Prediction of the prognosis of intrahepatic cholangiocarcinoma patients after hepatectomy via propensity score matching: a competitive risk model analysis
Highlight box
Key findings
• This study developed a competing-risk-based nomogram to predict 1-, 2-, and 3-year cancer-specific survival (CSS) rates for intrahepatic cholangiocarcinoma (ICCA) patients post-hepatectomy. The nomogram showed moderate predictive performance following internal validation.
What is known and what is new?
• Traditional nomogram models commonly employ Cox regression for survival estimation; yet, studies utilizing competing risks to evaluate the outcomes of ICCA patients post-hepatectomy are notably lacking.
• In this study, we excluded the interference of competing events and successfully constructed a CSS nomogram for ICCA patients after hepatectomy.
What is the implication, and what should change now?
• The nomogram can effectively predict the prognosis of ICCA patients after hepatectomy, identify high-risk populations, and provide insights for optimizing future personalized treatment strategies.
Introduction
Intrahepatic cholangiocarcinoma (ICCA) is a rare malignancy whose global incidence is gradually increasing (1,2). In its early phases, ICCA often remains asymptomatic, yet its aggressive nature is evident, as indicated by a 5-year overall survival (OS) rate of approximately 9% (3). Although surgical resection remains the primary treatment for ICCA, the heterogeneity of this tumor in terms of anatomy, molecular characteristics, pathology, and clinical presentation poses significant challenges to the accurate prediction of postoperative prognosis (4). The tumor node metastasis (TNM) system represents the predominant classification for staging ICCA. Although studies have evaluated the prognostic value of the 8th edition of the TNM classification for ICCA, its superiority in stratifying patient OS remains inconclusive (5,6). Recently, the nomogram has gained prominence as an advanced instrument for individualized prognostic prediction, increasingly acknowledged for its enhanced precision in forecasting patient outcomes. However, current nomogram models primarily utilize the Cox regression model for proportional hazards (7,8), which has notable limitations in addressing competing risks. Traditional models inadequately account for competing risk events, such as mortality from other causes during the postoperative period, potentially resulting in an overestimation of prognostic risk in patients with ICCA. This limitation can adversely impact the scientific rigor and precision of clinical decision-making. In contrast, the competing risk model provides a broader evaluation of patient prognosis by integrating other causes of postoperative mortality into its analysis, thereby enhancing the accuracy of risk assessments (9). At present, studies related to the prognosis of ICCA patients after hepatectomy by competing risk models are insufficient, and these studies are limited by sample size and population range (10).
Accordingly, this study aimed to construct a nomogram based on a model that accounts for competing risks, thereby improving the predictive precision of posthepatectomy outcomes for ICCA patients, using a comprehensive dataset from the Surveillance, Epidemiology, and End Results (SEER) database. This model provides a scientific and reliable foundation for the formulation of individualized treatment plans and postoperative follow-up strategies for ICCA patients. We present this article in accordance with the TRIPOD reporting checklist (available at https://tcr.amegroups.com/article/view/10.21037/tcr-24-1819/rc).
Methods
Data source and patients
For this study, the patient data were sourced from SEER Research Data [17 registries, November 2023 submission (2000–2021)]. Specifically, clinicopathological data from 2004 to 2015 were taken from the SEER*Stat database (version 8.4.3) for this retrospective analysis. This study included patients with a histological code of 8,160/3, with tumour primary sites in the liver and intrahepatic bile ducts according to the International Classification of Disease for Oncology third edition (ICD-O-3)/World Health Organization (WHO) in 2008 (n=8,705). The inclusion criteria were as follows: (I) had a pathologically confirmed diagnosis of ICCA; (II) had undergone hepatectomy; and (III) had complete data. The exclusion criteria were as follows: (I) were under 18 years of age; (II) reported sources limited only to death certificates or autopsy reports; (III) had a survival time of less than 1 month; and (IV) had unavailable (NA) or unknown data. The study was conducted in accordance with the Declaration of Helsinki (as revised in 2013). As the SEER database is openly available and all participant data are anonymized, ethical review board approval was not necessary for this study.
Study variables and outcomes
The study examined numerous variables, including sex, age, race, grade, marital status, tumor size, T stage, N stage, M stage, lymph node dissection (LND), radiation, and chemotherapy. Age was stratified into two categories: less than 60 years or older than 60 years. Tumor size was classified into three categories: less than 5 cm, greater than or equal to 5 cm but less than 10 cm, and greater than or equal to 10 cm. Marital status was divided into three categories: single, married (which encompassed common-law partnerships), and other (including divorced, widowed, and separated). The participants were assigned to one of four histological grade-based groups: grade I (well differentiated), grade II (moderately differentiated), grade III (poorly differentiated), or grade IV (undifferentiated). Chemotherapy and radiotherapy were recorded in the SEER database as binary categories (‘yes’ or ‘no/unknown’), without further differentiation between neoadjuvant, adjuvant, or perioperative use. The primary endpoint of the study was cancer-specific survival (CSS), defined as the time span commencing at ICCA diagnosis and ending with ICCA-related mortality, with fatalities attributable to other causes classified as competing events. The definition of OS encompassed the time span from the diagnosis of ICCA to death due to any cause.
Construction and validation of competitive risk nomogram
Patients extracted from the SEER database were randomly allocated to training (70%) or test (30%) cohorts. Using the training cohort, the cumulative incidence function (CIF) was utilized to conduct univariate variance analyses, and intergroup differences were assessed via Gray’s test. Variables demonstrating statistical significance in the initial univariate assessments were subsequently incorporated into the multivariate analysis, and independent prognostic indicators for patients with ICCA after hepatectomy were identified via the Fine-Gray model (11). A competing risk nomogram was developed incorporating the significant prognostic variables identified in the training cohort, aiming to predict 1-, 2-, and 3-year CSS rates in ICCA surgery patients. The nomogram’s predictive accuracy was subsequently confirmed in the test cohort.
