A gene signature associated with cellular senescence serves as an important prognostic indicator in hepatocellular carcinoma
Highlight box
Key findings
• Cellular senescence is one of the hallmarks of cancer, and its related prognostic-gene signatures can provide critical information for clinical decision making. Cellular senescence-related genes may serve as good prognostic indicators of hepatocellular carcinoma (HCC).
What is known, and what is new?
• HCC can be evaluated based on cellular senescence-related genes.
• This study identified HCC subtypes and biomarkers related to cellular senescence.
What is the implication, and what should change now?
• We established a prognostic model of HCC based on cellular senescence-related gene expression. Our findings may provide insights into novel potential targeted therapies.
Introduction
Hepatocellular carcinoma (HCC) is an especially aggressive type of cancer. In 2020, approximately one million new cases were reported worldwide (1,2). The primary risk factors contributing to the onset of HCC are chronic alcohol consumption, diabetes mellitus, metabolic disorders, and infection with the hepatitis B virus (2-4). Each of these risk factors can lead to cirrhotic changes, subsequently elevating the risk of HCC development (3-5). The limitations in treatment options for HCC primarily stem from factors such as tumor size, the presence of metastasis, and underlying liver conditions like cirrhosis. As HCC often presents at an advanced stage, surgical interventions become less viable, and systemic therapies may have limited efficacy due to the tumor’s heterogeneity and resistance mechanisms. If HCC is diagnosed at an advanced stage, the range of available treatment options becomes markedly restricted (5).
Extensive research has shown that several factors can significantly compromise the efficacy of targeted therapeutic interventions for HCC. These factors include the disruption of telomere maintenance, alterations in chromatin structure, dysregulation of the cell cycle, oxidative stress in HCC cells, and substantial genetic mutations or atypical gene expression (6,7). Ongoing research into various gene expression profiles continues to refine therapeutic strategies; however, research on prognostic prediction for HCC patients remains limited. While existing prognostic models provide some insights, they often fail to account for the molecular heterogeneity of HCC and do not integrate key factors such as aging-related genetic alterations. Additionally, there is a scarcity of research focusing on the specific impact of these aging-related genes on prognosis, which limits our understanding of their role in HCC progression. Thus, novel biomarkers urgently need to be identified to facilitate clinical decision making and identify individuals with an increased risk of HCC.
Over the past decade, research has revealed a close association between cellular senescence—a crucial hallmark of cancer—and the conventional mechanisms of carcinogenesis, tumor advancement, and the invasiveness of HCC (8-11). Cellular senescence is characterized by an irreversible cessation of cellular proliferation (10,11). Moreover, these genes can induce cellular senescence, a state that halts the proliferation of damaged or stressed cells, thereby preventing the progression of potential tumors. This tumor-suppressive effect is crucial in maintaining tissue homeostasis and preventing the accumulation of mutations that could lead to cancer. Various therapeutic modalities can induce senescence in malignant cells via mechanisms such as genotoxic stress, enhanced mitogenic signaling, and oxidative stress, leading to the permanent arrest of the cell cycle (12). Therapy-induced senescence is an initial anti-tumor strategy aimed at curtailing cellular proliferation and alleviating genomic instability. In the tumor microenvironment, senescent cells can secrete pro-inflammatory cytokines, growth factors, and proteases, collectively known as the senescence-associated secretory phenotype (SASP).
Despite extensive research, the exact role of cellular senescence in HCC remains unclear. An in-depth evaluation of prognostic signatures associated with cellular senescence in HCC patients could extend our understanding of the underlying mechanisms of HCC, and yield innovative approaches for accurate diagnosis and treatment. Gene signatures derived from machine-learning methodologies could enhance cancer prognosis assessment and inform immunotherapeutic strategies (13). This study conducted a comprehensive analysis of gene expression data relevant to prognosis, integrating relevant clinical information from HCC patients. Consequently, a model was developed based on the genes associated with cellular senescence scores, which could serve as a novel prognostic tool for individuals diagnosed with HCC. We present this article in accordance with the TRIPOD reporting checklist (available at https://tcr.amegroups.com/article/view/10.21037/tcr-2025-335/rc).
