Development of coenzyme Q10-related molecular subtypes and a prognostic signature for predicting breast cancer prognosis and response to immunotherapy
Highlight box
Key findings
• The coenzyme Q10 (CoQ10) pathway was found to be significantly associated with iron death and breast cancer (BRCA) prognosis.
• The reliability of different CoQ10 expression patterns in assessing the immune profile and immunotherapy of BRCA samples was elucidated.
• The study identified potential chemotherapeutic agents for the treatment of BRCA.
What is known and what is new?
• Machine learning can be used to identify biomarkers for different types of tumor.
• This study extended this approach by examining the iron death pathway activity in BRCA to identify commonalities that could serve as key points of intervention.
What is the implication, and what should change now?
• The risk model in this study may serve to better inform the clinical diagnosis of patients with BRCA and related treatment strategies. However, follow-up clinical samples are needed to determine the clinical value of the model.
Introduction
Breast cancer (BRCA) is the most prevalent and deadliest malignant tumor in women worldwide. Approximately, 2,300,000 women are diagnosed with BRCA each year, 30% of whom succumb to this disease (1). Typically, BRCA is classified into five subtypes based on historical and molecular differences, including HER2-positive, triple-negative/basal, normal, and luminal A and B (2). Surgery combined with radiotherapy, targeted therapy, endocrine therapy, and immunotherapy are the specific options available to treat patients with the different subtypes of BRCA (3-6). However, there is a considerable variability in the prognosis of patients with BRCA primarily due to the enormous intratumor heterogeneity across the different endogenous subtypes. Cases of BRCA also differ in terms of the immune microenvironment (7-10). Immunotherapy, which mainly consists of immune checkpoint inhibitors (ICIs) and immune cell therapies, has significantly improved the prognosis of patients with advanced BRCA (11,12). However, due to the lack of large-scale prospective clinical trials, it remains unclear how immunotherapy can be specifically and practically applied in clinic (13,14). Therefore, in order to obtain relevant evidence regarding the development of appropriate individual treatment strategies, signatures that can credibly assess the prognosis and response to immunotherapy in patients with BRCA are urgently needed.
Ferroptosis is an iron-dependent form of non-apoptotic cell death (15,16). It has attracted considerable attention in recent years due to its unique mechanism of cell death. It is essentially an imbalance in the antioxidant system due to lipid peroxidation resulting from intracellular iron accumulation. The field of iron death has long been focused on the membrane lipid repair enzyme, glutathione peroxidase (GPX4) (17). Recently, researchers have become interested in discovering a regulator of iron death independent of GPX4, and ferroptosis suppressor protein 1 (FSP1) is the first factor to have been discovered that can regulate ferroptosis independently of the GPX4 system (18). FSP1 uses NADPH to convert oxidized coenzyme Q10 (CoQ10) to reduce CoQ10, which directly reduces the formation of lipid peroxides and prevents the onset of ferroptosis (19). CoQ10 is a coenzyme present in all eukaryotes undergoing aerobic respiration and acts mainly in the mitochondrial oxidative phosphorylation process. In addition, it is a fat-soluble antioxidant that plays an important role in the metabolism of fatty acids, pyrimidines, and lysosomes. CoQ10 has been widely used due to its powerful antioxidant properties in numerous diseases, such as cardiovascular disease, diabetes mellitus, neurodegenerative diseases, and certain cancers. High-throughput sequencing is a rapidly evolving technology that may provide a basis for revealing CoQ10-related and prospective prognostic biomarkers. Therefore, we performed this comprehensive bioinformatics analysis to investigate the relevant functions of CoQ10 to determine their potential value in predicting survival among patients with BRCA.
Interferon gamma-mediated ferroptosis secreted by CD8+ T cells exerts a synergistic role in immunotherapy. This is a key antitumor mechanism of iron death and can be widely used in the immunotherapy of tumors in clinic. However, whether CoQ10 plays a considerable role in this process remains unknown. In this study, we identified CoQ10-related biomarkers by studying the CoQ10 pathway associated with iron death. We constructed a CoQ10-associated signature that demonstrated good predictive accuracy for predicting outcomes; this study may provide a theoretical basis for immunotherapeutic targets in BRCA. We present this article in accordance with the TRIPOD reporting checklist (available at https://tcr.amegroups.com/article/view/10.21037/tcr-2025-425/rc).
Methods
Bulk RNA-sequencing data acquisition and preprocessing
The transcriptomic data and associated clinical information for BRCA were sourced from The Cancer Genome Atlas (TCGA) database, which is available on the cBioPortal database (20). To ensure consistency between patient samples, we excluded samples with incomplete survival data. Additionally, we retrieved RNA-sequencing (RNA-seq) data and relevant clinical information from the Gene Expression Omnibus (GEO) database for BRCA cohorts GSE20711, GSE20685, and GSE19615 (21-23). Data with a cohort of patients with melanoma treated with nivolumab, GSE91061, were also obtained from the GEO database (24). We addressed issues related to duplicate gene symbols or multiple probes by selecting genes with the highest average expression levels. A total of 22 ferroptosis-related metabolic pathways were collected from the relevant literature and databases (table available at https://cdn.amegroups.cn/static/public/tcr-2025-425-1.xlsx). The study was conducted in accordance with the Declaration of Helsinki (as revised in 2013).
Differential gene expression
Differential gene expression analysis was performed using the “DESeq2” software package (25), with an absolute log fold change (|logFC|) threshold of greater than 1 and a P value of less than 0.05 used as the criteria for significance.