Statistical analysis
R software (version 4.4.1) was utilized for conducting the statistical analyses, with descriptive statistics expressed in terms of counts and proportionate representations. Comparisons between groups were evaluated via the Chi-squared test, supplemented by Fisher’s exact test when necessary. The model’s discriminatory power and predictive accuracy were evaluated via receiver operating characteristic (ROC) curves, the area under the curve (AUC), and calibration plots. Furthermore, decision curve analysis (DCA) was employed to determine the clinical utility of the nomogram. The X-tile software was used to categorize patients into three distinct risk groups (low, medium, and high) based on the scores derived from the nomogram, to construct the risk stratification model (12). Kaplan-Meier (KM) curves and log-rank tests were then utilized to perform survival analyses, comparing the differences in survival among these groups (13). Additionally, the use of propensity score matching (PSM) analysis helps to reduce bias in the study. The primary R packages utilized in the preceding analysis were “survival”, “survminer”, “MatchIt”, “rms”, “riskRegression”, “cmprsk”, “mstate”, “prodlim”, “regplot”, “timeROC”, “QHScrnomo” and “nomogramFormula”. Statistical significance was set at a two-tailed P value of less than 0.05.
Results
Baseline patient characteristics
This study included a preliminary analysis of 1,299 ICCA patients who underwent liver resection between 2004 and 2015, as recorded in the SEER database, to evaluate their prognostic outcomes. The cohort comprised 47.19% male and 52.81% female participants. A significant proportion of the patients were elderly (≥60 years), comprising 64.59% of the cohort, and 63.28% were married, including those in common-law relationships. The racial distribution of patients revealed a lower representation of White and Black individuals, with the majority (80.52%) belonging to other racial groups, including American Indians, Alaskan Natives, Asians, and Pacific Islanders. We consolidated grade III and grade IV cases into a single category, grade III/IV, which represented 32.72% of the cases. A total of 54.50% of patients who underwent hepatectomy had LND. Additionally, 13.63% of the patients received radiotherapy, and 42.03% received chemotherapy. PSM was employed to harmonize baseline information disparities between the training and test cohorts, utilizing a 1:2 matching ratio with the nearest-neighbor approach within a caliper of 0.2. The PSM analysis demonstrated equivalence in baseline characteristics between the cohorts, with P values exceeding the 0.05 threshold. Ultimately, this study included 1,131 ICCA patients after PSM for further analytical evaluation. Comprehensive details are available in Table 1.
Table 1
Variables | Before PSM | After PSM | ||||||
---|---|---|---|---|---|---|---|---|
Total (n=1,299) | Test (n=390) | Train (n=909) | P | Test (n=340) | Train (n=791) | P | ||
Sex | 0.99 | 0.40 | ||||||
Female | 686 (52.81) | 206 (52.82) | 480 (52.81) | 174 (51.18) | 426 (53.86) | |||
Male | 613 (47.19) | 184 (47.18) | 429 (47.19) | 166 (48.82) | 365 (46.14) | |||
Age (years) | 0.21 | 0.89 | ||||||
<60 | 460 (35.41) | 148 (37.95) | 312 (34.32) | 119 (35.00) | 280 (35.40) | |||
≥60 | 839 (64.59) | 242 (62.05) | 597 (65.68) | 221 (65.00) | 511 (64.60) | |||
Race | 0.28 | 0.99 | ||||||
Black | 82 (6.31) | 19 (4.87) | 63 (6.93) | 23 (6.76) | 53 (6.70) | |||
Other | 1,046 (80.52) | 323 (82.82) | 723 (79.54) | 276 (81.18) | 644 (81.42) | |||
White | 171 (13.16) | 48 (12.31) | 123 (13.53) | 41 (12.06) | 94 (11.88) | |||
Marital | 0.005 | 0.36 | ||||||
Single | 162 (12.47) | 63 (16.15) | 99 (10.89) | 46 (13.53) | 114 (14.41) | |||
Married | 822 (63.28) | 223 (57.18) | 599 (65.90) | 191 (56.18) | 470 (59.42) | |||
Other | 315 (24.25) | 104 (26.67) | 211 (23.21) | 103 (30.29) | 207 (26.17) | |||
Grade | 0.042 | 0.38 | ||||||
I | 149 (11.47) | 58 (14.87) | 91 (10.01) | 45 (13.24) | 102 (12.90) | |||
II | 725 (55.81) | 210 (53.85) | 515 (56.66) | 173 (50.88) | 436 (55.12) | |||
III/IV | 425 (32.72) | 122 (31.28) | 303 (33.33) | 122 (35.88) | 253 (31.98) | |||
LND | 0.76 | 0.67 | ||||||
No | 591 (45.50) | 175 (44.87) | 416 (45.76) | 154 (45.29) | 369 (46.65) | |||
Yes | 708 (54.50) | 215 (55.13) | 493 (54.24) | 186 (54.71) | 422 (53.35) | |||
Radiation | 0.74 | 0.35 | ||||||
No/unknown | 1,122 (86.37) | 335 (85.90) | 787 (86.58) | 300 (88.24) | 682 (86.22) | |||
Yes | 177 (13.63) | 55 (14.10) | 122 (13.42) | 40 (11.76) | 109 (13.78) | |||
Chemotherapy | 0.46 | 0.13 | ||||||
No/unknown | 753 (57.97) | 232 (59.49) | 521 (57.32) | 211 (62.06) | 453 (57.27) | |||
Yes | 546 (42.03) | 158 (40.51) | 388 (42.68) | 129 (37.94) | 338 (42.73) | |||
Size (cm) | 0.49 | 0.54 | ||||||
<5 | 568 (43.73) | 175 (44.87) | 393 (43.23) | 145 (42.65) | 346 (43.74) | |||
≥5 and <10 | 552 (42.49) | 168 (43.08) | 384 (42.24) | 153 (45.00) | 332 (41.97) | |||
≥10 | 179 (13.78) | 47 (12.05) | 132 (14.52) | 42 (12.35) | 113 (14.29) | |||
T-stage | 0.11 | 0.82 | ||||||
T1 | 566 (43.57) | 166 (42.56) | 400 (44.00) | 141 (41.47) | 352 (44.50) | |||
T2 | 320 (24.63) | 95 (24.36) | 225 (24.75) | 88 (25.88) | 194 (24.53) | |||
T3 | 258 (19.86) | 70 (17.95) | 188 (20.68) | 68 (20.00) | 149 (18.84) | |||
T4 | 155 (11.93) | 59 (15.13) | 96 (10.56) | 43 (12.65) | 96 (12.14) | |||
N-stage | 0.30 | 0.76 | ||||||
No | 1,057 (81.37) | 324 (83.08) | 733 (80.64) | 279 (82.06) | 643 (81.29) | |||
Yes | 242 (18.63) | 66 (16.92) | 176 (19.36) | 61 (17.94) | 148 (18.71) | |||
M-stage | 0.36 | 0.33 | ||||||
No | 1,199 (92.30) | 356 (91.28) | 843 (92.74) | 317 (93.24) | 724 (91.53) | |||
Yes | 100 (7.70) | 34 (8.72) | 66 (7.26) | 23 (6.76) | 67 (8.47) | |||
Survival status | – | – | ||||||
Alive | 275 (21.17) | 76 (19.49) | 199 (21.89) | 71 (20.88) | 163 (20.61) | |||
Dead of ICCA | 837 (64.43) | 257 (65.90) | 580 (63.81) | 221 (65.00) | 518 (65.49) | |||
Dead of other causes | 187 (14.40) | 57 (14.62) | 130 (14.30) | 48 (14.12) | 110 (13.91) |
Data are presented as n (%). Chi-squared tests were used to compare groups, and P<0.05 were considered statistically significant. PSM, propensity score matching; LND, lymph node dissection; ICCA, intrahepatic cholangiocarcinoma.