Methods
Data acquisition and preparation
Gene expression datasets along with clinical follow-up information pertaining to patients with HCC were sourced from The Cancer Genome Atlas (TCGA) repository. To ensure the integrity of the prognostic evaluations, samples lacking overall survival (OS) data were excluded from the analysis, and only those with relevant prognostic indicators were retained. The study was conducted in accordance with the Declaration of Helsinki (as revised in 2013).
Subtype identification using cellular senescence-related genes
HCC was categorized into subtypes based on significant expression differences in the cellular senescence-related genes. Unsupervised hierarchical clustering was performed using R (version 3.6.1) of the ConsensusClusterPlus package. A range of 2 to 6 was used to determine the ideal number of clusters (k value). To examine the correlation between various HCC subtypes and survival outcomes, Kaplan-Meier (KM) survival curves were generated using the R survival package (version 2.41-1).
Clinical characteristics associated with HCC subtypes
The clinical characteristics, including age, tumor grade, pathological stage, tumor node metastasis (TNM) classification, and prior exposure to radiation, of the HCC patients was assessed. The relationships between these clinical characteristics and the identified HCC subtypes were examined using the chi-square test, with the significance threshold set at P<0.05.
DEG identification across HCC subtypes
To examine the molecular mechanisms that differentiate the HCC subtypes, linear regression and empirical Bayesian techniques were employed to screen the differentially expressed genes (DEGs) using the limma package (version 3.10.3). In our study, the gene expression values were normalized using the transcripts per million (TPM) method. This normalization approach was chosen to account for both sequencing depth and gene length, allowing for a more accurate comparison of gene expression levels across samples. Differences were considered statistically significant if the following criteria were met: a P value threshold of <0.05, and a |log2 fold change| >1. A heatmap illustrating the DEGs was generated using the R package “pheatmap” (version 1.0.12). Additionally, Gene Ontology (GO) and Kyoto Encyclopedia of Genes and Genomes (KEGG) pathway enrichment analyses were performed using the “clusterProfiler” package in R.
Comparative analysis of the immune microenvironment among the subtypes
The “immunoeconomics” framework was employed to evaluate the immune functionality of the cellular senescence-related genes. A comparative analysis of immune activity between the two clusters related to cellular senescence was conducted by examining the expression levels of 10 immune checkpoint genes (i.e., CD274, PDCD1, PDCD1LG2, CTLA4, LAG3, HAVCR2, IGSF8, ITPRIPL1, TIGIT, and SIGLEC1). Heatmaps and box plots were generated using the R packages “pheatmap” and “ggplot2”, respectively. The Wilcoxon test was used to assess the infiltration of the immune cells and the activation of immune pathways across the two clusters. P values <0.05 were considered statistically significant.
Development of a risk score-based prognostic model
Using the DEGs identified in our study, a univariate Cox regression analysis was conducted to identify the gene expression levels that were significantly associated with survival based on a significance threshold of P<0.05. The least absolute shrinkage and selection operator (LASSO) algorithm in the R survminer package (version 0.4.9) was also used to analyze the prognostic-gene signatures and to develop a prognostic model based on the risk scores. For each sample in TCGA dataset, individual risk scores were computed, and the patients were subsequently categorized into high- and low-risk groups based on the median score. To evaluate variances in the actual survival outcomes, a KM curve was subsequently plotted.
Statistical analysis
Differences were considered statistically significant if the following criteria were met: a P value threshold of <0.05, and a |log2 fold change| >1. The relationships between these clinical characteristics and the identified HCC subtypes were examined using the chi-square test, with the significance threshold set at P<0.05. A univariate Cox regression analysis was conducted to identify the gene expression levels that were significantly associated with survival based on a significance threshold of P<0.05.
