Investigation of mitochondrial DNA methylation-related prognostic biomarkers in hepatocellular carcinoma using The Cancer Genome Atlas (TCGA) database
Highlight box
Key findings
• Based on a bioinformatics analysis, this study identified ADH4 and DNASE1L3 as key prognostic genes associated with mitochondrial DNA methylation (MTDM) in hepatocellular carcinoma (HCC). A risk model was then constructed based on these genes to effectively categorize HCC patients into high- and low-risk groups, and the results showed that the overall survival of the high-risk group was lower than that of the low-risk group. The study also identified different immune cell infiltration profiles and drug sensitivity characteristics between the two groups, highlighting potential therapeutic targets and biomarkers for HCC.
What is known, and what is new?
• Despite advances in the understanding of the pathogenesis of HCC, the role of MTDM in HCC remains poorly understood. Previous studies have focused on nuclear DNA methylation; however, the effect of MTDM on mitochondrial function and cancer progression has not yet been fully explored.
• This study sought to address this gap in the literature by exploring MTDM-related genes using public databases. Our findings provide novel insights into the prognostic value of these genes and the molecular mechanisms of HCC.
What is the implication, and what should change now?
• This study provides a comprehensive understanding of the role of MTDM in HCC, which has important implications for prognosis, immunomodulation, and personalized therapy. The identification of ADH4 and DNASE1L3 as prognostic markers paves the way for targeted therapy. Further research will be conducted to validate these findings in larger populations and explore the functional mechanisms of these genes in HCC progression to translate these insights into clinical applications.
Introduction
Hepatocellular carcinoma (HCC) is the most prevalent type of primary liver cancer (1). It accounts for 75–85% of all deaths from primary liver cancer, and is the third-leading cause of cancer-related death worldwide (1). In the early stages, HCC patients are often asymptomatic; however, as the disease progresses, symptoms such as hepatic pain, weight loss, jaundice, and ascites may occur, along with complications such as tumor rupture hemorrhage and hepatic encephalopathy (2). The occurrence of HCC is associated with various factors, such as hepatotropic virus infections, aflatoxin exposure, heavy alcohol consumption, excess body weight, type 2 diabetes, and smoking (3). Genetic and epigenetic aberrations are also common in HCC. Epigenetic processes, including chromatin remodeling, histone changes, DNA methylation, and non-coding RNA expression, are linked to the progression and metastasis of HCC (4).
Surgical resection or hepatectomy has long been the main potentially curative radical treatment for HCC. However, at approximately 50–70%, the postoperative 5-year survival rate of HCC patients remains relatively low; thus, more effective therapeutic approaches need to be established (5,6). Currently, emerging therapies for HCC are being extensively investigated, but their clinical translation and therapeutic efficacy require further validation. Prospective epidemiological models project that HCC—which accounts for 75–90% of primary liver cancers—will reach one million annual cases globally by 2025, with patients affected by chronic liver diseases (e.g., hepatitis B/C, metabolic dysfunction-associated steatotic liver disease) exhibiting a markedly elevated risk, particularly those progressing to cirrhosis (3).
In the context of chronic liver disease, persistent hepatocyte injury and ongoing inflammatory responses disrupt normal cellular processes. This leads to an imbalance between cell proliferation and apoptosis, genome instability, and increased oxidative stress, ultimately promoting the transformation of normal hepatocytes into cancer cells (7). Therefore, the identification of informative prognostic genes is of great importance to the early diagnosis, pathogenesis research, and the development of therapeutic targets for HCC.
Mitochondrial DNA methylation (MTDM) is an epigenetic modification involving the addition of methyl groups to mitochondrial DNA. The most common form is the formation of 5-methylcytosine on cytosine residues, which is mediated by DNA methyltransferases using S-adenosylmethionine as the methyl donor (8). MTDM regulates mitochondrial gene transcription and replication. Through bidirectional interactions with the nuclear genome, these two systems reciprocally modulate their respective gene expression profiles and metabolic responses (9). By regulating proteins related to mitochondrial function, MTDM affects normal mitochondrial metabolism and function maintenance, thereby playing a role in the development and progression of mitochondrial function-related diseases (10). Alterations in MTDM have been observed in many tumors. In liver cancer stem cells, MTDM-mediated glycolytic metabolic reprogramming can promote self-renewal and immune escape (11). In lung adenocarcinoma, a study has found that abnormal DNA methylation in circulating tumor cells is associated with tumor metastasis (12). In breast cancer, such epigenetic dysregulation promotes tumor cell proliferation and malignant progression (13). Additionally, changes in MTDM levels are associated with alterations in cancer cell behavior and the prognosis of patients (14). However, the specific role of MTDM in HCC remains unclear.
This study used transcriptome data from public databases and bioinformatics approaches to delve into the prognostic value and potential molecular regulatory mechanisms of MTDM-related genes in HCC. Specifically, HCC datasets and MTDM-related HCC clinicopathological information from various databases available from the University of Santa Cruz (UCSC) Xena and the International Cancer Genome Consortium (ICGC) data portals were collected. Through a series of analyses, including a differential gene expression analysis, cluster analysis, and Cox regression analysis, candidate genes were identified, and a risk model for HCC was constructed. This study aimed to gain new insights to improve the clinical prognosis of HCC patients. We present this article in accordance with the TRIPOD reporting checklist (available at https://tcr.amegroups.com/article/view/10.21037/tcr-2025-546/rc).
Methods
Data source
The study was conducted in accordance with the Declaration of Helsinki (as revised in 2013). RNA sequencing (RNA-seq) data and HCC clinical information were downloaded from the UCSC Xena (https://xenabrowser.net/datapages/) database. The liver hepatocellular carcinoma (LIHC) dataset from The Cancer Genome Atlas (TCGA) database (i.e., TCGA-LIHC dataset) comprised 362 HCC tumor tissue samples and 50 adjacent tissue samples, and included the survival information, and clinical characteristics of the patients, and was used to construct the prognostic model in this study. The Liver Cancer-RIKEN, JP project (LIRI-JP) dataset obtained from the ICGC (https://dcc.icgc.org/) database comprised the tumor samples and survival information of 231 HCC patients, and was used to validate the prognostic model. TCGA-LIHC was used to identify the differentially expressed genes (DEGs). Additionally, eight MTDM-related genes were identified in the literature (13).
Identification of the DEGs and key subtype DEGs
R software was used to generate the expression matrix of the DEGs in TCGA-LIHC dataset. The gene expression data were log 2 converted and background corrected using the “DESeq2” package (version 1.42.0) (15), and the DEGs between the HCC and control samples were identified based on the following criteria: |log2 fold change (FC)| >0.5 and an adjusted P value <0.05. Additionally, the R ggplot2 package (version 3.4.4) (16) was used to draw a volcano plot and heatmap of the top 10 upregulated and downregulated DEGs.