Survival analysis
Before PSM, ICCA patients who underwent hepatectomy had a median OS of 34 months [95% confidence interval (CI): 32–37 months; Figure 1A] and a median CSS of 41 months (95% CI: 37–45 months; Figure 1B). Following PSM adjustment, the median OS was 33 months (95% CI: 30–36 months; Figure 1C), and the median CSS was 39 months (95% CI: 35–43 months; Figure 1D). Before PSM, 21.17% of the patients survived, 64.43% died from ICCA, and 14.40% died from other causes. According to the PSM analysis, the survival rate was 20.69%, with 65.34% of patients dying from ICCA and 13.97% from other causes (Table 1). In addition, the OS rates for patients were 79.4%, 59.8%, and 46.4% at 1, 2, and 3 years, respectively, while the CSS rates were 82.5%, 64.0%, and 51.3%, respectively (Table 2).
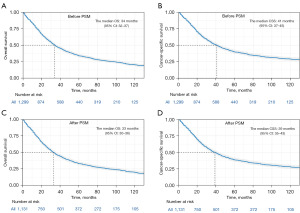
Table 2
Study endpoint | 1-year | 2-year | 3-year |
---|---|---|---|
OS | 79.4% (77.0–81.8%) | 59.8% (57.1–62.8%) | 46.4% (43.6–49.4%) |
CSS | 82.5% (80.2–84.7%) | 64.0% (61.2–66.9%) | 51.3% (48.3–54.4%) |
Data are presented as percentage (95% CI). CI, confidence interval; OS, overall survival; CSS, cancer-specific survival; ICCA, intrahepatic cholangiocarcinoma; PSM, propensity score matching.
Competitive risk analysis of CSS
A univariate analysis of CSS in the context of competing risk factors indicated that variables, including grade, LND, radiotherapy, chemotherapy, size, and TNM staging, were significantly correlated with CSS following hepatectomy in ICCA patients (Table 3). Figure 2 shows the associated CIF curves. Variables with statistical significance in the univariate competing risk analyses were included in the subsequent multivariate analysis. The analysis revealed that histological grade, T stage, N stage, and M stage significantly influenced survival outcomes in ICCA patients following hepatectomy (Table 3). Specifically, advanced tumor grade (II vs. I: HR =1.21, 95% CI: 0.906–1.620; III/IV vs. I: HR =1.67, 95% CI: 1.233–2.280), elevated T stage (T2 vs. T1: HR =1.42, 95% CI: 1.143–1.77; T3 vs. T1: HR =1.53, 95% CI: 1.187–1.97; T4 vs. T1: HR =1.80, 95% CI: 1.306–2.47), and the presence of N1 (HR =1.671; 95% CI: 1.330–2.097) and M1 stages (HR =1.548; 95% CI: 1.086–2.206) were significantly correlated with increased risk of CSS in ICCA patients following hepatectomy.
Table 3
Variables | Univariate analysis | Multivariate analysis | |||
---|---|---|---|---|---|
Gray’s test | P | HR (95% CI) | P | ||
Sex | 0.401 | 0.52 | |||
Female | – | – | |||
Male | – | – | |||
Age (years) | 1.878 | 0.17 | |||
<60 | – | – | |||
≥60 | – | – | |||
Race | 0.586 | 0.74 | |||
Black | – | – | |||
Other | – | – | |||
White | – | – | |||
Marital | 0.443 | 0.80 | |||
Single | – | – | |||
Married | – | – | |||
Other | – | – | |||
Grade | 35.161 | <0.001 | |||
I | Reference | ||||
II | 1.21 (0.906–1.620) | 0.20 | |||
III/IV | 1.67 (1.233–2.280) | 0.001 | |||
LND | 11.482 | <0.001 | |||
No | Reference | ||||
Yes | 1.03 (0.843–1.258) | 0.78 | |||
Radiation | 5.972 | 0.01 | |||
No/unknown | Reference | ||||
Yes | 1.20 (0.944–1.53) | 0.13 | |||
Chemotherapy | 19.187 | <0.001 | |||
No/unknown | Reference | ||||
Yes | 1.08 (0.886–1.33) | 0.43 | |||
Size (cm) | 21.342 | <0.001 | |||
<5 | Reference | ||||
≥5 and <10 | 1.20 (0.990–1.47) | 0.06 | |||
≥10 | 1.05 (0.787–1.41) | 0.72 | |||
T-stage | 70.077 | <0.001 | |||
T1 | Reference | ||||
T2 | 1.42 (1.143–1.77) | 0.002 | |||
T3 | 1.53 (1.187–1.97) | 0.001 | |||
T4 | 1.80 (1.306–2.47) | <0.001 | |||
N-stage | 69.054 | <0.001 | |||
No | Reference | ||||
Yes | 1.671 (1.330–2.097) | <0.001 | |||
M-stage | 32.838 | <0.001 | |||
No | Reference | ||||
Yes | 1.548 (1.086–2.206) | 0.01 |
ICCA, intrahepatic cholangiocarcinoma; CSS, cancer-specific survival; HR, hazard ratio; CI, confidence interval; LND, lymph node dissection.