Results
Two HCC subtypes were identified based on the cellular senescence-related genes
The critical genes associated with cellular senescence were integrated into the unsupervised clustering analysis, resulting in the identification of the optimal number of subtypes (designated as k=2). To assess the reliability of the clustering outcomes, the degree of ambiguous clustering was evaluated, which reaffirmed k=2 as the optimal choice (Figure 1A). The HCC samples were categorized into two distinct subtypes, referred to as Cluster 1 (C1) and Cluster 2 (C2) (Figure 1B,1C). The survival analysis revealed that the patients in C1 had a significantly worse prognosis than those in C2 (Figure 1D). These findings emphasize that the low expression of cellular senescence-related genes, as observed in C2, may serve as a favorable prognostic indicator.
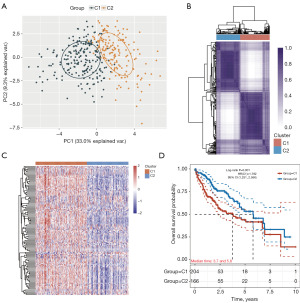
Differences in the clinical characteristics of the two HCC subtypes
The expression levels of the critical cellular senescence-related genes in relation to various clinical characteristics were examined. The analysis across the different subtypes showed that the distribution of C1 was significantly correlated with factors such as age, gender, pathological T stage, TNM classification, and grade staging (Figure 2). These findings suggest that C1 may serve as a prognostic indicator of risk, potentially reflecting the progression of clinical symptoms.
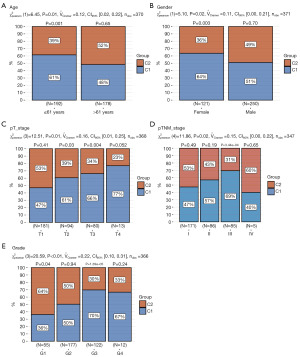
The molecular regulatory mechanisms based on the DEGs between the two HCC subtypes
To extend our understanding of the distinct molecular regulatory mechanisms present among the subtypes, a differential analysis of the gene expression levels between C1 and C2 was conducted. The distribution of the DEGs across these two clusters is illustrated in Figure 3A. Our analysis revealed that 947 genes were upregulated while 90 genes we downregulated (Figure 3A). The accompanying heatmap, in which red represents upregulation, and blue indicates downregulation (Figure 3B), further shows the expression levels of the DEGs across the various subgroups.
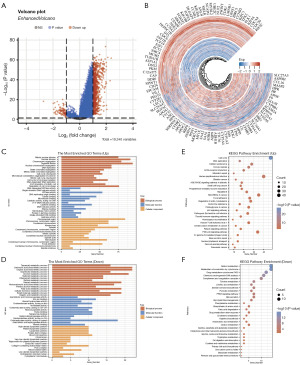
Subsequent KEGG and GO enrichment analyses were performed to examine the biological functions associated with the 1,137 DEGs identified. The results of the GO analysis pertaining to biological processes indicated that the majority of the upregulated genes were associated with mitotic nuclear division, nuclear division, chromosome segregation, DNA replication, and cell-cycle checkpoint activities (Figure 3C). Conversely, the downregulated genes were linked to processes such as metabolic functions, organic acid biosynthesis, isoprenoid metabolism, and alcohol metabolism (Figure 3D). The KEGG analysis further revealed that the 947 upregulated genes were predominantly involved in pathways associated with the cell cycle, DNA replication, cellular senescence, extracellular matrix (ECM)-receptor interactions, and mismatch repair mechanisms (Figure 3E). Conversely, the 90 downregulated genes were mainly implicated in metabolic pathways, chemical carcinogenesis-DNA adducts, complement and coagulation cascades, and the peroxisome proliferator-activated receptor (PPAR) signaling pathway (Figure 3F).
