Construction of a postoperative liver metastasis prediction model for colorectal cancer based on spectral CT imaging, CEA, and CA19-9
Highlight box
Key findings
• A nomogram model for predicting postoperative liver metastasis among patients with colorectal cancer (CRC) was established based on carcinoembryonic antigen (CEA), cancer antigen 19-9 (CA19-9), and preoperative spectral computed tomography (CT) parameters.
• The model demonstrated high predictive accuracy, with an area under the curve of 0.9078 in the training set and 0.9502 in the validation set.
• The model exhibited good clinical value, as confirmed by calibration and decision curve analyses.
What is known and what is new?
• Liver metastasis is a significant factor affecting prognosis in patients with CRC, and various biomarkers and imaging techniques have been investigated for predictive modeling.
• This study developed a novel predictive model based on spectral CT imaging parameters combined with tumor biomarkers, offering a promising tool for preoperative assessment of liver metastasis risk in patients with CRC.
What is the implication, and what should change now?
• The proposed nomogram model can help clinicians predict liver metastasis post-surgery, potentially leading to better individualized patient management and monitoring strategies.
• Further validation in larger, multicenter cohorts is necessary to confirm the model’s clinical utility and improve its integration into routine clinical practice.
Introduction
Colorectal cancer (CRC) ranks among the most prevalent cancers worldwide (1). In recent years, the prevalence of CRC in China has risen annually due to alterations in dietary patterns and an aging population. As per the 2022 data from the National Cancer Center of China, there are approximately 4.08 million new cases of CRC and 196,000 fatalities per year, making it the second most prevalent cancer and the fourth major cause of cancer-related mortality in the country (2,3). At present, radical surgery is the most effective treatment for CRC; however, patient prognosis remains suboptimal largely due to the high recurrence rates. The average 5-year overall survival rate is only 11% for patients with metastatic CRC, with distant metastasis being one of the leading contributors to poor prognoses among patients with CRC (4,5). The liver is the most often targeted organ in distant metastases. According to a report, almost 50% of individuals with CRC develop liver metastases shortly after surgery (6). Therefore, the preoperative identification of patients at high risk of liver metastasis is crucial for developing individualized treatment plans, reducing liver metastasis rates, improving treatment outcomes, and enhancing patient prognosis (7,8).
Existing prediction models for liver metastasis following CRC surgery often utilize clinical and histopathological factors; however, there are limitations in these models in terms of predictive accuracy and generalizability. The existing models also mostly rely predominantly on traditional imaging and specific tumor markers, which do not comprehensively account for the complex biological behavior of CRC metastasis. These limitations underline the clinical need for enhanced models that integrate more precise and varied data types. A common preoperative examination technique for patients with CRC is computed tomography (CT), which is used to evaluate the involvement of other tissues and organs, the size of lesions, and the status of regional lymph nodes. Contrast-enhanced CT further helps evaluate the blood supply characteristics of lesions, providing essential information for assessing the nature (9). Traditional CT primarily depends on visual observation, which restricts the beneficial effect garnered by the information acquired and limits the accuracy of the prediction of liver metastasis risk among patients with CRC. Spectral CT, a dual-energy CT technique, collects spectral data using both high-energy and low-energy X-rays, enabling the reconstruction of various spectral images, including virtual monoenergetic images, iodine density images, water-free iodine images, and virtual non-contrast images (10). Compared to conventional CT, spectral images provide detailed morphological information and allow for quantitative analysis, revealing tissue composition and blood supply characteristics of the lesion, thereby significantly improving disease diagnosis and risk prediction (11). Spectral CT has demonstrated considerable clinical value in tumor imaging. Integrating virtual native images with low-energy images enhances the detection and characterization of tumor lesions. In recent years, studies have applied spectral CT for the preoperative prediction of lymph node metastasis in CRC and have achieved a degree of success (12,13). The integration of spectral CT features with biological markers such as carcinoembryonic antigen (CEA) and cancer antigen 19-9 (CA19-9) is proposed as a method to potentially improve the accuracy of liver metastasis prediction. This combination leverages the detailed imaging capabilities of spectral CT with the proven tumor-associated biochemical markers, providing a multi-dimensional approach to risk assessment (14). This approach addresses the limitations of previous models by incorporating a broader range of data types and offering a more comprehensive analysis of tumor behavior before surgery.
The objective of this study was to construct a spectral CT-based predictive model to predict liver metastases among patients undergoing surgery for CRC. We present this article in accordance with the TRIPOD reporting checklist (available at https://tcr.amegroups.com/article/view/10.21037/tcr-2025-570/rc).
