834 (Figure 7E). Moreover, the ROC results showed that the AUC values of the validation group’s 1-, 3-, and 5-year OS were 0.836, 0.712, and 0.796 (Figure 7F). The above results suggest that the risk model we have constructed is of good prognostic value, both through the internal dataset and the external validation data.
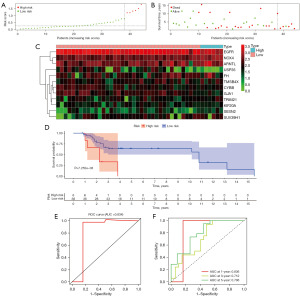
Clinical correlation analysis
To determine whether the risk model can predict patient survival independently of other clinical traits, we analyzed the age, sex, stage, and risk model of SKCM patients with univariate Cox regression and multiple Cox regression. We found that age, stage, and risk score could be used as independent prognostic predictors in the training group (Figure 8A,8B). Two meaningful clinical traits, stage and risk score were obtained in the validation group (Figure 8C,8D). Combining the training and validation groups results, we believe that the stage and risk model can be used as independent prognostic factors to predict patient survival. In order to verify the accuracy of clinical traits as independent prognostic factors in predicting patient outcomes, ROC analysis of clinical traits was performed. In the training group, AUC values for the risk model and stage were 0.708 and 0.656, respectively (Figure 8E). In the validation group, the AUC values for the risk model and stage were 0.712 and 0.602, respectively (Figure 8F). The above results illustrate that the risk model and stage can be used as independent prognostic factors to predict the prognosis of SKCM patients. Moreover, the risk model was superior to other clinical traits in predicting patient survival.
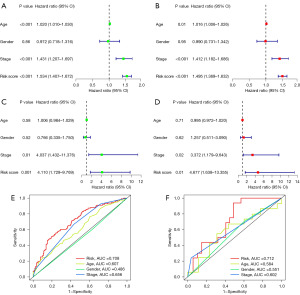
Establishment of nomogram based on PR-FRGs
Based on the 12 PR-FRGs, we constructed a nomogram model. With this nomogram, we can more intuitively observe the relationship between the expression of PR-FRGs and the survival status of patients at the corresponding time (Figure 9A). Based on the expression of these 12 PR-FRGs, we obtained the related total scores, and based on the total scores, we went on to predict the survival rate of the patients at the corresponding times (1, 3, 5 years). Calibration curves also verified the accuracy of the nomogram in predicting OS (Figure 9B). We found that the survival curves for patients at 1, 3, and 5 years were very close to the calibration curves of 45 degrees. This suggests that the nomogram we used to predict OS in SKCM patients is of good accuracy.
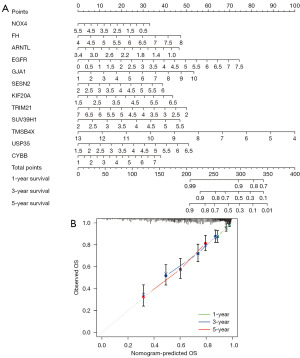
Confirmation of PR-FRGs expression levels
After our search, we found protein expression results for 9 of these PR-FRGs on the HPA website. We compared the mRNA expression levels of PR-FRGs in normal and tumor tissues and found that the expression of EGFR, GJA1, TMSB4X was decreased in tumor tissues, while the expression of FH, SESN2, KIF20A, TRIM21, USP35, CYBB was elevated (Figure 10A). To our surprise, except for CYBB, which showed low protein expression in both normal and tumor tissues, the protein expression of the other PR-FRGs in both normal and tumor tissues was consistent with the trend of mRNA expression (Figure 10B). This suggests that PR-FRGs can be used to construct prognostic risk model.