Construction of the CoQ10-related prognostic signature
To establish a CoQ10-related prognostic signature, we conducted a series of analyses including univariate and multivariate Cox regressions, as well as 10-fold cross-validation with least absolute shrinkage and selection operator (LASSO) regression (26). In the LASSO regression, we selected the “lambda.min” package to prevent overfitting. A set of thirteen genes (POU3F2, ABCC2, CEL, CD24, PXDNL, SPINK8, ACTL8, PAX7, CLEC3A, LRP1B, TNN, FABP7, and DTHD1) was used to construct the prognostic formula for the risk score as follows: risk score = ∑ni(Coefi × Expi), where Coefi represents the coefficients of the genes, and Expi represents the relative expression of genes in the cohort.
Validation and performance assessment
We performed Kaplan-Meier analysis to compare the overall survival (OS) rates of high-risk and low-risk subgroups based on the median risk score. The accuracy of the risk score in predicting 1-, 3-, and 5-year survival rates was evaluated using receiver operating characteristic (ROC) curves via the “timeROC” R package (The R Foundation for Statistical Computing) (27). Additionally, a prognostic nomogram incorporating the risk score and other clinical features was constructed using the “rms” R package (28). The performance of the nomogram was assessed through calibration curves and decision curve analysis (DCA).
Functional enrichment analysis
Functional enrichment analysis was conducted using the “clusterProfiler” R package, with a focus on Gene Ontology (GO) and Kyoto Encyclopedia of Genes and Genomes (KEGG) pathways (29). Additionally, gene set enrichment analysis (GSEA) and gene set variation analysis (GSVA) were employed to compare pathway activation between different groups (30). The relevant pathways were sourced from the Molecular Signatures Database (MSigDB) and associated research studies (31-33).
Immune infiltration analysis and immunotherapy responsiveness
The ESTIMATE (Estimation of Stromal and Immune Cells in Malignant Tumor Tissues Using Expression Data) algorithm was used to assess variations in immune scores, stromal scores, ESTIMATE scores, and tumor purity among samples (34). To investigate immune cell infiltration, we employed the Tumor Immune Estimation Resource 2.0 (TIMER2.0) algorithm to predict immune cell infiltration based on the gene expression data of tumors (http://timer.cistrome.org/). Additionally, the Tumor Immune Dysfunction and Exclusion (TIDE) algorithm was used to predict the response of patients with BRCA to ICI treatment.
Prediction of drug response
We used the R package “oncoPredict” to assess the predictive ability of risk score on chemotherapeutic agents by calculating patients’ half maximal inhibitory concentration (IC50) for various common chemotherapeutic agents (35).
Statistical analysis
All data analyses were performed using R software version 4.2.0. Unless otherwise specified, the two-tailed Wilcoxon test was used for comparisons. The Fisher exact test was used for contingency table analysis. Pearson correlation analysis was conducted to assess the correlations between variables. Survival differences were evaluated using Kaplan-Meier survival curves and log-rank tests. A P value of less than 0.05 was considered statistically significant. If not specified, P value abbreviations in the text represent (ns, P>0.05; *, P<0.05; **, P<0.01; ***, P<0.001; ****, P<0.0001).
Results
CoQ10 as a key ferroptosis metabolic pathway in BRCA
Heterogeneity in metabolic pathways leads to differences in clinical outcomes, and we sought to examine the key ferroptosis metabolic pathways associated with clinical outcomes. Through survey and statistics, we collected 22 metabolic pathways associated with ferroptosis from a series of studies. The 22 pathway activities were quantified by single-sample GSEA (ssGSEA) (Figure S1), and those that were associated with patients’ clinical prognosis were examined, with the most representative being CoQ10 (Figure S2A). Based on the median CoQ10 pathway activity, patients with BRCA were subtyped as having high or low CoQ10 activity. Higher CoQ10 activity was associated with poorer OS (Figure 1A) and with disease-free survival (DFS), disease-specific survival (DSS), and progression-free survival (PFS) (Figure 1B-1D). The overall level of CoQ10 activity and immune infiltration was further clarified. As shown in Figure 1E-1H, CoQ10 activity was significantly negatively correlated with immune and stromal infiltration and positively correlated with tumor purity (Figure 1E). In the immune cell infiltration analysis, there was a significant negative correlation between CoQ10 activity and the majority of immune cell infiltration, with the most significant negative correlations with naïve B-cell and CD8+ T-cell infiltration (Figure 1F). CoQ10 activity was also significantly negatively correlated with a variety of potential immune checkpoints, including CD274, HAVCR2, PDCD1, and CTLA4 (Figure 1G). Core biological pathway analysis indicated that CoQ10 activity was significantly negatively correlated with tumor-associated immune pathways including antigen presentation, cytokines, natural killer (NK) cell activity, and TGF-β (Figure 1H). Overall, CoQ10 metabolism appears to be a key metabolic pathway affecting the prognosis and immune microenvironment of BRCA.
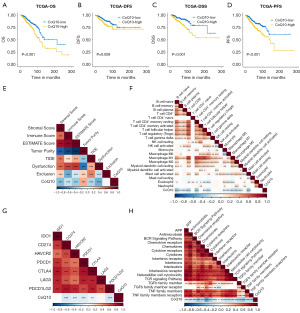
PDSS2 as a hub gene for CoQ10 metabolism
Given the importance of the CoQ10 metabolic pathway, we constructed a protein-protein interaction (PPI) pathway gene network and found extensive interactions within it. CoQ10 metabolic genes contained mainly PDSS family and COQ family proteins. In the gene network, PDSS2 was located in the central region (Figure S2B). The CoQ10 metabolic genes as a whole also showed good concordance in the TCGA data (Figure S2C). One-way Cox analysis showed that PDSS2 was significantly associated with the OS of patients with BRCA (Figure S2D). The PDSS2 protein is an enzyme that synthesizes the isoprene side chain of CoQ or ubiquinone, which determines the initiation of CoQ biosynthesis. In summary, we concluded that PDSS2 is a hub gene for CoQ10 metabolism.