Methods
Study population
This study enrolled patients with CRC who underwent resection at Jiangsu Cancer Hospital between June 2020 and June 2022. The inclusion criteria were the following: (I) a pathologically confirmed diagnosis of CRC; (II) no history of chemotherapy, radiation therapy, nor other anticancer treatments before admission; (III) a follow-up period of at least 3 months; and (IV) informed consent to participate in the study. Meanwhile, the exclusion criteria were as follows: presence of hepatic and extrahepatic metastases at the time of initial diagnosis; a history of other malignancies; a pregnant or nursing state; with missing clinical data; an inability to cooperate with follow-up; and the emergence of local recurrence or distant metastasis to organs other than the liver during the follow-up period.
Based on the sample size estimation rule for logistic regression models, which recommends at least 10 cases per predictor variable to ensure sufficient model stability and power, we calculated the required sample size for our study. The model includes five predictor variables: CEA, CA19-9, and three spectral CT parameters. Therefore, following this rule, we initially estimated that a minimum of 50 cases (10 × 5 predictor variables) were required. A total of 100 patients were included. All patients underwent preoperative spectral CT enhanced scanning and radical CRC surgery within 3 weeks after the spectral CT examination. Postoperatively, all patients were followed up for 2 years, with imaging examinations [enhanced CT/magnetic resonance imaging (MRI)] conducted every 3 months during the follow-up period. The endpoint event was the occurrence of liver metastasis or the follow-up cutoff date. Data from lost-to-follow-up patients were excluded from the analysis. Patients were separated into two groups based on whether liver metastases occurred during the follow-up period: those with liver metastases (n=60) and non-metastasis group (n=40). The Chinese CRC Liver Metastasis Diagnosis and Comprehensive Treatment Guidelines (2023 Edition) criteria were used to diagnose liver metastases (15). The criteria include: (I) Imaging diagnosis: Enhanced CT: Venous phase scan reveals single or multiple low-density nodules in the liver with ring enhancement. MRI: High signal on T2-weighted imaging (T2WI), high signal on diffusion-weighted imaging (DWI), and arterial phase marginal enhancement on dynamic contrast-enhanced imaging. (II) Pathological confirmation (if necessary): Ultrasound-guided liver biopsy of atypical lesions for histological confirmation as metastatic adenocarcinoma.
The study was conducted in accordance with the Declaration of Helsinki (as revised in 2013). The study was approved by the ethics committee of the Jiangsu Cancer Hospital (No. KY-2024-054), and informed consent was taken from all the patients.
Scanning protocol and parameters
All patients underwent plain routine abdominal and enhanced scans with a dual-layer detector spectral CT scanner. The scan range included the diaphragm to the pubic symphysis. Before the scan, participants were required to fast for at least 4 to 6 hours and consume 800 mL of warm water 10 minutes prior to the scan. The scanning parameters were as follows: pitch, 0.985; collimator width, 64×0.625 mm; tube rotation speed, 0.5 s/r; tube voltage, 120 kVp; and an automatically adjusted tube current (mA) based on patient size and anatomy. Ioversol (350 mg I/mL) was the contrast agent applied, and it was administered at a dose of 1.2 mL/kg at a flow rate of 3 mL/s. The region of interest (ROI) on the abdominal aorta was selected to initiate the scan, and imaging was carried out 30 seconds after the venous-phase threshold of 120 Hounsfield units (HU) was reached.
Image processing and analysis
The accompanying virtual monoenergetic pictures, effective atomic number (Zeff), and iodine concentration (IC) maps were created by transferring the spectral CT venous phase images to a spectral post-processing workstation. ROIs were drawn on the most significant visible layer of the tumor and the same plane as the abdominal aorta or iliac artery, with the blood vessels and necrotic areas inside the tumor being avoided. The tumor ROI diameter was required to be more than half its thickness. Each lesion was measured three times, and the average value was taken. The parameters measured included the following: (I) the CT values of the tumor lesions on the 40-keV (CT40 keV), 70-keV (CT70 keV), and-100 keV (CT70 keV) monoenergetic images of the venous phase; (II) the spectral slope (λ) of the lesion in the venous phase [λHU = (CT40 keV – CT100 keV)/(100 – 40) HU]; (III) the IC of the lesion and the same-plane abdominal aorta or iliac artery; (IV) the normalized iodine concentration (NIC) of the lesion [NIC = IClesion/IC abdominal aorta (or external iliac artery)]; and (V) the Zeff of the lesion in the venous phase.