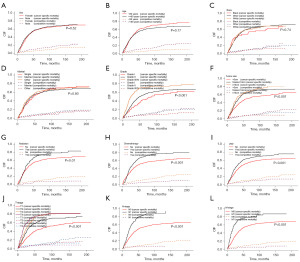
Construction of the CSS nomogram and the risk stratification model
In this study, a nomogram for CSS was constructed based on independent prognostic factors to estimate the 1-, 2-, and 3-year survival rates following hepatectomy for ICCA patients (Figure 3A). Utilizing the same set of independent prognostic factors, a nomogram for predicting OS was also developed (Figure 3B). As depicted in Figure 3A,3B, a significant discrepancy was observed in the predicted survival risks between the CSS and OS nomograms. Patients were successfully categorized into low-, intermediate-, and high-risk groups on the basis of the prognostic scores derived from the nomogram, utilizing cutoff points of 38 and 158 for risk stratification model. A notable difference in survival outcomes was identified across the various risk groups (P<0.001), confirming the high accuracy of the nomogram in determining the patients’ level of risk for death (Figure 3C,3D).
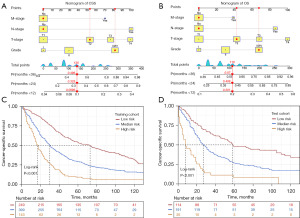
Validation of the nomogram
The 1-, 2-, and 3-year CSS prediction AUCs in the training cohort were 0.668 (95% CI: 0.619–0.716), 0.711 (95% CI: 0.673–0.749), and 0.710 (95% CI: 0.675–0.746), respectively (Figure 4A). The test cohort exhibited AUCs of 0.709 (95% CI: 0.635–0.783), 0.718 (95% CI: 0.661–0.775), and 0.721 (95% CI: 0.666–0.775), respectively, for the same CSS predictions (Figure 4B), highlighting the moderate discriminative ability of the nomogram. The time-dependent 5-year AUC curves of the nomogram further corroborated its predictive performance across various time points (Figure 4C,4D). Furthermore, the calibration curves revealed a high level of agreement between the nomogram-predicted survival rates and the actual observed rates (Figure 5). The nomogram provided significant clinical benefit and outperformed the sole use of histological grade and the TNM staging system, as illustrated by the DCA plots (Figure 6).
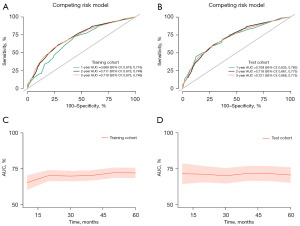
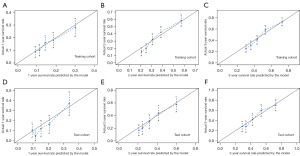
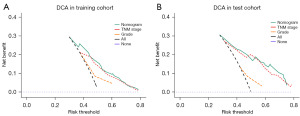
Chemotherapy and radiation: do they improve survival in ICCA?
Although hepatectomy is the chief treatment for ICCA, high postoperative recurrence rates and significant prognostic differences still present major clinical challenges. Therefore, it is particularly important to deeply explore other effective treatment methods and optimise clinical management. In this study, we conducted a survival analysis of 1,131 ICCA patients who underwent hepatectomy, evaluating the effects of chemotherapy and radiotherapy. To enhance the reliability of the findings and minimize potential bias, we applied the PSM method. Variables with a P value <0.05 were matched using the “nearest neighbor” method, with a caliper width of 0.2 and a matching ratio of 1:1. Additionally, density plots were generated (Figure 7A,7B) to visualize the data distribution before and after PSM, with detailed data presented in Tables 4,5. Survival analyses of the PSM-matched ICCA patients revealed that neither radiotherapy (Figure 7C) nor chemotherapy (Figure 7D) significantly improved patient survival outcomes.