The ConsensusClusterPlus package (version 1.66.0) (17) was used for the unsupervised clustering analysis. Based on the expression levels of the eight MTDM-related genes for each HCC sample in TCGA-LIHC dataset and according to the proportion of the principal component analysis (PCA), different molecular subtypes associated with HCC were identified. Additionally, to assess the survival data obtained from TCGA-LIHC cohort clusters, the R survminer package (version 0.4.9) (https://CRAN.R-project.org/package=survminer) was used to perform the survival analysis of these data to generate Kaplan-Meier survival curves for the HCC-associated subtypes. Differences in overall survival (OS) between the HCC-associated subtypes were assessed using the log-rank test, and the two subtypes with the most significant differences in terms of OS were selected and defined as the key subtypes for the subsequent analyses to identify informative prognostic genes by assigning the HCC patients into high- and low-risk groups based on the DEGs and clinical features.
Based on TCGA-LIHC dataset, the two isoforms obtained in the previous step were grouped as subgroups, and the DEGs of the key subtypes were identified using the DESeq2 package (version 1.42.0) in the R environment based on the following filtering criteria: P adjusted value <0.05 and |log2 FC| >2.
Candidate gene identification and enrichment analysis
To identify the candidate genes, the key isoform DEGs and DEGs were intersected using the R package ggVennDiagram (version 1.2.1) (18). To identify the biological pathways of the upregulated and downregulated candidate genes associated with HCC, including the metabolic and signaling pathways, and to explore the functions and mechanisms in which they were involved, clusterProfiler R package (version 4.10.0) (19) was used to perform the Gene Ontology (GO) and Kyoto Encyclopedia of Genes and Genomes (KEGG) pathway enrichment analyses (P<0.05). The GO enrichment analysis included the following three non-overlapping categories: molecular function (MF), cellular component (CC), and biological process (BP).
Weighted gene co-expression network analysis (WGCNA)
To identify genes associated with MTDM, we used MTDM score as a trait and performed WGCNA analysis on tumor samples from the training set using the R package “WGCNA” (version 1.71) (20). First, we calculated the MTDM score for tumor samples in the training set using the ssGSEA algorithm from the “GSVA” package (version1.46.0) (21). We then divided the samples into high and low score groups based on the optimal threshold (2.98), followed by plotting the Kaplan-Meier curve to determine if there were significant differences between the high and low score groups (P<0.05) (22). Next, we performed clustering on the samples and excluded outliers to ensure the accuracy of the analysis. After determining the optimal soft-thresholding power, we set the minimum number of genes in each gene module to be 100 according to the standard of dynamic tree cutting. MEDissThres was set to 0.4 to merge similar modules identified by the dynamic tree cut algorithm. Finally, to identify the modules most strongly correlated with the MTDM score, we analyzed the correlation between each module and the MTDM score, and plotted a heatmap of module-trait correlations by “ggplot2” R package (version 3.5.1) (|cor| >0.4 and P<0.05) (23).
Building and validating predictive models
To identify the informative prognostic genes associated with the prognosis of HCC patients, the HCC samples in TCGA-LIHC dataset were divided at a ratio of 7:3, such that seven copies of TCGA-LIHC dataset were used as the training set in this study, and three copies of TCGA-LIHC dataset were used as the internal validation set in this study. Based on the candidate genes, the “survival” R package (version 3.5-7) (24) was used to perform the univariate Cox hazard regression analysis to calculate the hazard ratios (HRs) and P values (HR ≠1 and P<0.05) in the training set. To identify the survival-associated genes, a univariate Cox hazard regression analysis was conducted to identify the genes that passed the proportional hazard (PH) assumption test (P<0.05), and a forest plot was plotted using the “forestplot” R package (version 2.0.1) (25) to visualize the results. To construct the risk model, identify the prognostic genes, and prevent the model from overfitting, a least absolute shrinkage and selection operator (LASSO) logistic regression analysis of the prognostic gene data from the training set was conducted using the “glmnet” R package (version 4.1-8) (26), and the prognostic genes were ultimately identified by a multivariate Cox regression analysis. The risk model was based on the prognostic genes, and the risk score of developing HCC was calculated using the following formula: .
Based on the optimum cut-off value for the risk assessment, the HCC training set patients in the inner validation set and validation set were allocated to high- and low-risk groups. Risk profiles, scatter plots, and heat maps of the expression of the prognostic genes were then plotted according to the risk groupings. The patients were classified as high and low risk to produce the populations of the prognostic genetic expression training set, and the Kaplan-Meier survival curves were analyzed using the log-rank test. To enhance the robustness of the predictive models, 1-, 2-, and 3-year survival receiver operating characteristic (ROC) curves were constructed using the “survivalROC” R package (version 1.0.3.1) (27).
Independent prognostic analysis of risk scores and clinical relevance analysis
First, the clinicopathological features of the tumor samples (n=253) from TCGA-LIHC training dataset, including the follow-up time, were included in the univariate Cox regression analysis (P<0.05, HR ≠1) to determine the risk scores and the independent prognosis of the clinicopathological features. Next, the significant clinical characteristics from the univariate analysis were included in the multivariate Cox regression analysis, and the stepwise regression method was used to establish the optimal regression model and construct the multivariate Cox regression model with equal proportional risk assumptions to obtain the final clinical features (P<0.05, HR ≠1). Next, based on the independent prognostic features identified by the multivariate Cox independent prognostic analysis, the independent prediction model of the clinical characteristics was constructed, and the cph function in the rms package (version 6.7-1) in R (https://CRAN.R-project.org/package=rms) was used to draw a column-line graph to visualize the predictive model and predict the likelihood of survival rates of the patients at 1, 3, and 5 years of follow-up. Additionally, the validity of the column charts was confirmed by plotting the overall calibration curves and decision curve analysis (DCA) curves using the R rms package (version 6.7-1) and ggDCA package (version 1.2) (28). To further assess the applicability of the independent prognostic models, ROC curves of the clinical data were plotted using the survivalROC R package with 1, 3, and 5 years as the survival time nodes [area under the curve (AUC) of the ROC curve >0.6]. The distribution of the different types of clinical characteristics between the high- and low-risk groups was also analyzed using the chi-square test (P<0.05).
Immune cell infiltration
In the training set, the single-sample gene set enrichment analysis (ssGSEA) algorithm (version 1.1.0) (29) was used to estimate the relative infiltrating scores of 28 immune cells in each sample, and the distribution and difference in the immune system infiltrating the hazardous and non-hazardous cell groups were found to be statistically significant. To identify the differential immune cells, the Wilcoxon signed rank-sum test was used to analyze the differences in the immune system cells between the high- and low-risk groups, and significant differences were found between the high- and low-risk groups. The immune cells identified as differential immune cells were subjected to further analysis using P<0.05 as the threshold. A Spearman’s correlation analysis between each prognostic gene and the differentiated cells of the immune system was then conducted to examine the correlation between the prognostic genes and immune cells that differed from the others using the psych R package (version 2.2.9) (30) and the following criteria: |cor| >0.3 and P value <0.05. Next, the Wilcoxon rank-sum test (wilcox.test) was used to compare the immune checkpoint genes between the high- and low-risk groups (P<0.05), and the comparisons were visualized using box-and-line plots drawn using the ggpubr R package (version 1.1.0) (29).