Statistical analysis
Normality of the data was evaluated using the Kolmogorov-Smirnov test. Group comparisons were performed using independent or paired t-tests. Normally distributed data were expressed as the mean ± standard deviation (χ±s) and non-normally distributed data as the median and interquartile range. The Mann-Whitney test or the Chi-squared test was used for group comparisons, and categorical data were expressed as counts and percentages. The prediction accuracy of quantitative spectral CT parameters for liver metastases was examined using the receiver operating characteristic (ROC) curve. A multivariable logistic regression analysis was conducted to identify independent risk variables for liver metastases following CRC surgery. The “rms“ package in R software (The R Foundation for Statistical Computing) was used to construct a nomogram prediction model for liver metastases following CRC surgery and the dataset was randomly divided into a training set (n=70) and a validation set (n=30) in a 7:3 ratio. The model’s performance was evaluated using ROC curves, calibration curves, and decision curve analysis (DCA). All hypothesis tests were two-sided, with a P value of <0.05 considered statistically significant. SPSS version 26.0 (IBM Corp., Armonk, NY, USA) was used to conduct the statistical analysis. Sensitivity = TP/(TP + FN). [TP (true positive) is the number of positive samples correctly identified. FN (false negative) is the number of positive samples incorrectly identified as negative]. Specificity = TN/(TN + FP). [TN (true negative) is the number of negative samples correctly identified by the model. FP (false positive) is the number of negative samples incorrectly identified as positive by the model].
Results
Comparison of preoperative quantitative spectral CT parameters between the two groups
The liver metastasis group had significantly higher venous-phase IClesion, λHU, and CT40keV values than the non-metastasis group (P<0.05 for all values). The venous-phase parameters of NIC, Zeff, CT70 keV, and CT100keV did not differ between the two groups (P>0.05; Table 1).
Table 1
Parameter (venous phase) | Non-metastasis group (n=40) | Liver metastasis group (n=60) | t | P |
---|---|---|---|---|
IClesion (mg/mL) | 1.25±0.37 | 1.64±0.36 | −5.26 | <0.001 |
NIC | 0.14±0.05 | 0.15±0.04 | −1.33 | 0.19 |
Zeff | 8.05±0.20 | 8.11±0.23 | −1.17 | 0.25 |
λHU | 1.47±0.48 | 2.16±0.72 | −5.75 | <0.001 |
CT40 keV (HU) | 143.52±27.08 | 188.00±43.24 | −6.32 | <0.001 |
CT70 keV (HU) | 77.04±13.06 | 76.76±11.34 | 0.11 | 0.91 |
CT100 keV (HU) | 55.25±11.98 | 58.40±10.63 | −1.38 | 0.17 |
Data are presented as mean ± standard deviation. CT, computed tomography; HU, Hounsfield units; IC, iodine concentration; NIC, normalized iodine concentration; Zeff, effective atomic number.
Preoperative spectral CT quantitative parameters for predicting CRC liver metastasis: ROC curve analysis
ROC curve analysis was used to evaluate the performance of venous-phase IClesion, λHU, and CT40 keV values in predicting liver metastasis among patients with CRC. The area under the curve (AUC) for IClesion, λHU, and CT40 keV was 0.7629, 0.7798, and 0.8008, respectively; the sensitivity was 73.33%, 60.00%, and 75.00%; and the specificity was 70.00%, 87.50%, and 77.50%, respectively (all P values <0.05). All three parameters demonstrated good clinical predictive value (Table 2 and Figure 1).
Table 2
Parameter (venous phase) | AUC (95% CI) | SE | P | Sensitivity (%) | Specificity (%) | Cutoff value | Youden index |
---|---|---|---|---|---|---|---|
IClesion (mg/mL) | 0.7629 (0.6703–0.8556) | 0.0473 | <0.001 | 73.33 | 70.00 | 1.43 | 43.33 |
λHU | 0.7798 (0.6909–0.8687) | 0.0454 | <0.001 | 60.00 | 87.50 | 1.97 | 47.50 |
CT40 keV (HU) | 0.8008 (0.7161–0.8856) | 0.0433 | <0.001 | 75.00 | 77.50 | 163.14 | 52.50 |
AUC, area under the curve; CI, confidence interval; CRC, colorectal cancer; CT, computed tomography; HU, Hounsfield units; IC, iodine concentration; SE, standard error.