Previous research has indicated that cell cycle, DNA replication, cellular senescence, and ECM-receptor interactions can serve as tumor markers (6-10). Based on the aforementioned finding, it appears that the C1 HCC subtypes had a greater propensity for migration and proliferation than their C2 HCC counterparts.
Immune activity between the two cellular senescence-related groups in HCC
Numerous studies have reported a relationship between cellular senescence and immune response across various cancer types (6-8). Using a significance threshold of P<0.05, a significant difference in the infiltration of 10 distinct immune cell types was detected between C1 and C2 (Figure 3A). The resulting boxplots illustrated a pronounced variation in the immune cell populations, including B cells, endothelial cells, natural killer (NK) cells, macrophages, cluster of differentiation (CD)4+ T cells, and CD8+ T cells, in the C1 HCC samples compared to the C2 HCC samples (Figure 4A).
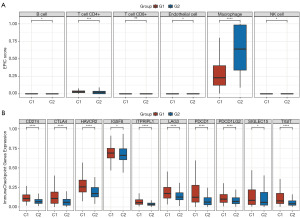
Further, the boxplots revealed that the expression levels of the 10 immune checkpoint inhibitor (ICI)-related genes (i.e., CD274, PDCD1, PDCD1LG2, CTLA4, LAG3, HAVCR2, IGSF8, ITPRIPL1, TIGIT, and SIGLEC15) were significantly higher in C1 than C2 (Figure 4B). These results revealed a notable connection between cellular senescence and immune activity.
Prognostic signature development of the two cellular senescence-related groups in HCC
A univariate Cox analysis was performed on the patients from TCGA-HCC cohort to identify the prognostic genes and their respective functions. A total of 4,352 prognostic genes were identified, and a Venn diagram was used to isolate those associated with cellular senescence. Ultimately, 41 genes relevant to this biological phenomenon were identified (Figure 5A,5B). Further, the LASSO regression analysis suggested a significant correlation between the genes related to cellular senescence and OS (Figure 5A,5B). Through this thorough analysis, 12 prognostic genes were identified: VDAC2, CXCL8, MYBL2, RAD9A, LIN52, RHEB, GADD45G, E2F5, MAP2K2, CDC25A, PPP1CB, and HRAS (Figure 5A,5B). The KM survival analysis revealed that individuals in the high-risk group had significantly worse clinical outcomes in terms of OS than those in the low-risk group (Figure 5C). Additionally, an evaluation of the area under the curve (AUC) values for the prognostic markers of the three aforementioned genes in comparison to each gene individually indicated that the composite marker formed by these three genes produced a higher AUC value. This suggests that the risk-score model, which incorporates these genes, showed improved stability in predicting survival outcomes at 1 year (AUC: 0.764), 3 years (AUC: 0.701), and 5 years (AUC: 0.706) (Figure 5D).
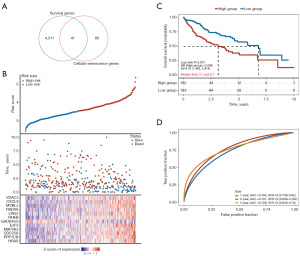
Discussion
In this study, cellular senescence-related genes were employed to stratify patients from TCGA-HCC dataset into low- and high-risk groups. The subtype analysis revealed that the distribution of C1 was significantly correlated with numerous factors, including age, sex, pathological T stage, TNM classification, and grade staging. Further, the KEGG analysis indicated that the genes that were upregulated in the high-risk C1 group were primarily engaged in pathways related to the cell cycle, DNA replication, cellular senescence, ECM-receptor interactions, and mechanisms of mismatch repair. Conversely, the 90 genes that were downregulated were mainly associated with metabolic pathways, chemical carcinogenesis involving DNA adducts, complement and coagulation cascades, and the PPAR signaling pathway. The resultant boxplots revealed significant differences in the populations of immune cells, such as B cells, endothelial cells, NK cells, macrophages, CD4+ T cells, and CD8+ T cells, in C1 compared to C2. Additionally, the prognostic outcomes of the HCC patients were predicted using a cellular senescence-related gene model that included VDAC2, CXCL8, MYBL2, RAD9A, LIN52, RHEB, GADD45G, E2F5, MAP2K2, CDC25A, PPP1CB, and HRAS.