Gene set enrichment analysis (GSEA)
To examine the differences between the high- and low-risk groups in terms of the biological functions and pathways, the DESeq2 package (version 1.42.0) was used to analyze differential gene expression between the two risk groups at low hazard-trained samples, and the log2FC was calculated, and the log2FC values were sorted and ordered in decreasing order. For the GSEA, the clusterProfiler R package (version 4.10.0) was used to identify the enriched pathways, and an adjusted P<0.05 was set as the significant enrichment screening threshold. The results were sorted according to their significance. Ultimately, based on their significance, the top five enriched pathways were selected for visualization.
Chemotherapy response and small-molecule drugs
In this study, the response of the HCC patients to the chemotherapeutic drugs was predicted using the Genomics of Drug Sensitivity in Cancer (GDSC) database (https://ngdc.cncb.ac.cn/databasecommons/database/). The response of the patients to the chemotherapeutic drugs was assessed by first calculating the half-maximal inhibitory concentration (IC50) using the pRRophetic R package. Next, the IC50 values between the low- and high-risk groups were compared to identify the drugs that differed significantly between the two groups based on a threshold P value <0.05.
Statistical analysis
All the data were analyzed using R language software (version 4.3.2). The comparisons were analyzed using the Wilcoxon test to determine statistical significance based on a P value <0.05.
Results
Identification of candidate genes
In this study, the DEGs in TCGA-LIHC dataset were analyzed, and 2,311 DEGs were identified, of which, 2,011 were upregulated and 300 were downregulated (Figure S1A,S1B). Consistent clustering analysis is commonly used in studies of cancer subtype classification. In this study, the clustering process was repeated 1,000 times based on HCC samples from TCGA-LIHC dataset, with 80% of the items sub-sampled, and each sample was assigned into groups using the k-means algorithm. The results were then visualized using consensus matrix plots and cumulative distribution function plots (Figure 1A,1B). Based on the above-determined optimal number of clusters, the HCC samples were classified into three subtypes defined as HCC-related subtypes to identify patterns and visualize the dispersion of samples between the HCC-related subtypes. The PCA revealed that the three subtypes were classified with higher accuracy (Figure 1C,1D). As the Kaplan-Meier survival curves for the three HCC-related subtypes show, the most significant difference in OS was observed between subtype 2 and subtype 3 (P<0.05), which were selected as the most important subtypes for the subsequent analyses (Figure 1E). The DEGs of the two subtypes were further analyzed, and 781 significant DEGs were identified, of which 258 were upregulated and 523 were downregulated (Figure 1F,1G). Thus, 2,311 DEGs were intersected with 781 key subtype DEGs to obtain 333 intersecting genes as candidates for the subsequent analyses (Figure 1H).

Functional enrichment of the 333 identified candidate genes
The 333 candidate genes were further subjected to a GO enrichment analysis, yielding 81 GO-BP entries, describing a wide range of cellular activities and locations (Table S1). For example, ranked in order of significance (P value in ascending order), the top four GO results were: pattern designation process, embryonic skeletal joint morphogenesis, regionalization, and cellular response to cadmium ions (Figure S2A,S2B). Additionally, 16 enriched KEGG pathways were identified (Table S2), including entries on mineral uptake, retinol metabolism, and drug metabolism-cytochrome P450 (Figure S2C,S2D).
Identification of key modules and genes associated with MTDM
To identify genes associated with MTDM, we used MTDM score as a trait and performed WGCNA analysis. The Kaplan-Meier curve indicated a significant difference in MTDM scores between the high and low score groups (P=0.03) (Figure S3A). The sample clustering results showed that there were no outlier samples that needed to be excluded (Figure S3B). Therefore, the remaining samples were used for subsequent analysis. When the soft-thresholding power was set to 12, the network closely resembled a distribution with no network scale (Figure S3C). After merging, a total of 9 modules were obtained (Figure S3D). The MEturquoise module (Cor =0.4, P<0.05) showed the strongest correlation with the MTDM score (Figure S3E).
Construction and validation of prognostic models
To examine the robust predictive power of the risk model, a follow-up analysis of the training set comprising the 333 candidate genes identified by the univariate Cox regression analysis was conducted. The results revealed that 57 genes, all of which passed the Proportional Hazards (PH) assumption test, were significantly associated with patient survival (P<0.05) (Figure 2A). The subsequent evaluation of the PH assumption test supported the predictive reliability of these 57 prognostic genes (P>0.05), which were further screened by a LASSO logistic regression analysis. Based on the best minimum value of lambda (lambda.min =0.1106), two prognostic genes were identified by a multivariate Cox regression analysis. Thus, alcohol dehydrogenase 4 (class II), pi polypeptide (ADH4) and deoxyribonuclease 1L3 (DNASE1L3) were identified as important prognostic indicator genes, and their risk ratios were calculated (Figure 2B,2C). Based on these results, the following improved risk score equation was developed: Risk Score = ho(t) * [ADH4 * (−0.09766) + DNASE1L3 * (−0.24361)]. This equation provides a quantitative tool for the prediction of HCC.
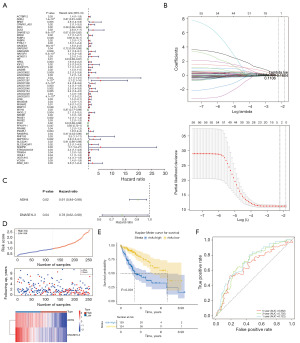
The optimal threshold of the training set was 0.958, and the final training set included 129 samples from the high-risk group and 124 samples from the low-risk group. The plotting of the risk graphs according to the risk grouping revealed that the survival rate and survival distribution of the low-risk group patients were better than those of the high-risk group patients (Figure 2D). In addition, Kaplan-Meier survival curves showing the OS rates of the high- and low-risk groups revealed significant differences in patient survival between the high- and low-risk groups (P<0.0001), with patients in the high-risk group surviving at a lower rate (Figure 2E). The AUC values for survival at 1, 3, and 5 years were all greater than 0.6, which provided further evidence of the validity of the risk prediction model (Figure 2F).
Similarly, the optimal threshold for the validation set was 0.812, while that for the internal validation set was 0.867. After assigning the samples to the high- and low-risk groups, and plotting risk maps based on the risk groupings, the results revealed that the survival rate and the survival rate distribution of the low-risk group were better than those of the high-risk group (Figure S4A,S4B). Further, there was a significant difference in the number of surviving patients between the high- and low-risk groups (P<0.001). The Kaplan-Meier survival curves also showed that patients in the high-risk group were much less likely to survive (Figure S4C,S4D), and had AUC values greater than 0.6 at 1, 3, and 5 years, which provided further evidence of the accuracy of the risk prediction model (Figure S4E,S4F).