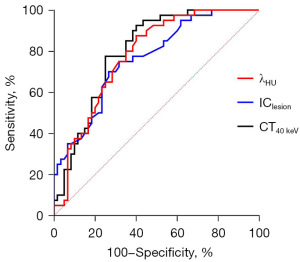
Comparison of preoperative clinical and pathological characteristics between the two groups
The liver metastasis group, compared to the non-liver metastasis group, had a significantly higher percentage of patients with tumor-node-metastasis (TNM) stage III–IV, CEA levels ≥5 ng/mL, CA19-9 levels ≥37 U/mL, extramural vascular invasion, and vascular cancer thrombi (P<0.05). The two groups showed no significant differences relative to the other clinical and pathological features (P>0.05; Table 3).
Table 3
General information | Non-metastasis group (n=40) | Liver metastasis group (n=60) | χ2 | P |
---|---|---|---|---|
Age | ||||
<60 years | 19 (47.50) | 31 (51.67) | 0.1667 | 0.68 |
≥60 years | 21 (52.50) | 29 (48.33) | – | – |
Sex | ||||
Male | 24 (60.00) | 36 (60.00) | 0.0000 | >0.99 |
Female | 16 (40.00) | 24 (40.00) | – | – |
TNM staging | ||||
Stage I–II | 25 (62.50) | 16 (26.67) | 12.74 | <0.001 |
Stage III–IV | 15 (37.50) | 44 (73.33) | – | – |
Tumor location | ||||
Rectum | 22 (55.00) | 40 (66.67) | 1.387 | 0.24 |
Colon | 18 (45.00) | 20 (33.33) | – | – |
Histological type | ||||
Adenocarcinoma | 31 (77.50) | 42 (70.00) | 0.6849 | 0.41 |
Signet ring cell carcinoma | 9 (22.50) | 18 (30.00) | – | – |
BMI | ||||
<24 kg/m2 | 31 (77.50) | 41 (68.33) | 1.000 | 0.32 |
≥24 kg/m2 | 9 (22.50) | 19 (31.67) | – | – |
CEA | ||||
<5 ng/mL | 24 (60.00) | 17 (28.33) | 9.949 | 0.002 |
≥5 ng/mL | 16 (40.00) | 43 (71.67) | – | – |
CA19-9 | ||||
<37 U/mL | 23 (57.50) | 17 (28.33) | 8.507 | 0.004 |
≥37 U/mL | 17 (42.50) | 43 (71.67) | – | – |
Extramural vascular invasion | ||||
No | 23 (57.50) | 17 (28.33) | 8.507 | 0.004 |
Yes | 17 (42.50) | 43 (71.67) | – | – |
Tumor diameter | ||||
<5 cm | 23 (57.50) | 28 (46.67) | 1.127 | 0.29 |
≥5 cm | 17 (42.50) | 32 (53.33) | – | – |
History of hypertension | ||||
No | 28 (70.00) | 44 (73.33) | 0.1323 | 0.72 |
Yes | 12 (30.00) | 16 (26.67) | – | – |
Tumor differentiation | ||||
Poorly differentiated | 18 (45.00) | 35 (58.33) | 1.713 | 0.19 |
Moderately/highly differentiated | 22 (55.00) | 25 (41.67) | – | – |
Vascular cancer thrombosis | ||||
No | 23 (57.50) | 17 (28.33) | 8.507 | 0.004 |
Yes | 17 (42.50) | 43 (71.67) | – | – |
Nerve invasion | ||||
No | 24 (60.00) | 30 (50.00) | 0.9662 | 0.33 |
Yes | 16 (40.00) | 30 (50.00) | – | – |
Family history of cancer | ||||
No | 30 (75.00) | 51 (85.00) | 1.559 | 0.21 |
Yes | 10 (25.00) | 9 (15.00) | – | – |
Data are presented as n (%). BMI, body mass index; CA19-9, cancer antigen 19-9; CEA, carcinoembryonic antigen; TNM, tumor-node-metastasis.
Construction of a predictive nomogram model for liver metastasis among patients with CRC following surgery
Examination of independent risk variables for liver metastasis among individuals with CRC following surgery was performed. The development of liver metastases within 2 years after surgery was the dependent variable (no =0 and yes =1), while the aforementioned significant covariates were regarded as independent variables. The multivariate logistic regression analysis identified venous-phase parameters (IClesion, λHU, and CT40 keV), CEA, and CA19-9 as independent risk factors for liver metastases following surgery among patients with CRC (Table 4).