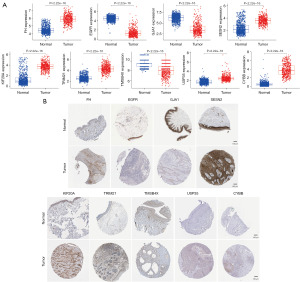
Discussion
SKCM is the malignant tumor with the highest frequency of gene mutations, highly aggressive and very easily metastasized, of which the mutated genes of most interest are the oncogene BRAF and the tumor suppressor gene CDKN2A (25). Mutations in BRAF and CDKN2A will contribute to SKCM cell proliferation and escape from apoptosis (26). Compared with the traditional TNM staging and clinical traits, predicting prognosis by studying individualized genomic biomarkers in SKCM patients has become a current research hotspot (27,28). Indeed, the biological behavior and subsequent clinical behavior of each SKCM patient can be better predicted by genomic technology, and even the changes in the molecular level of tumor cells and the tumor microenvironment can be dynamically observed during the development of SKCM, so as to achieve individualized treatment for SKCM patients (27).
In our study, we obtained 180 FRGs differentially expressed in normal skin tissues and SKCM tissues and performed enrichment analysis of their pathways and functions. Results showed that DE-FRGs were significantly enriched in fatty acid and ROS, heme and iron ion binding, and regulation of oxidoreductase activity. These results demonstrated that DE-FGRs promoted the occurrence of ferroptosis in tumor cells by affecting iron metabolism and ROS production. Next, through the screening of the PPI network and Cox regression analysis, we got 12 PR-FRGs markers and constructed a prognostic risk model. Survival analysis was performed on the risk model, and it was found that patients in the low-risk group achieved better prognostic survival. ROC analysis validated the accuracy of the risk model and the patient survival analysis results, which showed that the risk model we constructed demonstrated excellent predictive ability. Similarly, our risk model also showed encouraging results in the validation group. Next, to explore which factors can serve as independent predictors related to prognosis, we performed Cox regression on the clinical traits as well as the risk model in the training and validation groups, and the results showed that the risk model, as well as the tumor stage based on TNM staging, can serve as independent prognostic factors to predict the clinical prognosis of patients. Interestingly, the risk model has better predictive ability than other clinical traits. This is supported by the findings of the ROC curves for clinical traits. We next constructed a nomogram by integrating the expression of 12 PR-FRGs, which will help to make an optimal prediction of the prognosis of SKCM patients. In our constructed nomogram, the scoring of each gene marker was obtained by the transcript levels of 12 PR-FRGs, and the total score was calculated, and then the OS of the patients at year 1, 3, 5 was obtained based on the total score. Finally, calibration curves were used to confirm the nomogram’s accurateness.
It is an up-and-coming field for treating organ ischemia-reperfusion injury, drug-resistant malignant tumors, and degenerative diseases related to lipid peroxidation by regulating ferroptosis (9,29). Tumors susceptible to drug resistance and high malignancy have a very high chance of ferroptosis because they have a higher metabolic and ROS burden (30). Ferroptosis has been revealed to have a crucial growth-regulating function in malignancies like ovarian cancer, hepatocellular carcinoma, pancreatic cancer, and renal cell carcinoma in recent research (31). Little is known, however, regarding the mechanism of ferroptosis in SKCM. Regulation of related genes has been shown to have an essential role in ferroptosis. For example, mutant p53 inhibits cystine uptake and increases sensitivity to ferroptosis by suppressing the expression of SLC7A11 (32). The resistance of hepatocellular carcinoma cells to sorafenib could be reversed by down-regulating the expression of MT1G, which promotes the occurrence of ferroptosis in tumor cells (33).