Constructing a nomogram against independent prognostic factors
The clinicopathological characteristics of the tumor samples (n=253) from TCGA-LIHC cohort (training set), including the follow-up time, were included in a one-way Cox regression analysis, and a total of four factors (i.e., T stage, stage, risk score, and M stage) were found to be significant (P<0.05), and all passed the PH assumption test (Figure 3A). Subsequently, a multivariate Cox regression analysis with equal proportional risk assumptions was performed, and two clinical factors (i.e., T stage and risk score) were ultimately identified, both of which met the equal proportional risk assumptions (Figure 3B). A nomogram, which was plotted based on these two independent post-hoc factors, had a Concordance index of 0.713 and a good predictive effect (Figure 3C). In addition, after plotting the calibration curve with either side of the diagonal line, the slope of the 1-year calibration curve was 0.6766 (Figure 3D), that of the 3-year calibration curve was 0.4357, and that of the 5-year calibration curve was 0.363 (Figure 3E); the closer the slope to 1, the more accurate the model in its prediction. The survival ROC curve results at 1, 3, and 5 years also showed that the AUCs were greater than or equal to 0.6; thus, the independent prognostic model was able to account for the effect on survival outcomes (Figure 3F).
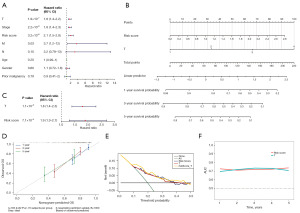
Significantly different clinical features between the high- and low-risk patients
The distribution of the different clinical characteristics between the high- and low-risk groups was analyzed using the chi-square test, and a significant difference was found in the distribution of the stage, T stage, and race between the high- and low-risk groups (P<0.05) (Figure 4A). The next three clinical features that exhibited significant differences were analyzed for their proportions in the high- and low-risk groups. Specifically, 236 samples (low risk =118, high risk =118) from TCGA-LIHC cohort (training set) (n=253) had T stage clinical feature information, of which I, II, III, and IV accounted for 50%, 24%, 24%, and 2%, respectively (Figure 4B). In addition, 251 samples (low risk =122, high risk =129) had T stage information, of which I, II, III, and IV accounted for 50%, 24%, 21%, and 5%, respectively (Figure 4C). Additionally, 246 samples (low risk =120, high risk =126) had Race clinical profile information, of which White, Asian, Black or African American, and American Indian or Alaska native accounted for 50%, 44%, 5%, and 0%, respectively (Figure 4D).
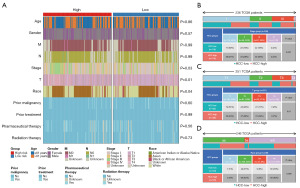
GSEA and immune infiltration analysis
We also investigated the potential biological differences between the patients in the high- and low-risk groups by GSEA, and found that the high- and low-risk groups exhibited significant differences in the enrichment of biological functions and signaling pathways, including fatty acid degradation and peroxisome (Figure 5A).
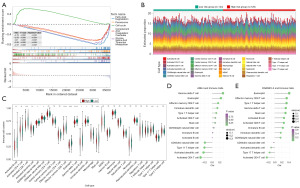
To evaluate the differences in the immune system infiltrating cells between the high- and low-risk groups, and determine whether the differences were statistically significant, 14 differential immune cells were screened (Figure 5B,5C). The results of the analysis of the differential immune cells and prognostic genes showed that the strongest negative correlation was between the ADH4 and activated CD4+ T cells (r=−0.311) (Figure 5D). The strongest positive correlation was between DNASE1L3 and eosinophils [correlation coefficient (r) =0.402], followed by that between DNASE1L3 and effector memory cluster of differentiation (CD)8+ T cells (r=0.332) (Figure 5E).
Comparative assessment and drug sensitivity analysis of the high- and low-risk patients
Differences in the ImmuneScore, StromalScore, and ESTIMATEScore between the high- and low-risk groups were compared using the Wilcoxon rank-sum test. The results revealed significant differences in the levels of ESTIMATEScore and StromalScore between TCGA-LIHC cohort (training set) samples (n=253) and between the high- and the low-risk groups (P<0.05) (Figure 6A). Eleven immune checkpoint genes showed significant differences in expression levels, including BTLA, HAVCR2, LGALS9, PDCD1LG2 and SIRPA, between the high- and low-risk groups (P<0.05). Notably, LGALS9 and SIRPA showed highly significant differences between the high- and low-risk groups (P<0.0001) (Figure 6B).
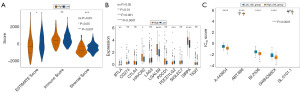
Based on the 138 drugs obtained, the IC50 value differences of each drug between the patients in the high- and low-risk groups were determined, and 48 drugs with significant differences were identified (P<0.0001), including BI.2536 [a Polo-like kinase 1 (Plk1) inhibitor], A.443654 [a protein kinase B (Akt) 1/2 inhibitor], and ABT.888 [Veliparib, a Poly(ADP-ribose) polymerase 1/2 (PARP1/2) inhibitor], indicating that there are still more choices of HCC-targeted drugs (Figure 6C and Table S3).
Discussion
Mitochondrial DNA (mtDNA) methylation networks exhibit significant heterogeneity across cell types and diseases, driven by dynamic interactions between control regions (e.g., D-loop, MT-ND1, and MT-CO2), enzymatic regulators (e.g., DNMT3A, TET2/3), and environmental or metabolic stressors. For instance, neuronal cells in neurodegenerative disorders show elevated D-loop methylation linked to mitochondrial dysfunction, while hepatocytes in HCC display MT-ND1 hypermethylation associated with ROS accumulation (31,32). Enzymes such as DNMT3A, which governs non-CpG methylation on the light chain, and TET2/3, which oxidize 5mC to 5hmC, are central to these processes but exhibit tissue-specific activity modulated by factors like hyperglycemia or pollutant exposure (e.g., PM2.5 exposure). To unravel these networks, multi-omics integration is critical: single-cell epigenomics can resolve cell-type-specific methylation patterns (e.g., neurons vs. β-cells) (31,33), spatial transcriptomics can map methylation sites to mitochondrial sub-compartments, and machine learning (e.g., dlGRN algorithms) can identify hidden regulators like non-coding RNAs or metabolites that fine-tune DNMT/TET activity (34,35). Key therapeutic and functional nodes include methyltransferases (DNMT3A as a “writer”), mt-rRNA modifications (e.g., 12S rRNA m5C sites influencing translation) (33,36), and cross-talk with nuclear pathways (e.g., SAM/SAH ratios regulating DNMT3A localization) (34,35). Targeting these hubs offers translational potential—selective DNMT3A inhibitors (e.g., RG108 analogs) could reverse pathological hypermethylation (31), while CRISPR-free base editors like DdCBEs enable precise mtDNA editing without double-strand breaks (32). Clinically, disease-specific methylation hotspots (e.g., MT-CO1 in cancer, D-loop in diabetes) may serve as liquid biopsy biomarkers, and links to immune microenvironments (e.g., HCC-associated H4 regulators) highlight broader therapeutic implications.