Table 4
Influencing factors | β | SE | Wald χ2 | P | Exp(B) | 95% CI |
---|---|---|---|---|---|---|
TNM stage | 1.0770 | 0.6847 | 2.4744 | 0.12 | 2.9358 | 0.7673–11.2333 |
CEA | 1.8682 | 0.6977 | 7.1697 | 0.007 | 6.4768 | 1.6499–25.4250 |
CA19-9 | 2.2783 | 0.7816 | 8.4972 | 0.004 | 9.7596 | 2.1094–45.1543 |
Extramural vascular invasion | −2.3785 | 2.2643 | 1.1034 | 0.29 | 0.0927 | 0.0011–7.8411 |
Vascular cancer thrombosis | 1.9248 | 2.1614 | 0.7930 | 0.37 | 6.8540 | 0.0991–473.9910 |
IClesion | 1.7035 | 0.6522 | 6.8214 | 0.009 | 5.4932 | 1.5298–19.7246 |
λHU | 2.0347 | 0.8451 | 5.7959 | 0.02 | 7.6496 | 1.4597–40.0896 |
CT40 keV | 1.6517 | 0.7260 | 5.1760 | 0.03 | 5.2159 | 1.2571–21.6422 |
CA19-9, cancer antigen 19-9; CEA, carcinoembryonic antigen; CRC, colorectal cancer; CT, computed tomography; HU, Hounsfield units; IC, iodine concentration; TNM, tumor-node-metastasis.
Nomogram development
The R package “rms” was used to randomly allocate the 100 included patients into a training and a validation set in a 7:3 ratio based on the findings of the logistic multivariate analysis. A predictive nomogram model was subsequently developed. This model predicted the risk of developing liver metastases among individuals with CRC following surgery (Figure 2).
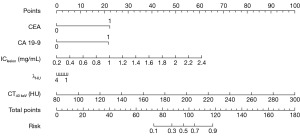
The ROC curve for this nomogram model was plotted (Figure 3). The AUC in the training dataset was 0.9078 [95% confidence interval (CI): 0.8419–0.9738], the sensitivity was 88.89% (95% CI: 77.03–100.00%), and the specificity was 83.72% (95% CI: 72.69–94.76%). Internal validation of the nomogram was conducted using 1000-bootstrap analyses, yielding an AUC of 0.9502 (95% CI: 0.8792–1.0000), a sensitivity of 84.62% (95% CI: 65.00–100.00%), and a specificity of 82.35% (95% CI: 64.23–100.00%), indicating that the model demonstrated excellent accuracy. The detailed results are provided in Table 5.
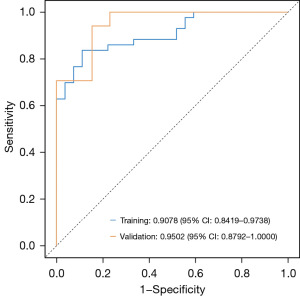
Table 5
Data | AUC (95% CI) | Sensitivity (95% CI) (%) | Specificity (95% CI) (%) | Cutoff |
---|---|---|---|---|
Training | 0.9078 (0.8419–0.9738) | 88.89 (77.03–100.00) | 83.72 (72.69–94.76) | 0.6460 |
Test | 0.9502 (0.8792–1.0000) | 84.62 (65.00–100.00) | 82.35 (64.23–100.00) | 0.6460 |
AUC, area under the curve; CI, confidence interval; ROC, receiver operating characteristic.
This model’s cutoff value was set at 0.6460. A risk score of ≥0.6460 indicates a high chance of liver metastases following surgery, whereas a score of <0.6460 indicates a low risk. The following is an example of the nomogram’s application: A CRC patient had a preoperative CEA level of 32.54 ng/mL (greater than 5 ng/mL), a CA19-9 level of 121.05 U/mL (greater than 37 U/mL), an IClesion value of 0.88 mg/mL, a λHU value of 1.21, and a CT40 keV value of 120.33 HU; the corresponding “Points” values for these parameters in the nomogram were as follows: CEA, 22.5; CA19-9, 22.0; IClesion, 16.5; λHU, 3.1; and CT40 keV, 17.1. These values were summed to yield a total score of 81.2 and then located on the “Total Points” scale. Finally, the corresponding value on the “Risk” axis was approximately 0.28. The model indicated that this patient had a low chance of liver metastases within 2 years following surgery since 0.28<0.6460.