Recent research has revealed a significant correlation between cellular senescence, and the established pathways of carcinogenesis, tumor progression, and the invasive behavior of HCC. Cellular senescence is defined as a permanent halt in cellular division (11). Numerous therapeutic approaches can induce senescence in cancerous cells via mechanisms, such as genotoxic stress, augmented mitogenic signaling, and oxidative stress, which results in a definitive interruption of the cell cycle (10-12). Our research indicates that senescence-related genes may indeed contribute to tumor progression through the SASP. The SASP is characterized by the secretion of various pro-inflammatory cytokines, growth factors, and proteases that can modify the tumor microenvironment (10-12). This secretory phenotype has the potential to promote tumor growth, invasion, and metastasis by enhancing cellular proliferation and survival of adjacent tumor cells. Furthermore, components of the SASP can recruit immune cells to the tumor site, which might initially have a tumor-suppressive effect but can also lead to immune evasion strategies employed by the cancer cells over time (10-12). Despite substantial research, the precise function of cellular senescence in HCC remains inadequately understood. The prognostic outcomes of HCC patients were predicted using a model comprising 12 cellular senescence-related genes (i.e., VDAC2, CXCL8, MYBL2, RAD9A, LIN52, RHEB, GADD45G, E2F5, MAP2K2, CDC25A, PPP1CB, and HRAS). Additionally, this model can serve as a complementary tool to traditional staging systems, helping to refine prognosis and enabling better risk stratification. We envision that with further validation in larger cohort studies, our 12-gene model could be incorporated into clinical guidelines, assisting healthcare providers in making informed decisions that improve patient outcomes.
Celastrol liposomes promote ferroptosis and apoptosis via the direct modulation of the voltage-dependent anion channel 2 (VDAC2) in HCC (14). C-X-C motif ligand 8 (CXCL8) serves as a chemokine that functions as a crucial multifunctional cytokine involved in regulating tumor growth, invasion, and migration (15). The transcription factor MYB proto-oncogene like 2 (MYBL2) facilitates the enhancement of both proliferation and metastasis in bladder cancer via the transactivation of CDCA3 (16). The silencing of cell-cycle checkpoint control protein RAD9A augments the DNA damage elicited by trichostatin A in esophageal cancer cell lines (17). MicroRNA-146a affects advanced gastric cancer patients’ sensitivity to chemotherapy and prognosis by modulating the expression of LIN52 (18). RHEB enhances the viability of cancer cells via the p27Kip1-mediated stimulation of autophagy (19). 4-methoxydalbergione induces anti-cancer activity via the upregulation of GADD45G in human HCC cells (20). E2F5 enhances the proliferation and invasion of gastric cancer by directly increasing the transcription of UBE2T (21). CDC25A plays a role in obstructing autophagy-driven ferroptosis by enhancing the expression of ErbB2 via the dephosphorylation of PKM2 in cervical cancer cells (22). Histone methyltransferase NSD3 plays a critical role in inhibiting the glycolytic process in lung adenocarcinoma by engaging with PPP1CB (23). Notably, our senescence-related gene signature (SRGS) holds particular promise for optimizing patient stratification in systemic therapy. Recent clinical studies highlight capecitabine—an oral fluoropyrimidine prodrug—as an emerging option for advanced HCC patients refractory to first-line therapies (24-26). Mechanistically, capecitabine induces tumor cell senescence through thymidylate synthase inhibition and DNA damage response activation (26), mirroring key pathways enriched in our SRGS-high subgroup (e.g., p16INK4a/CDKN2A upregulation and SASP factor overexpression). Further functional validation and screening will be necessary to determine the efficacy and specificity of targeting these genes. Our findings lay a foundation for future studies aimed at exploring these therapeutic avenues in the context of HCC treatment.