Functional characterization between different CoQ10 subtypes
To further determine the differences in biological functionality between the two clusters, we conducted an analysis of the differentially expressed genes (DEGs) (Figure 2A). Subsequent to this, we employed gene GSEA, leveraging the fold changes identified in the DEG analysis, which indicated that CoQ10-high cluster patients exhibited heightened enrichment scores in E2F targets, G2M checkpoint, and MTORC1 signaling (Figure 2B). Conversely, patients with BRCA in the CoQ10-low cluster exhibited elevated enrichment in epithelial-mesenchymal transition (EMT), interferon (IFN) response, and myogenesis signaling pathways. Furthermore, we conducted comprehensive KEGG and GO functional enrichment analyses, which revealed a prominent upregulation of cell cycle-related signaling pathways in the CoQ10-high cluster. These pathways were related to nuclear division, meiotic cell cycle, and mitotic nuclear division (Figure 2C,2D). In contrast, CoQ10-low cluster patients displayed a heightened enrichment in a spectrum of immune-related pathways, particularly cytokine-cytokine receptor interaction, antigen processing and presentation, and leukocyte-mediated immunity (Figure S3A,S3B). To obtain a more detailed understanding of the transcriptional heterogeneity across patients with BRCA, we used the GSVA algorithm to quantify 16 recurrent cancer cell states that interface with the tumor microenvironment (TME), forming organized systems conducive to immune evasion, metastasis, and drug resistance. Our findings indicated that the patients in the CoQ10-high cluster were enriched in a variety of gene modules, such as cycle and oxidative phosphorylation modules. In contrast, patients in the CoQ10-low cluster demonstrated a higher score for astrocyte (AC)-like, oligodendrocyte progenitor cell (OPC)-like, and neural progenitor cell (NPC)-like modules (Figure 2E). Collectively, our systematic exploration of the functional state disparities between the two molecular subtypes of BRCA not only underscores their distinct biological characteristics but also offers fresh perspectives into the intricate mechanisms that underpin BRCA progression.
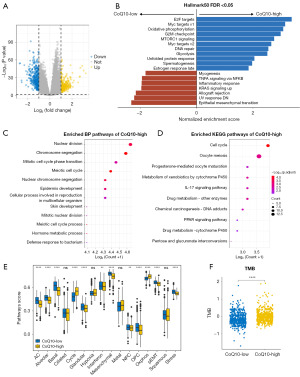
Mutational spectrum of different CoQ10 insomnia
Somatic mutations play a key role in aging and tumorigenesis, so we additionally examined the differences in mutational profiles between different CoQ10 isoforms (36). Tumor mutational burden (TMB) is the number of somatic nonsynonymous mutations in a given genomic region. A study has shown that patients with a higher TMB may benefit from ICI therapy (31). In this study, patients with high CoQ10 activity had higher TMB levels (Figure 2F). In addition, the TP53 and CDH1 genes also differed between CoQ10 isoforms, as indicated in the mutation waterfall plot in Figure S3C. In addition, patients with better CoQ10 activity showed high loading in terms of aneuploidy, mutation count, and frequency (Figure S3D-S3F). Overall, there was a level of variation in tumor mutation profiles between CoQ10 subtypes, with patients with higher CoQ10 activity having a higher TMB.
Distinct immune landscapes of the CoQ10 subtypes
We next sought to determine whether variations in CoQ10-related gene expression patterns reflect intertumoral immune heterogeneity and microenvironmental differences in BRCA by detecting a panel of immunologically pertinent signatures. We found that in the CoQ10-low group, a substantial proportion of immune-associated pathways exhibited augmented enrichment (Figure 3A and Figure S4A-S4D). To further substantiate our results, we used the GSVA algorithm to analyze the TME scores across the two CoQ10 clusters. Intriguingly, the CoQ10-low cluster exhibited higher HLA and TFG-β expression, suggesting a more pronounced immune presence (Figure 3B). Recognizing the heterogeneity in the TME between these two clusters, we employed CIBERSORT-ABS to assess the relative abundance of distinct immune cell subpopulations in BRCA. Notably, the CoQ10-low cluster was characterized by higher infiltration levels of M1-like macrophages, M2-like macrophages, and CD8+ T cells (Figure 3C and Figure S4E-S4J). Thus, this cluster was considered to exhibit an immune “hot” phenotype, partly explaining why patients in this group had better outcomes compared to those in the CoQ10-high cluster. Moreover, through TIDE analysis, we found the CoQ10-low cluster exhibited notably elevated TIDE scores, exclusion scores, and dysfunction scores, suggesting a heightened likelihood of immune evasion among these patients (Figure 3D-3F). Additionally, we observed a diminished immunotherapy response among patients in the CoQ10-low cluster as compared to those in the CoQ10-high cluster (Figure 3G). Overall, we comprehensively characterized the immune landscape and responsiveness to immunotherapy across the distinct BRCA subgroups, underscoring the complexity and heterogeneity of the immune microenvironment in BRCA.