Decision curve and calibration curve
The model-fitting indicator was the Hosmer-Lemeshow test (H-L test). There was no discernible difference between the expected and actual numbers when the P value exceeded 0.05. The findings of the H-L test result for the model (χ²=5.572, P=0.70) suggested that the model had good goodness of fit. Furthermore, the calibration curve demonstrated that, on average, the projected and actual probability matched (Figure 4A).
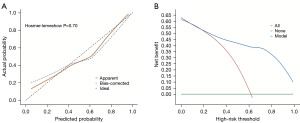
Decision curve analysis (DCA) is a method for evaluating the clinical effectiveness of predictive models. The DCA of the nomogram for liver metastasis risk demonstrated that within the threshold range of 0 to 1, the nomogram model offers a good clinical net benefit for identifying individuals at risk of liver metastasis following CRC surgery in comparison to the situations where all patients have liver metastases (red diagonal line in Figure 4B) or none have liver metastases (green horizontal line in Figure 4B).
Discussion
An emerging imaging technology known as spectral CT expands on the single-parameter scanning mode of conventional CT by providing multiple quantitative parameters, including Zeff images, material decomposition images (such as iodine- or water-based), and monochromatic images at 40–140 keV (16,17). Consequently, spectral CT has been widely used in the diagnosis and treatment of clinical practice in CRC, including prognostic assessment and malignant lymph node identification (18-20). In addition, spectral CT has shown that certain specific parameters in the early assessment of patients with CRC are correlated with the risk of metastasis after treatment and possess certain predictive value. According to Cao et al. (21), in preoperative spectral CT parameters, ICAP [iodine concentration (IC) values in the arterial phase], ICVP (IC value in the venous phase), and Eff ZVP (effective atomic number in the venous phase) are significantly correlated with CRC lymph node metastasis and are independent predictors. The results of our study indicated that the levels of IClesion, λHU, and CT40 keV parameters in the venous phase of the preoperative liver metastasis group of patients with CRC were independently associated with the development of postoperative liver metastasis following CRC resection. It has been noted that tumor cells with metastatic potential often show stronger proliferative ability and metabolic activity. This hypermetabolic state increases the tumor’s demand for oxygen and nutrients, which induces neovascularization and an increase in microvascular density, resulting in a significant enhancement of local blood supply. This characteristic can be reflected by spectral CT as an increase in IClesion, λHU, and CT40 keV parameters (22), which is consistent with the results of this study. ROC curve analysis also showed that preoperative IClesion, λHU, and CT40 keV values in the venous stage had good predictive efficacy for postoperative liver metastasis among patients with CRC. These results provide strong evidence supporting the use of preoperative spectral CT for predicting postoperative liver metastasis among patients with CRC following resection.
A statistical model that visualizes the correlation between several risk variables, tumor occurrence, and/or prognosis is referred to as a nomogram. Converting complex regression equations into an intuitive graphical representation can aid clinicians in assessing the probability of a given outcome event and improve the readability of prediction model findings (23). Cao et al. (21) developed a nomogram that combines clinical risk factors (CEA, CA19-9, and rectal perivisceral fat invasion) and spectral CT parameters (arterial phase IC, venous phase IC, and Zeff) for preoperative prediction of lymph node metastasis among patients with CRC. Moreover, Feng et al. (24) devised an effective noninvasive predictive nomogram based on spectral CT parameters (IC, NIC, and Zeff) to detect occult peritoneal metastasis among patients with advanced gastric cancer, increasing the preoperative detection rate. In our study, we constructed a nomogram to predict the risk of liver metastasis after CRC surgery based on five risk factors: CEA, CA19-9, and the preoperative venous-phase parameters IClesion, λHU, and CT40 keV. Excellent prediction performance was indicated by the training and validation sets’ respective AUC values (both >0.9). The H-L test and calibration curve indicated good consistency between the actual and predicted risk, supporting the reliability and reproducibility of the model. The nomogram prediction model’s therapeutic value was further validated via DCA. This model offers several benefits: CEA and CA19-9 are both commonly used tumor markers in clinical practice, and high levels of CEA and CA19-9 suggest an increased risk of liver metastasis (25). Among the spectral CT parameters, IC reflects the density and blood flow distribution of tumor neovascularity, while λHU and CT40 keV reflect the metabolic activity and hemodynamic characteristics of tumor tissues (26,27). The nomogram prediction model composed of these five indices could comprehensively account for the factors affecting liver metastasis in multiple dimensions, more accurately reflect the biological characteristics and metastasis risk of tumors, and thus improve the prediction accuracy.