It is essential to acknowledge the limitations of this study. Further in vitro and in vivo analyses of the 12 cellular senescence-related genes are required to validate our findings. It is essential to validate the model using independent datasets to enhance its generalizability. Future research plan to conduct a comprehensive analysis of existing patient databases to evaluate the correlation between identified molecular subtypes and clinical outcomes. By stratifying patients based on these subtypes, we can assess overall survival, disease-free survival, and response to treatment, which will help to reinforce the prognostic value of our molecular classifications. Additionally, future research should take into account the underlying molecular mechanisms pertinent to this study.
Conclusions
In summary, this study found a significant correlation between cellular senescence-related genes, and tumor classification and the immune response in patients with HCC. The cellular senescence-related signature established could serve as promising prognostic tools for HCC. Our findings may lead to developments in diagnostic and therapeutic approaches for HCC.
Acknowledgments
None.
Footnote
Reporting Checklist: The authors have completed the TRIPOD reporting checklist. Available at https://tcr.amegroups.com/article/view/10.21037/tcr-2025-335/rc
Peer Review File: Available at https://tcr.amegroups.com/article/view/10.21037/tcr-2025-335/prf
Funding: None.
Conflicts of Interest: All authors have completed the ICMJE uniform disclosure form (available at https://tcr.amegroups.com/article/view/10.21037/tcr-2025-335/coif). The authors have no conflicts of interest to declare.
Ethical Statement: The authors are accountable for all aspects of the work in ensuring that questions related to the accuracy or integrity of any part of the work are appropriately investigated and resolved. The study was conducted in accordance with the Declaration of Helsinki (as revised in 2013).
Open Access Statement: This is an Open Access article distributed in accordance with the Creative Commons Attribution-NonCommercial-NoDerivs 4.0 International License (CC BY-NC-ND 4.0), which permits the non-commercial replication and distribution of the article with the strict proviso that no changes or edits are made and the original work is properly cited (including links to both the formal publication through the relevant DOI and the license). See: https://creativecommons.org/licenses/by-nc-nd/4.0/.
References
- Petrick JL, Kelly SP, Altekruse SF, et al. Future of Hepatocellular Carcinoma Incidence in the United States Forecast Through 2030. J Clin Oncol 2016;34:1787-94. [Crossref] [PubMed]
- Llovet JM, Kelley RK, Villanueva A, et al. Hepatocellular carcinoma. Nat Rev Dis Primers 2021;7:6. [Crossref] [PubMed]
- Kim E, Viatour P. Hepatocellular carcinoma: old friends and new tricks. Exp Mol Med 2020;52:1898-907. [Crossref] [PubMed]
- Mittal S, El-Serag HB. Epidemiology of hepatocellular carcinoma: consider the population. J Clin Gastroenterol 2013;47:S2-6. [Crossref] [PubMed]
- Llovet JM, Ricci S, Mazzaferro V, et al. Sorafenib in advanced hepatocellular carcinoma. N Engl J Med 2008;359:378-90. [Crossref] [PubMed]
- Llovet JM, Montal R, Sia D, et al. Molecular therapies and precision medicine for hepatocellular carcinoma. Nat Rev Clin Oncol 2018;15:599-616. [Crossref] [PubMed]