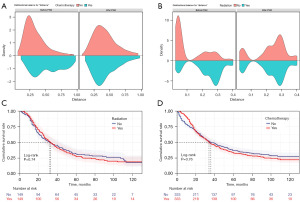
Table 4
Variables | Before PSM | After PSM | |||||||
---|---|---|---|---|---|---|---|---|---|
Total (n=1,131) | Non-chemotherapy (n=664) | Chemotherapy (n=467) | P | Total (n=666) | Non-chemotherapy (n=333) | Chemotherapy (n=333) | P | ||
Sex | 0.02 | 0.69 | |||||||
Female | 600 (53.05) | 334 (50.30) | 266 (56.96) | 367 (55.11) | 181 (54.35) | 186 (55.86) | |||
Male | 531 (46.95) | 330 (49.70) | 201 (43.04) | 299 (44.89) | 152 (45.65) | 147 (44.14) | |||
Age (years) | <0.001 | 0.42 | |||||||
<60 | 399 (35.28) | 186 (28.01) | 213 (45.61) | 248 (37.24) | 119 (35.74) | 129 (38.74) | |||
≥60 | 732 (64.72) | 478 (71.99) | 254 (54.39) | 418 (62.76) | 214 (64.26) | 204 (61.26) | |||
Race | 0.11 | 0.46 | |||||||
Black | 76 (6.72) | 53 (7.98) | 23 (4.93) | 31 (4.65) | 13 (3.90) | 18 (5.41) | |||
Other | 920 (81.34) | 530 (79.82) | 390 (83.51) | 565 (84.83) | 288 (86.49) | 277 (83.18) | |||
White | 135 (11.94) | 81 (12.20) | 54 (11.56) | 70 (10.51) | 32 (9.61) | 38 (11.41) | |||
Marital | 0.01 | 0.93 | |||||||
Single | 160 (14.15) | 90 (13.55) | 70 (14.99) | 105 (15.77) | 54 (16.22) | 51 (15.32) | |||
Married | 661 (58.44) | 371 (55.87) | 290 (62.10) | 398 (59.76) | 199 (59.76) | 199 (59.76) | |||
Other | 310 (27.41) | 203 (30.57) | 107 (22.91) | 163 (24.47) | 80 (24.02) | 83 (24.92) | |||
Grade | 0.006 | 0.97 | |||||||
I | 147 (13.00) | 95 (14.31) | 52 (11.13) | 73 (10.96) | 36 (10.81) | 37 (11.11) | |||
II | 609 (53.85) | 373 (56.17) | 236 (50.54) | 343 (51.50) | 173 (51.95) | 170 (51.05) | |||
III/IV | 375 (33.16) | 196 (29.52) | 179 (38.33) | 250 (37.54) | 124 (37.24) | 126 (37.84) | |||
LND | <0.001 | 0.93 | |||||||
No | 523 (46.24) | 350 (52.71) | 173 (37.04) | 279 (41.89) | 140 (42.04) | 139 (41.74) | |||
Yes | 608 (53.76) | 314 (47.29) | 294 (62.96) | 387 (58.11) | 193 (57.96) | 194 (58.26) | |||
Radiation | <0.001 | 0.15 | |||||||
No/unknown | 982 (86.83) | 636 (95.78) | 346 (74.09) | 599 (89.94) | 305 (91.59) | 294 (88.29) | |||
Yes | 149 (13.17) | 28 (4.22) | 121 (25.91) | 67 (10.06) | 28 (8.41) | 39 (11.71) | |||
Size (cm) | <0.001 | 0.44 | |||||||
<5 | 491 (43.41) | 321 (48.34) | 170 (36.40) | 255 (38.29) | 131 (39.34) | 124 (37.24) | |||
≥5 and <10 | 485 (42.88) | 277 (41.72) | 208 (44.54) | 305 (45.80) | 155 (46.55) | 150 (45.05) | |||
≥10 | 155 (13.70) | 66 (9.94) | 89 (19.06) | 106 (15.92) | 47 (14.11) | 59 (17.72) | |||
T stage | <0.001 | 0.85 | |||||||
T1 | 493 (43.59) | 337 (50.75) | 156 (33.40) | 249 (37.39) | 121 (36.34) | 128 (38.44) | |||
T2 | 282 (24.93) | 166 (25.00) | 116 (24.84) | 180 (27.03) | 89 (26.73) | 91 (27.33) | |||
T3 | 217 (19.19) | 96 (14.46) | 121 (25.91) | 145 (21.77) | 77 (23.12) | 68 (20.42) | |||
T4 | 139 (12.29) | 65 (9.79) | 74 (15.85) | 92 (13.81) | 46 (13.81) | 46 (13.81) | |||
N stage | <0.001 | 0.92 | |||||||
N0 | 922 (81.52) | 580 (87.35) | 342 (73.23) | 521 (78.23) | 261 (78.38) | 260 (78.08) | |||
N1 | 209 (18.48) | 84 (12.65) | 125 (26.77) | 145 (21.77) | 72 (21.62) | 73 (21.92) | |||
M stage | <0.001 | 0.57 | |||||||
M0 | 1,041 (92.04) | 626 (94.28) | 415 (88.87) | 610 (91.59) | 303 (90.99) | 307 (92.19) | |||
M1 | 90 (7.96) | 38 (5.72) | 52 (11.13) | 56 (8.41) | 30 (9.01) | 26 (7.81) |
Data are presented as n (%). Chi-squared tests were used to compare groups, and P<0.05 were considered statistically significant. PSM, propensity score matching; LND, lymph node dissection.
Table 5
Variables | Before PSM | After PSM | |||||||
---|---|---|---|---|---|---|---|---|---|
Total (n=1,131) | Non-radiation (n=982) | Radiation (n=149) | P | Total (n=298) | Non-radiation (n=149) | Radiation (n=149) | P | ||
Sex | 0.005 | >0.99 | |||||||
Female | 600 (53.05) | 505 (51.43) | 95 (63.76) | 190 (63.76) | 95 (63.76) | 95 (63.76) | |||
Male | 531 (46.95) | 477 (48.57) | 54 (36.24) | 108 (36.24) | 54 (36.24) | 54 (36.24) | |||
Age (years) | 0.008 | 0.90 | |||||||
<60 | 399 (35.28) | 332 (33.81) | 67 (44.97) | 135 (45.30) | 68 (45.64) | 67 (44.97) | |||
≥60 | 732 (64.72) | 650 (66.19) | 82 (55.03) | 163 (54.70) | 81 (54.36) | 82 (55.03) | |||
Race | 0.66 | 0.86 | |||||||
Black | 76 (6.72) | 68 (6.92) | 8 (5.37) | 14 (4.70) | 6 (4.03) | 8 (5.37) | |||
Other | 920 (81.34) | 795 (80.96) | 125 (83.89) | 252 (84.56) | 127 (85.23) | 125 (83.89) | |||
White | 135 (11.94) | 119 (12.12) | 16 (10.74) | 32 (10.74) | 16 (10.74) | 16 (10.74) | |||
Marital | 0.99 | 0.84 | |||||||
Single | 160 (14.15) | 139 (14.15) | 21 (14.09) | 39 (13.09) | 18 (12.08) | 21 (14.09) | |||
Married | 661 (58.44) | 574 (58.45) | 87 (58.39) | 178 (59.73) | 91 (61.07) | 87 (58.39) | |||
Other | 310 (27.41) | 269 (27.39) | 41 (27.52) | 81 (27.18) | 40 (26.85) | 41 (27.52) | |||
Grade | 0.87 | 0.38 | |||||||
I | 147 (13.00) | 129 (13.14) | 18 (12.08) | 39 (13.09) | 21 (14.09) | 18 (12.08) | |||
II | 609 (53.85) | 526 (53.56) | 83 (55.70) | 154 (51.68) | 71 (47.65) | 83 (55.70) | |||
III/IV | 375 (33.16) | 327 (33.30) | 48 (32.21) | 105 (35.23) | 57 (38.26) | 48 (32.21) | |||
LND | 0.08 | 0.90 | |||||||
No | 523 (46.24) | 464 (47.25) | 59 (39.60) | 117 (39.26) | 58 (38.93) | 59 (39.60) | |||
Yes | 608 (53.76) | 518 (52.75) | 90 (60.40) | 181 (60.74) | 91 (61.07) | 90 (60.40) | |||
Chemotherapy | <0.001 | >0.99 | |||||||
No/unknown | 664 (58.71) | 636 (64.77) | 28 (18.79) | 56 (18.79) | 28 (18.79) | 28 (18.79) | |||
Yes | 467 (41.29) | 346 (35.23) | 121 (81.21) | 242 (81.21) | 121 (81.21) | 121 (81.21) | |||