We constructed a risk model composed of 12 FRGs, which have been reported in previous oncology studies (34-36). Down-regulation of NOX4 expression can inhibit the adhesion and metastasis of gastric cancer cells via the JAK2/STAT3 pathway (37). Mutations in FH, the gene encoding fumarate hydratase, can lead to rare renal cell carcinoma (38). As an oncogene, the up-regulation of SUV39H1 in renal cell carcinoma inhibits the expression of DPP4, the regulator of ferroptosis, thus contributing to the escape of tumor cells from the killing effect of ferroptosis (39). ARNTL, a biological clock protein that regulates circadian rhythms and is closely related to cancer, can be selectively degraded by autophagy-dependent pathways in response to GPX4 inhibitors, which in turn initiates the ferroptosis pathway in tumor cells (40). The EGFR mutation can lead to treatment resistance in people with NSCLC. However, the combination of β-elemene and erlotinib induces ferroptosis in cells targeting EGFR by up-regulating the expression of lncRNA H19 (41). In addition, GJA1 has been found to have elevated expression in lung cancer patients, and MiR-613 can inhibit it as a target gene, increasing lung cancer cells’ sensitivity to cisplatin (42,43). Stress proteins expressed by SESN2 are induced by inflammation, autophagy, and oxidative stress, exert protective effects on the body, and regulate ferroptosis (44). Overexpression of KIF20A inhibits the occurrence of ferroptosis in colon cancer and then promotes tumor cells to Oxaliplatin resistance through the GSK3β/Nrf2 pathway, making KIF20A a promising biological target for the treatment of drug resistance in colon cancer (45). TRIM2A is extensively involved in the ferroptosis process in human cancers. It negatively regulates ferroptosis by ubiquitinating K63 on FSP1, which is promising as a new therapeutic target for chemotherapeutic agents (46). Studies have shown that TMSB4X plays a fundamental role in the development of melanoma and glioblastoma, and the Thymosin β4 encoded by TMSB4X has a critical regulatory role in cell adhesion and migration (47,48). TMSB4X is promising as a new therapeutic target. In addition, CYBB, which is highly expressed in glioblastomas, also exhibits resistance to ferroptosis through the Nrf2/SOD2 pathway (49). USP35-encoded deubiquitinase regulates the proliferation and migration of lung cancer cells and is widely expressed in lung cancer tissues. Knockdown of USP35 promoted cancer cell ferroptosis and inhibited cancer cell growth and metastasis (50). Similar results were also found in renal cell carcinoma, but the role of USP35 in SKCM was not investigated (51). The above results indicate that these 12 PR-FRGs can be used to construct prognostic risk models and perform well in the prognostic analysis of SKCM patients.
Conclusions
In conclusion, we constructed a prognostic risk model with 12 PR-FRGs. Through the analysis of the prognostic value of the prognostic risk model and the validation of the GSE19234 dataset, we found that the prognostic risk model does indeed serve as a good and stable independent predictor. In addition, the nomogram constructed based on the expression of 12 PR-FRGs also showed significant advantages in predicting the prognostic survival of patients. These findings will help us to identify new prognostic-related markers that can contribute to better identification and individualized treatment for SKCM patients, thus improving the clinical prognosis and enhancing the quality of life of SKCM patients.
Acknowledgments
Thanks to the TCGA and GEO public databases for supporting this study. Thanks to all the team members for participating in this study.
Footnote
Reporting Checklist: The authors have completed the TRIPOD reporting checklist. Available at https://tcr.amegroups.com/article/view/10.21037/tcr-24-1506/rc
Peer Review File: Available at https://tcr.amegroups.com/article/view/10.21037/tcr-24-1506/prf
Funding: None.
Conflicts of Interest: All authors have completed the ICMJE uniform disclosure form (available at https://tcr.amegroups.com/article/view/10.21037/tcr-24-1506/coif). The authors have no conflicts of interest to declare.
Ethical Statement: The authors are accountable for all aspects of the work in ensuring that questions related to the accuracy or integrity of any part of the work are appropriately investigated and resolved. This study was conducted in accordance with the Declaration of Helsinki (as revised in 2013).