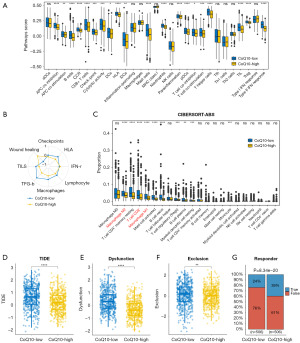
Development of a CoQ10-related prognostic model for BRCA
The aforementioned findings were able to substantiate the link between CoQ10-related genes and both the prognosis and response to immunotherapy among patients with BRCA, indicating the potential of CoQ10-related classification for evaluating prognosis and treatment efficacy. Consequently, we built a novel CoQ10-related prognostic model based on the DEGs between these BRCA clusters. By using univariate Cox regression and LASSO analysis, we selected 13 genes, including POU3F2, ABCC2, CEL, CD24, PXDNL, SPINK8, ACTL8, PAX7, CLEC3A, LRP1B, TNN, FABP7, and DTHD1, and developed a risk assessment model using data of the patients with BRCA among the TCGA cohort as the training set (Figure 4A).
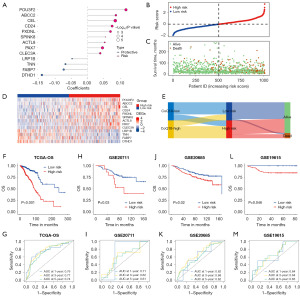
Following the computation of the CoQ10-related risk score for each patient within the BRCA cohort, we stratified patients into high-risk and low-risk groups based on the median risk score (Figure 4B). In parallel, we determined the survival status of the patients (Figure 4C) and the expression patterns of the 13 genes comprising the prognostic signature (Figure 4D). We noted that patients with higher CoQ10-related risk scores exhibited poorer survival outcomes, which was mirrored by the increased expression levels of the “risk” genes, specifically POU3F2 and ABCC2. We then employed a Sankey diagram to visually illustrate the intricate interplay between the identified clusters, CoQ10-related risk subgroups, and the survival outcomes in BRCA (Figure 4E). This diagram vividly demonstrated that patients with elevated CoQ10-related risk scores were disproportionately represented in the CoQ10-high cluster and were more likely to have a poorer prognosis. This observation was corroborated by Kaplan-Meier survival analysis (Figure 4F), which corroborated the significant survival advantage for patients in the low-risk group, further validating the prognostic value of the CoQ10-related prognostic model in stratifying patients with BRCA according to OS outcomes.
To evaluate the predictive value of our prognostic signature, we drew ROC curves for OS at 1, 3, and 5 years (Figure 4G). The area under the curve (AUC) yielded values of 0.76, 0.77, and 0.74, respectively, supporting predictive ability of our model, particularly in long-term survival predictions. Additionally, we verified the accuracy of our signature in three distinct external validation sets (GSE20711, GSE20685, and GSE19615), yielding satisfactory results and higher 1-, 3-, and 5-year AUC values (Figure 4H-4M). These findings not only confirm the robustness of our prognostic model but also highlight its potential for clinical application.
Establishment of a nomogram for forecasting survival in BRCA
We additionally conducted univariate and multivariate Cox regression analyses, and the CoQ10-related prognostic signature score emerged as an independent prognostic factor for patients with BRCA, regardless of age, pathological stage, and histologic grade (Figure 5A). Subsequently, we devised a predictive nomogram to enhance the prognostic efficacy of the CoQ10-related risk model. This nomogram serves as a quantitative and visualization tool for predicting 1-, 3-, and 5-year OS rates (Figure 5B). To assess the performance of the nomogram, we plotted calibration curves, which demonstrated that the prediction curves of the model closely aligned with the ideal curve, indicating good agreement between predicted and observed outcomes (Figure 5C). Moreover, the nomogram exhibited a significant positive net benefit in predicting the risk of death, surpassing the traditional age and tumor node metastasis (TNM) staging system in this regard (Figure 5D). In summary, these findings underscore the substantial clinical value of the nomogram model in predicting survival outcomes among patients with BRCA.
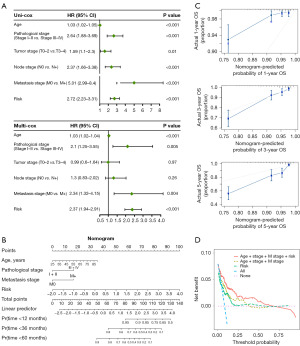
Functional and characteristics features of patients in the high- and low-risk groups
For patients in the different risk groups, we first analyzed differences in mutation profiles. We depicted the mutation profiles of patients with BRCA in the high- and low-risk and observed that the most frequently mutant genes showed comparable mutation frequency; however, TP53, CDH1, and SPTA1 showed higher mutation frequency (Figure 6A). Interestingly, patients in the high-risk group had significantly higher TMB levels, and the risk scores were significantly positively correlated with TMB (Figure 6B,6C). Aneuploidy, mutation frequency, and microsatellite instability scores were higher in the high-risk patients than in the low-risk group (Figure 6D-6G). To gain further insights, we conducted GSEA, which revealed that E2F, G2M, and oxidative phosphorylation were enriched in high-risk patients, suggesting a more aggressive phenotype. In contrast, IFN-γ response and IL6-JAK-STAT3 signaling pathway were highly enriched in low-risk patients, indicative of a more indolent disease state (Figure 6H). By applying GSVA to calculate the signature scores of 16 recurrent cancer cell states, we found that the cycle and oxidative phosphorylation gene modules were significantly overexpressed in high-risk patients as compared to in low-risk patients (Figure 6I). Collectively, these findings indicate there being distinct functional and genomic characteristics between high- and low-risk BRCA patients, providing valuable insights into the underlying biological mechanisms that differentiate these two risk strata.