Size (cm) | 0.56 | 0.92 | |||||||
<5 | 491 (43.41) | 431 (43.89) | 60 (40.27) | 121 (40.60) | 61 (40.94) | 60 (40.27) | |||
≥5 and <10 | 485 (42.88) | 420 (42.77) | 65 (43.62) | 127 (42.62) | 62 (41.61) | 65 (43.62) | |||
≥10 | 155 (13.70) | 131 (13.34) | 24 (16.11) | 50 (16.78) | 26 (17.45) | 24 (16.11) | |||
T stage | 0.02 | 0.83 | |||||||
T1 | 493 (43.59) | 439 (44.70) | 54 (36.24) | 114 (38.26) | 60 (40.27) | 54 (36.24) | |||
T2 | 282 (24.93) | 245 (24.95) | 37 (24.83) | 68 (22.82) | 31 (20.81) | 37 (24.83) | |||
T3 | 217 (19.19) | 188 (19.14) | 29 (19.46) | 58 (19.46) | 29 (19.46) | 29 (19.46) | |||
T4 | 139 (12.29) | 110 (11.20) | 29 (19.46) | 58 (19.46) | 29 (19.46) | 29 (19.46) | |||
N stage | 0.03 | 0.89 | |||||||
N0 | 922 (81.52) | 810 (82.48) | 112 (75.17) | 225 (75.50) | 113 (75.84) | 112 (75.17) | |||
N1 | 209 (18.48) | 172 (17.52) | 37 (24.83) | 73 (24.50) | 36 (24.16) | 37 (24.83) | |||
M stage | 0.78 | 0.02 | |||||||
M0 | 1,041 (92.04) | 903 (91.96) | 138 (92.62) | 264 (88.59) | 126 (84.56) | 138 (92.62) | |||
M1 | 90 (7.96) | 79 (8.04) | 11 (7.38) | 34 (11.41) | 23 (15.44) | 11 (7.38) |
Data are presented as n (%). Chi-squared tests were used to compare groups, and P<0.05 were considered statistically significant. PSM, propensity score matching; LND, lymph node dissection.
Discussion
Currently, hepatectomy remains the only potentially curative treatment option for patients with ICCA (14). However, ICCA is often diagnosed at advanced stages, with approximately 20–30% of patients meeting surgical criteria at diagnosis (3). Despite undergoing curative resection, most patients experience disease recurrence, resulting in a poor prognosis (15-17). Therefore, developing an accurate prognostic model to predict postoperative outcomes in ICCA patients is crucial. The aim of this study is to develop a prognostic model based on a nomogram to predict CSS after surgery, thereby providing valuable guidance for clinical decision-making. In this study, we employed a competitive risk analysis method to mitigate the impact of other competing events and identified four independent prognostic factors—histological grade, T stage, N stage, and M stage. These factors were used to construct a nomogram for predicting CSS in ICCA patients after hepatectomy. The results demonstrated that the nomogram exhibited moderate discriminatory ability and performed well in calibration. Furthermore, DCA further indicated that, compared to the traditional TNM staging system and degree of tumour differentiation, the nomogram model developed in this study offers greater clinical utility. Through the risk stratification system based on the nomogram, we can effectively categorize the postoperative risks of patients, significantly enhancing risk discrimination. More importantly, this system provides valuable support for personalized clinical decision-making, particularly in identifying high- and moderate-risk patient groups. Based on this model, clinicians can adopt more tailored follow-up strategies and, in the case of early recurrence or metastasis, promptly implement effective interventions, thereby significantly improving patients’ survival outcomes. In this process, PSM played a critical role. By performing PSM matching on the dataset, baseline characteristic differences between the two groups were successfully balanced, mitigating the impact of selection bias, thereby enhancing the reliability and robustness of the prognostic model. Although external validation was not performed in this study, the use of PSM improved the model’s applicability and generalizability across different clinical settings, further reinforcing its clinical value.
This study identified four independent prognostic factors for ICCA patients following hepatectomy: histological grade, T stage, N stage, and M stage. These factors are widely recognized in the literature as key determinants of survival in ICCA patients. The higher histological grade typically reflects a lower degree of differentiation and increased invasiveness of the tumor, which is commonly associated with poorer survival outcomes (18). This study also found that poorer tumor differentiation was linked to worse survival prognosis, consistent with existing literature (19). Similarly, the T stage is another important factor in predicting the prognosis of ICCA. Advanced-stage (T3/T4) tumors, due to invasion into adjacent structures or vessels, usually demonstrate significantly poorer survival outcomes, which is consistent with previous research (20). ICCA is characterized by significant local spread and early lymphatic metastasis, and lymph node positivity typically suggests tumor progression with an increased likelihood of distant metastasis, further contributing to a worse prognosis (21). This study also confirmed that lymph node metastasis is an independent risk factor for postoperative prognosis in ICCA patients, a finding consistent with the research of Rhee et al. (22). Furthermore, the M stage, indicating the presence of distant metastasis, is another key factor affecting the prognosis of ICCA. Patients with distant metastasis generally have a shorter survival period, often accompanied by increased tumor resistance to treatment and disease progression. The results of this study showed that patients with M1 stage had a poorer survival prognosis, which is also in line with the findings of Yuan et al. (23).