However, certain limitations to this model should be noted. For instance, the lack of external validation limits the assessment of its generalizability. In the future, external validation using datasets from sources different from the training set will be performed to further optimize the model. Additionally, our study, being a single-center retrospective design, is subject to inherent biases such as selection bias and information bias, which could influence the results. The limited statistical power resulting from the small sample size also poses a challenge. Another consideration is the variability in the production of cancer antigen markers such as CA19-9. Not all patients produce CA19-9, which might affect the predictive accuracy of our model in a broader population. Future studies could explore alternative markers or a combination of markers to accommodate such variability.
Conclusions
The nomogram model established based on preoperative spectral CT parameters, CEA and CA19-9 has the potential to predict postoperative liver metastasis among patients with CRC following resection. It can accurately assess the risk of postoperative metastasis; provide a scientific basis for early screening, individualized diagnosis and treatment, and postoperative management; and further promote the development of precision medicine.
Acknowledgments
None.
Footnote
Reporting Checklist: The authors have completed the TRIPOD reporting checklist. Available at https://tcr.amegroups.com/article/view/10.21037/tcr-2025-570/rc
Data Sharing Statement: Available at https://tcr.amegroups.com/article/view/10.21037/tcr-2025-570/dss
Peer Review File: Available at https://tcr.amegroups.com/article/view/10.21037/tcr-2025-570/prf
Funding: None.
Conflicts of Interest: All authors have completed the ICMJE uniform disclosure form (available at https://tcr.amegroups.com/article/view/10.21037/tcr-2025-570/coif). D.I.T. serves as an unpaid editorial board member of Translational Cancer Research from May 2024 to December 2026. The other authors have no conflicts of interest to declare.
Ethical Statement: The authors are accountable for all aspects of the work in ensuring that questions related to the accuracy or integrity of any part of the work are appropriately investigated and resolved. The study was conducted in accordance with the Declaration of Helsinki (as revised in 2013). The study was approved by the ethics committee of the Jiangsu Cancer Hospital (No. KY-2024-054), and informed consent was taken from all the patients.
Open Access Statement: This is an Open Access article distributed in accordance with the Creative Commons Attribution-NonCommercial-NoDerivs 4.0 International License (CC BY-NC-ND 4.0), which permits the non-commercial replication and distribution of the article with the strict proviso that no changes or edits are made and the original work is properly cited (including links to both the formal publication through the relevant DOI and the license). See: https://creativecommons.org/licenses/by-nc-nd/4.0/.
References
- Ruan Y, Lu G, Yu Y, et al. PF-04449913 Inhibits Proliferation and Metastasis of Colorectal Cancer Cells by Down-regulating MMP9 Expression through the ERK/p65 Pathway. Curr Mol Pharmaco. 2023. [Epub ahead of print]. doi:
10.2174/1874467217666230915125622 . - Pérez-Santiago L, Dorcaratto D, Garcés-Albir M, et al. The actual management of colorectal liver metastases. Minerva Chir 2020;75:328-44. [Crossref] [PubMed]
- Nemati M, Rasmi Y, Rezaie J. Therapeutic application of mesenchymal stem cells-derived extracellular vesicles in colorectal cancer. Biocell 2023;47:455-64.
- Zheng R, Zhang S, Zeng H, et al. Cancer incidence and mortality in China, 2016. J Natl Cancer Cent 2022;2:1-9. [Crossref] [PubMed]
- Xu Y, Li H, You G. CMTM6 deletion affects chemoresistance and macrophage M2 polarization in colorectal cancer cells. Biocell 2024;48:229-37.