Open Access Statement: This is an Open Access article distributed in accordance with the Creative Commons Attribution-NonCommercial-NoDerivs 4.0 International License (CC BY-NC-ND 4.0), which permits the non-commercial replication and distribution of the article with the strict proviso that no changes or edits are made and the original work is properly cited (including links to both the formal publication through the relevant DOI and the license). See: https://creativecommons.org/licenses/by-nc-nd/4.0/.
References
- Saginala K, Barsouk A, Aluru JS, et al. Epidemiology of Melanoma. Med Sci (Basel) 2021;9:63. [Crossref] [PubMed]
- Long GV, Swetter SM, Menzies AM, et al. Cutaneous melanoma. Lancet 2023;402:485-502. [Crossref] [PubMed]
- Kim HJ, Kim YH. Molecular Frontiers in Melanoma: Pathogenesis, Diagnosis, and Therapeutic Advances. Int J Mol Sci 2024;25:2984. [PubMed]
- Carlino MS, Larkin J, Long GV. Immune checkpoint inhibitors in melanoma. Lancet 2021;398:1002-14. [Crossref] [PubMed]
- Dimitrova M, Weber J. Melanoma-Modern Treatment for Metastatic Melanoma. Cancer J 2024;30:79-83. [Crossref] [PubMed]
- Maher NG, Vergara IA, Long GV, et al. Prognostic and predictive biomarkers in melanoma. Pathology 2024;56:259-73. [Crossref] [PubMed]
- Holder AM, Dedeilia A, Sierra-Davidson K, et al. Defining clinically useful biomarkers of immune checkpoint inhibitors in solid tumours. Nat Rev Cancer 2024;24:498-512. [Crossref] [PubMed]
- Xie Y, Hou W, Song X, et al. Ferroptosis: process and function. Cell Death Differ 2016;23:369-79. [Crossref] [PubMed]
- Chang S, Tang M, Zhang B, et al. Ferroptosis in inflammatory arthritis: A promising future. Front Immunol 2022;13:955069. [Crossref] [PubMed]
- Bebber CM, Müller F, Prieto Clemente L, et al. Ferroptosis in Cancer Cell Biology. Cancers (Basel) 2020;12:164. [Crossref] [PubMed]
- Yang F, Xiao Y, Ding JH, et al. Ferroptosis heterogeneity in triple-negative breast cancer reveals an innovative immunotherapy combination strategy. Cell Metab 2023;35:84-100.e8. [PubMed]
- Poursaitidis I, Wang X, Crighton T, et al. Oncogene-Selective Sensitivity to Synchronous Cell Death following Modulation of the Amino Acid Nutrient Cystine. Cell Rep 2017;18:2547-56. [PubMed]
- Zhu Y, Han D, Duan H, et al. Identification of Pyroptosis-Relevant Signature in Tumor Immune Microenvironment and Prognosis in Skin Cutaneous Melanoma Using Network Analysis. Stem Cells Int 2023;2023:3827999. [Crossref] [PubMed]
- Wan Q, Jin L, Su Y, et al. Development and validation of autophagy-related-gene biomarker and nomogram for predicting the survival of cutaneous melanoma. IUBMB Life 2020;72:1364-78. [Crossref] [PubMed]
- Shen X, Shang L, Han J, et al. Immune-related gene signature associates with immune landscape and predicts prognosis accurately in patients with skin cutaneous melanoma. Front Genet 2023;13:1095867. [Crossref] [PubMed]
- Ping S, Wang S, Zhao Y, et al. Identification and validation of a ferroptosis-related gene signature for predicting survival in skin cutaneous melanoma. Cancer Med 2022;11:3529-41. [Crossref] [PubMed]