Currently, the clinical value of LND in ICCA remains a subject of debate (24,25). A systematic review and meta-analysis (26) showed that clinical node-negative ICCA patients who underwent LND had improved OS compared to those who did not undergo LND. Another systematic review and meta-analysis (25) pointed out that the efficacy of routine LND in ICC treatment has not been confirmed. In this study, LND was included as a variable in the analysis, but the results indicated that LND did not have a significant impact on the prognosis of ICCA patients. This finding may be attributed to the lack of detailed clinicopathological data in the SEER database, which limited our ability to comprehensively assess the role of LND in the prognosis of ICCA patients. Nevertheless, it is noteworthy that LND plays a critical role in accurate staging, subsequent interventions, and follow-up care. As such, many clinical guidelines recommend regional LND as a standard procedure for ICCA hepatectomy (27,28). Furthermore, the surgical margin status has a significant impact on the postoperative prognosis of ICCA patients. R0 resection (complete tumor resection with no residual cancer cells at the margin under microscopic examination) is generally associated with better survival outcomes. In contrast, R1 resection (microscopic residual tumor at the margin) and R2 resection (macroscopic residual tumor) are linked to higher recurrence rates and poorer prognosis (29,30). However, due to the limitations of the SEER database, this study was unable to fully assess the impact of surgical margin status on the prognosis of ICCA patients.
Due to the low resectability, high recurrence risk, and poor long-term prognosis of intrahepatic cholangiocarcinoma (ICC) patients, adjuvant therapy has become an important strategy to improve the prognosis of patients with intermediate to advanced stages. Currently, relevant guidelines (30) recommend chemotherapy and radiotherapy as key adjuvant treatment strategies for ICC. However, the survival benefit of adjuvant chemotherapy following hepatectomy for ICC remains controversial. Additionally, there is limited prospective research on adjuvant chemotherapy for ICC patients after hepatectomy, with relevant clinical trials still ongoing (3). Radiotherapy is primarily used for treating localized non-metastatic disease, particularly in patients who are not candidates for surgical resection, and can also be employed for symptom relief in patients with metastatic disease. In this study, no significant survival difference was observed between ICC patients who received chemotherapy or radiotherapy and those who did not. This finding may be related to the incomplete data on chemotherapy and radiotherapy in the SEER database, particularly the lack of key information such as the timing and regimen of treatments. Due to the absence of these detailed data, an accurate assessment of the survival benefit of chemotherapy and radiotherapy could not be conducted.
In conclusion, although this study provides important insights into the postoperative prognosis of ICCA, several limitations exist. The retrospective study design may introduce selection bias, and the SEER database lacks key prognostic factors such as viral hepatitis and detailed postoperative pathological information, which may affect the accuracy of the results. Future prospective studies should incorporate more comprehensive clinical data, particularly treatment details and surgical margin information, to further evaluate the true impact of these factors on ICCA prognosis and provide more precise guidance for individualized treatment strategies.
Conclusions
In conclusion, this study demonstrates the feasibility and clinical utility of a prognostic nomogram based on the competitive risk model for assessing the specific survival outcomes of ICCA patients following hepatectomy. While the model provides a reliable tool for predicting individual patient prognosis, further prospective studies incorporating additional prognostic factors, such as surgical margin status, are warranted to refine its predictive capabilities and enhance its clinical applicability.
Acknowledgments
We express our deep appreciation to the Surveillance, Epidemiology, and End Results (SEER) Program of the NCI for the invaluable data that facilitated our research.
Footnote
Reporting Checklist: The authors have completed the TRIPOD reporting checklist. Available at https://tcr.amegroups.com/article/view/10.21037/tcr-24-1819/rc
Peer Review File: Available at https://tcr.amegroups.com/article/view/10.21037/tcr-24-1819/prf
Funding: This study was funded by
Conflicts of Interest: All authors have completed the ICMJE uniform disclosure form (available at https://tcr.amegroups.com/article/view/10.21037/tcr-24-1819/coif). The authors have no conflicts of interest to declare.
Ethical Statement: The authors are accountable for all aspects of the work in ensuring that questions related to the accuracy or integrity of any part of the work are appropriately investigated and resolved. The study was conducted in accordance with the Declaration of Helsinki (as revised in 2013). Considering the SEER database is openly available and all participant data are anonymized, ethical review board approval was not necessary for this study.
Open Access Statement: This is an Open Access article distributed in accordance with the Creative Commons Attribution-NonCommercial-NoDerivs 4.0 International License (CC BY-NC-ND 4.0), which permits the non-commercial replication and distribution of the article with the strict proviso that no changes or edits are made and the original work is properly cited (including links to both the formal publication through the relevant DOI and the license). See: https://creativecommons.org/licenses/by-nc-nd/4.0/.
References
- Wei T, Lu J, Xiao XL, et al. Classification of Intrahepatic Cholangiocarcinoma into Perihilar Versus Peripheral Subtype. Ann Surg Oncol 2024;31:1232-42. [PubMed]
- Yang JQ, Wang XG, Wu B. Incidence trend and prognosis of intrahepatic cholangiocarcinoma: a study based on the SEER database. Transl Cancer Res 2023;12:3007-15. [PubMed]
- Moris D, Palta M, Kim C, et al. Advances in the treatment of intrahepatic cholangiocarcinoma: An overview of the current and future therapeutic landscape for clinicians. CA: A Cancer Journal for Clinicians 2023;73:198-222. [Crossref] [PubMed]
- Bragazzi MC, Venere R, Ribichini E, et al. Intrahepatic cholangiocarcinoma: Evolving strategies in management and treatment. Digestive and Liver Disease 2024;56:383-93. [PubMed]
- Yamamoto Y, Sugiura T, Okamura Y, et al. The Evaluation of the Eighth Edition of the AJCC/UICC Staging System for Intrahepatic Cholangiocarcinoma: a Proposal of a Modified New Staging System. Journal of Gastrointestinal Surgery 2020;24:786-95.