HCC is a highly prevalent and lethally malignant liver tumor, closely associated with factors such as hepatotropic virus infections, alcohol abuse, and non-alcoholic fatty liver disease (3). HCC poses a significant threat to patients’ health and lives and is a leading cause of cancer-related death worldwide (37). In HCC, MTDM may be involved in disease progression by affecting mitochondrial function and related signaling pathways (10). Emerging evidence highlights mtDNA methylation as a driver of mitochondrial dysfunction in HCC. Hypermethylation of the D-loop and OXPHOS-related genes (e.g., MT-ND6) suppresses their transcription, impairing electron transport chain activity and amplifying ROS production (38,39). This metabolic stress triggers a compensatory shift toward glycolysis and lipid synthesis, mediated by nuclear pathways such as PPARα and SREBP1. Notably, ACSL3—a key regulator of lipid metabolism—is upregulated in HCC and correlates with poor prognosis, whereas CPT1A perpetuates mitochondrial dysfunction. These findings collectively suggest a feedforward loop linking mtDNA methylation to lipid dysregulation. Therapeutic strategies targeting DNMT3A or critical lipid metabolic hubs (e.g., ACSL3/CPT1A) may disrupt this axis, thereby offering novel avenues for HCC treatment (40,41). However, the precise mechanisms underlying the role of MTDM in HCC remain unclear. Thus, exploring the role of MTDM in HCC, identifying prognosis-related genes associated with MTDM in HCC, and investigating their potential mechanisms of action could provide new references for the clinical diagnosis and treatment of HCC.
In this study, we used the RNA-seq and clinical data of HCC patients from the UCSC Xena database. By integrating the MTDM-related genes, we first identified the DEGs. We then identified major subtypes by unsupervised clustering, resulting in 333 candidate genes. The univariate Cox regression analysis of these 333 candidate genes revealed that 57 genes were significantly associated with patient survival. In addition, the LASSO logistic regression analysis and multivariate Cox regression analysis further narrowed down the selection, identifying the genes ADH4 and DNASE1L3 as key prognostic genes, leading to the construction of a risk model for HCC. The validation results confirmed that this model was able to effectively distinguish between the high- and low-risk groups in the training, validation, and internal validation sets. Further, the results showed that the low-risk group had significantly higher survival rates than the high-risk group.
The immune cell infiltration analysis of the high- and low-risk HCC groups using the ssGSEA algorithm revealed that 14 immune cells differed significantly between the two groups. Notably, ADH4 was significantly negatively correlated with activated CD4+ T cells (r=−0.312), while DNASE1L3 was significantly positively correlated with effector memory CD8+ T cells (r=0.332). Additionally, differences in the expression of the immune checkpoint genes were found between the high- and low-risk groups. The GSEA results also revealed significant enrichment differences in pathways such as chemical carcinogenesis-DNA adducts and neuroactive ligand-receptor interaction between the two groups.
An analysis of chemotherapy drug sensitivity, based on 138 identified drugs, revealed significant differences in the sensitivity of the patients to 48 drugs, including BI.2536, A.443654, and ABT.888, between the high- and low-risk groups. These findings provide a potential basis for drug selection and new research directions for the development of clinical treatments for HCC.
In this study, ADH4 and DNASE1L3 were identified as prognostic genes associated with MTDM in HCC. ADH4 plays a crucial role in the hepatic regulation of metabolism associated with various lipid peroxidation processes. As a key enzyme involved in alcohol metabolism, variations in ADH4 contribute to an increased susceptibility to alcohol and drug dependence (42-44). In liver samples from patients with alcoholic hepatitis, the expression of alcohol metabolism genes, including ADH4, is significantly downregulated (45). Similarly, the protein levels of ADH4 are reduced in non-alcoholic steatohepatitis. Further, ADH4 expression is significantly decreased in HCC (46). Moreover, many studies have shown that the downregulation of ADH4 is correlated with decreased OS rates in HCC patients (47,48). In addition, ADH4 plays a protective role in immune metabolism, and its low level may be related to the high-risk prognosis of HCC patients (49). These findings confirmed that ADH4, a pivotal enzyme in alcohol metabolism, could serve as a significant prognostic marker for HCC and wields substantial influence on the pathogenesis and progression of this malignancy.
DNASE1L3 is an endonuclease that belongs to the deoxyribonuclease I family and has the ability to digest DNA within chromatin (50). Recent studies have shown that DNASE1L3 may also serve as a biomarker in breast, liver, lung, colon, and stomach cancer (51), and as a favorable prognostic factor for HCC (52). The overexpression of DNASE1L3 in ovarian cancer cells can lead to the degradation of the tumor cell genome and ultimately cell death. More importantly, the expression of DNASE1L3 is closely related to the staging of clear cell renal cell carcinoma (53). Moreover, DNASE1L3 has been shown to play roles in immune escape and senescence-induced angiogenesis in HCC cells (54). A reverse transcription quantitative polymerase chain reaction (RT-qPCR) analysis also showed that the expression of DNASE1L3 messenger RNA was significantly lower in HCC tissues than normal tissues. Further, positive DNASE1L3 expression was found to be an independent prognostic factor for better survival in HCC patients following radical resection (52). Therefore, DNASE1L3 activators may offer a potential opportunity for combating HCC.
Through the GSEA, we found that the high- and low-risk groups were significantly enriched in five KEGG pathways, including fatty acid degradation and peroxisome. Fatty acid degradation, a critical component of lipid catabolism, generates several fatty acids that are necessary for cancer cells to survive (55,56), which not only can provide the energy they require for rapid proliferation but also support oncogenic signals that promote tumorigenesis and cancer progression (57,58). A study has shown that fatty acid metabolism is closely linked to the progression of gastric cancer (59). Notably, the liver plays a central role in fatty acid metabolism.
Peroxisomes are single membrane organelles involved in more than 50 different enzymatic activities in mammals (60). These enzymes include metabolic proteins that are essential for lipid metabolism. Various types of tumors exhibit alterations in peroxisome abundance and activity (61). Remarkably, in our study, peroxisome metabolism itself was also a pathway affecting the prognosis of the HCC patients. Peroxisome protein levels or enzymatic activities of peroxisome metabolism were found to be greatly reduced in colon cancer, breast cancer, renal cell cancer, and HCC (62). Therefore, HCC may be affected by increasing peroxisome activity.
Through an immune infiltration analysis, we found differences in 11 types of immune cells between the high- and low-risk patients. Activated CD4+ T cells are immune cells that express CD4 molecules on their surface. CD4+ T cells are thought to play an auxiliary immune role in promoting cytotoxic CD8+ T lymphocytes and innate immune responses (63); however, in recent years, the cytotoxicity of CD4+ T cells in cancer has also attracted increasing attention (64). Previous study has shown that the early activation of CD4+ T cells is crucial for establishing an antitumor immune response (65). Multiple cytotoxic CD4+ T cell states in bladder cancer can kill autologous tumors in a major histocompatibility complex (MHC) class II-dependent manner (66). One study showed that an antibody targeting human epidermal growth factor receptor 2 (HER2) and T cells inhibits breast cancer growth via CD4+ T cells rather than CD8+ T cells (67). Additionally, CD4+ T cells also play an immunosurveillance role in HCC initiation and progression (68). Our study further confirmed that CD4+ T cells are antitumor effector cells, which corresponds with their long-standing role as central participants and coordinators in innate and antigen-specific immune responses (69). Thus, CD4+ T cells may affect HCC by playing a role in the immune response.