- Liu C, Liu Y, Yu Y, et al. Comprehensive analysis of ferroptosis-related genes and prognosis of cutaneous melanoma. BMC Med Genomics 2022;15:39. [Crossref] [PubMed]
- Khorsandi K, Esfahani H, Ghamsari SK, et al. Targeting ferroptosis in melanoma: cancer therapeutics. Cell Commun Signal 2023;21:337. [Crossref] [PubMed]
- Zhou N, Bao J. FerrDb: a manually curated resource for regulators and markers of ferroptosis and ferroptosis-disease associations. Database (Oxford) 2020;2020:baaa021. [Crossref] [PubMed]
- Meng J, Du H, Lu J, et al. Construction and validation of a predictive nomogram for ferroptosis-related genes in osteosarcoma. J Cancer Res Clin Oncol 2023;149:14227-39. [Crossref] [PubMed]
- Yu G, Wang LG, Han Y, et al. clusterProfiler: an R package for comparing biological themes among gene clusters. OMICS 2012;16:284-7. [Crossref] [PubMed]
- Szklarczyk D, Franceschini A, Kuhn M, et al. The STRING database in 2011: functional interaction networks of proteins, globally integrated and scored. Nucleic Acids Res 2011;39:D561-8. [Crossref] [PubMed]
- Shannon P, Markiel A, Ozier O, et al. Cytoscape: a software environment for integrated models of biomolecular interaction networks. Genome Res 2003;13:2498-504. [Crossref] [PubMed]
- Balachandran VP, Gonen M, Smith JJ, et al. Nomograms in oncology: more than meets the eye. Lancet Oncol 2015;16:e173-80. [Crossref] [PubMed]
- Nurla LA, Aşchie M, Cozaru GC, et al. Multiple Primary Melanoma Associated with CDKN2A Mutation-Case Report and Review of the Literature. Medicina (Kaunas) 2024;60:763. [Crossref] [PubMed]
- Al Hmada Y, Brodell RT, Kharouf N, et al. Mechanisms of Melanoma Progression and Treatment Resistance: Role of Cancer Stem-like Cells. Cancers (Basel) 2024;16:470. [Crossref] [PubMed]
- Scatena C, Murtas D, Tomei S. Cutaneous Melanoma Classification: The Importance of High-Throughput Genomic Technologies. Front Oncol 2021;11:635488. [Crossref] [PubMed]
- Ogata D, Namikawa K, Takahashi A, et al. A review of the AJCC melanoma staging system in the TNM classification (eighth edition). Jpn J Clin Oncol 2021;51:671-4.
- Benjamin EJ, Blaha MJ, Chiuve SE, et al. Heart Disease and Stroke Statistics-2017 Update: A Report From the American Heart Association. Circulation 2017;135:e146-603. [Crossref] [PubMed]
- Jiang X, Stockwell BR, Conrad M. Ferroptosis: mechanisms, biology and role in disease. Nat Rev Mol Cell Biol 2021;22:266-82. [Crossref] [PubMed]
- Xia X, Fan X, Zhao M, et al. The Relationship between Ferroptosis and Tumors: A Novel Landscape for Therapeutic Approach. Curr Gene Ther 2019;19:117-24. [Crossref] [PubMed]
- Liu Y, Su Z, Tavana O, et al. Understanding the complexity of p53 in a new era of tumor suppression. Cancer Cell 2024;42:946-67. [Crossref] [PubMed]
- Sun X, Niu X, Chen R, et al. Metallothionein-1G facilitates sorafenib resistance through inhibition of ferroptosis. Hepatology 2016;64:488-500. [Crossref] [PubMed]
- Wang L, Gong W. NOX4 regulates gastric cancer cell invasion and proliferation by increasing ferroptosis sensitivity through regulating ROS. Int Immunopharmacol 2024;132:112052. [Crossref] [PubMed]
- Li X, Liu C, Gao Y. SUV39H1 Regulates Gastric Cancer Progression via the H3K9me3/ALDOB Axis. Cell Biochem Biophys 2025;83:919-28. [Crossref] [PubMed]