- Kim Y, Moris DP, Zhang XF, et al. Evaluation of the 8th edition American Joint Commission on Cancer (AJCC) staging system for patients with intrahepatic cholangiocarcinoma: A surveillance, epidemiology, and end results (SEER) analysis. J Surg Oncol 2017;116:643-50.
- Sun Y, Liu B, Shen H, et al. Cox model risk score to predict survival of intrahepatic cholangiocarcinoma after ultrasound-guided ablation. Abdominal Radiology 2024;49:1653-63. [PubMed]
- Jeong S, Luo G, Gao Q, et al. A combined Cox and logistic model provides accurate predictive performance in estimation of time-dependent probabilities for recurrence of intrahepatic cholangiocarcinoma after resection. Hepatobiliary surgery and nutrition 2021;10:464-75. [PubMed]
- Wang T, Zhou J, Zheng Q, et al. A Competing Risk Model Nomogram to Predict the Long-Term Prognosis of Lung Carcinoid. Annals of Surgical Oncology 2023;30:5830-9. [PubMed]
- Wang T, Zhang J, Wang W, et al. Development and Validation of Nomograms for Predicting Cancer-Specific Survival in Elderly Patients with Intrahepatic Cholangiocarcinoma After Liver Resection: A Competing Risk Analysis. Cancer Management and Research 2020;12:11015-29. [PubMed]
- Putter H, Fiocco M, Geskus RB. Tutorial in biostatistics: competing risks and multi‐state models. Statistics in Medicine 2007;26:2389-430. [PubMed]
- Camp RL, Dolled-Filhart M, Rimm DL. X-Tile. Clinical Cancer Research 2004;10:7252-9. [PubMed]
- Andrade C. Survival Analysis, Kaplan-Meier Curves, and Cox Regression: Basic Concepts. Indian journal of psychological medicine 2023;45:434-5. [PubMed]
- Valle JW, Kelley RK, Nervi B, et al. Biliary tract cancer. Lancet 2021;397:428-44. [Crossref] [PubMed]
- Xian F, Song X, Bie J, et al. Development and Validation of Nomograms to Predict the Overall Surviva l and Progression-Free Survival in Patients with Advanced Unresectable Intrahepatic Cholangiocarcinoma. Cancer Management and Research 2024;16:1835-49. [Crossref] [PubMed]
- Huang L, Li J, Zhu S, et al. Machine learning-based prognostic prediction and surgical guidance for intrahepatic cholangiocarcinoma. BioScience Trends 2024; [Crossref] [PubMed]
- Wei T, Zhang XF, He J, et al. Prognostic impact of perineural invasion in intrahepatic cholangiocarcinoma: multicentre study. Br J Surg 2022;109:610-6. [Crossref] [PubMed]
- Song J, Zhou H, Gu D, et al. Hepatocellular Carcinoma Differentiation: Research Progress in Mechani sm and Treatment. Frontiers in Oncology 2022;11. [Crossref]
- He C, Cai Z, Zhang Y, et al. Prognostic Model to Predict Cancer-Specific Survival for Patients With Gallbladder Carcinoma After Surgery: A Population-Based Analysis. Front Oncol 2019;9:1329. [Crossref] [PubMed]
- Yang F, Wu C, Bo Z, et al. The clinical value of regional lymphadenectomy for intrahepatic cholangiocarcinoma. Asian J Surg 2022;45:376-80. [PubMed]
- Wang J, Shu M, Peng H, et al. The influence of the extent of lymph node metastasis on the prognosis for patients with intrahepatic cholangiocarcinoma. Ann Surg Treat Res 2023;104:258-68. [Crossref] [PubMed]
- Rhee H, Lim HJ, Han K, et al. A preoperative scoring system to predict lymph node metastasis in intrahepatic cholangiocarcinoma. Hepatol Int 2023;17:942-53. [Crossref] [PubMed]
- Yuan C, Zou S, Wang K, et al. Establishment and external validation of prognosis prediction nomogram for patients with distant metastatic intrahepatic cholangiocarcinoma: based on a large population. BMC cancer 2024;24:227. [Crossref] [PubMed]
- Chen C, Su J, Wu H, et al. Prognostic value of lymphadenectomy in node-negative intrahepatic cholangiocarcinoma: A multicenter, retrospectively study. Eur J Surg Oncol 2023;49:780-7. [Crossref] [PubMed]
- Ishii T, Iwaki K, Nakakura A, et al. Is routine lymph node dissection recommended for liver resection of intrahepatic cholangiocarcinoma? A systematic review and meta-analysis. HPB (Oxford) 2024;26:731-40. [Crossref] [PubMed]
- Yeow M, Fong KY, Zhao JJ, et al. Value of lymph node dissection in intrahepatic cholangiocarcinoma: a systematic review and meta-analysis. HPB (Oxford) 2024;26:161-70. [Crossref] [PubMed]
- Fong ZV, Brownlee SA, Qadan M, et al. The Clinical Management of Cholangiocarcinoma in the United States and Europe: A Comprehensive and Evidence-Based Comparison of Guidelines. Ann Surg Oncol 2021;28:2660-74. [Crossref] [PubMed]
- Benson AB, D’Angelica MI, Abbott DE, et al. Hepatobiliary Cancers, Version 2.2021, NCCN Clinical Practice Guidelin es in Oncology. Journal of the National Comprehensive Cancer Network 2021;19:541-65. [PubMed]
- Ejaz A, Cloyd JM, Pawlik TM. Advances in the Diagnosis and Treatment of Patients with Intrahepatic Cholangiocarcinoma. Ann Surg Oncol 2020;27:552-60. [Crossref] [PubMed]
- Liver EAftSot. EASL-ILCA Clinical Practice Guidelines on the management of intrahepatic cholangiocarcinoma. J Hepatol 2023;79:181-208. [Crossref] [PubMed]