Eosinophils have been shown to play a role in the tumor immune microenvironment (70). In recent years, a study has shown that changes in the proportion of eosinophils in peripheral blood may be correlated with the efficacy of immunotherapy (71). For example, tumor immunotherapy has shown that the eosinophil ratio in the peripheral blood changes significantly after patients are treated with programmed cell death protein 1 (PD-1) antibodies, and that such changes are correlated with the efficacy of the treatment (72). Research has also shown that among patients with advanced malignant tumors treated with PD-1 antibodies, those with a significant increase in the percentage of eosinophils in the peripheral blood after treatment had significantly prolonged progression-free survival and OS, which implies that eosinophils may be used as a new biomarker for predicting the efficacy of immunotherapy (73-76). In addition, eosinophil infiltration in HCC has been found to be significantly correlated with patient survival (71). In our study, there was a significant difference in eosinophils between the high- and low-risk groups. However, the specific mechanism of their action remains unclear and requires further in-depth research.
In this study, two prognostic genes were identified by a univariate analysis, LASSO analysis, and multivariate regression analysis. Based on the two prognostic genes, a risk model was constructed and verified. A functional GSEA, immune infiltration analysis, and drug sensitivity analysis were performed on the patients in the high- and low-risk groups. Our results provide novel insights into the prognosis and treatment of HCC patients. While our study provides mechanistic insights into MTDM’s role in HCC, we recognize that additional functional assays—such as copy number karyotyping and quantitative proliferation analysis—could further validate its impact on genome stability and cell cycle regulation. Future studies employing these approaches will help clarify MTDM’s broader functional network. To fully dissect MTDM’s biological functions, we propose integrating copy number karyotyping (e.g., via SNP arrays) to evaluate its role in chromosomal integrity, combined with EdU-based proliferation assays to quantify cell cycle dynamics. These approaches will complement our current findings and provide a more comprehensive understanding of MTDM’s pleiotropic effects.
Conclusions
This study identified two prognostic genes (ADH4 and DNASE1L3) that are associated with MTDM in HCC, and successfully constructed a prognostic risk model related to MTDM in HCC. Our findings may provide novel insights into the clinical management of HCC, and pave the way for the development of novel therapeutic approaches. With further research and practical application, these results offer hope for the development of more effective treatments, and could thus improve the prognosis and quality of life of HCC patients.
Acknowledgments
We sincerely acknowledge the support from the Undergraduate Mentorship Program at Nanjing University of Chinese Medicine (NJUCM), which provided academic guidance and methodological oversight, and the Undergraduate Scientific Research and Innovation Pioneer Program of its School of Medicine, which offered access to research facilities.
Footnote
Reporting Checklist: The authors have completed the TRIPOD reporting checklist. Available at https://tcr.amegroups.com/article/view/10.21037/tcr-2025-546/rc
Peer Review File: Available at https://tcr.amegroups.com/article/view/10.21037/tcr-2025-546/prf
Funding: This study was supported by
Conflicts of Interest: All authors have completed the ICMJE uniform disclosure form (available at https://tcr.amegroups.com/article/view/10.21037/tcr-2025-546/coif). The authors have no conflicts of interest to declare.
Ethical Statement: The authors are accountable for all aspects of the work in ensuring that questions related to the accuracy or integrity of any part of the work are appropriately investigated and resolved. The study was conducted in accordance with the Declaration of Helsinki (as revised in 2013).
Open Access Statement: This is an Open Access article distributed in accordance with the Creative Commons Attribution-NonCommercial-NoDerivs 4.0 International License (CC BY-NC-ND 4.0), which permits the non-commercial replication and distribution of the article with the strict proviso that no changes or edits are made and the original work is properly cited (including links to both the formal publication through the relevant DOI and the license). See: https://creativecommons.org/licenses/by-nc-nd/4.0/.
References
- Ajoolabady A, Tang D, Kroemer G, et al. Ferroptosis in hepatocellular carcinoma: mechanisms and targeted therapy. Br J Cancer 2023;128:190-205. [Crossref] [PubMed]
- Wang W, Wei C. Advances in the early diagnosis of hepatocellular carcinoma. Genes & Diseases 2020;7:308-19. [Crossref] [PubMed]
- Seydi H, Nouri K, Rezaei N, et al. Autophagy orchestrates resistance in hepatocellular carcinoma cells. Biomed Pharmacother 2023;161:114487. [Crossref] [PubMed]
- Nagaraju GP, Dariya B, Kasa P, et al. Epigenetics in hepatocellular carcinoma. Semin Cancer Biol 2022;86:622-32. [Crossref] [PubMed]
- Wang Z, Qin H, Liu S, et al. Precision diagnosis of hepatocellular carcinoma. Chin Med J (Engl) 2023;136:1155-65. [Crossref] [PubMed]
- Yilma M, Houhong Xu R, Saxena V, et al. Survival Outcomes Among Patients With Hepatocellular Carcinoma in a Large Integrated US Health System. JAMA Netw Open 2024;7:e2435066. [Crossref] [PubMed]
- Sun L, Ke X, Guan A, et al. Intratumoural microbiome can predict the prognosis of hepatocellular carcinoma after surgery. Clin Transl Med 2023;13:e1331. [Crossref] [PubMed]
- Moore LD, Le T, Fan G. DNA Methylation and Its Basic Function. Neuropsychopharmacology 2013;38:23-38. [Crossref] [PubMed]
- Stoccoro A, Coppede F. Mitochondrial DNA Methylation and Human Diseases. Int J Mol Sci 2021;22. [Crossref] [PubMed]
- Shi Y, Wu Z, Liu S, et al. Targeting PRMT3 impairs methylation and oligomerization of HSP60 to boost anti-tumor immunity by activating cGAS/STING signaling. Nat Commun 2024;15:7930. [Crossref] [PubMed]
- Zhu H, Xie Z. Therapeutic potential of tLyp-1-EV-shCTCF in inhibiting liver cancer stem cell self-renewal and immune escape via SALL3 modulation in hepatocellular carcinoma. Transl Oncol 2024;49:102048. [Crossref] [PubMed]
- Gu X, Huang X, Zhang X, et al. Development and Validation of a DNA Methylation-related Classifier of Circulating Tumour Cells to Predict Prognosis and to provide a therapeutic strategy in Lung Adenocarcinoma. Int J Biol Sci 2022;18:4984-5000. [Crossref] [PubMed]
- Ma Y, Du J, Chen M, et al. Mitochondrial DNA methylation is a predictor of immunotherapy response and prognosis in breast cancer: scRNA-seq and bulk-seq data insights. Front Immunol 2023;14:1219652. [Crossref] [PubMed]
- van Gisbergen MW, Voets AM, Starmans MHW, et al. How do changes in the mtDNA and mitochondrial dysfunction influence cancer and cancer therapy? Challenges, opportunities and models. Mutation Research/Reviews in Mutation Research 2015;764:16-30. [Crossref] [PubMed]
- Wan D, Cheng A, Wang Y, et al. Analyzing RNA-Seq data from Chlamydia with super broad transcriptomic activation: challenges, solutions, and implications for other systems. BMC Genomics 2024;25:801. [Crossref] [PubMed]
- Gustavsson EK, Zhang D, Reynolds RH, et al. ggtranscript: an R package for the visualization and interpretation of transcript isoforms using ggplot2. Bioinformatics 2022;38:3844-6. [Crossref] [PubMed]
- Wilkerson MD, Hayes DN. ConsensusClusterPlus: a class discovery tool with confidence assessments and item tracking. Bioinformatics 2010;26:1572-3. [Crossref] [PubMed]
- Gao CH, Yu G, Cai P. ggVennDiagram: An Intuitive, Easy-to-Use, and Highly Customizable R Package to Generate Venn Diagram. Front Genet 2021;12:706907. [Crossref] [PubMed]
- Wu T, Hu E, Xu S, et al. clusterProfiler 4.0: A universal enrichment tool for interpreting omics data. Innovation (Camb) 2021;2:100141. [Crossref] [PubMed]
- Langfelder P, Horvath S. WGCNA: an R package for weighted correlation network analysis. BMC Bioinformatics 2008;9:559. [Crossref] [PubMed]
- Hanzelmann S, Castelo R, Guinney J. GSVA: gene set variation analysis for microarray and RNA-seq data. BMC Bioinformatics 2013;14:7. [Crossref] [PubMed]
- Therneau TM. survival: A Package for Survival Analysis in R. 3.8-3 ed: Comprehensive R Archive Network (CRAN); 2024.