- Shen J, Chen L, Liu J, et al. EGFR degraders in non-small-cell lung cancer: Breakthrough and unresolved issue. Chem Biol Drug Des 2024;103:e14517. [Crossref] [PubMed]
- Gao X, Sun J, Huang C, et al. RNAi-mediated silencing of NOX4 inhibited the invasion of gastric cancer cells through JAK2/STAT3 signaling. Am J Transl Res 2017;9:4440-9. [PubMed]
- Yoo A, Tang C, Zucker M, et al. Genomic and Metabolic Hallmarks of SDH- and FH-deficient Renal Cell Carcinomas. Eur Urol Focus 2022;8:1278-88. [Crossref] [PubMed]
- Wang J, Yin X, He W, et al. SUV39H1 deficiency suppresses clear cell renal cell carcinoma growth by inducing ferroptosis. Acta Pharm Sin B 2021;11:406-19. [Crossref] [PubMed]
- Yang M, Chen P, Liu J, et al. Clockophagy is a novel selective autophagy process favoring ferroptosis. Sci Adv 2019;5:eaaw2238. [Crossref] [PubMed]
- Xu C, Jiang ZB, Shao L, et al. β-Elemene enhances erlotinib sensitivity through induction of ferroptosis by upregulating lncRNA H19 in EGFR-mutant non-small cell lung cancer. Pharmacol Res 2023;191:106739. [Crossref] [PubMed]
- Li D, Meng D, Niu R. Exosome-Reversed Chemoresistance to Cisplatin in Non-Small Lung Cancer Through Transferring miR-613. Cancer Manag Res 2020;12:7961-72. [Crossref] [PubMed]
- Dai W, Chao X, Li S, et al. Long Noncoding RNA HOTAIR Functions as a Competitive Endogenous RNA to Regulate Connexin43 Remodeling in Atrial Fibrillation by Sponging MicroRNA-613. Cardiovasc Ther 2020;2020:5925342. [PubMed]
- El-Horany HE, Atef MM, Abdel Ghafar MT, et al. Empagliflozin Ameliorates Bleomycin-Induced Pulmonary Fibrosis in Rats by Modulating Sesn2/AMPK/Nrf2 Signaling and Targeting Ferroptosis and Autophagy. Int J Mol Sci 2023;24:9481. [PubMed]
- Yang C, Zhang Y, Lin S, et al. Suppressing the KIF20A/NUAK1/Nrf2/GPX4 signaling pathway induces ferroptosis and enhances the sensitivity of colorectal cancer to oxaliplatin. Aging (Albany NY) 2021;13:13515-34. [PubMed]
- Gong J, Liu Y, Wang W, et al. TRIM21-Promoted FSP1 Plasma Membrane Translocation Confers Ferroptosis Resistance in Human Cancers. Adv Sci (Weinh) 2023;10:e2302318. [Crossref] [PubMed]
- Makowiecka A, Malek N, Mazurkiewicz E, et al. Thymosin β4 Regulates Focal Adhesion Formation in Human Melanoma Cells and Affects Their Migration and Invasion. Front Cell Dev Biol 2019;7:304. [Crossref] [PubMed]
- Wirsching HG, Krishnan S, Florea AM, et al. Thymosin β 4 gene silencing decreases stemness and invasiveness in glioblastoma. Brain 2014;137:433-48. [Crossref] [PubMed]
- Su IC, Su YK, Setiawan SA, et al. NADPH Oxidase Subunit CYBB Confers Chemotherapy and Ferroptosis Resistance in Mesenchymal Glioblastoma via Nrf2/SOD2 Modulation. Int J Mol Sci 2023;24:7706. [Crossref] [PubMed]
- Tang Z, Jiang W, Mao M, et al. Deubiquitinase USP35 modulates ferroptosis in lung cancer via targeting ferroportin. Clin Transl Med 2021;11:e390. [Crossref] [PubMed]
- Wang S, Wang T, Zhang X, et al. The deubiquitylating enzyme USP35 restricts regulated cell death to promote survival of renal clear cell carcinoma. Cell Death Differ 2023;30:1757-70. [Crossref] [PubMed]