- Zhang S, Li X, Zhang X, et al. The Pyroptosis-Related Gene Signature Predicts the Prognosis of Hepatocellular Carcinoma. Front Mol Biosci 2021;8:781427. [Crossref] [PubMed]
- Nault JC, Ningarhari M, Rebouissou S, et al. The role of telomeres and telomerase in cirrhosis and liver cancer. Nat Rev Gastroenterol Hepatol 2019;16:544-58. [Crossref] [PubMed]
- Hanahan D. Hallmarks of Cancer: New Dimensions. Cancer Discov 2022;12:31-46. [Crossref] [PubMed]
- Liu P, Tang Q, Chen M, et al. Hepatocellular Senescence: Immunosurveillance and Future Senescence-Induced Therapy in Hepatocellular Carcinoma. Front Oncol 2020;10:589908. [Crossref] [PubMed]
- Birch J, Gil J. Senescence and the SASP: many therapeutic avenues. Genes Dev 2020;34:1565-76. [Crossref] [PubMed]
- Wang L, Lankhorst L, Bernards R. Exploiting senescence for the treatment of cancer. Nat Rev Cancer 2022;22:340-55. [Crossref] [PubMed]
- Dai L, Wang X, Bai T, et al. Cellular Senescence-Related Genes: Predicting Prognosis in Gastric Cancer. Front Genet 2022;13:909546. [Crossref] [PubMed]
- Luo P, Zhang Q, Shen S, et al. Mechanistic engineering of celastrol liposomes induces ferroptosis and apoptosis by directly targeting VDAC2 in hepatocellular carcinoma. Asian J Pharm Sci 2023;18:100874. [Crossref] [PubMed]
- Liu Q, Li A, Tian Y, et al. The CXCL8-CXCR1/2 pathways in cancer. Cytokine Growth Factor Rev 2016;31:61-71. [Crossref] [PubMed]
- Liu W, Shen D, Ju L, et al. MYBL2 promotes proliferation and metastasis of bladder cancer through transactivation of CDCA3. Oncogene 2022;41:4606-17. [Crossref] [PubMed]
- Pang X, He G, Luo C, et al. Knockdown of Rad9A enhanced DNA damage induced by trichostatin A in esophageal cancer cells. Tumour Biol 2016;37:963-70. [Crossref] [PubMed]
- Luo Z, Li X, Zhao Z, et al. MicroRNA-146a affects the chemotherapeutic sensitivity and prognosis of advanced gastric cancer through the regulation of LIN52. Oncol Lett 2017;13:1386-92. [Crossref] [PubMed]
- Campos T, Ziehe J, Palma M, et al. Rheb promotes cancer cell survival through p27Kip1-dependent activation of autophagy. Mol Carcinog 2016;55:220-9. [Crossref] [PubMed]
- Zeng L, Qin Y, Lu X, et al. 4-Methoxydalbergione Elicits Anticancer Effects by Upregulation of GADD45G in Human Liver Cancer Cells. J Healthc Eng 2023;2023:6710880. [Crossref] [PubMed]
- Li L, Liu J, Huang W. E2F5 promotes proliferation and invasion of gastric cancer through directly upregulating UBE2T transcription. Dig Liver Dis 2022;54:937-45. [Crossref] [PubMed]
- Wang C, Zeng J, Li LJ, et al. Cdc25A inhibits autophagy-mediated ferroptosis by upregulating ErbB2 through PKM2 dephosphorylation in cervical cancer cells. Cell Death Dis 2021;12:1055. [Crossref] [PubMed]
- Zhou Y, Peng X, Fang C, et al. Histones Methyltransferase NSD3 Inhibits Lung Adenocarcinoma Glycolysis Through Interacting with PPP1CB to Decrease STAT3 Signaling Pathway. Adv Sci (Weinh) 2024;11:e2400381. [Crossref] [PubMed]
- Trevisani F, Brandi G, Garuti F, et al. Metronomic capecitabine as second-line treatment for hepatocellular carcinoma after sorafenib discontinuation. J Cancer Res Clin Oncol 2018;144:403-14. [Crossref] [PubMed]
- Granito A, Marinelli S, Terzi E, et al. Metronomic capecitabine as second-line treatment in hepatocellular carcinoma after sorafenib failure. Dig Liver Dis 2015;47:518-22. [Crossref] [PubMed]
- Altieri P, Murialdo R, Barisione C, et al. 5-fluorouracil causes endothelial cell senescence: potential protective role of glucagon-like peptide 1. Br J Pharmacol 2017;174:3713-26. [Crossref] [PubMed]