- Wickham H. ggplot2: Elegant Graphics for Data Analysis (2nd ed.). Springer Cham; 2016.
- In J, Lee DK. Survival analysis: part II - applied clinical data analysis. Korean J Anesthesiol 2019;72:441-57. [Crossref] [PubMed]
- Balduzzi S, Rücker G, Schwarzer G. How to perform a meta-analysis with R: a practical tutorial. Evid Based Ment Health 2019;22:153-60. [Crossref] [PubMed]
- Bi X, Rexer B, Arteaga CL, et al. Evaluating HER2 amplification status and acquired drug resistance in breast cancer cells using Raman spectroscopy. J Biomed Opt 2014;19:025001. [Crossref] [PubMed]
- Heagerty PJ, Lumley T, Pepe MS. Time-dependent ROC curves for censored survival data and a diagnostic marker. Biometrics 2000;56:337-44. [Crossref] [PubMed]
- Vickers AJ, Elkin EB. Decision curve analysis: a novel method for evaluating prediction models. Med Decis Making 2006;26:565-74. [Crossref] [PubMed]
- Aran D, Hu Z, Butte AJ. xCell: digitally portraying the tissue cellular heterogeneity landscape. Genome Biol 2017;18:220. [Crossref] [PubMed]
- Rahnev D, Desender K, Lee ALF, et al. The Confidence Database. Nat Hum Behav 2020;4:317-25. [Crossref] [PubMed]
- Yu L, Ji T, Liao W, et al. H4-methylation regulators mediated epitranscriptome patterns and tumor microenvironment infiltration characterization in hepatocellular carcinoma. Clin Epigenetics 2023;15:43. [Crossref] [PubMed]
- Seritrakul P, Gross JM. Tet-mediated DNA hydroxymethylation regulates retinal neurogenesis by modulating cell-extrinsic signaling pathways. PLoS Genet 2017;13:e1006987. [Crossref] [PubMed]
- Ma C, Tu D, Xu Q, et al. Identification of m(7)G regulator-mediated RNA methylation modification patterns and related immune microenvironment regulation characteristics in heart failure. Clin Epigenetics 2023;15:22. [Crossref] [PubMed]
- Shi M, Tan S, Xie XP, et al. Globally learning gene regulatory networks based on hidden atomic regulators from transcriptomic big data. BMC Genomics 2020;21:711. [Crossref] [PubMed]
- Bam M, Yang X, Zumbrun EE, et al. Dysregulated immune system networks in war veterans with PTSD is an outcome of altered miRNA expression and DNA methylation. Sci Rep 2016;6:31209. [Crossref] [PubMed]
- Khan FA, Nsengimana B, Awan UA, et al. Regulatory roles of N6-methyladenosine (m(6)A) methylation in RNA processing and non-communicable diseases. Cancer Gene Ther 2024;31:1439-53. [Crossref] [PubMed]
- Li N, Lu B, Luo C, et al. Incidence, mortality, survival, risk factor and screening of colorectal cancer: A comparison among China, Europe, and northern America. Cancer Lett 2021;522:255-68. [Crossref] [PubMed]
- Basu U, Bostwick AM, Das K, et al. Structure, mechanism, and regulation of mitochondrial DNA transcription initiation. J Biol Chem 2020;295:18406-25. [Crossref] [PubMed]
- Shock LS, Thakkar PV, Peterson EJ, et al. DNA methyltransferase 1, cytosine methylation, and cytosine hydroxymethylation in mammalian mitochondria. Proc Natl Acad Sci U S A 2011;108:3630-5. [Crossref] [PubMed]
- Huang L, Xu R, Chen S, et al. Modulating lipid metabolism by nanoparticles (NPs)-mediated ACSL3 silencing to inhibit hepatocellular carcinoma growth and metastasis. Mol Cancer 2025;24:73. [Crossref] [PubMed]
- Liu Y, Wang F, Yan G, et al. CPT1A loss disrupts BCAA metabolism to confer therapeutic vulnerability in TP53-mutated liver cancer. Cancer Lett 2024;595:217006. [Crossref] [PubMed]
- Luo X, Kranzler HR, Zuo L, et al. ADH4 gene variation is associated with alcohol dependence and drug dependence in European Americans: results from HWD tests and case-control association studies. Neuropsychopharmacology 2006;31:1085-95. [Crossref] [PubMed]
- Pochareddy S, Edenberg HJ. Identification of a FOXA-dependent enhancer of human alcohol dehydrogenase 4 (ADH4). Gene 2010;460:1-7. [Crossref] [PubMed]
- Turchi C, Piva F, Solito G, et al. ADH4 intronic variations are associated with alcohol dependence: results from an Italian case-control association study. Pharmacogenet Genomics 2012;22:79-94. [Crossref] [PubMed]
- Luo J, Hou Y, Ma W, et al. A novel mechanism underlying alcohol dehydrogenase expression: hsa-miR-148a-3p promotes ADH4 expression via an AGO1-dependent manner in control and ethanol-exposed hepatic cells. Biochem Pharmacol 2021;189:114458. [Crossref] [PubMed]
- Li L, Huang YT, Wang LT, et al. ADH4-a potential prognostic marker for hepatocellular carcinoma with possible immune-related implications. BMC Cancer 2024;24:927. [Crossref] [PubMed]
- Liu X, Li T, Kong D, et al. Prognostic implications of alcohol dehydrogenases in hepatocellular carcinoma. BMC Cancer 2020;20:1204. [Crossref] [PubMed]
- Sun Y, Chen ZY, Gan X, et al. A novel four-gene signature for predicting the prognosis of hepatocellular carcinoma. Scand J Gastroenterol 2022;57:1227-37. [Crossref] [PubMed]