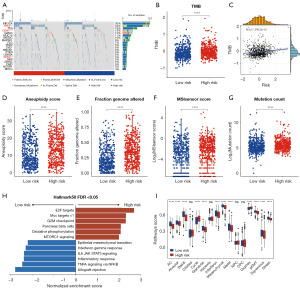
TME heterogeneity and immunotherapy responses in the two risk groups
Despite the limited body of research investigating the relationship between CoQ10 and TME, elucidating the TME landscape in the context of varying CoQ10 expression patterns in BRCA is of paramount importance. Consequently, this study sought to delineate the differences in TME characteristics between high-risk and low-risk BRCA patients. Initial analysis using the ESTIMATE algorithm demonstrated that TME scores were significantly higher in patients with lower risk scores, exhibiting a negative correlation with the risk score (Figure 7A and Figure S5A-S5D). Furthermore, the CIBERSORT algorithm was employed to characterize the compositional variations in TME between high-risk and low-risk patients (Figure 7B). High-risk BRCA patients displayed an increased abundance of M2-like macrophages, coupled with a reduced presence of CD8+ T cells and M1-like macrophages (Figure 7B). Notably, the risk score exhibited a positive correlation with the abundance of M2-like macrophages, while demonstrating a negative correlation with the levels of CD8+ T cells and M1-like macrophages (Figure S5E-S5G). Of particular interest, the CoQ10-related risk score was inversely correlated with the expression of multiple immune checkpoint markers (Figure 7C). Given that TME heterogeneity is a critical determinant of immunotherapy responsiveness, we conducted TIDE analysis to predict the immunotherapy response in patients from both risk groups. The results revealed that high-risk patients had significantly lower TIDE and dysfunction scores (Figure 7D,7E). Moreover, a higher proportion of high-risk patients were predicted to exhibit a favorable response to immunotherapy (Figure 7F). To corroborate these findings, we analyzed additional immunotherapy datasets, observing consistent results in melanoma patients treated with nivolumab (Figure 7G). Collectively, these findings underscore the distinct TME profiles between high-risk and low-risk BRCA patients and provide compelling evidence that high-risk patients may derive greater therapeutic benefit from immunotherapy compared to their low-risk counterparts.
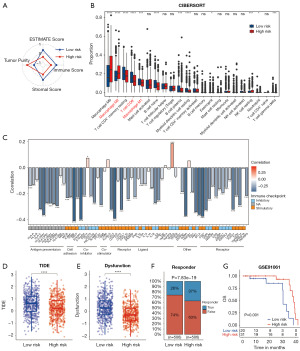
Comparison of anticancer drug sensitivity between the different risk groups
A critical consequence of tumor heterogeneity is the differential response of distinct cancer patient subgroups to various therapeutic interventions, which can result in treatment inefficacy and tumor recurrence. To elucidate the relationship between drug sensitivity, the CoQ10-related risk score, and candidate prognostic genes, we computed the IC50 values for each drug in the training set samples. We subsequently evaluated the significance and associations among drug sensitivities, risk scores, and the expression levels of candidate prognostic genes in BRCA patients (Figure 8A). Our analysis revealed that the low-risk group exhibited greater sensitivity to palbociclib and ribociclib compared to the high-risk group (Figure 8B,8C). Conversely, patients in the high-risk group demonstrated increased sensitivity to entinostat and lapatinib relative to those in the low-risk group (Figure 8D,8E). These findings highlight the potential utility of the CoQ10-related risk score in guiding personalized therapeutic strategies for BRCA patients.
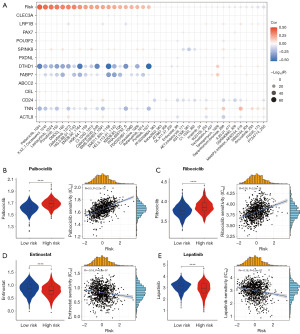
Discussion
Approximately 2.3 million patients are diagnosed with BRCA worldwide each year, representing a massive burden on human health. However, due to the highly heterogeneous tissue origin of BRCA, the efficacy of standard therapies varies greatly from patient to patient. In recent years, immunotherapy has been meaningfully applied in the treatment of BRCA, not only improving treatment outcomes but also reducing the adverse effects of treatments such as radiotherapy. However, similar to standard therapies, immunotherapy has considerable heterogeneity (37). Identifying populations that can potentially benefit from ICIs has been challenging and warrants further research attention (38). The advent of high-throughput sequencing has enabled the mining of a large number of cancer-related biomarkers, offering the possibility of addressing these challenges.
Ferroptosis is newly discovered mode of non-apoptotic cell death caused by the iron-dependent accumulation of lipid peroxides. Ferroptosis enhances the antitumor immune efficacy mediated by multiple pathways (39,40). It has been shown that ferroptosis-associated lipid peroxides can be used as recognition signals to promote recognition, phagocytosis, the processing of tumor antigens by dendritic cells, and presentation of tumor-associated antigens to CD8+ T lymphocytes, which can activate cytotoxic T lymphocytes to enhance tumor immunotherapy. Effector T cells and radiotherapy interact via ferroptosis to promote tumor clearance. Immunotherapy-activated CD8+ T cells increase lipid peroxidation and ferroptosis via IFN-γ, with the latter directly sensitizing tumor cells to radiotherapy (41,42). In addition to this, ferroptosis can facilitate immunotherapy through various pathways, for instance by promoting the transformation of tumor-associated macrophages and targeting tumor metabolic addiction. Therefore, we are committed to exploring the critical role of ferroptosis-related metabolic pathways in patients with BRCA through transcriptomics and genomic studies.