- Giannis D, Sideris G, Kakos CD, et al. The role of liver transplantation for colorectal liver metastases: A systematic review and pooled analysis. Transplant Rev (Orlando) 2020;34:100570. [Crossref] [PubMed]
- Xing Y, Yu G, Jiang Z, et al. Development of prediction models for liver metastasis in colorectal cancer based on machine learning: a population-level study. Transl Cancer Res 2024;13:5943-52. [Crossref] [PubMed]
- Zhou B, Sun M, Yang M, et al. The current status and reflections on 3D in vitro modeling of liver metastasis in colorectal cancer. Hepatobiliary Surg Nutr 2024;13:180-3. [Crossref] [PubMed]
- Hong Y, Zhong L, Lv X, et al. Application of spectral CT in diagnosis, classification and prognostic monitoring of gastrointestinal cancers: progress, limitations and prospects. Front Mol Biosci 2023;10:1284549. [Crossref] [PubMed]
- Lu W, Tan X, Zhong Y, et al. Spectral CT in the evaluation of perineural invasion status in rectal cancer. Jpn J Radiol 2024;42:1012-20. [Crossref] [PubMed]
- Clancy NT, Jones G, Maier-Hein L, et al. Surgical spectral imaging. Med Image Anal 2020;63:101699. [Crossref] [PubMed]
- Sauerbeck J, Adam G, Meyer M. Spectral CT in Oncology. Rofo 2023;195:21-9. [Crossref] [PubMed]
- Li Q, Hong R, Zhang P, et al. A clinical-radiomics nomogram based on spectral CT multi-parameter images for preoperative prediction of lymph node metastasis in colorectal cancer. Clin Exp Metastasis 2024;41:639-53. [Crossref] [PubMed]
- Peng W, Wan L, Zhao R, et al. Novel biomarkers based on dual-energy computed tomography for risk stratification of very early distant metastasis in colorectal cancer after surgery. Quant Imaging Med Surg 2024;14:618-32. [Crossref] [PubMed]
- Chinese College of Surgeons. Section of Colorectal Surgery, Branch of Surgery, Chinese Medical Association. China guideline for diagnosis and comprehensive treatment of colorectal liver metastases (version 2023). Zhonghua Wei Chang Wai Ke Za Zhi 2023;26:1-15. [Crossref] [PubMed]
- So A, Nicolaou S. Spectral Computed Tomography: Fundamental Principles and Recent Developments. Korean J Radiol 2021;22:86-96. [Crossref] [PubMed]
- Kruis MF. Improving radiation physics, tumor visualisation, and treatment quantification in radiotherapy with spectral or dual-energy CT. J Appl Clin Med Phys 2022;23:e13468. [Crossref] [PubMed]
- Li S, Yuan L, Yue M, et al. Early evaluation of liver metastasis using spectral CT to predict outcome in patients with colorectal cancer treated with FOLFOXIRI and bevacizumab. Cancer Imaging 2023;23:30. [Crossref] [PubMed]
- Luo M, Chen G, Xie H, et al. Preoperative diagnosis of metastatic lymph nodes by CT-histopathologic matching analysis in gastric adenocarcinoma using dual-layer spectral detector CT. Eur Radiol 2023;33:8948-56. [Crossref] [PubMed]
- Chen Y, Liu X, Zeng H, et al. The clinical applications of dual-layer spectral detector CT in digestive system diseases. Eur Radiol 2024; Epub ahead of print. [Crossref]
- Cao Y, Zhang J, Bao H, et al. Development of a Nomogram Combining Clinical Risk Factors and Dual-Energy Spectral CT Parameters for the Preoperative Prediction of Lymph Node Metastasis in Patients With Colorectal Cancer. Front Oncol 2021;11:689176. [Crossref] [PubMed]
- Lv J, Li X, Mu R, et al. Comparison of the diagnostic efficacy between imaging features and iodine density values for predicting microvascular invasion in hepatocellular carcinoma. Front Oncol 2024;14:1437347. [Crossref] [PubMed]
- Luo B, Yang M, Han Z, et al. Establishment of a Nomogram-Based Prognostic Model (LASSO-COX Regression) for Predicting Progression-Free Survival of Primary Non-Small Cell Lung Cancer Patients Treated with Adjuvant Chinese Herbal Medicines Therapy: A Retrospective Study of Case Series. Front Oncol 2022;12:882278. [Crossref] [PubMed]
- Feng QX, Zhu ZN, Li Q, et al. Dual-energy CT quantitative parameters to evaluate occult peritoneal metastasis in advanced gastric cancer preoperatively. Abdom Radiol (NY) 2024;49:3309-18. [Crossref] [PubMed]
- Gong LZ, Wang QW, Zhu JW. The combined detection of carcinoembryonic antigen, carcinogenic antigen 125, and carcinogenic antigen 19-9 in colorectal cancer patients. World J Gastrointest Surg 2024;16:2073-9. [Crossref] [PubMed]
- Deng L, Yang J, Ren T, et al. Can spectral computed tomography (CT) replace perfusion CT to assess the histological classification of non-small cell lung cancer? Quant Imaging Med Surg 2023;13:4960-72. [Crossref] [PubMed]
- Zhao L, Zhou W, Fu Y, et al. Diagnostic value of one-stop CT energy spectrum and perfusion for angiogenesis in colon and rectum cancer. BMC Med Imaging 2024;24:116. [Crossref] [PubMed]
(English Language Editor: J. Gray)