- Zhang Y, Jiang HH, Wang ZY, et al. Alcohol dehydrogenase 4 is a TP53-associated gene signature for the prediction of prognosis in hepatocellular carcinoma. Oncol Lett 2023;25:3. [Crossref] [PubMed]
- Sun J, Wang X, Shen Q, et al. DNASE1L3 inhibits hepatocellular carcinoma by delaying cell cycle progression through CDK2. Cellular Oncology 2022;45:1187-202. [Crossref] [PubMed]
- Deng Z, Xiao M, Du D, et al. DNASE1L3 as a Prognostic Biomarker Associated with Immune Cell Infiltration in Cancer. Onco Targets Ther 2021;14:2003-17. [Crossref] [PubMed]
- Wang S, Ma H, Li X, et al. DNASE1L3 as an indicator of favorable survival in hepatocellular carcinoma patients following resection. Aging (Albany NY) 2020;12:1171-85. [Crossref] [PubMed]
- Malecki M, Dahlke J, Haig M, et al. Eradication of Human Ovarian Cancer Cells by Transgenic Expression of Recombinant DNASE1, DNASE1L3, DNASE2, and DFFB Controlled by EGFR Promoter: Novel Strategy for Targeted Therapy of Cancer. J Genet Syndr Gene Ther 2013;4:152. [Crossref] [PubMed]
- Guo D, Ma D, Liu P, et al. DNASE1L3 arrests tumor angiogenesis by impairing the senescence-associated secretory phenotype in response to stress. Aging (Albany NY) 2021;13:9874-99. [Crossref] [PubMed]
- Koundouros N, Poulogiannis G. Reprogramming of fatty acid metabolism in cancer. British Journal of Cancer 2020;122:4-22. [Crossref] [PubMed]
- Ma J, Wang S, Zhang P, et al. Emerging roles for fatty acid oxidation in cancer. Genes & Diseases 2024;101491.
- Ma Y, Temkin SM, Hawkridge AM, et al. Fatty acid oxidation: An emerging facet of metabolic transformation in cancer. Cancer Letters 2018;435:92-100. [Crossref] [PubMed]
- Li Z, Zhang H. Reprogramming of glucose, fatty acid and amino acid metabolism for cancer progression. Cellular and Molecular Life Sciences 2016;73:377-92. [Crossref] [PubMed]
- Li C, Zhang L, Qiu Z, et al. Key Molecules of Fatty Acid Metabolism in Gastric Cancer. Biomolecules 2022;12. [Crossref] [PubMed]
- Wanders RJ, Waterham HR. Biochemistry of mammalian peroxisomes revisited. Annu Rev Biochem 2006;75:295-332. [Crossref] [PubMed]
- Dahabieh MS, Di Pietro E, Jangal M, et al. Peroxisomes and cancer: The role of a metabolic specialist in a disease of aberrant metabolism. Biochimica et Biophysica Acta (BBA) - Reviews on Cancer 2018;1870:103-21. [Crossref] [PubMed]
- Kim JA. Peroxisome Metabolism in Cancer. Cells 2020;9. [Crossref] [PubMed]
- Swain SL, McKinstry KK, Strutt TM. Expanding roles for CD4+ T cells in immunity to viruses. Nature Reviews Immunology 2012;12:136-48. [Crossref] [PubMed]
- Tay RE, Richardson EK, Toh HC. Revisiting the role of CD4(+) T cells in cancer immunotherapy-new insights into old paradigms. Cancer Gene Ther 2021;28:5-17. [Crossref] [PubMed]
- Wang S, Meng L, Xu N, et al. Hepatocellular carcinoma-specific epigenetic checkpoints bidirectionally regulate the antitumor immunity of CD4 + T cells. Cell Mol Immunol 2024;21:1296-308. [Crossref] [PubMed]
- Oh DY, Kwek SS, Raju SS, et al. Intratumoral CD4(+) T Cells Mediate Anti-tumor Cytotoxicity in Human Bladder Cancer. Cell 2020;181:1612-25.e13. [Crossref] [PubMed]
- Seung E, Xing Z, Wu L, et al. A trispecific antibody targeting HER2 and T cells inhibits breast cancer growth via CD4 cells. Nature 2022;603:328-34. [Crossref] [PubMed]
- Zheng C, Snow BE, Elia AJ, et al. Tumor-specific cholinergic CD4(+) T lymphocytes guide immunosurveillance of hepatocellular carcinoma. Nat Cancer 2023;4:1437-54. [Crossref] [PubMed]
- Speiser DE, Chijioke O, Schaeuble K, et al. CD4(+) T cells in cancer. Nat Cancer 2023;4:317-29. [Crossref] [PubMed]
- Ghaffari S, Rezaei N. Eosinophils in the tumor microenvironment: implications for cancer immunotherapy. J Transl Med 2023;21:551. [Crossref] [PubMed]
- Alves A, Dias M, Campainha S, et al. Peripheral blood eosinophilia may be a prognostic biomarker in non-small cell lung cancer patients treated with immunotherapy. J Thorac Dis 2021;13:2716-27. [Crossref] [PubMed]
- Li C, Xu X, Wei S, et al. Tumor-associated macrophages: potential therapeutic strategies and future prospects in cancer. J Immunother Cancer 2021;9. [Crossref] [PubMed]
- Moreira A, Leisgang W, Schuler G, et al. Eosinophilic count as a biomarker for prognosis of melanoma patients and its importance in the response to immunotherapy. Immunotherapy 2017;9:115-21. [Crossref] [PubMed]
- Martens A, Wistuba-Hamprecht K, Geukes Foppen M, et al. Baseline Peripheral Blood Biomarkers Associated with Clinical Outcome of Advanced Melanoma Patients Treated with Ipilimumab. Clin Cancer Res 2016;22:2908-18. [Crossref] [PubMed]
- Harbaum L, Pollheimer MJ, Kornprat P, et al. Peritumoral eosinophils predict recurrence in colorectal cancer. Mod Pathol 2015;28:403-13. [Crossref] [PubMed]
- Safiri S, Ashrafi-Asgarabad A. Peripheral Blood Biomarkers Associated with Clinical Outcome in Non-Small Cell Lung Cancer Patients Treated with Nivolumab: Methodological Issues. J Thorac Oncol 2018;13:e85-e6. [Crossref] [PubMed]