After preliminary investigation and exploration, we selected 22 important pathways related to ferroptosis metabolism. We sought to identify those pathways associated with OS in patients with BRCA. Quantifying ferroptosis metabolic pathway activity by ssGSEA through one-way Cox regression analysis, we found that CoQ10 activity was not only associated with OS, but also with DFS, DSS, and PFS. High CoQ10 activity was associated with poorer patient prognosis. We defined different CoQ10 activities as different CoQ10 subtypes, each with unique clinical, survival, biological, and immunological characteristics. Our study indicated that CoQ10 hyperactive isoforms exhibited higher metabolic activity during the tumor cell cycle, including the specific activation of E2F, G2M, and nuclear division pathways. The cell cycle unfolds in a precisely functioning manner, ensuring that cells inherit precise copies of DNA to their daughter cells (43,44). If any errors occur during DNA replication prior to division or DNA migration into the newly formed cell, then the cell’s genetic program can go awry, leading to the development of cancer or other diseases. Furthermore, we found that multiple neuron-related pathways were enriched in the CoQ10 hyperactive subgroup of patients with BRCA. The complex interplay between the nervous system and cancer has profound effects on all aspects of tumorigenesis and progression. This complex crosstalk regulates key processes such as tumorigenesis, tumor growth, invasion, metastatic dissemination, development of therapeutic resistance, stimulation of protumoral inflammation, and impairment of anticancer immune responses. Inadequate IFN signaling activity in CoQ10-low patients may partly explain the poor prognosis of this group. Tumor cells are able to survive in their microenvironment where they evade immune surveillance and resist pharmacological intervention. Theoretically, high CoQ10 activity can predict decreased levels of immune checkpoint expression and reduced infiltration of antitumor immune cells. These results suggest that the overall suppression of immune function further fuels the growth of tumour cells. Our results showed that patients with CoQ10 hypoactivity exhibited higher immune scores and multiple immune-related modules, suggesting an immune “hot” phenotype. However, patients with low CoQ10 activity were less sensitive to immunotherapy than those with high activity, which we speculate may be related to their immune cell depletion.
To further clarify the prognostic value of CoQ10-associated genes, we examined the DEGs between the high- and low-CoQ10 groups and developed a robust CoQ10-associated prognostic model via univariate Cox regression and LASSO regression analyses. The lysosome-associated prognostic features included 13 DEGs between the two CoQ10 subgroups, including POU3F2, ABCC2, CEL, CD24, PXDNL, SPINK8, ACTL8, PAX7, CLEC3A, LRP1B, TNN, FABP7, and DTHD1. CoQ10-associated prognostic features were proved valuable in predicting the prognosis of patients with BRCA in the different datasets. Notably, patients in the high-risk group demonstrated significantly worse outcomes compared to the low-risk group. By combining this risk score with clinical parameters, a nomogram was formed which demonstrated the clinical predictive value for BRCA. Further analyses showed that the high-risk group was associated with an EMT process characterized by the loss of epithelial cell polarity, reduced intercellular adhesion, and acquisition of mesenchymal traits. In the comparison of TME between the high-risk and low-risk groups, the high-risk group as a whole showed reduced immune cell infiltration. Our findings suggested that the ratio of M2-like to M1-like macrophages in patients with BRCA was significantly and positively correlated with CoQ10-related prognostic risk scores. Macrophages are in a quiescent state, called M0, and can differentiate into two distinct phenotypes: M1-like and M2-like (45). These polarized macrophages play a key role in mediating inflammatory processes. Specifically, M1-like macrophages are primarily involved in driving proinflammatory responses, whereas M2-like macrophages are primarily involved in modulating anti-inflammatory responses (46). In addition, the low-risk group also had higher levels of T follicular helper cells, which support B-cell function and antibody-mediated immune responses and tend to correlate with a better prognosis in a variety of solid tumors (47). TIDE analyses suggested that high-risk patients were more likely to respond well to immunotherapy. These extensive analyses highlight the potential of CoQ10-associated prognostic traits as reliable predictors of the prognosis and response to immunotherapy among patients with BRCA. Finally, this study examined the correlation between risk scores and IC50 values for commonly used chemotherapeutic agents. This analysis provides valuable insights for combination therapy or selection of patients with BRCA who may be more sensitive to specific chemotherapeutic agents. Overall, this study highlights the potential clinical application of CoQ10-related prognostic features in guiding personalized treatment strategies for those with BRCA.
Although innovative molecular classifications based on CoQ10-related genes and the robust prognostic models that they provide are noteworthy, inherent limitations must be addressed. The practical application of these CoQ10-centered approaches in the clinical setting is likely to encounter a number of challenges. It is critical to work closely with clinical experts to refine and standardize the process so as to improve clinical applicability and ease of use. In addition, the retrospective nature of the recruitment of patients with BRCA might have affected the results. In the future, more cost-effective genetic tests will bring more universal convenience to the research of this technology. This technology, combined with the judgement of clinicians, will provide patients with personalized guidance for diagnosis and treatment. It is important to validate the findings through rigorous, multicenter, randomized controlled trials. These trials should have reliable methods, broad patient samples, and thorough follow-up periods to ensure reliability and generalizability of the findings.
Conclusions
In this study, patients with BRCA were stratified into two distinct CoQ10 clusters, which exhibited significant disparities in survival outcomes, immune cell infiltration, and other critical variables. Furthermore, we have established the CoQ10-related prognostic signature as a novel and independent prognostic factor for predicting the clinical outcomes of BRCA patients. The CoQ10-based molecular subtyping and prognostic signature demonstrated robust utility as indicators of tumor progression, offering potential as a valuable tool for informing personalized clinical decision-making, particularly in the context of immunotherapy for BRCA.
Acknowledgments
We acknowledge and appreciate our colleagues for their valuable efforts and comments on this paper.
Footnote
Reporting Checklist: The authors have completed the TRIPOD reporting checklist. Available at https://tcr.amegroups.com/article/view/10.21037/tcr-2025-425/rc
Peer Review File: Available at https://tcr.amegroups.com/article/view/10.21037/tcr-2025-425/prf
Funding: This study was supported by
Conflicts of Interest: All authors have completed the ICMJE uniform disclosure form (available at https://tcr.amegroups.com/article/view/10.21037/tcr-2025-425/coif). The authors have no conflicts of interest to declare.
Ethical Statement: The authors are accountable for all aspects of the work in ensuring that questions related to the accuracy or integrity of any part of the work are appropriately investigated and resolved. The study was conducted in accordance with the Declaration of Helsinki (as revised in 2013).
Open Access Statement: This is an Open Access article distributed in accordance with the Creative Commons Attribution-NonCommercial-NoDerivs 4.0 International License (CC BY-NC-ND 4.0), which permits the non-commercial replication and distribution of the article with the strict proviso that no changes or edits are made and the original work is properly cited (including links to both the formal publication through the relevant DOI and the license). See: https://creativecommons.org/licenses/by-nc-nd/4.0/.
References
- Bray F, Laversanne M, Sung H, et al. Global cancer statistics 2022: GLOBOCAN estimates of incidence and mortality worldwide for 36 cancers in 185 countries. CA Cancer J Clin 2024;74:229-63. [Crossref] [PubMed]
- Coombes RC, Badman PD, Lozano-Kuehne JP, et al. Results of the phase IIa RADICAL trial of the FGFR inhibitor AZD4547 in endocrine resistant breast cancer. Nat Commun 2022;13:3246. [Crossref] [PubMed]
- Rizzo A, Cusmai A, Acquafredda S, et al. KEYNOTE-522, IMpassion031 and GeparNUEVO: changing the paradigm of neoadjuvant immune checkpoint inhibitors in early triple-negative breast cancer. Future Oncol 2022;18:2301-9. [Crossref] [PubMed]
- Rizzo A, Schipilliti FM, Di Costanzo F, et al. Discontinuation rate and serious adverse events of chemoimmunotherapy as neoadjuvant treatment for triple-negative breast cancer: a systematic review and meta-analysis. ESMO Open 2023;8:102198. [Crossref] [PubMed]
- Sahin TK, Ayasun R, Rizzo A, et al. Prognostic Value of Neutrophil-to-Eosinophil Ratio (NER) in Cancer: A Systematic Review and Meta-Analysis. Cancers (Basel) 2024;16:3689. [Crossref] [PubMed]
- Caputo R, Buono G, Piezzo M, et al. Sacituzumab Govitecan for the treatment of advanced triple negative breast cancer patients: a multi-center real-world analysis. Front Oncol 2024;14:1362641. [Crossref] [PubMed]
- Guven DC, Erul E, Kaygusuz Y, et al. Immune checkpoint inhibitor-related hearing loss: a systematic review and analysis of individual patient data. Support Care Cancer 2023;31:624. [Crossref] [PubMed]
- Sorlie T, Tibshirani R, Parker J, et al. Repeated observation of breast tumor subtypes in independent gene expression data sets. Proc Natl Acad Sci U S A 2003;100:8418-23. [Crossref] [PubMed]
- Hammerl D, Smid M, Timmermans AM, et al. Breast cancer genomics and immuno-oncological markers to guide immune therapies. Semin Cancer Biol 2018;52:178-88. [Crossref] [PubMed]
- Zhang X, Shen L, Cai R, et al. Comprehensive Analysis of the Immune-Oncology Targets and Immune Infiltrates of N (6)-Methyladenosine-Related Long Noncoding RNA Regulators in Breast Cancer. Front Cell Dev Biol 2021;9:686675. [Crossref] [PubMed]
- Denkert C, von Minckwitz G, Darb-Esfahani S, et al. Tumour-infiltrating lymphocytes and prognosis in different subtypes of breast cancer: a pooled analysis of 3771 patients treated with neoadjuvant therapy. Lancet Oncol 2018;19:40-50. [Crossref] [PubMed]
- Pruneri G, Vingiani A, Denkert C. Tumor infiltrating lymphocytes in early breast cancer. Breast 2018;37:207-14. [Crossref] [PubMed]
- Voorwerk L, Slagter M, Horlings HM, et al. Immune induction strategies in metastatic triple-negative breast cancer to enhance the sensitivity to PD-1 blockade: the TONIC trial. Nat Med 2019;25:920-8. [Crossref] [PubMed]
- Ribas A, Wolchok JD. Cancer immunotherapy using checkpoint blockade. Science 2018;359:1350-5. [Crossref] [PubMed]
- Mou Y, Wang J, Wu J, et al. Ferroptosis, a new form of cell death: opportunities and challenges in cancer. J Hematol Oncol 2019;12:34. [Crossref] [PubMed]
- Gao W, Wang X, Zhou Y, et al. Autophagy, ferroptosis, pyroptosis, and necroptosis in tumor immunotherapy. Signal Transduct Target Ther 2022;7:196. [Crossref] [PubMed]
- Wang W, Green M, Choi JE, et al. CD8(+) T cells regulate tumour ferroptosis during cancer immunotherapy. Nature 2019;569:270-4. [Crossref] [PubMed]
- Doll S, Freitas FP, Shah R, et al. FSP1 is a glutathione-independent ferroptosis suppressor. Nature 2019;575:693-8. [Crossref] [PubMed]
- Bersuker K, Hendricks JM, Li Z, et al. The CoQ oxidoreductase FSP1 acts parallel to GPX4 to inhibit ferroptosis. Nature 2019;575:688-92. [Crossref] [PubMed]
- Cerami E, Gao J, Dogrusoz U, et al. The cBio cancer genomics portal: an open platform for exploring multidimensional cancer genomics data. Cancer Discov 2012;2:401-4. [Crossref] [PubMed]
- Dedeurwaerder S, Desmedt C, Calonne E, et al. DNA methylation profiling reveals a predominant immune component in breast cancers. EMBO Mol Med 2011;3:726-41. [Crossref] [PubMed]
- Kao KJ, Chang KM, Hsu HC, et al. Correlation of microarray-based breast cancer molecular subtypes and clinical outcomes: implications for treatment optimization. BMC Cancer 2011;11:143. [Crossref] [PubMed]
- Li Y, Zou L, Li Q, et al. Amplification of LAPTM4B and YWHAZ contributes to chemotherapy resistance and recurrence of breast cancer. Nat Med 2010;16:214-8. [Crossref] [PubMed]
- Riaz N, Havel JJ, Makarov V, et al. Tumor and Microenvironment Evolution during Immunotherapy with Nivolumab. Cell 2017;171:934-949.e16. [Crossref] [PubMed]
- Love MI, Huber W, Anders S. Moderated estimation of fold change and dispersion for RNA-seq data with DESeq2. Genome Biol 2014;15:550. [Crossref] [PubMed]
- Friedman J, Hastie T, Tibshirani R. Regularization Paths for Generalized Linear Models via Coordinate Descent. J Stat Softw 2010;33:1-22.
- Blanche P, Dartigues JF, Jacqmin-Gadda H. Estimating and comparing time-dependent areas under receiver operating characteristic curves for censored event times with competing risks. Stat Med 2013;32:5381-97. [Crossref] [PubMed]
- Harrell FE Jr. (2023). _rms: Regression Modeling Strategies_. R package version 6.5-0. Available online: https://CRAN.R-project.org/package=rms
- Wu T, Hu E, Xu S, et al. clusterProfiler 4.0: A universal enrichment tool for interpreting omics data. Innovation (Camb) 2021;2:100141. [Crossref] [PubMed]
- Hänzelmann S, Castelo R, Guinney J. GSVA: gene set variation analysis for microarray and RNA-seq data. BMC Bioinformatics 2013;14:7. [Crossref] [PubMed]
- Angelova M, Charoentong P, Hackl H, et al. Characterization of the immunophenotypes and antigenomes of colorectal cancers reveals distinct tumor escape mechanisms and novel targets for immunotherapy. Genome Biol 2015;16:64. [Crossref] [PubMed]
- Bhattacharya S, Dunn P, Thomas CG, et al. ImmPort, toward repurposing of open access immunological assay data for translational and clinical research. Sci Data 2018;5:180015. [Crossref] [PubMed]
- Barkley D, Moncada R, Pour M, et al. Cancer cell states recur across tumor types and form specific interactions with the tumor microenvironment. Nat Genet 2022;54:1192-201. [Crossref] [PubMed]
- Yoshihara K, Kim H, Verhaak RG. (2016). _estimate: Estimate of Stromal and Immune Cells in Malignant Tumor Tissues from Expression Data_. R package version 1.0.13/r21. Available online: https://R-Forge.R-project.org/projects/estimate/
- Maeser D. (2021). _oncoPredict: Drug and Biomarker Discovery_. R package version 0.2. Available online: https://CRAN.R-project.org/package=oncoPredict
- Bertucci F, Ng CKY, Patsouris A, et al. Genomic characterization of metastatic breast cancers. Nature 2019;569:560-4. [Crossref] [PubMed]
- Sukumar J, Gast K, Quiroga D, et al. Triple-negative breast cancer: promising prognostic biomarkers currently in development. Expert Rev Anticancer Ther 2021;21:135-48. [Crossref] [PubMed]
- Li Y, Zhang H, Merkher Y, et al. Recent advances in therapeutic strategies for triple-negative breast cancer. J Hematol Oncol 2022;15:121. [Crossref] [PubMed]
- Song R, Li T, Ye J, et al. Acidity-Activatable Dynamic Nanoparticles Boosting Ferroptotic Cell Death for Immunotherapy of Cancer. Adv Mater 2021;33:e2101155. [Crossref] [PubMed]
- Lei G, Zhang Y, Koppula P, et al. The role of ferroptosis in ionizing radiation-induced cell death and tumor suppression. Cell Res 2020;30:146-62. [Crossref] [PubMed]
- Gwangwa MV, Joubert AM, Visagie MH. Crosstalk between the Warburg effect, redox regulation and autophagy induction in tumourigenesis. Cell Mol Biol Lett 2018;23:20. [Crossref] [PubMed]
- Dai E, Han L, Liu J, et al. Autophagy-dependent ferroptosis drives tumor-associated macrophage polarization via release and uptake of oncogenic KRAS protein. Autophagy 2020;16:2069-83. [Crossref] [PubMed]
- Butt AJ, Caldon CE, McNeil CM, et al. Cell cycle machinery: links with genesis and treatment of breast cancer. Adv Exp Med Biol 2008;630:189-205. [Crossref] [PubMed]
- Fang Z, Gao ZJ, Yu X, et al. Identification of a centrosome-related prognostic signature for breast cancer. Front Oncol 2023;13:1138049. [Crossref] [PubMed]
- Watkins SK, Egilmez NK, Suttles J, et al. IL-12 rapidly alters the functional profile of tumor-associated and tumor-infiltrating macrophages in vitro and in vivo. J Immunol 2007;178:1357-62. [Crossref] [PubMed]
- Chen S, Saeed AFUH, Liu Q, et al. Macrophages in immunoregulation and therapeutics. Signal Transduct Target Ther 2023;8:207. [Crossref] [PubMed]
- Gutiérrez-Melo N, Baumjohann D. T follicular helper cells in cancer. Trends Cancer 2023;9:309-25. [Crossref] [PubMed]
(English Language Editor: J. Gray)